Abstract
Keywords
s-triazine derivatives In-silico ADME PCA Polynomial regression
Introduction
Traditional drug development includes compound synthesis and pre-clinical in-vitro and in-vivo studies to determine whether such a compound can be considered as a candidate for clinical trial. Such procedures are normally accompanied with enormous costs measured in billions of dollars and more than a decade of interdisciplinary endeavor. In addition to high investments, many of tested candidates in later stages of drug development might demonstrate lack of efficiency, poor pharmacokinetics, animal toxicity and adverse effects in humans. For this, modern process of drug development is based on combinatorial chemistry, genomics, chemometrics and in-silico processing. While one group of these computational methods focuses on biological activity, trying to forecast interactions with target receptors (toxicodynamic), others tend to predict the fate of the substance in the human body i.e. its absorption, distribution, metabolism and excretion (ADME). Chemometrics has an important place in relating structural or property descriptors of a drug candidate to its biological activity (QSAR - Quantitative structure-activity relationship).
The 1,3,5-triazine (s-triazine) heterocyclic system is today found in a number of bioactive molecules such as herbicides and pharmaceutical products (1). Various triazine substituted molecules exhibit diverse biological activities, having thus been reported as potentially cardiotonic (2,3), anti-HIV (4,5), antitumor (6) and anticancer agents (7).
s-Triazine is a weak base with six-membered heterocyclic ring containing three nitrogens replacing carbon-hydrogen units in the benzene ring. The compound, so as its derivatives, has an excellent potential for the formation of non-covalent bonds, such as coordination and H-bonds, via its nitrogen ion-pairs (8). Non-covalent bonds have a very important role in biological activity of these compounds (9), but also in understanding of their physiological behavior, namely absorption, metabolism and elimination. Furthermore, such chemical properties of s-triazines are responsible for their characteristic chromatographic behaviour.
Molecular lipophilicity is one of the major physicochemical properties affecting oral absorption, cell uptake, protein binding, blood-brain penetration, and metabolism of bioactive substances (10). Cell membranes are relatively impermeable to hydrophilic compounds, so these are transported predominantly via paracellular route. Thus lipophilic character of the molecules enables passive diffusion through cell membrane and highly hydrophobic substances enter the cells easily. On the other hand, too high lipophilicity of drugs can be a limiting factor to oral absorption. In order to be absorbed via gut mucose, the substance needs first to be dissolved in hydrophilic mucose. Excessive lipophilicity is, thus, often linked to incomplete drug absorption after oral administration. Other mechanisms of compound transfer across the membrane not involving previous dissolution exist, such as endocytosis, but are mostly characteristic for large molecules (11). It is also generally believed that very lipophilic compounds have greater affinity for plasma-protein binding and are easily transported across the blood-brain barrier (12).
Chromatographic approach has been shown to be quite successful in modeling physicochemical and biological processes (13,14). Owing to its simplicity and efficiency, reversed-phase thin-layer chromatography appears especially attractive for lipophilicity determination (15,16). Taking into consideration that in reversed-phase chromatography solutes distribute between polar and nonpolar phases, calculated retention parameters can be adopted as indirect designators of compounds lipophilicity.
Considering the practical importance of s-triazine derivatives, the main objective of this study was to examine the retention behavior of four classes synthesized s-triazine derivatives in reversed-phase chromatographic systems of five different mobile phases. Novelty of the paper is the correlation between in-silico ADME properties of s-triazine derivatives and its retention behaviour in RP-HPTLC systems.
Chromatographic data were correlated to selected physicochemical properties related to ADME properties, obtained by the established computational medicinal chemistry methods (17). Observed parameters included human intestinal absorption (HIA), plasma protein binding (PPB), blood-brain barrier (BBB) penetration, skin permeability (SP) and oral absorption (expressed as Madin-Darby canine kidney cells (MDCK) and human colorectal carcinoma cells (Caco-2) permeability). In addition, bonding affinities to different receptors (ion channel modulator (ICM), G protein-coupled receptor (GPCR) and nuclear receptor (NRL)) were estimated for studied s-triazine derivatives, as well as protease inhibition (PI) and kinase inhibition (KI) ability.
Statistical validity of established correlation was tested by standard statistical parameters, such as Fisher’s criterion (F), correlation coefficient (r) and standard deviation (s), and cross-validation parameters (cross-validated coefficient of determination - r2cv, adjusted coefficient of determination - r2adj, predicted residual sum of squares - PRESS, total sum of squares - TSS, PRESS/TSS ratio , standard deviation based on predicted residual sum of squares - SPRESS).
