Omics-Driven Biotechnology for Industrial Applications
- 1Lawrence Berkeley National Laboratory, Joint BioEnergy Institute, Emeryville, CA, United States
- 2Biological Systems and Engineering, Lawrence Berkeley National Laboratory, Berkeley, CA, United States
- 3U.S. Department of Energy, Agile BioFoundry, Emeryville, CA, United States
Biomanufacturing is a key component of biotechnology that uses biological systems to produce bioproducts of commercial relevance, which are of great interest to the energy, material, pharmaceutical, food, and agriculture industries. Biotechnology-based approaches, such as synthetic biology and metabolic engineering are heavily reliant on “omics” driven systems biology to characterize and understand metabolic networks. Knowledge gained from systems biology experiments aid the development of synthetic biology tools and the advancement of metabolic engineering studies toward establishing robust industrial biomanufacturing platforms. In this review, we discuss recent advances in “omics” technologies, compare the pros and cons of the different “omics” technologies, and discuss the necessary requirements for carrying out multi-omics experiments. We highlight the influence of “omics” technologies on the production of biofuels and bioproducts by metabolic engineering. Finally, we discuss the application of “omics” technologies to agricultural and food biotechnology, and review the impact of “omics” on current COVID-19 research.
Introduction
Biotechnology employs biological processes, organisms, or systems to yield products and technologies that are improving human lives (Bhatia, 2018). The use of biological systems to manufacture bioproducts of commercial relevance (i.e., biomanufacturing) is a key component of the biotechnology industry. This manufacturing approach is used by the energy, material, pharmaceutical, food, agriculture, and cosmetic industries (Zhang et al., 2017). The bioproducts made from biomanufacturing processes are typically metabolites and proteins, which can be obtained from cells, tissues, and organs. The biological systems producing these bioproducts can be natural or modified by genetic engineering (Zhang et al., 2017), metabolic engineering (optimizing metabolic networks and pathways for increased production of metabolites and/or by-products), synthetic biology (applying engineering principles to the chemical design of biological systems), and protein engineering (optimizing protein design to develop valuable proteins) (Zhang et al., 2017).
Modern biotechnology-based biomanufacturing started in the early twentieth century with the production of short-chain alcohols and ketones, amino acids, organic acids, and vitamin C by microbial mono-culture fermentation (Zhang et al., 2017). This was followed by the production of antibiotics via microbial fermentation in the 1940s and protein drug production in animal cells and microorganisms in the 1980s (Zhang et al., 2017). With the advent of translational research (e.g., stem cell research) in the 2000s, the bioproducts can now be engineered cells, tissues, and organs (e.g., by stem cell engineering) (Roh et al., 2016; Zhang et al., 2017). In addition to this, advancements in synthetic biology and metabolic and protein engineering have been applied to renewable energy research in the development of advanced biofuel and hydrogen production by engineered microorganisms (Zhang et al., 2017). Research efforts are underway at bioenergy research centers (e.g., US DOE Bioenergy Research Centers) to make biofuels more affordable by coproducing them with renewable bioproducts. This is of great importance, as environmental, geopolitical, and economic factors are reshaping our view of global energy and manufacturing demands (Clomburg et al., 2017). The tools (and ideology) from these approaches have also been leveraged by the food industry to produce artificial food products via synthetic biocatalysts in a sustainable way (Zhang et al., 2017).
Synthetic biology and metabolic engineering can benefit from systems biology approaches, which in turn rely on “omics” technologies to characterize and understand metabolic networks. The considerable amount of knowledge obtained from omics-driven systems biology experiments can be used in the development of synthetic biology tools and the advancement of metabolic engineering. This facilitates the manipulation of complex biological systems toward establishing robust industrial biomanufacturing platforms (Baidoo and Teixeira Benites, 2019).
In this review, we aim to examine the influence of “omics” technologies on biotechnology research. “Omics” techniques were compared to understand their relevance and applicability to biotechnology research, especially in the context of microbial biotechnology, with the aim of facilitating the experimental design of individual “omics” and multi-omics studies. Finally, we compared the trends in “omics” utilization during the last two decades to determine their progression in biotechnology research.
A Comparison of the Major “Omics” Technologies
Biological engineering requires the accurate prediction of phenotype from genotype. Thus, testing and validating modified and synthesized genomes (i.e, genomics) as well as the study of the transcriptome (the complete set of RNA transcripts), which is expressed from the genome (i.e., transcriptomics), are crucial to evaluating genome engineering. Proteomics and metabolomics have also gained a lot of attention due to their provision of metabolic information pertaining to both function and phenotype (Baidoo, 2019). Forty years ago, scientists realized that the flow of biochemical information in biological systems is not unidirectional from the genome to metabolome, but rather a set of interactions between the “omes” (Roberts et al., 2012). Therefore, a multi-omics approach is necessary for the elucidation of chemical structure, function, development, adaptation, and evolution of biological systems for deeper understanding of the principles of life (Baidoo, 2019) (Figure 1).
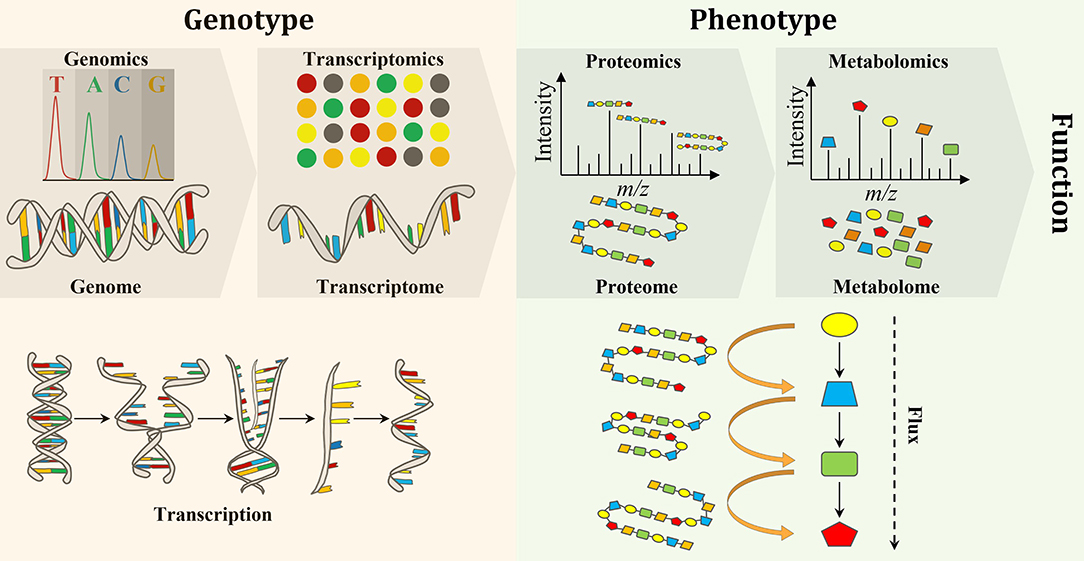
Figure 1. An overview of the flow of molecular information from genes to metabolites to function and phenotype, and the interactions between the “omes” and the “omics” techniques used to measure them.
