A clinical nomogram incorporating salivary Desulfovibrio desulfuricans level and oral hygiene index for predicting colorectal cancer
Introduction
Colorectal cancer (CRC) is a major public health problem with a high incidence and mortality rate (1). In China, there are approximately 376,300 newly diagnosed CRC cases and 191,000 CRC-related deaths annually (2). Early identification of CRC can significantly reduce cancer incidence and mortality rates (3). Traditional CRC screening methods include testing for serum carcinoembryonic antigen (CEA), colonoscopy, and a fecal occult bleeding test (FOBT), all of which are widely used in clinical practice. However, these screening methods either lack sensitivity or are invasive and have low patient compliance (4,5). Thus, the search for a novel non-invasive biomarker with higher sensitivity and patient compliance is warranted.
Typically, the progression of colorectal tumorigenesis from a precancerous adenoma to CRC involves several stepwise genetic events (6). A previous study has reported that microbiota dysbiosis plays a pivotal role in the initiation and development of CRC and that the fecal microbiome can act as a biomarker for diagnosing CRC (7). Given the concordance and relevance between the oral and gut microbiome (8), many researchers have started to explore the structure and function of the oral microbiome in CRC (9). Nevertheless, these studies lack extensive investigation of the clinical parameters and oral health status of patients. Also, the detection of certain oral bacteria using a qPCR-based technique has not yet been reported.
Poor oral hygiene has been demonstrated to be associated with a higher risk of oral cancer, as well as head and neck cancer (10,11). Additionally, one study conducted in Austria reported that head and neck squamous cell carcinoma patients presented with significant dental care needs, but most patients underprioritized this (12). Oral hygiene indicators comprise several parameters, including tooth-brushing frequency, number of teeth lost, the regularity of dental visits, and presence of dental caries (11).
This study measured salivary microbial biomarkers that have previously been identified as playing a role in carcinogenesis. Additionally, we assessed the oral hygiene status of each patient. Overall, this study aimed to establish a predictive model of the risk of developing CRC based on clinical characteristics, oral hygiene indicators, and the salivary microbiome. We hypothesized that the combined use of an oral hygiene index (OHI) with salivary biomarker abundance levels could maximize this model’s prediction accuracy in diagnosing CRC. We present the following article in accordance with the TRIPOD reporting checklist (available at http://dx.doi.org/10.21037/atm-20-8168).
Methods
Patient selection and sample collection
Consecutive patients who underwent colonoscopy or surgical resections in Shanghai Jiao Tong University, affiliated with Renji Hospital, were enrolled in this study from January 2018 to January 2020. Patients that underwent colonoscopy and were confirmed to have no neoplasms or pathologically diagnosed adenocarcinoma were assigned to either a healthy control (HC) or CRC group, respectively. Subjects were excluded if they demonstrated any of the following: (I) a history of gastrointestinal neoplasia; (II) a history of upper gastrointestinal tract surgery; (III) specific types of CRCs including Lynch Syndrome, familial adenomatous polyposis (FAP), and Peutz-Jeghers syndromes; (IV) treatment with chemotherapy or radiotherapy; (V) use of any of the following drugs in the month before enrolment: nonsteroidal anti-inflammatory drugs (NSAIDs), immuno-inhibitors, antibiotics, or probiotics. The workflow chart is shown in Figure 1. A total number of 237 patients were included in the final statistical analysis. Before undergoing their scheduled operation, approximately 500 µL–2 mL unstimulated saliva were collected from each patient. Patients were restrained from using oral hygiene cleaners, eating or drinking for 2 hours. Saliva was transferred to a sterilized EP tube and further stored in −80 °C. The procedures were all performed by specialized doctors who were blind to the research content. This study received approval from the Renji Hospital Ethics Committee, and informed consent was obtained from each patient. The study protocol conforms to the ethical guidelines of the 1975 Declaration of Helsinki (as revised in 2013) as reflected by a priori approval by the ethics committee of Renji Hospital (No.: 2018RJ-033).