Principal component analysis (PCA), as a statistical tool for reducing dimensionality of a large number of interrelated variables and revelation of similarities among examined entities, was applied on the set of the calculated ADME properties of studied molecules. With PCA a set of new variables (principal components, PC) is defined instead of the original variables. PCs are formed by combination of the original data in such a way that the PC1 covers as much of the variation within the data set as posible. The PC2 describes the maximum amount of residual variation after the PC1 has been taken into consideration, etc (18). The scores plot of the two PC is a 2-D map, that provides a data overview and displays patterns or grouping within the data. The loadings plot shows relationships between variables that contribute to the positioning of the objects on the scores plot.
Experimental
Synthesis of s-Triazine derivatives
The investigated compounds were 1,3,5-triazines substituted at positions 4 and 6 by smaller and larger groups with various lipophilic characteristics, chosen for investigation are presented in Table 1. Their melting points experimentaly (19, 20) and theoretical (21) observed, are shown in Table 1. All of investigated s-triazine derivatives were synthesized by the modified procedure of Thurston from cyanuric chloride and corresponding amines (22). In synthesis commercial cyanuric chloride (2,4,6-trichloro-1,3,5-triazine), was used (Fluka, Germany).
The chemical structures of studied s-triazines
Reversed-phase high performance thin-layer chromatography
Precoated RP-18W/UV254 plates (Macherey-Nagel GmbH and Co., Düren, Germany) were used for RP HPTLC analysis. Investigated solvent mixtures used as mobile phases: acetone-water (φ = 0.5 - 0.8; v/v), acetonitrile-water (φ = 0.5 - 0.9; v/v), methanol-water (φ = 0.65 - 0.95; v/v), 2-propanol-water (φ = 0.4 - 0.7; v/v), tetrahydrofuran-water (φ = 0.5 - 0.75; v/v).
The investigated compounds were dissolved in an appropriate solvent, methanol, 1 mg mL-1) and the solutions (0.2 μL) were separately spotted into the plates. All the reagents used were of analytical purity. The plates were developed by the ascending technique at room temperature without previous saturation of the chamber with mobile phase. All measurements were carried out at ambient temperature. After drying of the plates, the spots were visualized under UV light at λ = 254 nm. RF values were calculated as average from three measurements for each solute-mobile phase combination. For subsequent calculations mean RM values were used; these were calculated by using the formula:
The calculated RM values for different concentrations of organic solvent were used to check the linearity of their relationship with the volume fraction of organic modifier according to the equation (23):
Where φ is the volume fraction of organic solvent in the mobile phase, RM0 is the intercept obtained by extrapolation to φ = 0% of modifier, and S is the slope of the linear plot. Equations (1) and (2) served for deriving data for further QSAR studies.
Statistical methods and descriptors calculation
The complete regression analysis and PCA were carried out by PASS 2005, GESS 2006, NCSS Statistical Softwares and Statistica version 8 program (24). Physicochemical and ADME properties were calculated using the PreADMET (25) and Molinspiration online programs (26).
Results and Discussion
PCA
In order to obtain some basic insight into the similarities/dissimilarities among studied molecules on the basis of their ADME properties, PCA was carried out on the set of the calculated ADME properties. The first principal component (PC1) accounted for 47.31% of data variance and the second one (PC2) for 21.41%.
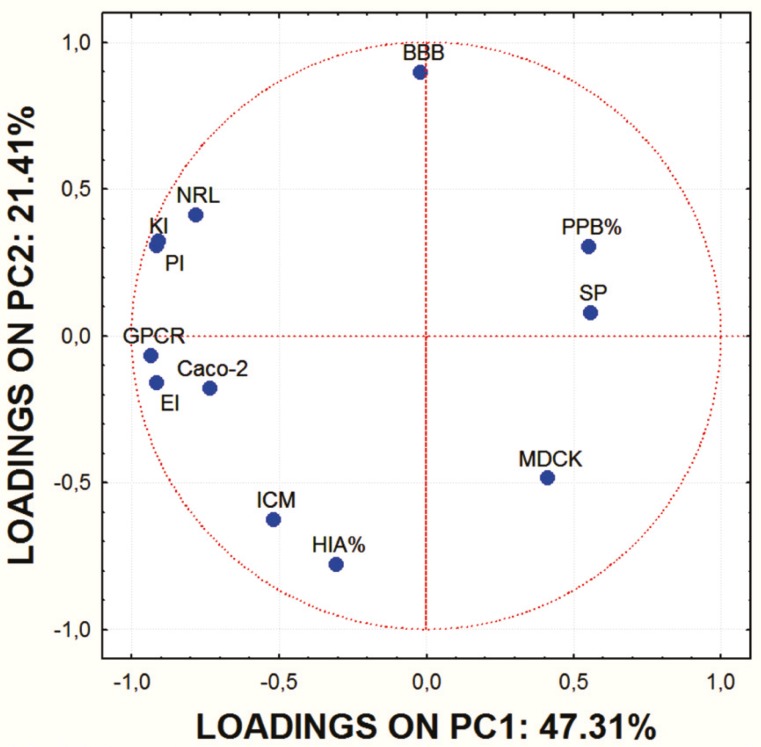
Score values for PC1 and PC2 are shown in Figure 1. and the mutual projections of the loading vectors in Figure 2. The loading graph reveals that significant negative influence on the PC1 have NRL, KI, PI, GPCR, EI and Caco-2 parameters. PPB, SP and MDCK have positive influence on the PC1. The most positive impact on the PC2 has BBB parameter, while ICM, HIA and MDCK parameters express negative impact on the PC2. Mentioned ADME properties are responsible for distribution of molecules on score plot that shows four well-separated groups of studied compounds. On the basis of presented PCA results it can be concluded that groups of examined molecules on the score plot are equal to the groups shown in Table 1 which are based on the molecular structure and substituents present in the examined compounds.