In comparison to metabolites and proteins, genes are less chemically heterogeneous. Each gene is made up of DNA that is composed of only four basic nucleotides (i.e., guanine, adenine, cytosine and thymine), whereas each protein is composed of a mixture of 32 amino acids, while metabolites are much more variable in their chemical structures (Wang et al., 2010). Therefore, it is analytically less challenging to perform genomics and transcriptomics, when compared to proteomics and metabolomics (Aizat et al., 2018). Consequently, genomics and transcriptomics provide the most comprehensive and robust platforms for biotechnology applications. Over the past few decades, research has shown that genomics and transcriptomics cannot solely provide a complete description of complex biological systems as genetic information can produce more questions than answers. For instance, genomics can describe genes and their interactions (measure genotype) but cannot explain phenotypes. Thus, the attention is turned to the utilization of other “omics” techniques, such as proteomics and metabolomics, which can bridge the gap between genetic potential and final phenotype to facilitate a greater understanding of biological systems (Smith and Figeys, 2006; Wilmes et al., 2015). While transcriptomics (transcription) and proteomics (translation) provide information on gene expression, the latter directly links genotype to phenotype. In addition to providing phenotypic information, the metabolome provides an instant response to genetic and/or environmental perturbations and, therefore, provides a snap shot of the actual metabolic and physiological state of a cell (Tang, 2011). However, metabolomics alone is not able to measure changes at the gene level and correlate them with the observable properties of organisms, the phenotypes, which are produced by the genotype in the first place (Fiehn, 2001). Therefore, a comprehensive understanding of an organism on a molecular level requires the integration of “omics” data in order to discover new molecules and pathways (Wang et al., 2010) (Figure 1). Integration of “omics” data helps to assess the flow of information from one “omics” level to the other and, therefore, links genotype to phenotype (Subramanian et al., 2020). Furthermore, the combination of “omics” techniques is important to address open biological questions (i.e., data driven research) that accelerate our understanding of the system as a whole and boost the use of systems metabolic engineering tools in industrial settings (Zhao et al., 2020).
Genomics and Transcriptomics
The construction of predictable and preferred phenotypes is crucial in synthetic biology; therefore, tight and tunable control of gene expression is highly desirable. Biological engineering, moreover, is greatly benefiting from the recent innovations in genomics and genome editing technologies, which offer advanced tools to re-engineer naturally evolved systems and to build new systems as well. In addition, advances in de novo synthesis and in vivo gene targeting enable efficient testing of model-driven hypotheses. Furthermore, genomics allows the high-throughput DNA sequencing and large-scale bimolecular modeling of metabolic and signaling networks in natural and engineered strains (Pagani et al., 2012).
Genomics and Transcriptomics Analysis
One of the challenges facing traditional genomics (and other “omics”) analyses is that not all microorganisms can be cultured in a laboratory setting. Furthermore, isolated strains might behave differently in culture than in their natural environments. Therefore, there was an urgent need to develop cultivation independent methods to study microbial communities (VerBerkmoes et al., 2009). Metatranscriptomics can reveal the diversity of active genes within microbial communities (e.g., 16S rRNA sequencing for reconstructing phylogenies) (Bashiardes et al., 2016).
Metagenomics studies the structure and function of genetic material in complex samples of multi-organisms as well as of entire microbial communities without a cultivation step and can offer a solution for such challenges and facilitate the discovery of novel genes, enzymes, and metabolic pathways. Metagenomics analyses are classified as sequence-based and function-based screening, which are used to discover and identify, respectively, novel natural genes and compounds from environmental samples (Chistoserdova, 2010; Gilbert and Heiner, 2015; Kumar Awasthi et al., 2020). For example, metagenomics is actively used in agricultural research to understand the microbial communities in the soil system (Durot et al., 2009), to examine various microbes that can stimulate the cycling of macro- and micro-nutrients, and the release of essential enzymes, which enhance crop production (Cupples, 2005).
Nanopore sequencers are massively parallel sequencing technologies. Oxford Nanopore Technologies (ONT), in particular, provides a single molecule sequencer using a protein nanopore that realizes direct sequencing without DNA synthesis or amplification (Brown and Clarke, 2016; Roumpeka et al., 2017). The ONT sequencer can determine DNA/RNA modifications and sequence an ultra-long read limited by the input nucleotide length (Kono and Arakawa, 2019). However, ONT reads require polishing and great care needs to be taken when contigs are polished individually to avoid the removal of true, natural sequence diversity due to cross mapping of reads in repeat regions. Therefore, it was found that it is crucial to apply long-range information technologies (e.g., 10x genomics, Hi-C, synthetic long reads) and to develop new algorithms to simplify the extensive assembly and polishing workflow (Somerville et al., 2018).
Sort-seq is a single-cell sequencing platform, which combines flow cytometry, binned fluorescence-activated cell sorting (FACS), next-generation sequencing (NGS), and statistical inference to quantify the dynamic range of many biosensor variants in parallel (Rohlhill et al., 2017; Batrakou et al., 2020; Koberstein, 2020). FACS, which enables the sorting of single cells, allows the enrichment of specific cells to generate high-resolution gene expression and transcriptional maps (Kambale et al., 2019). NGS and RNA sequencing (RNA-seq) technologies enable the large-scale DNA and RNA sequencing of the entire genome and transcriptome, respectively, providing an unbiased and comprehensive view of biological systems towards understanding genomic function (Frese et al., 2013; Alfaro et al., 2019; Stark et al., 2019). Examples of NGS platforms include the Illumina HiSeq, Genome Analyzer Systems, 454 Genome Sequencer FLX Titanium System, the Helicos HeliScope, the SOLiD sequencing platform and the Ion Torrent Sequencing platform. In addition, there are other techniques used to measure the interaction between proteins and DNA, such as chromatin immunoprecipitation (ChIP). ChIP followed by NGS sequencing (ChIP-seq) has high potential for detailing the binding sites of various transcription factors and assaying protein–DNA interaction at a whole-genome level (Roukos, 2012).