Data collection
Patient demographics, including age, sex, body weight, height, tobacco and alcohol use, and comorbidities, were retrieved from the hospital medical system or obtained using face-to-face questionnaires. Body mass index (BMI) was calculated as weight (kg) divided by height (m) squared. Patients’ self-reported oral hygiene information was obtained from questionnaires recorded by doctors, a method which has proven to be reliable (13,14). Due to restrictions in dental assessments availability, an OHI was constructed based on three variables (15), with minor modifications. The three variables were scored categorically as follows: the number of teeth lost ≥5, score =1; tooth-brushing frequency <twice/day, score =1; and irregular dental visits, score =1. Otherwise, the variable was scored 0. The sum of the three variables equaled the total OHI score, ranging from 0 to 3. The presence of dental caries was defined as a categorical variable. Tobacco or alcohol use was defined as consumption in the past six months. Subjects with no use or past consumption were defined as non-users. Comorbidities such as diabetes or hypertension were also recorded as categorical variables.
Genomic DNA extraction and quantitative polymerase chain reaction (qPCR)
After the saliva was thawed, the genomic DNA was extracted according to the manufacturer’s instructions using a QIAamp DNA mini kit (Qiagen, Germany). Upon DNA extraction, the purity and concentration of the DNA were detected by a Nanodrop 2000 spectrophotometer (Thermofisher Scientific, America) and stored at −20 °C for further use. Primers for the salivary microbial biomarker quantification were identified from previous literature (16,17) or designed via Primer-BLAST in NCBI (National Center for Biotechnology Information) by targeting the flavodoxin gene and hemolysin gene, respectively (18,19). Sequences of the primers used in this study are detailed in the Table S1. qPCR was performed in a 10 µL reaction system of SYBR green mix in an ABI thermocycler (Thermofisher, USA). The qPCR reactions were performed in duplicates for each target using 16S rRNA as the internal control, which has previously been shown to indicate total bacterial DNA load (20). Salivary genomic DNA with a final concentration of 10 ng/µL was used for the qPCR assay per well. The qPCR conditions included denaturation at 95 °C for 5 s, annealing, and extension at 60 °C for 30 s for a total of 40 cycles, after a short pre-denaturation at 95 °C for 30 s, which was consistent with a previous study conducted in our institute (16).
Data processing and statistical analysis
For undetermined qPCR readouts, the Ct values were replaced with a maximum value of 40. The relative abundance of target salivary microbial biomarkers was denoted as the 16S rRNA Ct value subtracted from the target Ct value. The Kolmogorov-Smirnov normality test was first applied to assess the distribution of continuous data. Values were expressed as mean ± standard deviation (SD), median with interquartile range (IQR), or whole number with percentage, as appropriate. Univariate comparisons of clinical characteristics and oral conditions between the CRC and HC patients were conducted using independent t-tests (normal distribution) or the Wilcoxon-Mann Whitney U nonparametric test (non-normal distribution). The chi-square test was used for the comparison of categorical variables. Factors independently associated with CRC were selected using multivariate logistic analysis with forwarding selection. All statistical analyses were performed with SPSS version 25 (SPSS Inc., Armonk, NY, USA). There were missing data on the salivary microbiome as some patients had limited volumes of saliva. Some data on BMI (0.4%), dental caries (2.5%), and salivary microbiome level [2.5% of data for Fusobacterium nucleatum (Fn), 2.1% for Porphyromonas gingivalis (Pg), 0.4% for Desulfovibrio desulfuricans (Dd), and 2.1% for Prevotella melaninogenica (Pm)] were missing and were compared separately. These data were multiply imputed with the mean or median when analyzed in the multivariate logistic analysis according to Rubin’s rule (21). A Variance Inflation Factor (VIF) was used to assess the collinearity between variables. A P value ≤0.05 was considered significant.
Internal validation of the predictive model and the nomogram creation
Bootstrapping with 237 repetitions was used for the internal validation, and bias-corrected accuracy measures of our predictive model were obtained. The Brier score was used to assess the difference between observed and predicted values, with a value closer to 0 indicating better predictive ability. By contrast, the Hosmer-Lemeshow goodness of fit test is an overall assessment of the difference between the predicted and actual values, with a P value ≥0.05 indicating no difference and good calibration ability. The calibration curve reflects the agreement between the observed and predicted values, with a value closer to 1 indicating better performance. The Concordance-index (C-index) was used to assess the model’s discriminatory ability with values ranging between 0.5 and 1 (22). A nomogram was constructed by R Software (R Foundation for Statistical Computing, Vienna, Austria) based on the odds ratio (OR) calculated from the multivariate logistic analysis. The uppermost line represents the reference for scoring points from 0 to 100. The predictive variables are displayed below with regularly segmented bars that visually demonstrate each variable’s relative weight. The values are assigned to each variable accordingly, with total points for the nomogram and the corresponding predicted probability of CRC being shown in the bottom two bars.