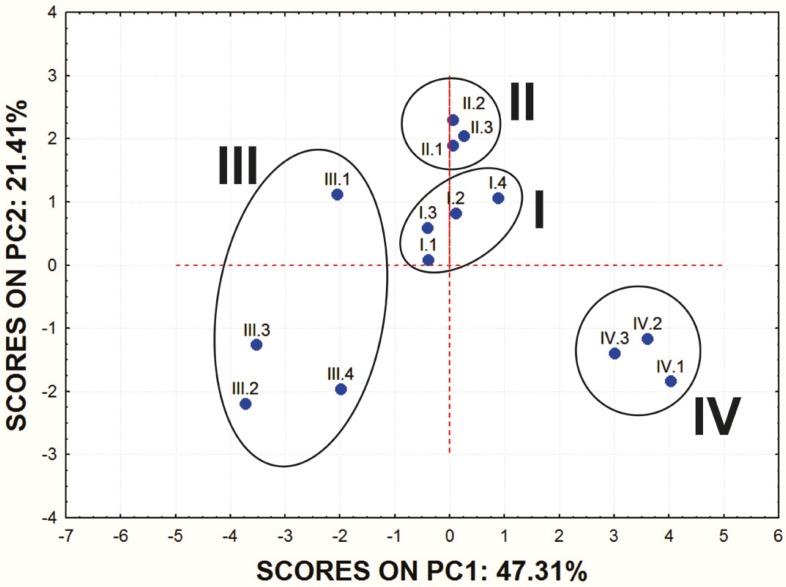
Results from regression analysis using well-known equation (2) according a procedure is described earlier (27). Calculated statistics illustrate that assumed linear dependence correlates very good with experimental data.
Correlation of RM0 with biological activity predictors
PPB, HIA, BBB, SP, MDCK and Caco-2 values (Table 2) were estimated for synthesized s-triazine derivatives by mathematical modeling using the PreADMET program. Molinspiration online program was used for druglikeness calculation (NRL, ICM, KI, PI, EI and GPCR). The retention data, their standard deviations and the characteristics of the
The characteristics of the equations RM=RM0=S∅ obtained for RP HPTLC separation of analysed s-triazines
Comp. | methanol – water | 2-propanol – water | acetone – water | acetonitrile – water | tetrahydrofuran - water | ||||||||||||||
---|---|---|---|---|---|---|---|---|---|---|---|---|---|---|---|---|---|---|---|
RM0 | S | r | RM0 | S | r | RM0 | S | r | RM0 | S | r | RM0 | S | r | |||||
I.1 | 2.457 | -3.087 | 0.996 | 1.900 | -3.34 | 0.999 | 3.185 | -4.721 | 0.999 | 2.110 | -3.501 | 0.998 | 3.439 | -5.218 | 0.998 | ||||
I.2 | 3.188 | -3.738 | 0.997 | 2.525 | -4.196 | 0.993 | 3.580 | -5.078 | 0.997 | 2.786 | -4.194 | 0.995 | 3.567 | -5.289 | 0.993 | ||||
I.3 | 3.976 | -4.570 | 0.992 | 2.617 | -4.153 | 0.991 | 3.839 | -5.370 | 0.997 | 2.887 | -4.215 | 0.996 | 3.538 | -5.214 | 0.996 | ||||
I.4 | 4.407 | -4.984 | 0.999 | 2.764 | -4.332 | 0.993 | 4.303 | -5.984 | 0.998 | 2.896 | -4.098 | 0.995 | 3.351 | -4.912 | 0.996 | ||||
II.1 | 3.181 | -3.829 | 0.995 | 2.183 | -3.826 | 0.997 | 3.743 | -5.197 | 0.993 | 2.388 | -3.347 | 0.997 | 3.289 | -4.748 | 0.995 | ||||
II.2 | 3.320 | -3.868 | 0.994 | 2.384 | -4.024 | 0.998 | 3.935 | -5.262 | 0.997 | 2.753 | -3.699 | 0.997 | 3.401 | -4.816 | 0.996 | ||||
II.3 | 4.530 | -5.070 | 0.998 | 3.013 | -4.811 | 0.997 | 4.218 | -5.446 | 0.998 | 3.000 | -3.788 | 0.995 | 4.071 | -5.730 | 0.994 | ||||
III.1 | 3.440 | -4.086 | 0.991 | 2.251 | -3.962 | 0.996 | 4.118 | -5.741 | 0.993 | 2.356 | -3.304 | 0.995 | 3.426 | -5.06 | 0.991 | ||||