Bar-seq (barcode analysis by sequencing) is another high-throughput quantitative DNA sequencing technique that enables the parallel phenotyping of pools of thousands of mutants and monitoring thousands of gene-chemical interactions (Smith et al., 2010; Robinson et al., 2014). Techniques, such as bar-seq can lower the complexity of data obtained from a large number of sequence reads, thus, making NGS more efficient and affordable (Smith et al., 2009).
New computational tools have enabled researchers to perform fast and accurate analysis of big genomics data. Extracted genomic information has been used to model metabolic processes and signaling networks across the entire cell, generating many new testable hypotheses (Lewis et al., 2012; Esvelt and Wang, 2013). Due to the robustness of genomic measurements, there are numerous genomics databases and data analysis tools available (Roumpeka et al., 2017).
Proteomics
Proteomics focuses on the analysis of proteins and peptides produced by cells at different stages of development and life cycle and in biological systems under a given growth condition. Proteomics is also used to elucidate the temporal dynamics of protein expression levels or post-translational modification (PTM) (VerBerkmoes et al., 2009).
Proteomics Sample Preparation
High biological sample diversity and complexity and the dynamic range of protein levels present in such samples are the main challenges that proteomics encounter. These factors, in addition to the large number of proteins, complicate the analysis of low abundance proteins. The development of automated sample preparation workflows are becoming more common for high-throughput, quantitative proteomic assays of microbes. One automated workflow was able to quantify >600 peptides with a 15.8% median coefficient of variation, demonstrating the robustness of this approach (Chen et al., 2019). Another high-throughput automatable workflow was developed to increase the yield of lysis of several representative bacterial and eukaryotic microorganisms via vigorous bead-beating with silica and glass beads in presence of detergents (Hayoun et al., 2019). Interestingly, a universal, high-throughput and a detergent-free sample preparation protocol was developed this year for peptide generation from various microbes [i.e., Escherichia coli (E. coli), Staphylococcus aureus and Bacillus cereus]. The protocol holds the potential to dramatically simplify and standardize sample preparation while improving the depth of proteome coverage especially for challenging samples (Doellinger et al., 2020).
Proteomics Data Acquisition
Protein Identification and Structural Elucidation
Most proteomics workflows are based on a bottom-up approach, where protein is extracted, digested (e.g., trypsin digestion) into proteolytic peptides, then analyzed via MS (Kleiner et al., 2017). When liquid-chromatography is coupled to mass spectrometry (LC-MS), both qualitative and quantitative data analysis of proteins are improved. Moreover, the application of multidimensional LC separation prior to MS protein analysis provides enhanced MS sensitivity by reducing sample complexity and increasing the number of chromatographic peaks that can be resolved in a single analytical run (Hinzke et al., 2019; Duong et al., 2020). Targeted proteomics via LC-tandem MS (LC-MS/MS) is a commonly used MS method, where the analysis focuses on a subset of biological proteins of interest (Marx, 2013). When a bottom-up approach is applied to all proteins within a given biological system, it is called shotgun (untargeted) proteomics (Wolters et al., 2001; Nesvizhskii and Aebersold, 2005). Top-down proteomics, conversely, is based on the analysis (via LC-MS or LC-MS/MS) of intact proteins, and thus, provides unique information about the molecular structure of proteins (e.g., PTM) (Catherman et al., 2014). However, it is not always possible to separate intact proteins, especially large proteins, prior to MS analysis in a top-down approach. Besides that, top-down is less sensitive and has a lower throughput than a bottom-up approach (Catherman et al., 2014).
Accurate determination of protein structure helps to define their roles and functions in biological systems. However, many folded proteins have complex structures, which complicates their structural elucidation (Yates, 2019). Therefore, cryogenic electron microscopy and ion-mobility-MS are used to determine the structures of such proteins such proteins (Yates, 2019). Moreover, a combination of MALDI, high resolution MS (i.e., orbitrap and ion trap MS) and a UV–Vis-based reduction assay is used to elucidate peptide modification via the analysis of specific fragmentation of synthesized peptides, which might have inhibitory effects on various diseases (Rühl et al., 2019).
The identification of PTM peptides can be difficult in the case of labile modifications (e.g., phosphorylation and S-nitrosylation) that might break down during MS/MS fragmentation. Such modifications require soft fragmentation and high-resolution methods to identify and determine the location of a PTM. Electron-transfer dissociation is considered to be the favorable choice for the identification of liable PTM as it transfers electrons to multi-protonated proteins or peptides, which leads to N-Cα backbone bond cleavage (Chen et al., 2017).
Metaproteomics is the large-scale characterization of the entire protein complement of environmental microbiota at a given point in time to determine structure (Wilmes and Bond, 2004; Kleiner et al., 2017), metabolism and physiology of community components (Kleiner et al., 2012). The recent advancement in LC and high-resolution MS have enabled the identification and quantification of more than 10,000 peptides and proteins per sample in metaproteomics (Kleiner, 2019). Metaproteomics can also measure interactions between community components (Hamann et al., 2016) and assess substrate consumption (Bryson et al., 2016; Kleiner et al., 2018).
Protein Quantification
Besides identification, MS-based technologies became the tools of choice for the quantification of proteins in an organism (Karpievitch et al., 2010). Stable isotope labeling is one approach that can be used to quantify proteins by measuring the relative abundance of labeled protein to non-labeled protein (VerBerkmoes et al., 2009). However, the variation in ionization efficiency among peptides and proteins and the low recovery of some peptides (e.g., hydrophobic peptides adhere to surfaces) can affect the accuracy of their direct quantification. Recent advances in MS acquisition rate, detection, and resolution have addressed much of the sensitivity concerns of MS-based quantification for proteomics (Iwamoto and Shimada, 2018). MS sensitivity was further enhanced with the application of micro-flow (Krisp et al., 2015; Bian et al., 2020) and nano-flow (Wilson et al., 2015) LC-MS. Another major advancement for global protein quantification was the introduction of isobaric tags or multiplexed proteomics, which in a single experiment enables the quantification of proteins across multiple samples (Pappireddi et al., 2019). Tandem-mass-tags are examples of commonly used isobaric tags for instance in human cerebrospinal fluids (Dayon et al., 2008).