Results
Patient characteristics and oral hygiene indicators
A total number of 237 patients met our inclusion criteria and were incorporated in the final cohort, including 95 HC and 142 CRC patients (Figure 1). The patients’ overall characteristics, oral hygiene indicators, and microbial biomarker levels are described as follows: The average age at diagnosis was 59.49±12.48 years, and sex was approximately evenly distributed with a slight preponderance of males (53.16%). The comorbidity rate for hypertension and type 2 diabetes in the cohort was 32.07% and 10.13%, respectively. There were no significant differences between the two groups in patient BMI and alcohol drinking status (P>0.05). However, there were significant differences with regards to age, sex, OHI scores, the presence of dental caries, comorbidities, and smoking status (P<0.05). The average age at diagnosis was 51.16±10.75 years for the HC group, while the CRC group was significantly older with an average age of 65.07±10.27 years. The HC group had a lower proportion of males (44.21% vs. 59.15%, P=0.024). The CRC group had an OHI median value of 2, which was higher than that of the HC group, which had a median value of 1. This indicated that the CRC group had worse oral hygiene habits in comparison with the HC group. Furthermore, a greater proportion of CRC patients had dental caries, comorbidities for type 2 diabetes, hypertension, and smoking status, as demonstrated in Table 1.
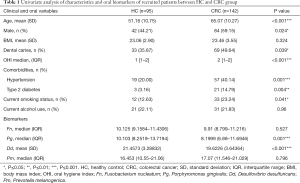
Full table
Biomarker comparisons
The current study selected four pathogens as qPCR targets in the saliva, namely Fn, Pg, Dd, and Pm. Next, we compared these salivary microbial biomarkers between the two groups. The relative abundance of salivary Fn and Pm were not significantly different between the two groups. On the other hand, the relative abundance of salivary Pg and Dd in the CRC group was greater than that in the HC group. Scatter plots of comparisons for the relative abundance of salivary Pg and Dd between the HC and CRC groups are shown in Figure 2. The median relative Pg Ct values were 10.10 and 8.20, while mean Dd Ct values were 21.46 and 19.62 for HC and CRC groups, respectively. The optimal cut-off points for salivary Pg or Dd for discriminating CRC from HC were 8.31 and 21.95, respectively. Accordingly, the area under the receiver operating curve (AUC) was 0.629 for Pg and 0.653 for Dd. Among all 237 samples, we identified a positive correlation between the OHI and the salivary Pg or Dd relative abundance (Spearman correlation coefficient =0.188 and 0.133, P=0.004 and 0.04, respectively). We detected the presence of sulfate-reducing bacteria (SRB), including Dd, in the periodontal pocket, and Dd co-occurred with Pg. Co-occurrence of Pg and Dd was reduced after treatment (23). Furthermore, we found a positive correlation between salivary Pg and Dd levels in our cohort (Spearman correlation coefficient =0.269, P<0.001). Univariate analysis results of the clinical characteristics and salivary biomarker levels between the HC and CRC groups are summarized in Table 1.
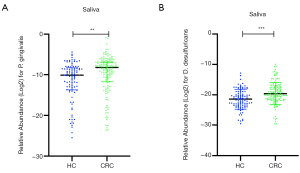
Development of the predictive model
Statistically, significant variables derived from the univariate analysis were subjected to multivariate logistic regression analysis. Logistic regression analysis revealed that age (OR =1.111, 95% CI, 1.072–1.151), male sex (OR =2.111, 95% CI, 1.068–4.175), OHI scores (OR =1.769, 95% CI, 1.116–2.804), and relative salivary Dd abundance (OR =1.156, 95% CI, 1.05–1.272) were independent risk factors for CRC, after adjusting for the presence of dental caries, comorbidities, current smoking status, and the salivary Pg level (Table 2). A four-variable model was constructed based on the four risk factors derived from the logistic analysis. All variables included in the model showed no collinearity between each other (VIF ranged from 1 to 2).