III.2 | 3.998 | -4.452 | 0.994 | 2.769 | -4.437 | 0.996 | 4.626 | -6.004 | 0.998 | 3.284 | -4.197 | 0.998 | 3.611 | -5.140 | 0.994 | ||||
III.3 | 3.980 | -4.471 | 0.985 | 2.691 | -4.383 | 0.988 | 4.019 | -5.306 | 0.988 | 3.101 | -4.076 | 0.988 | 3.387 | -4.912 | 0.996 | ||||
III.4 | 5.295 | -5.764 | 0.995 | 2.747 | -4.117 | 0.998 | 4.693 | -5.951 | 0.996 | 3.556 | -4.394 | 0.997 | 3.902 | -5.503 | 0.998 | ||||
IV.1 | 1.526 | -2.206 | 0.999 | 1.118 | -2.422 | 0.992 | 1.672 | -2.707 | 0.994 | 1.497 | -2.592 | 0.997 | 1.733 | -2.897 | 0.996 | ||||
IV.2 | 1.626 | -2.242 | 0.988 | 1.400 | -2.910 | 0.991 | 2.034 | -3.184 | 0.985 | 1.590 | -2.629 | 0.995 | 1.795 | -2.900 | 0.996 | ||||
IV.3 | 1.756 | -2.090 | 0.993 | 1.779 | -3.164 | 0.984 | 2.257 | -3.286 | 0.995 | 1.956 | -2.821 | 0.996 | 2.222 | -3.340 | 0.985 |
Standard errors of the retention data
Molecule | HIA% | Caco-2 (nm/sec) | MDCK (nm/sec) | SP | PPB% | BBB (Cbrain/Cblood) | GPCR | ICM | KI | NRL | PI | EI |
---|---|---|---|---|---|---|---|---|---|---|---|---|
I.1 | 95.01 | 29.72 | 0.20 | -2.30 | 89.27 | 4.47 | 0.07 | -0.12 | 0.10 | -0.20 | -0.35 | -0.09 |
I.2 | 95.28 | 31.99 | 0.13 | -2.24 | 88.82 | 7.21 | 0.03 | -0.17 | 0.06 | -0.21 | -0.38 | -0.13 |
I.3 | 95.95 | 44.38 | 0.09 | -2.22 | 93.61 | 7.94 | 0.06 | -0.12 | 0.08 | -0.20 | -0.35 | -0.10 |
I.4 | 96.22 | 47.80 | 0.02 | -2.12 | 100.00 | 8.32 | -0.03 | -0.18 | 0.05 | -0.29 | -0.44 | -0.15 |
II.1 | 92.84 | 24.25 | 1.79 | -2.40 | 100.00 | 5.62 | 0.14 | -0.19 | 0.01 | -0.03 | -0.32 | -0.03 |
II.2 | 93.28 | 26.82 | 0.52 | -1.95 | 100.00 | 7.36 | 0.15 | -0.17 | 0.02 | 0.04 | -0.26 | -0.08 |
II.3 | 93.70 | 30.23 | 11.18 | -1.59 | 100.00 | 8.75 | 0.15 | -0.16 | 0.02 | 0.05 | -0.21 | -0.07 |
III.1 | 92.85 | 24.86 | 0.55 | -2.99 | 100.00 | 6.62 | 0.23 | -0.03 | 0.16 | -0.02 | -0.24 | 0.10 |
III.2 | 97.69 | 51.33 | 0.25 | -2.58 | 91.59 | 1.21 | 0.34 | 0.02 | 0.12 | -0.03 | -0.18 | 0.15 |
III.3 | 97.61 | 52.76 | 1.49 | -2.53 | 92.25 | 1.87 | 0.39 | -0.08 | 0.26 | -0.01 | -0.20 | 0.10 |
III.4 | 98.03 | 53.78 | 20.59 | -1.98 | 92.75 | 3.02 | 0.26 | -0.07 | 0.10 | -0.04 | -0.17 | 0.05 |
IV.1 | 95.36 | 26.68 | 14.97 | -2.16 | 100.00 | 1.44 | -0.13 | -0.15 | -0.45 | -0.53 | -0.86 | -0.18 |