Proteomics Data Analysis
Proteomics data analysis tools are generally used for protein identification (via bioinformatics) and quantification, and bioinformatics techniques tools used to process the proteomics data. A few examples of data analysis tools that are used for the identification of peptides and proteins include Mascot (Eng et al., 1994), Swiss-Prot (Bairoch and Boeckmann, 1994), Sequest (Perkins et al., 1999), Tandem (Craig and Beavis, 2004), Skyline (MacLean et al., 2010), Uni-Prot,1 UniNovo (Jeong et al., 2013), and SWPepNovo (Li et al., 2019). Such algorithm-based software were developed to match the MS collected data from peptide/protein analysis to their base peptides/proteins and with in silico predicted intact masses and fragmentation patterns (Urgen Cox and Mann, 2011). Moreover, they determine both the mass and exact location of any possible modifications (Hansen et al., 2001; Savitski et al., 2006). Common bioinformatics techniques tools for proteomics data analysis include CRONOS (Waegele et al., 2009), COVAIN (Sun and Weckwerth, 2012), SIGNOR (Perfetto et al., 2016), KEGG (Kanehisa et al., 2017), and STRING v11 (Szklarczyk et al., 2019).
Metabolomics
Metabolomics, which is the measurement of small molecule substrates, intermediates, and/or end products of cellular metabolism (i.e. metabolites), provides an immediate and dynamic response to genetic and/or environmental perturbations in a biological system (Fiehn, 2002; Ellis and Goodacre, 2012; Zhao et al., 2020). Targeted and untargeted metabolomics are used to quantify a group of defined metabolites and determine all measurable metabolites in a biological sample, respectively (Scalbert et al., 2009). MS-based metabolomics, like proteomics, normally employs separation [e.g. LC and gas chromatography (GC)] or capillary electrophoresis (CE) before MS detection (Fiehn, 2002). Whereas, MALDI-MS conducts high-throughput screening without separation.
Nuclear magnetic resonance (NMR) spectroscopy is a powerful analytical technique for high-throughput metabolic fingerprinting and provides more reliable metabolite structure (e.g., via 2D NMR) identification than MS (Giraudeau, 2020). However, although NMR offers unambiguous structure determination of unknown metabolites via 1H- and 13C-NMR, MS-based methods comprise widely accessed metabolomics techniques due to higher sensitivity and lower instrumentation cost (Chatham and Blackband, 2001). Furthermore, NMR is semi-quantitative whereas MS is quantitative, thus, NMR and MS are highly complementary techniques. In addition, the diverse physiochemical properties (e.g., solubility, reactivity, stability, and polarity) of the metabolome limits our ability to analyze all metabolites from a biological system with a single or even a limited-set of analytical techniques (Fiehn, 2002). Therefore, multiple methods are used for comprehensive metabolome characterization.
Metabolomics Sample Preparation
Metabolites are constantly going under reformation and transformation in biochemical reactions within a cell and/or being thermally degraded (and in some cases oxidized) at ambient conditions (Scalbert et al., 2009). Therefore, quick and efficient metabolic quenching protocols are required to accurately quantify metabolic information. Not surprisingly, researchers tend to develop metabolic quenching methods in conjunction to and metabolite extraction protocols. Doran et al. (2017), for example, proposed an acidic-based metabolic quenching to aqueous-alcohol metabolite extraction. This protocol yielded low metabolite leakage and high extraction recovery in Acidithiobacillus ferrooxidans. Complex biological sample matrices can also suppress metabolite MS detection. Thus, clean up strategies, such as solid phase extraction (SPE) and solid phase micro-extraction (SPME) can reduce the complexity of sample matrices prior to LC-MS and GC-MS analysis, thereby increasing the quantitative capability of metabolomics methods (Yang et al., 2011). The last 5 years witnessed the development of high-throughput 96-well plate SPE (Li et al., 2015) and 96-well automated SPME (Mousavi et al., 2015) for the simultaneous extraction of metabolites and lipids from biological samples.
In addition, robotics and microfluidics tools can be applied to high-throughput synthetic biology applications by automating cell preparation and metabolite extraction to increase coverage (Yizhak et al., 2010; Koh et al., 2018; Vavricka et al., 2020). Automated liquid handler technologies, therefore, are important for high-throughput sample preparation as they ensure good quality and reproducibility of sample extraction and processing for unbiased measurement of metabolic differences (e.g., based on disease states or interventions stimuli) (Liu et al., 2019).
Metabolomics Data Acquisition
The development of nanoelectrospray-ionization and direct infusion nanoelectrospray high-resolution MS have led to a considerable increase in the dynamic range and detection sensitivity of metabolites from tissues and biofluids in human studies (Chekmeneva et al., 2017; Southam et al., 2017). Generally, nanospray technology is more sensitive than electrospray, but suffer from low robustness. However, nanoelectrospray employs low level of nebulization and flow rate to achieve high sensitivity without compromising robustness (Guo et al., 2016). Besides, the application of ion mobility and high resolution MS has improved the identification of isomers, thereby enabling a more accurate assessment of their biological roles (Ren et al., 2018; Rathahao-Paris et al., 2019). Moreover, new developments in orbitrap MS systems have improved metabolites annotation and coverage in GC- and LC-MS studies (Simirgiotis et al., 2017; Misra et al., 2018; Manier et al., 2019; Stettin et al., 2020).
Although GC-MS requires more sample preparation steps when derivatizing hydrophilic non-volatile metabolites, it is more robust than LC-MS. Moreover, method development is easier for GC-MS than for LC-MS. GC-MS also achieves better identification of untargeted metabolites due to standardized ionization conditions, which makes it possible to set up a universal compound identification library/database, such as NIST. While CE achieves the highest separation efficiency, CE-MS is the least robust and sensitive of the three separation techniques.