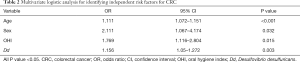
Full table
Evaluation of the model’s predictive accuracy
Our four-variable model’s predictive accuracy was evaluated using the Brier score, the Hosmer-Lemeshow goodness of fit test, the calibration curve, and the concordance-index (C-index). The Brier score was 0.144, which indicated a good predictive ability of the model. The Hosmer-Lemeshow goodness of fit test P value was over 0.05, indicating no statistical difference between the predictive and actual values. The calibration curve (Figure 3A) and C-index (Figure 3B) were high, with a value of 0.834 and 0.866, respectively.
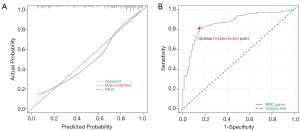
Nomogram creation
Nomograms graphically represent a complex mathematical algorithm that incorporates biological and clinical variables (24). We developed a nomogram based on the predictive variables and respective ORs calculated from the logistic regression (Figure 4).
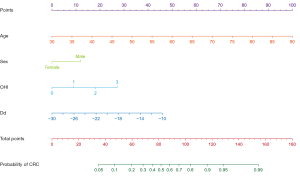
Discussion
Various microorganisms inhabit the oral cavity, which has a microbial diversity secondary only to the gut microbiome (25). The oral microbiome has been associated with human health and diseases, including oral cancer (26), pancreatic cancer (27), and systemic disease (28). One prospective study that assessed the association between the oral microbiome and CRC risk was conducted in an African American population, where the oral microbiome was represented by mouth rinse samples (29). The study preferentially recruited low-income populations, so selection bias existed. Saliva is a fluid secreted by the salivary glands and has various biological functions (30). The salivary microbiome diversity exhibits temporal stability (31), which makes it an optimal research target. In a study conducted by Italian scholars, preliminary comparisons of oral and gut microbiota led to identifying a different taxonomic composition in CRC patients from the HC group (9). However, a 16S rRNA high throughput sequencing-based methodology was adopted, which was costly. In the present study, we utilized quantitative PCR to measure the relative abundance of certain pathogens in the saliva.
After a thorough search for candidate salivary biomarkers based on the previous literature, we selected Fn, Pg, Dd, and Pm as qPCR targets in our cohort (29,32-34). Fn is one of the most widely investigated pathogens associated with CRC (7,16), although it has not been extensively investigated in the oral cavity. Fn was first found to be enriched in CRC tissues through whole-genome sequencing, followed by qPCR and 16S rRNA sequencing analysis (32). Fecal Fn has also been widely reported as a diagnostic biomarker for CRC, and a high abundance of mucosal Fn is associated with chemotherapy resistance (7,35). Mechanistically, Fn may promote carcinogenesis through E-cadherin/β-catenin signaling via FadA adhesin (36). Pg is a gram-negative anaerobe associated with chronic periodontitis, which can eventually lead to tooth loss (33). Pg acts as an important player in the development of various diseases, including Alzheimer’s disease (37). In fact, in a previously developed cohort using a 16S rRNA sequencing analysis, we identified that the salivary Pg level in CRC patients was higher than that of HC patients (data not shown, submitted article). One recent study reported that the salivary Fn level in CRC patients was higher than HCs, while the amount of salivary Pg was similar in both groups (38). However, the sample size was limited, and a different methodology was adopted. Pg oral carriage has shown to be associated with an increased risk of pancreatic cancer in a prospective study (27). Dd is also a gram-negative anaerobe that belongs to the SRB group. Functionally, SRB degrades the organic matter that enters the gastrointestinal tract and use a wide range of substrates to reduce sulfate to hydrogen sulfide (H2S) (39). Bacteria-derived H2S is toxic to the colon epithelium, causing DNA damage to the colon epithelial cells and promoting colon cancer cell proliferation (40,41). In vitro experiments have shown that the Dd endotoxin transcriptionally activates NF-kB and IKB-α genes in Caco2 colon cancer cells (42). Recently, one study conducted in the United States revealed that sulfur-metabolizing bacteria in the stool, including Dd, are associated with a higher risk of distal CRC in men (34). Another previous study using an algorithmic model identified that the gut Desulfovibrio genus was significantly elevated in a CRC group (43). The relative abundance of the Desulfovibrio genus, especially certain species under this genus, remains largely unexplored in the saliva of CRC patients. All of the above have prompted us to investigate the role of salivary Dd in CRC. As for Pm, one prospective study demonstrated that Pm in pre-diagnostic mouth rinse samples was associated with a decreased risk of CRC (29). Pm was enriched in the tumoral microhabitat among a cohort of 276 gastric cancer patients (44), suggesting a potential role in carcinogenesis. Moreover, it has been reported that Pm in the saliva may be a diagnostic indicator of oral squamous cell carcinoma (45). The diagnostic role of salivary Pm in CRC remains unexplored.