IV.2 | 95.41 | 7.91 | 6.83 | -1.99 | 100.00 | 2.10 | -0.07 | -0.11 | -0.39 | -0.39 | -0.78 | -0.20 |
IV.3 | 95.46 | 8.93 | 18.92 | -1.83 | 100.00 | 2.99 | -0.01 | -0.08 | -0.30 | -0.30 | -0.67 | -0.15 |
In silico ADME characteristics of studied compounds
Modifier | Acetone | Acetonitrile | Methanol | 2-Propanol | Tetrahydrofuran | |||||
---|---|---|---|---|---|---|---|---|---|---|
Molecule | Standard error of Rm0 | Standard error of S | Standard error of Rm0 | Standard error of S | Standard error of Rm0 | Standard error of S | Standard error of Rm0 | Standard error of S | Standard error of Rm0 | Standard error of S |
I. 1 | 0.0475 | 0.0705 | 0.7039 | 0.9303 | 0.0922 | 0.1106 | 0.1365 | 0.0498 | 0.1435 | 0.2276 |
I. 2 | 0.2225 | 0.3162 | 0.1381 | 0.1918 | 0.0950 | 0.1139 | 0.2608 | 0.1041 | 0.1723 | 0.2732 |
I. 3 | 0.1292 | 0.1916 | 0.1236 | 0.1717 | 0.1940 | 0.2405 | 0.2718 | 0.1085 | 0.1440 | 0.2283 |
I. 4 | 0.1341 | 0.1990 | 0.0610 | 0.0846 | 0.3027 | 0.3753 | 0.2598 | 0.1037 | 0.1133 | 0.1796 |
II. 1 | 0.1286 | 0.1828 | 0.1090 | 0.1513 | 0.3633 | 0.4355 | 0.1956 | 0.0781 | 0.1467 | 0.2326 |
II. 2 | 0.1399 | 0.2076 | 0.0967 | 0.1342 | 0.1077 | 0.1336 | 0.2893 | 0.1060 | 0.1316 | 0.2087 |
II. 3 | 0.2048 | 0.3038 | 0.1993 | 0.2767 | 0.0828 | 0.1027 | 0.2992 | 0.1194 | 0.1975 | 0.3132 |
III. 1 | 0.4059 | 0.6023 | 0.1985 | 0.2756 | 0.3306 | 0.4099 | 0.2246 | 0.0897 | 0.2856 | 0.4528 |
III. 2 | 0.2187 | 0.3246 | 0.1007 | 0.1398 | 0.1800 | 0.2231 | 0.2647 | 0.1057 | 0.2115 | 0.3353 |
III. 3 | 0.1401 | 0.2079 | 0.0901 | 0.1252 | 0.2069 | 0.2566 | 0.2537 | 0.1013 | 0.1313 | 0.2082 |
III. 4 | 0.2511 | 0.3727 | 0.2038 | 0.2830 | 0.1744 | 0.2163 | 0.2139 | 0.0854 | 0.1582 | 0.2509 |
IV. 1 | 0.1121 | 0.1737 | 0.3298 | 0.4579 | 0.4987 | 0.6184 | 0.1184 | 0.0472 | 0.2064 | 0.3273 |
IV. 2 | 0.1443 | 0.2235 | 0.0744 | 0.1033 | 0.0853 | 0.1057 | 0.1523 | 0.0608 | 0.1260 | 0.1998 |
IV. 3 | 0.3347 | 0.4757 | 0.0944 | 0.1311 | 0.2832 | 0.3512 | 0.2373 | 0.0947 | 0.1222 | 0.1938 |
Correlation analysis showed that the retention parameter correlates the best with KI and PI. Established mathematical models and its basic statistical parameters (r, F, s) are presented in Table 5.