Real-time metabolomics enables the simultaneous and high-throughput analysis of microbial metabolites without the need for time-consuming sample preparation steps (Link et al., 2015; Boguszewicz et al., 2019; Nguyen et al., 2020). However, the lack of chromatographic or electrophoretic separation in this approach reduces the quantitative capability of this technique (Baidoo and Teixeira Benites, 2019). While MALDI can be used for high-throughput metabolite screening, MALDI imaging MS has emerged as a powerful tool for analyzing tissue specimen in an unprecedented detail. MALDI imaging MS has made significant contributions to the understanding of the biology of disease and its perspectives for pathology research and practice, as well as in pharmaceutical studies (Aichler and Walch, 2015; Mahajan and Ontaneda, 2017; Schulz et al., 2019).
Metabolomics technologies are regularly applied to metabolic flux analysis (MFA, i.e., 13C) studies (Baidoo and Teixeira Benites, 2019). MFA determines the rates of in vivo metabolic reactions. Thus, enabling an understanding of carbon and energy flow throughout the metabolic network in a cell. Overall, MFA accelerates the discovery of novel metabolic pathways and enzymes for improved synthetic bioproduction (Feng et al., 2010; Ando and García Martín, 2019; Babele and Young, 2019; Vavricka et al., 2020). However, the availability and high cost of stable isotope compounds can limit MFA capability (Gonzalez and Pierron, 2015).
Metabolomics Data Analysis
Multivariate data analysis methods, such as principal component analysis (PCA) and partial least squares (PLS) analysis are used to analyze large quantities of metabolic profiling data (i.e., reveal clustering-based on features). In addition, there is a need for advanced pathway analysis tools to interpret metabolomics data to solve some of the most challenging biological paradoxes and reveal optimal conditions for biological systems. Such techniques enable systems biology researchers to utilize metabolomics data as a resource that contributes to an iterative cycle of hypothesis generating and hypothesis testing phases (Kell, 2004; Vavricka et al., 2020). To address all of this, more attention is being paid to the area of big data and machine learning. Thus, the state-of-the-art understanding of cell metabolism can be improved and further combined with mechanistic models to automate synthetic biology and intelligent biomanufacturing (Oyetunde et al., 2018). To this end, recent advancements in metabolomics tools for data analysis, storing and sharing have been developed [e.g., WebSpecmine (Cardoso et al., 2019), SIRIUS 4 (Dührkop et al., 2019), MetaboAnalyst 4.0 (Chong et al., 2018), and SECIM (Kirpich et al., 2018)]. Knowledge of biology (e.g., regulation, metabolism, physiology, etc.) is still, however, necessary for efficient experimental design and accurate data interpretation in order to understand and accurately characterize biological systems.
Multi-Omics for Systems Biology
The recent advancement in omics technologies has improved the analysis efficiency by reducing cost and time, but also by collecting informative and meaningful multi-omics data. Thus, facilitating the implementation of multi-omics techniques in systems biology studies. However, integrating multi-omics platforms is still an ongoing challenge due to their inherent data differences (Saito and Matsuda, 2010; Yizhak et al., 2010; Brunk et al., 2016; Koh et al., 2018; Pinu et al., 2019; Vavricka et al., 2020). For example, genomics data are qualitative, accurate and reproducible, while other “omics” data, such as proteomics and metabolomics are both qualitative and quantitative, not as reproducible, and noisy (Kuo et al., 2002; MacLean et al., 2010; Guo et al., 2013; Gross et al., 2018). Further, multi-omics data is normally pre-treated by various data treatment methods (e.g., deconvolution, normalization, scaling, and transformation) and software before being integrated. Multi-omics studies also require experts in their respective “omics” fields (as well as IT support) to validate multi-omics data. While this provides greater data interpretation accuracy it does, however, complicate data acquisition and analysis.
Recently, Pinu et al. discussed some recommendations to overcome the major challenge facing the implementation of multi-omics techniques in systems biology, which is the differences among their inherent data. The aim of their recommendations is to make researchers aware of the importance of having a proper experimental design in the first place. Thus, the appropriate biological samples should be carefully selected, prepared, and stored before planning any “omics” study. Afterward, researchers should carefully collect quantitative multi-omics data and associated meta-data and select better tools for integration and interpretation of the data. Finally, develop new resources for the deposition of intact multi-omics data sets (Pinu et al., 2019). It is also necessary to select or develop methods that keep the optimum balance between high recovery and low degradation of extracted biological features.
As scientists are becoming more aware of the importance of multi-omics analysis, a number of tools, databases, and methods are being developed for the aim of integrating multi-omics data sets. These tools perform advanced statistics (e.g., multivariate data analysis) and data illustration (e.g., correlation maps). Examples of databases used for multi-omics analysis include ECMDB 2.0 (Sajed et al., 2016), Saccharomyces Genome Database (MacPherson et al., 2017), YMDB 2.0 (Ramirez-Gaona et al., 2017), GenBank (Benson et al., 2013), KEGG (Kanehisa and Subramaniam, 2002), and many others. A recent review by Subramaniam et al. showed that common multi-omics data integration and interpretation tools were able to derive new insights from data, conduct disease subtyping, and obtain diagnostic biomarker prediction (Subramanian et al., 2020).
Table 1 provides a comprehensive comparison of the major “omics” technologies. The aim of this comparison is to facilitate the experimental design of individual “omics” and multi-omics studies by highlighting the general characteristics of each technology.
Omics-Guided Biotechnology
“Omics” technologies are becoming increasingly involved in the development of biotechnological processes for the production of many substantial products. The use of “omics” technologies to characterize and understand biological systems has enabled researchers to select and predict phenotypes (Abid et al., 2018; Babar et al., 2018), which aids the optimization of biotechnological processes toward enhanced production (in quality and quantity) of commercially relevant products (Figure 2). This section discusses the application of “omics” in the development of biofuels and bioproducts, agricultural biotechnology, food biotechnology, and bio-therapeutics. In addition, this section discusses the involvement of “omics” technologies in the development of bio-therapeutics for COVID-19.
Omics-Guided Metabolic Engineering of E. coli and Yeast Toward the Production of Primary and Secondary Metabolism-Based Biofuels and Bioproducts
Microbial production of bio-based chemicals represents an appealing and more sustainable alternative to traditional petrochemicals (Opgenorth et al., 2019) and has led to a growing catalog of natural products and high-value chemicals (Carbonell et al., 2018). The use of lignocellulosic biomass offers an economical approach to generate biofuels and bioproducts (Fatima et al., 2018). However, to achieve consistent conversion of low-cost input material into value-added products (Yan and Fong, 2018) at industrial levels requires systematic engineering workflows.