In our study, both salivary Pg and Dd levels were associated with a diagnosis of CRC. After adjusting for confounding factors, only Dd was found to be an independent risk factor for CRC. However, the discriminative ability of Dd in differentiating CRC from HC was limited when used alone. Apart from salivary Dd abundance, older age, male sex, and a higher OHI were also found to be independent risk factors for CRC after multivariate logistic regression analysis. The fact that older age and a higher OHI value are independent risk factors for CRC is concordant with another study conducted by the same researchers from our institute (data not shown, submitted article). As mentioned previously, although a positive correlation between the OHI and relative salivary Dd abundance was observed, the collinearity between the two variables disappeared after adjusting for other risk factors for CRC (1< VIF <2). According to the latest statistics (1), the incidence rates for males and those of older age are higher, especially for those over the age of 50, which is consistent with our results. A high OHI is inversely correlated with good oral hygiene habits and was demonstrated to be a risk factor for CRC. A retrospective research investigation from the Nurses’ Health Study found that women with fewer teeth and moderate to severe periodontal diseases are more likely to develop CRC (46), which is consistent with our results. As expected, we observed a higher incidence of type 2 diabetes in the CRC group. This is in agreement with findings from a prospective study that showed type 2 diabetes mellitus was associated with a higher risk of CRC (47). Nevertheless, due to the low proportion of diabetes comorbidity in our cohort, this variable does not enter into the final prediction model.
Colonoscopy is currently the most widely used screening method for detecting colorectal neoplasia. However, it is invasive, expensive and has low patient compliance (4). Less invasive screening methods include FOBT, serum CEA, and CA199 detection. Although FOBT has been demonstrated to reduce mortality rates, its sensitivity is limited (5). Serum CEA is a relatively mature biomarker that is widely used in clinical practice to monitor CRC recurrence and response to therapy, but it lacks sensitivity and specificity when used as a screening method (48). Elevated serum CA199 levels are usually seen in late-stage CRC but have demonstrated good value in monitoring the prognosis of CRC (49). Hence, there is an urgent need to develop a novel classifier for predicting the risk for CRC with less invasiveness, more convenience, and improved patient compliance.
Derived from logistic analysis, the predictive accuracy of our model was internally validated. The model had a higher AUC value than when Dd was used alone, with higher sensitivity and specificity. The Hosmer-Lemeshow test showed that the model’s predicted value was not significantly different compared to the actual value. The value of the C-index for our model is 0.866, which indicates good concordance. The model’s calibration slope was performed by comparing the predicted value with the observed value after bias correction and demonstrating a value of 0.834. The Brier score is a parameter evaluating the overall performance of the model and equals 0.144. Oncologists have long used nomograms to predict cancer outcomes with superiority (50). In the current study, we developed a visual nomogram that can be used to predict the probability of developing CRC.
To our knowledge, this is the first oral microbiome-based predictive model that incorporates oral hygiene habits for the diagnosis of CRC. Our research expands on the scope of CRC risk factors, such as a high OHI and elevated salivary Dd levels. Other than these two risk factors, there are two other variables included in this model: well-established risk factors for CRC incidence. The four variables selected in the model are easy and convenient to collect in the clinic. Additionally, this predictive model’s performance is encouraging as it demonstrates a high predictive ability for CRC. Using the visualized nomogram of this study, clinicians would be better positioned to identify at-risk CRC patients individually.
This is a single-center study and, therefore, may not be generalizable. The present study’s major limitation is a lack of serum preoperational CEA levels for most outpatients, which could not be included in the logistic regression analysis. Another limitation is that precancerous lesions were not available due to the slow speed of collection. Additional basic studies are warranted to explore Dd’s mechanistic role in the initiation and development of CRC.