Polynomial correlations between retention parameter (RM0) and ADME descriptors of studied compounds
Modifier | Dependent variable | Polynomial regression:Y = a · (RM0)2 + b · RM0 + c | Eq. | |||||
---|---|---|---|---|---|---|---|---|
Y | a | b | c | r | F | s | ||
Methanol | KI | -0.0807 | 0.6604 | -1.2173 | 0.9200 | 30.3 | 0.0903 | 3 |
Acetone | KI | -0.0885 | 0.7579 | -1.5169 | 0.9407 | 42.3 | 0.0782 | 4 |
Tetrahydrofuran | KI | -0.1545 | 1.1238 | -1.9476 | 0.9481 | 48.8 | 0.0733 | 5 |
Methanol | PI | -0.0657 | 0.5851 | -1.5263 | 0.9153 | 28.4 | 0.0989 | 6 |
2-Propanol | PI | -0.1967 | 1.1643 | -1.9652 | 0.9002 | 23.5 | 0.1069 | 7 |
Acetone | PI | -0.0524 | 0.5535 | -1.6536 | 0.9528 | 54.2 | 0.0745 | 8 |
Acetonitrile | PI | -0.1739 | 1.1724 | -2.1923 | 0.9040 | 24.6 | 0.1049 | 9 |
Tetrahydrofuran | PI | -0.0548 | 0.5981 | -1.7104 | 0.9507 | 51.7 | 0.0761 | 10 |
Methanol | Caco-2 | 0.1015 | 9.8956 | -1.2877 | 0.8045 | 10.0968 | 9.7347 | 11 |
Acetone | GPCR | 0.0108 | 0.0520 | -0.2221 | 0.7534 | 7.2244 | 0.1097 | 12 |
2-Propanol | NRL | -0.1709 | 0.9461 | -1.375 | 0.8013 | 9.8696 | 0.1149 | 13 |
Acetonitrile | BBB% | -5.1906 | 26.874 | -28.00 | 0.7433 | 6.7929 | 2.0227 | 14 |
Acetone | MDCK | 5.6721 | -38.794 | 66.88 | 0.6624 | 4.3025 | 6.2033 | 15 |
Correlation coefficient higher than 0.90 indicates very high correlation between RM0 and selected ADME properties. F-value is found statistically significant at 99% level since all the calculated F values are higher as compared to tabulated values.
Equations 3-10 were cross-validated by the leave-one-out method (Table 6). High values of r2cv and r2adj (higher than 0.5) and PRESS values significantly less than TSS were obtained for all the models indicate that these models have very good predictive power (28). The prediction errors of the best equations (3-10) are presented in Table 7.
Cross-validation parameters for equations 3-15
Eq. | r2cv | r2adj | PRESS | TSS | PRESS/TSS | SPRESS |
---|---|---|---|---|---|---|
3 | 0.6543 | 0.8184 | 0.2018 | 0.5838 | 0.3457 | 0.1201 |
4 | 0.8261 | 0.8640 | 0.1015 | 0.5838 | 0.1739 | 0.0851 |
5 | 0.8418 | 0.8804 | 0.0923 | 0.5838 | 0.1581 | 0.0812 |
6 | 0.5995 | 0.8082 | 0.2653 | 0.6623 | 0.4006 | 0.1377 |
7 | 0.6916 | 0.7759 | 0.2043 | 0.6623 | 0.3085 | 0.1208 |
8 | 0.8703 | 0.8911 | 0.0859 | 0.6623 | 0.1297 | 0.0783 |
9 | 0.7295 | 0.7841 | 0.1792 | 0.6623 | 0.2706 | 0.1131 |
10 | 0.8678 | 0.8863 | 0.0876 | 0.6623 | 0.1323 | 0.0791 |
11 | 0.4387 | 0.5832 | 1659.09 | 2956.06 | 0.5613 | 10.8861 |
12 | 0.4132 | 0.4892 | 0.1799 | 0.3066 | 0.5867 | 0.1133 |
13 | 0.4486 | 0.5771 | 0.2238 | 0.4059 | 0.5514 | 0.1264 |
14 | 0.3017 | 0.4712 | 70.2399 | 100.59 | 0.6982 | 2.2398 |
15 | -0.1577 | 0.3369 | 873.40 | 754.43 | 1.1577 | 7.8985 |
The main purpose of the conducted correlation analysis was to determine the ability to predict ADME properties of these molecules using chromatographic retention data, since the chromatography has been shown to be quite successful in modeling physicochemical and biological properties. ADME processes are dynamic in nature, as the chromatographic separations are (29).