The Design-Build-Test-Learn (DBTL) cycle is becoming an increasingly adopted frame-work for metabolic engineering experiments (Opgenorth et al., 2019). It represents a systematic and efficient approach to strain development efforts in biofuels and bio-based products (Opgenorth et al., 2019). Growing interest in the DBTL cycle for metabolic engineering is largely due to improving capabilities in synthetic biology (e.g., synthetic biology tools, DNA synthesis, and genome editing), “omics” technologies, and Learning methods (Carbonell et al., 2018; Opgenorth et al., 2019; Robinson et al., 2020). The DBTL cycle uses synthetic biology to Design and Build genetic constructs in microbial hosts, after which the information gained from “omics” technologies, during the Test phase of the cycle, is passed on to Learning processes (Figure 3). What is Learned (e.g., COBRA models) is then fed back to new cycles of design to advance the engineering biology goal (Vavricka et al., 2020) for further strain development and optimization. Thus, facilitating the rapid optimization of microbial strains for production of any chemical compound of interest (Carbonell et al., 2018). Arguably the weakest link in the DBTL cycle workflow is the Learning process since mathematical models (of the engineered bioproduct, pathway, biological system, or biome) are only as good as their assumptions (Liu and Nielsen, 2019). Consequently, both high quality and large “omics” data sets are necessary to improve training models, ensuring increased accuracy and robustness of the Learning process.
Recently, Geiselman et al. utilized the DBTL cycle to engineer Rhodosporidium toruloides, an oleaginous yeast species with the ability to grow on lignocellulosic feedstock, to produce the diterpene ent-kaurene, a potential therapeutic, by the native mevalonate pathway and the non-native production of the diterpene precursor geranylgeranyl diphosphate (GGPP). Multi-omics data, in the first round of the DBTL cycle, suggested a limited availability of GGPP. In successive DBTL cycles, an optimal GGPP synthase (GGPPS) was selected, whose expression was balanced with the addition of kaurene synthase from Gibberella fujikuroi and a mutant version of farnesyl diphosphate (FPP) synthase from Gallus gallus that produces GGPP under strong promoters. The higher ent-kaurene titer achieved was the first demonstration of the production of a non-native diterpene from lignocellulosic hydrolysate in Rhodosporidium toruloides (Geiselman et al., 2020). Additionally, Opgenorth et al. used the DBTL cycle approach to optimize 1-dodecanol production in E. coli MG1655 strains by modulating ribosome binding sites and acyl-ACP/acyl-CoA reductase on a single operon. The proteomics and metabolomics data collected during the first DBTL cycle were used to train the Learning algorithms, with protein profiles being used to suggest the second DBTL cycle, which led to a 21% increase in 1-dodecanol titer. While this resulted in a 6-fold increase in what was previously reported, the study, however, highlighted the need for more accurate protein expression predictive tools and the importance of genomic sequencing checks on plasmids in cloning and production strains to establishing robust microbial cell factories (Opgenorth et al., 2019).
Adaptive laboratory evolution (ALE) studies the evolutionary forces and adaptive changes influencing microbial strain phenotypes, performance, and stability in order to acquire production strains containing beneficial mutations and positive traits (Dragosits and Mattanovich, 2013; Sandberg et al., 2019; Gibson et al., 2020). Microorganisms are cultured in a desired growth environment for an extended period of time, allowing natural selection to enrich for mutant strains with improved fitness (Johansen, 2018; Sandberg et al., 2019). Therefore, the throughput of ALE will depend on the experimental design. Furthermore, the task of identifying all beneficial mutations of an ALE experiment remains a major challenge for the field (Phaneuf et al., 2020). ALE is often used to optimize microbial growth rate, increase strain tolerance, improve stress regulation and adaptation, improve substrate utilization and uptake, increase product titer/rate/yield, as well as for biological discovery via systems biology, evolutionary modeling, and genome dynamics (Bergh, 2018; Dourou et al., 2018; Sun et al., 2018; Wang et al., 2018; Yan and Fong, 2018; Sandberg et al., 2019; Phaneuf et al., 2020). ALE experiments allow researchers to learn how to improve multiple strain properties simultaneously (Sandberg et al., 2019).
ALE has become a valuable tool in metabolic engineering for strain development and optimization by reliably facilitating microbial fitness improvements, via both predictable and non-intuitive mechanisms (Sandberg et al., 2019). ALE can be employed in the DBTL cycle in the Build step to recover strains with fitness issues or to optimize strains (Sandberg et al., 2019). Furthermore, ALE can be used in the Design step to enrich for mutant strains with improved fitness and can also replace both Design and Build steps in situations where a desired phenotype is tied to selection without the need for engineering (Sandberg et al., 2019; Lee and Kim, 2020). While ALE can precede the Test and Learn steps in the DBTL cycle, the information gained from these steps can also be utilized by ALE to produce strains with better properties. In this way, ALE may benefit from using “omics” technologies during the Test phase of the DBTL cycle (Horinouchi et al., 2018; Long and Antoniewicz, 2018; Walker et al., 2019; Wu et al., 2020).
In most microbial metabolic engineering studies, however, the Learn phase of the DBTL cycle is often addressed by hypothesis-driven user intuition that is often based on empirical evidence (Liu and Nielsen, 2019). As with the DBTL cycle, genomic sequence information in the traditional approach is invariably utilized in the initial stages of a study. However, genomics has come a long way in the last decade. Bar-seq can now be used to study population dynamics of Saccharomyces cerevisiae (S. cerevisiae) deletion libraries during bioreactor cultivation, enabling the identification of factors that impact the diversity of a mutant pool (Wehrs et al., 2020). Whereas, a sort-seq-guided engineering approach can be used to identify key mutated promotors for tuning expression levels, thereby facilitating the dynamic regulation of microbial growth as well as dynamic pathway regulation (Rohlhill et al., 2017). While transcriptomics yields gene expression data (i.e., activity of target genes, gene sequence data, and gene expression levels), proteomics and metabolomics approaches are increasingly being used for pathway analysis studies as they can measure protein translation and activity, respectively (Volke et al., 2019). Proteomics-guided approaches have been used to engineer polyketide biosynthesis platforms for aromatic compounds in yeast (Jakočiunas et al., 2020) and in vitro production of adipic acid (Hagen et al., 2016). In addition to this, metabolomics enables the assessment of pathway flux, carbon source diversion, and cofactor imbalance, which all contribute to the identification of pathway bottlenecks (Nielsen and Jewett, 2007; Zhao et al., 2020, Volke et al., 2019). Luo et al. used a metabolomics guided approach to characterize cannabinoid production in engineered S. cerevisiae and identified cannabinoid analogs produced by several promiscuous pathway genes (Luo et al., 2019). Furthermore, metabolomics analysis aided the design (Kang et al., 2016) and optimization (Kang et al., 2019) of a novel isopentenyl diphosphate-bypass mevalonate pathway in E. coli for C5 alcohol production. With a combined genetic, biochemical and fermentation approach, Uranukul et al. utilized the native glycolytic pathway in S. cerevisiae to produce monoethylene glycol, an important commodity chemical, and upon further metabolic engineering and process optimization were able to achieve 4.0 g/L (Uranukul et al., 2019). The integration of proteomics and metabolomics promises accurate assessment of pathway flux due to proper accounting of protein abundance. When pathway data is obtained in addition to transcriptomics data and/or large scale targeted/untargeted proteomics or metabolomics data, the impact of the engineered pathway on cellular metabolism and physiology can be determined. Exploring the interplay between heterologously expressed pathways and endogenous metabolism could reveal factors affecting strain variation, identify perturbed metabolic nodes, and produce new engineering targets (Chen, 2016).