Conclusions
In our retrospective, the single-center case-control study identified that elevated salivary Dd and a higher OHI were independent risk factors for CRC. We further established and internally validated a risk-prediction model, which provides clinicians with a more individualized risk estimation basis. Developing good oral hygiene habits, such as increasing tooth brushing and the frequency of dental visits, may act as an inexpensive intervention to reduce the risk of CRC. However, the nomogram we developed should be externally validated, and prospective studies should be conducted to evaluate the nomogram’s risk-predicting value.
Acknowledgments
Funding: This study was funded by National Natural Science Foundation of China Scientific Research and Innovation Group (81421001) and National Natural Science Foundation of China (81772517). The funders had no role in study design, data collection and analysis, decision to publish or preparation of the manuscript.
Footnote
Reporting Checklist: The authors have completed the TRIPOD Reporting Checklist. Available at http://dx.doi.org/10.21037/atm-20-8168
Data Sharing Statement: Available at http://dx.doi.org/10.21037/atm-20-8168
Conflicts of Interest: All authors have completed the ICMJE uniform disclosure form (available at http://dx.doi.org/10.21037/atm-20-8168). The authors have no conflicts of interest to declare.
Ethical Statement: The authors are accountable for all aspects of the work in ensuring that questions related to the accuracy or integrity of any part of the work are appropriately investigated and resolved. This study received approval from the Renji Hospital Ethics Committee, and informed consent was obtained from each patient. The study protocol conforms to the ethical guidelines of the 1975 Declaration of Helsinki (as revised in 2013) as reflected by a priori approval by the ethics committee of Renji Hospital (No.: 2018RJ-033).
Open Access Statement: This is an Open Access article distributed in accordance with the Creative Commons Attribution-NonCommercial-NoDerivs 4.0 International License (CC BY-NC-ND 4.0), which permits the non-commercial replication and distribution of the article with the strict proviso that no changes or edits are made and the original work is properly cited (including links to both the formal publication through the relevant DOI and the license). See: https://creativecommons.org/licenses/by-nc-nd/4.0/.
References
- Siegel RL, Miller KD, Jemal A. Cancer statistics, 2020. CA Cancer J Clin 2020;70:7-30. [Crossref] [PubMed]
- Chen W, Zheng R, Baade PD, et al. Cancer statistics in China, 2015. CA Cancer J Clin 2016;66:115-32. [Crossref] [PubMed]
- Schoen RE, Pinsky PF, Weissfeld JL, et al. Colorectal-cancer incidence and mortality with screening flexible sigmoidoscopy. N Engl J Med 2012;366:2345-57. [Crossref] [PubMed]
- Lieberman DA. Clinical practice. Screening for colorectal cancer. N Engl J Med 2009;361:1179-87. [Crossref] [PubMed]
- Hardcastle JD, Chamberlain JO, Robinson MHE, et al. Randomised controlled trial of faecal-occult-blood screening for colorectal cancer. Lancet 1996;348:1472-7. [Crossref] [PubMed]
- Fearon ER, Vogelstein B. A genetic model for colorectal tumorigenesis. Cell 1990;61:759-67. [Crossref] [PubMed]
- Liang Q, Chiu J, Chen Y, et al. Fecal Bacteria Act as Novel Biomarkers for Noninvasive Diagnosis of Colorectal Cancer. Clin Cancer Res 2017;23:2061-70. [Crossref] [PubMed]
- Zhang X, Zhang D, Jia H, et al. The oral and gut microbiomes are perturbed in rheumatoid arthritis and partly normalized after treatment. Nat Med 2015;21:895-905. [Crossref] [PubMed]
- Russo E, Bacci G, Chiellini C, et al. Preliminary Comparison of Oral and Intestinal Human Microbiota in Patients with Colorectal Cancer: A Pilot Study. Front Microbiol 2018;12;8:2699.