Prediction errors of the developed equations
Molecules | Prediction errors of the developed equations | |||||||
---|---|---|---|---|---|---|---|---|
Eq. 3 | Eq. 4 | Eq. 5 | Eq. 6 | Eq. 7 | Eq. 8 | Eq. 9 | Eq. 10 | |
I. 1 | 0.1819 | 0.1008 | 0.0101 | 0.1353 | 0.1131 | 0.0723 | 0.1428 | -0.0484 |
I. 2 | -0.0079 | -0.0021 | -0.0352 | -0.0513 | -0.1006 | -0.0364 | -0.1042 | -0.1058 |
I. 3 | -0.0527 | -0.0084 | -0.0145 | -0.1114 | -0.0846 | -0.0490 | -0.0930 | -0.0697 |
I. 4 | -0.0758 | -0.0557 | -0.0333 | -0.2162 | -0.1902 | -0.1979 | -0.1845 | -0.1185 |
II. 1 | -0.,0568 | -0.0700 | -0.0673 | 0.0099 | 0.0409 | -0.0040 | 0.0643 | 0.0160 |
II. 2 | -0.,0657 | -0.0751 | -0.0674 | 0.0479 | 0.0474 | 0.0270 | 0.0227 | 0.0501 |
II. 3 | -0.0983 | -0.0854 | -0.0469 | 0.0140 | 0.0328 | 0.0412 | 0.0302 | -0.0263 |
III. 1 | 0.0605 | 0.0566 | 0.0709 | 0.0510 | 0.1010 | 0.0229 | 0.1554 | 0.0645 |
III. 2 | -0.0131 | 0.0247 | 0.0241 | 0.0572 | 0.0694 | 0.0345 | 0.0376 | 0.0852 |
III. 3 | 0.1272 | 0.1604 | 0.1737 | 0.0383 | 0.0565 | 0.0755 | 0.0289 | 0.1133 |
III. 4 | 0.0831 | 0.0092 | 0.0149 | 0.1002 | 0.0812 | 0.0401 | 0.0522 | 0.0410 |
IV. 1 | -0.0525 | 0.0471 | 0.0141 | -0.0736 | 0.0494 | 0.0146 | -0.0331 | -0.0215 |
IV. 2 | -0.0331 | -0.0485 | 0.0382 | -0.0314 | -0.0593 | -0.0354 | -0.0122 | 0.0334 |
IV. 3 | 0.0065 | -0.0429 | -0.0867 | 0.0315 | -0.1536 | 0.0013 | -0.1056 | -0.0180 |
Conclusion
Retention constants in reverse-phase chromatography of proposed synthesized s-triazine derivatives have been shown to be a useful and simple way in predicting biological activity and drug likeness. For all investigated derivatives, experimentally determined retention parameters, RM0, could be reliably correlated with some of the ADME properties. It was found that experimentally determined retention parameter (RM0) of studied s-triazine derivatives was reliably correlated with in-silico calculated protease inhibition (PI) and kinase inhibition (KI) ability. Standard statistical measures and cross-validation parameters indicate that the established mathematical dependences between retention parametres and ADME properties are statistically valid. Also, PCA applied on both the retention parameters and calculated ADME properties showed similar grouping of molecules. That could indicate the similarity between retention behaviour and ADME properties of the examined molecules. On the basis of presented results it can be concluded that the retention parameters obtained by RP HPTLC could be successfully used for prediction of some in-silico ADME properties of studied compounds.
Acknowledgements
References
-
1.
Blotny G. Recent applications of 2,4,6-trichloro-1,3,5-triazine and its derivatives in organic synthesis. Tetrahedron. 2006;62:9507-9522.
-
2.
Kosary J, Kasztreiner E, Rabloczky G, Kurthy M. Synthesis and cardiotonic activity of 2,4-diamino-1.3,5-triazines. Eur. J. Med. Chem. 1989;24:97-99.
-
3.
Barraclough P, Firmin D, Lindon JC, Nobbs MS, Sanderson PN, Smith S, Gillam JM. Sites of protonation in cardiotonic polyazaindolizines by NMR spectroscopy. Magn. Reson. Chem. 1991;29:468-475.
-
4.
Patel RV, Kumari P, Rajani DP, Pannecouque C, De Clercq E, Chikhalia KH. Antimicrobial, anti-TB, anticancer and anti-HIV evaluation of new s-triazine-based heterocycles. Future Med. Chem. 2012;4:1053-1065. [PubMed ID: 22709250].
-
5.
Desai NC, Bhatt JJ, Shah BR, Trivedi PB, Undavia NK. Anti-HIV activity of some non-nucleoside s-triazine derivatives (Part-IV). Indian J. Exp. Biol. 1996;34:584-587. [PubMed ID: 8792647].
-
6.
Brzozowski Z, Saczewski F, Gdaniec M. Synthesis, structural characterization and antitumor acitivity of novel 2,4-diamino-1,3,5-triazine derivatives. Eur. J. Med. Chem. 2000;35:1053-1064. [PubMed ID: 11248404].
-
7.
Corbett TH, Leopold WR, Dykes DJ, Roberts BJ, Griswold DP Jr, Schabel FM Jr. Toxicity and anticancer activity of a new triazine antifolate (NSC 127755). Cancer Res. 1982;42:1707-1715. [PubMed ID: 7066891].