The Application of “Omics” Technologies to Agricultural and Food Biotechnology
Recent advances in agricultural biotechnology have led to new plant varieties being engineered by recombinant DNA technology and grown by farmers to respond to market demands and environmental challenges (https://www.usda.gov/topics/biotechnology). “Omics” technologies are being applied to agricultural biotechnology to enhance desirable phenotypic traits (e.g., color, taste, drought tolerance, pesticide resistance, etc.) (Aliferis and Chrysayi-tokousbalides, 2011; Van Emon, 2016). While “omics” plays a major role in improving crop quality, consistency, and productivity, they have also led to the development of food crops with enhanced nutritional composition (Van Emon, 2019) (Figure 2). Moreover, omics-driven systems biology provides an understanding of the interactions between the “omes” and mechanisms involved and provide links between genes and traits (Van Emon, 2016).
As arable land is being farmed more heavily, soil is becoming more susceptible to loss of structure, organic matter, minerals, and erosion. Thus, efforts are being made, via agricultural biotechnology, to maintain a sustainable supply of nutrients essential to the growth of crop plants. An integral part of this approach is the use of biofertilizers, which are the preparations containing specialized living organisms (i.e., microbial inoculants) that can fix, mobilize, solubilize, or decompose nutrient sources, and are applied through seed or soil to enhance nutrient uptake by plants (Mohanram and Kumar, 2019). The sustainable enhancement in food production from less available arable land relies on the balanced utilization of inorganic minerals, organic matter, and biofertilizer sources of plant nutrients to augment and maintain soil fertility and productivity. Widespread adoption of this approach, however, has been hindered by varying responses of microbial inoculants across fields and crops (Mohanram and Kumar, 2019). As a result, there is an urgent need to understand the mechanisms underlying the interdependencies between soil microbial communities and the host plant and their impact on crop productivity. These interactions are played out in the rhizosphere, which encompasses the region of soil that is directly influenced by root secretions and associated microbial communities (Zhalnina et al., 2018). In a recent comparative genomics and exo-metabolomics study, specific rhizosphere bacteria were shown to have a natural preference for certain aromatic organic acids exuded by plants, suggesting that plant exudation traits and microbial substrate uptake traits interact to yield the patterns of microbial community assembly (Zhalnina et al., 2018). Furthermore, the application of genomics and transcriptomics to the study of luxury phosphate uptake (i.e., the ability of microalgae to take up more phosphorus than necessary for immediate growth) revealed a range of Pi transporters in various microalgae and their expression patterns in relation to the availability of P (Yang et al., 2018; Mohanram and Kumar, 2019; Solovchenko et al., 2019). At present, “omics” technologies are being used to understand complex rhizospheric intercommunications, which is crucial to the development and choice of biofertilizer and, by extension, the construction of rhizopheres that promote stable plant growth, better crop productivity and yield (Mohanram and Kumar, 2019).
In the related field of food biotechnology, transcriptomics and metabolomics analysis showed that Bacillus pumilus LZP02 promote the growth of rice roots by enhancing carbohydrate metabolism and phenylpropanoid biosynthesis (Liu et al., 2020). Further, the application of “omics” to starch bioengineering is increasing our understanding of the specific contributions of the most important enzymes for starch biosynthesis. This has enhanced our ability to predict how starch-related phenotypes can be modified, thus ensuring further progress in the research field of rice starch biotechnology (Nakamura, 2018). “Omics” are solving issues surrounding food quality and traceability, to safeguard the origin of food, and discover biomarkers of potential food safety problems (Ferranti, 2018). In the wine industry, the wine microbiome associated with the fermentation of must has a great influence on factors transforming grapes to wine, including flavor and aroma. “Omics” characterization of the complex interactions between these microbes, the substrate and environment, is key to shaping wine production (Sirén et al., 2019). Finally, combining “omics” technologies with genome editing of food microbes can be used to generate enhanced probiotic strains, develop novel bio-therapeutics and alter microbial community structure in food matrices (Pan and Barrangou, 2020).
The Use of “Omics” Technologies in the Development of Therapeutics for COVID-19
The coronavirus disease 2019 (COVID-19) characterized by the Severe acute respiratory syndrome coronavirus 2 [i.e., SARS-CoV-2, which binds to the ACE2 receptor in the lung and other organs (Ahmed et al., 2020)] has caused a global pandemic and slowed much of the world's economy. To date (December 5th, 2020), there are more than 64 million confirmed cases and 1.5 million confirmed deaths world-wide (https://www.who.int/emergencies/diseases/novel-coronavirus-2019). Thus, there is a pressing need for an effective countermeasure to mitigate the spread of the pandemic (van Doremalen et al., 2020).