- Gupta B, Bray F, Kumar N, et al. Associations between oral hygiene habits, diet, tobacco and alcohol and risk of oral cancer: A case-control study from India. Cancer Epidemiol 2017;51:7-14. [Crossref] [PubMed]
- Hashim D, Sartori S, Brennan P, et al. The role of oral hygiene in head and neck cancer: results from International Head and Neck Cancer Epidemiology (INHANCE) consortium. Ann Oncol 2016;27:1619-25. [Crossref] [PubMed]
- Bertl K, Loidl S, Kotowski U, et al. Oral health status and dental care behaviours of head and neck cancer patients: a cross-sectional study in an Austrian tertiary hospital. Clin Oral Investig 2016;20:1317-27. [Crossref] [PubMed]
- Gilbert GH, Duncan RP, Kulley AM. Validity of self-reported tooth counts during a telephone screening interview. J Public Health Dent 1997;57:176-80. [Crossref] [PubMed]
- Buhlin K, Gustafsson A, Andersson K, et al. Validity and limitations of self-reported periodontal health. Community Dent Oral Epidemiol 2002;30:431-7. [Crossref] [PubMed]
- Ahrens W, Pohlabeln H, Foraita R, et al. Oral health, dental care and mouthwash associated with upper aerodigestive tract cancer risk in Europe: The ARCAGE study. Oral Oncol 2014;50:616-25. [Crossref] [PubMed]
- Xie YH, Gao QY, Cai GX, et al. Fecal Clostridium symbiosum for Noninvasive Detection of Early and Advanced Colorectal Cancer: Test and Validation Studies. EBioMedicine 2017;25:32-40. [Crossref] [PubMed]
- Dominy SS, Lynch C, Ermini F, et al. Porphyromonas gingivalis in Alzheimer’s disease brains: Evidence for disease causation and treatment with small-molecule inhibitors. Sci Adv 2019;5:eaau3333 [Crossref] [PubMed]
- Helms LR, Swenson RP. Cloning and characterization of the flavodoxin gene from Desulfovibrio desulfuricans. Biochim Biophys Acta 1991;1089:417-9. [Crossref] [PubMed]
- Allison HE, Hillman JD. Cloning and characterization of a Prevotella melaninogenica hemolysin. Infect Immun 1997;65:2765-71. [Crossref] [PubMed]
- Kostic AD, Chun E, Robertson L, et al. Fusobacterium nucleatum potentiates intestinal tumorigenesis and modulates the tumor-immune microenvironment. Cell Host Microbe 2013;14:207-15. [Crossref] [PubMed]
- Rubin DB, Schenker N. Multiple imputation in health-care databases: an overview and some applications. Stat Med 1991;10:585-98. [Crossref] [PubMed]
- Harrell FE. Regression modeling strategies, with applications to linear models, survival analysis and logistic regression. GET ADDRESS Springer; 2001.
- Langendijk-Genevaux PS, Grimm WD, van der Hoeven JS. Sulfate-reducing bacteria in relation with other potential periodontal pathogens. J Clin Periodontol 2001;28:1151-7. [Crossref] [PubMed]
- Grimes DA. The nomogram epidemic: resurgence of a medical relic. Ann Intern Med 2008;149:273-5. [Crossref] [PubMed]
- Kilian M, Chapple ILC, Hannig M, et al. The oral microbiome - an update for oral healthcare professionals. Br Dent J 2016;221:657-66. [Crossref] [PubMed]
- Gholizadeh P, Eslami H, Yousefi M, et al. Role of oral microbiome on oral cancers, a review. Biomed Pharmacother 2016;84:552-8. [Crossref] [PubMed]
- Fan X, Alekseyenko AV, Wu J, et al. Human oral microbiome and prospective risk for pancreatic cancer: a population-based nested case-control study. Gut 2018;67:120-7. [Crossref] [PubMed]
- Kumar PS. Oral microbiota and systemic disease. Anaerobe 2013;24:90-3. [Crossref] [PubMed]
- Yang Y, Cai Q, Shu XO, et al. Prospective study of oral microbiome and colorectal cancer risk in low-income and African American populations. Int J Cancer 2019;144:2381-9. [Crossref] [PubMed]
- Soini HA, Klouckova I, Wiesler D, et al. Analysis of Volatile Organic Compounds in Human Saliva by a Static Sorptive Extraction Method and Gas Chromatography-Mass Spectrometry. J Chem Ecol 2010;36:1035-42. [Crossref] [PubMed]
- Cameron SJS, Huws SA, Hegarty MJ, et al. The human salivary microbiome exhibits temporal stability in bacterial diversity. Marchesi J, editor. FEMS Microbiol Ecol 2015;91:fiv091.