-
8.
Mooibroek TJ, Gamez P. The s-triazine ring, a remarkable unit to generate supramolecular interactions. Inorg. Chim. Acta. 2007;360:381-404.
-
9.
Ma JC, Dougherty DA. The Cation-π Interaction. Chem. Rev. 1997;97:1303-1324. [PubMed ID: 11851453].
-
10.
Walther B, Vis P, Taylor A. V Pliska, B Testa, H Van de Waterbeemd (eds, editors. Lipophilicity in Drug Action and Toxicology. VCH, Weinheim; 1996. p. 253-261.
-
11.
Švarc-Gajić J. General Toxicology. New York: Novascience Publishers; 2009. p. 1-11.
-
12.
Mornar A, Medić-Šarić M, Jasparica I. ADME data for polyphenols characterized by reversed-phase thin-layer chromatography. J. Planar Chromatogr. 2006;19:409-417.
-
13.
Djaković-Sekulić T, Smoliński A. Chemometric characterization of s -triazine derivatives in relation to structural parameters and biological activity. Drug Dev. Ind. Pharm. 2010;36:954-961. [PubMed ID: 20184415].
-
14.
Perišić-Janjić N, Kaliszan R, Wiczling P, Milošević N, Uscumlic G, Banjac N. Reversed-Phase TLC and HPLC Retention Data in Correlation Studies with in Silico Molecular Descriptors and Druglikeness Properties of Newly Synthesized Anticonvulsant Succinimide Derivatives. Mol. Pharmaceut. 2011;8:555-563.
-
15.
Jevrić L, Jovanović B, Velimirović S, Tepić A, Koprivica G, Mišljenović N. Application of lipophilicity parameters in QSRR analysis of newly synthesized s-triazine derivatives – prediction of the retention behaviour. Chem. Ind. 2011;65:533-540.
-
16.
Jevrić L, Velimirović S, Koprivica G, Mišljenović N, Kuljanin T, Tepić A. Prediction og s-triazine components lipophilicity of total herbicides. Rom. Biotehnolog. Lett. 2012;17:6882-6892.
-
17.
Buchwald P, Bodor N. Computer-aided drug design: The role of quantitative structure-property, structure-activity, and structure-metabolism relationships (QSPR/QSAR/QSMR). Drug Future. 2002;27:577-588.
-
18.
Djaković Sekulić T, Perišić Janjić N. Study of the characteristics and separating power of unconventional tlc supports. ii. principal-components analysis. J. Planar Chromatogr. 2007;20:7-11.
-
19.
Caričić GB. Thermal dealkylation of N-alkylamino of s-triazine derivatives. Correlation between mass spectrometry and pyrolitic reactivity. Serbia: Faculty of Technology and Metallurgy, University of Belgrade; 1985. p. 59-67.
-
20.
Jovanović BŽ. Study the kinetics of reactions of thermal dealkylation of 2,4-bis-(alkylamino)-6-chlor-s-triazine. Serbia: Faculty of Technology and Metallurgy, University of Belgrade; 1978. p. 51-56.
-
21.
Muškatirović MD, Jovanović BŽ, Caričić GB, Tadić ŽD. Thermal Dealkylation of 2,4-Bis(alkylamino)-6-chloro-s-triazines. effect of ring size on formation of Alicyclic Olefins. J. C. S. Perkin II. 1978;9:948-951.
-
22.
Thurston T, Dudley R, Kaiser W, Hechenbleikner I, Schaefer C, Holm-Hansen J. Cyanuric Chloride Derivatives, 1. (Aminochloro)-s-triazines. J. Am. Chem. Soc. 1951;73:2981-2983.
-
23.
Soczewinski E, Wachtmeister CAJ. The relation between the composition of certain ternary two-phase solvent systems and RM values. Chromatogr. 1962;7:311-320.
-
24.
-
25.
-
26.
-
27.
Perišić-Janjić N, Đaković-Sekulić T, Jevrić L, Jovanović B. Study of quantitative structure-retention relationships for s-triazine derivatives in different RP HPTLC systems. J. Planar Chromatogr. 2005;18:212-216.
-
28.
Podunavac Kuzmanović SO, Jevrić LR, Kovačević SZ, Kalajdžija ND. Chemometric Approach for Prediction of Antifungal Activity of some Benzoxazole Derivatives Against Candida albicans. Apteff. 2012;43:273-282.
-
29.
Kaliszan R. QSPR: Quantitative Structure-(Chromatographic) Retention Relationships. Chem. Rev. 2007;107:3212-3246. [PubMed ID: 17595149].