Abstract
This work presents a review and perspectives on recent developments in the use of machine learning (ML) to augment Reynolds-averaged Navier-Stokes (RANS) and large eddy simulation (LES) models of turbulent flows. Different approaches of applying supervised learning to represent unclosed terms, model discrepancies, and subfilter scales are discussed in the context of RANS and LES modeling. Particular emphasis is placed on the impact of the training procedure on the consistency of ML augmentations with the underlying physical model. Techniques to promote model-consistent training, and to avoid the requirement of full fields of direct numerical simulation data are detailed. This is followed by a discussion of physics-informed and mathematical considerations on the choice of the feature space, and imposition of constraints on the ML model. With a view towards developing generalizable ML-augmented RANS and LES models, outstanding challenges are discussed, and perspectives are provided. While the promise of ML-augmented turbulence modeling is clear, and successes have been demonstrated in isolated scenarios, a general consensus of this paper is that truly generalizable models require model-consistent training with careful characterization of underlying assumptions and imposition of physically and mathematically informed priors and constraints to account for the inevitable shortage of data relevant to predictions of interest. Thus, machine learning should be viewed as one tool in the turbulence modeler's toolkit. This modeling endeavor requires multidisciplinary advances, and thus the target audience for this paper is the fluid mechanics community, as well as the computational science and machine learning communities.
- Received 14 September 2020
- Accepted 6 January 2021
DOI:https://doi.org/10.1103/PhysRevFluids.6.050504
©2021 American Physical Society
Physics Subject Headings (PhySH)
Collections
This article appears in the following collection:
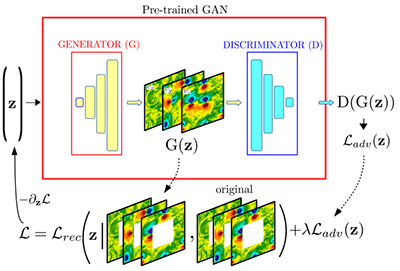
Machine Learning in Fluid Mechanics Invited Papers
Physical Review Fluids publishes a collection of invited papers which advance the use of machine learning in fluid mechanics.