- Kostic AD, Gevers D, Pedamallu CS, et al. Genomic analysis identifies association of Fusobacterium with colorectal carcinoma. Genome Res 2012;22:292-8. [Crossref] [PubMed]
- Bostanci N, Belibasakis GN. Porphyromonas gingivalis: an invasive and evasive opportunistic oral pathogen. FEMS Microbiol Lett 2012;333:1-9. [Crossref] [PubMed]
- Nguyen LH, Ma W, Wang DD, et al. Association Between Sulfur-Metabolizing Bacterial Communities in Stool and Risk of Distal Colorectal Cancer in Men. Gastroenterology 2020;158:1313-25. [Crossref] [PubMed]
- Yu T, Guo F, Yu Y, et al. Fusobacterium nucleatum Promotes Chemoresistance to Colorectal Cancer by Modulating Autophagy. Cell 2017;170:548-563.e16. [Crossref] [PubMed]
- Rubinstein MR, Wang X, Liu W, et al. Fusobacterium nucleatum promotes colorectal carcinogenesis by modulating E-cadherin/β-catenin signaling via its FadA adhesin. Cell Host Microbe 2013;14:195-206. [Crossref] [PubMed]
- Taylor JJ, Preshaw PM, Lalla E. A review of the evidence for pathogenic mechanisms that may link periodontitis and diabetes. J Clin Periodontol 2013;40:S113-34. [Crossref] [PubMed]
- Guven DC, Dizdar O, Alp A, et al. Analysis of Fusobacterium nucleatum and Streptococcus gallolyticus in saliva of colorectal cancer patients. Biomark Med 2019;13:725-35. [Crossref] [PubMed]
- Scanlan PD, Shanahan F, Marchesi JR. Culture-independent analysis of desulfovibrios in the human distal colon of healthy, colorectal cancer and polypectomized individuals: Desulfovibrio sp. in the human distal gut. FEMS Microbiol Ecol 2009;69:213-21. [Crossref] [PubMed]
- Guo FF, Yu TC, Hong J, et al. Emerging Roles of Hydrogen Sulfide in Inflammatory and Neoplastic Colonic Diseases. Front Physiol 2016;7:156. [Crossref] [PubMed]
- Linden DR. Hydrogen Sulfide Signaling in the Gastrointestinal Tract. Antioxid Redox Signal 2014;20:818-30. [Crossref] [PubMed]
- Kapral M, Węglarz L, Parfiniewicz B, et al. Quantitative Evaluation of Transcriptional Activation of NF-κB p65 and p50 Subunits and IκBα Encoding Genes in Colon Cancer Cells by Desulfovibrio desulfuricans Endotoxin. Folia Microbiol (Praha) 2010;55:657-61. [Crossref] [PubMed]
- Ai D, Pan H, Li X, et al. Identifying Gut Microbiota Associated With Colorectal Cancer Using a Zero-Inflated Lognormal Model. Front Microbiol 2019;10:826. [Crossref] [PubMed]
- Liu X, Shao L, Liu X, et al. Alterations of gastric mucosal microbiota across different stomach microhabitats in a cohort of 276 patients with gastric cancer. EBioMedicine 2019;40:336-48. [Crossref] [PubMed]
- Mager DL, Haffajee AD, Devlin PM, et al. The salivary microbiota as a diagnostic indicator of oral cancer: a descriptive, non-randomized study of cancer-free and oral squamous cell carcinoma subjects. J Transl Med 2005;3:27-7. [Crossref] [PubMed]
- Momen-Heravi F, Babic A, Tworoger SS, et al. Periodontal disease, tooth loss and colorectal cancer risk: Results from the Nurses’ Health Study. Int J Cancer 2017;140:646-52. [Crossref] [PubMed]
- Ma Y, Yang W, Song M, et al. Type 2 diabetes and risk of colorectal cancer in two large U.S. prospective cohorts. Br J Cancer 2018;119:1436-42. [Crossref] [PubMed]
- Fakih MG, Padmanabhan A. CEA monitoring in colorectal cancer. What you should know. Oncology (Williston Park) 2006;20:579. [PubMed]
- Wang RF, Song BR, Peng JJ, et al. The Prognostic Value of Preoperative Serum CEA and CA19-9 Values in Stage I-III Colorectal Cancer. Hepatogastroenterology 2014;61:994-9. [PubMed]
- Shariat SF, Karakiewicz PI, Suardi N, et al. Comparison of nomograms with other methods for predicting outcomes in prostate cancer: a critical analysis of the literature. Clin Cancer Res 2008;14:4400-7. [Crossref] [PubMed]