Consequently, efforts are underway to fast-track the development and production of safe and effective vaccines against SARS-CoV-2. Prior knowledge of SARS and Middle East respiratory syndrome (MERS) has enabled scientists to target the spike protein as the viral antigen (via the ACE2 receptor). Moreover, the release of the SARS-CoV-2 genome sequence in January 2020 made it possible to expedite the development of next generation mRNA [e.g., mRNA-1273 from NIH/Moderna (Jackson et al., 2020) and BNT162 from Pfizer/BioNTech (Mulligan et al., 2020)] and DNA [e.g., ChAdOx1 nCoV-19 from University of Oxford/Vaccitech/AstraZeneca (Folegatti et al., 2020; van Doremalen et al., 2020)] vaccine platforms that encode for the antigen. Once injected into a host, the former (which is encased in lipid nano-particles) remains in the cytoplasm while the latter (which is encased in an attenuated adenovirus vector) enters its nucleus. The host cell translates these genetic materials into the spike protein, which decorates the surface of the cell and elicits an adaptive immune response mediated by T cells (e.g., CD4+ and CD8+) and B cells (i.e., antibodies). These vaccines were reported to be efficacious against SARS-CoV-2 in recent clinical trials, which underscores the importance of genomics to this new era of vaccine development.
Gordon et al. produced a SARS-CoV-2 protein interaction map via a proteomics-based approach to reveal targets for drug repurposing. They cloned, affinity tagged and expressed 26 of the 29 SARS-CoV-2 proteins in human cells and identified the associated proteins via proteomics analysis. A total of 66 human proteins or host factors were identified as possible drug targets of 69 compounds, from which two sets of these pharmacological agents showed antiviral activity. This work highlights the potential of host-factor-targeting agents, when acting alone or in combination with drugs that target viral enzymes, to be used as therapeutic treatments for COVID-19 (Gordon et al., 2020). Furthermore, computational immunoproteomics studies have the potential to guide lab-based investigations to evaluate specificity of diagnostic products, to forecast on potential adverse effects of vaccines and to reduce the use of animal models (Tilocca et al., 2020).
Recently, metabolomics was able to distinguish COVID-19 patients from healthy controls via the analysis of 10 plasma metabolites. Additionally, lipidomics data from this study suggests monosialodihexosyl ganglioside enriched exosomes could be involved in pathological processes related to COVID-19 pathogenesis (Song et al., 2020). Recent proteomics and metabolomics studies in COVID-19 patient sera suggest that SARS-CoV-2 infection causes metabolic dysregulation of macrophage and lipid metabolism, platelet degranulation, complement system pathways, and massive metabolic suppression (Shen et al., 2020); with the plasma metabolomic signatures appearing to be similar to those described for sepsis syndrome (Langley et al., 2013, 2014; Migaud et al., 2020). Furthermore, transcriptomics results indicate higher expression of genes related to oxidative phosphorylation both in peripheral mononuclear leukocytes and bronchoalveolar lavage fluid, suggesting a critical role for mitochondrial activity during SARS-CoV-2 infection (Gardinassi et al., 2020). Understanding the clinical presentation of COVID-19 as well as metabolomic, proteomic, and genetic profiles could lead to the discovery of diagnostic, prognostic and predictive biomarkers, ensuring the development of more effective medical therapy (Ahmed et al., 2020). Moreover, identifying metabolic biomarkers of severe vs. mild disease states in the lung during respiratory infections could lead to the development of novel therapeutics that modulate symptom and disease severity (Bernatchez and McCall, 2020; Shen et al., 2020). It is, therefore, critical to develop new approaches to early assess which cases will likely become clinically severe (Shen et al., 2020).
Trends in “Omics” Related Biotechnology Research
The aim of this section is to present the trends in “omics” techniques utilization in biotechnology research (i.e., food, natural products, agriculture, pharmaceutical, materials, and bioenergy) during the course of last two decades. Such trends, which are based on the number of annual publications obtained from a search in Web of Science (www.webofknowledge.com) topics, are used to illustrate the progression of “omics” technologies in biotechnology. In Web of Science, the topics searched are as follows: title, abstract, author keywords, and keywords plus. It is worth noting that the trends in this section showing a reduction in the number of publications for the current year (i.e., 2020), which is expected to be a result of the COVID-19 related shut-down that has affected scientific labs worldwide and the mid-2020 collection of the data. Furthermore, it is also important to note that search entries, such as food, and bioenergy can generate publications based on the contributions of both closely and, to a lesser extent, loosely related topics. Additionally, it is worth noting that not all “omics” research data (e.g., industrial-based studies) is being published and made available to the public. Therefore, the trends data (Figures 4, 5) represents a qualitative rather than a quantitative measure of “omics” utilization.
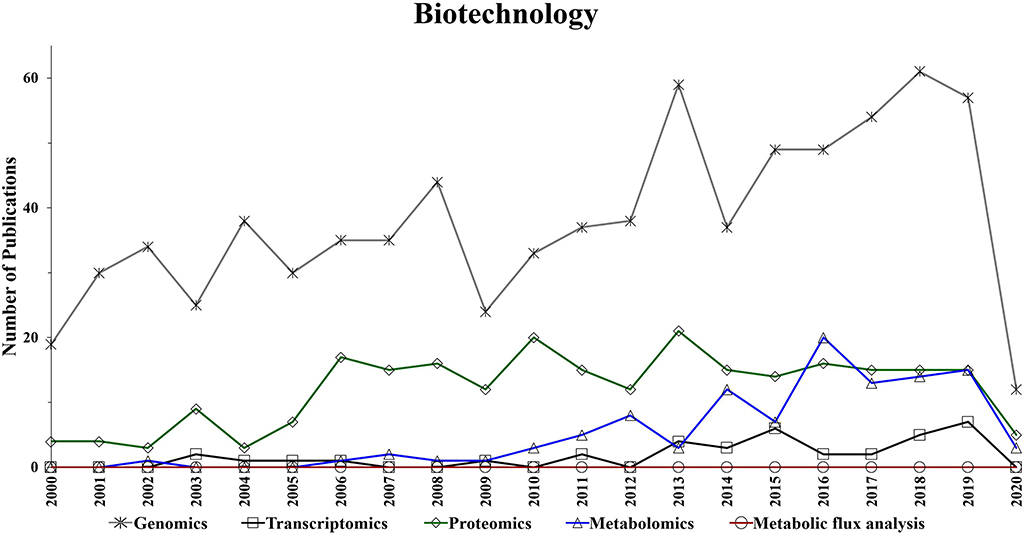
Figure 4. Number of annual publications that utilize “omics” technologies for biotechnology during the period of 2000–2020. Search criteria: the individual “omics” technology was selected. The search was conducted using the Web of Science platform.
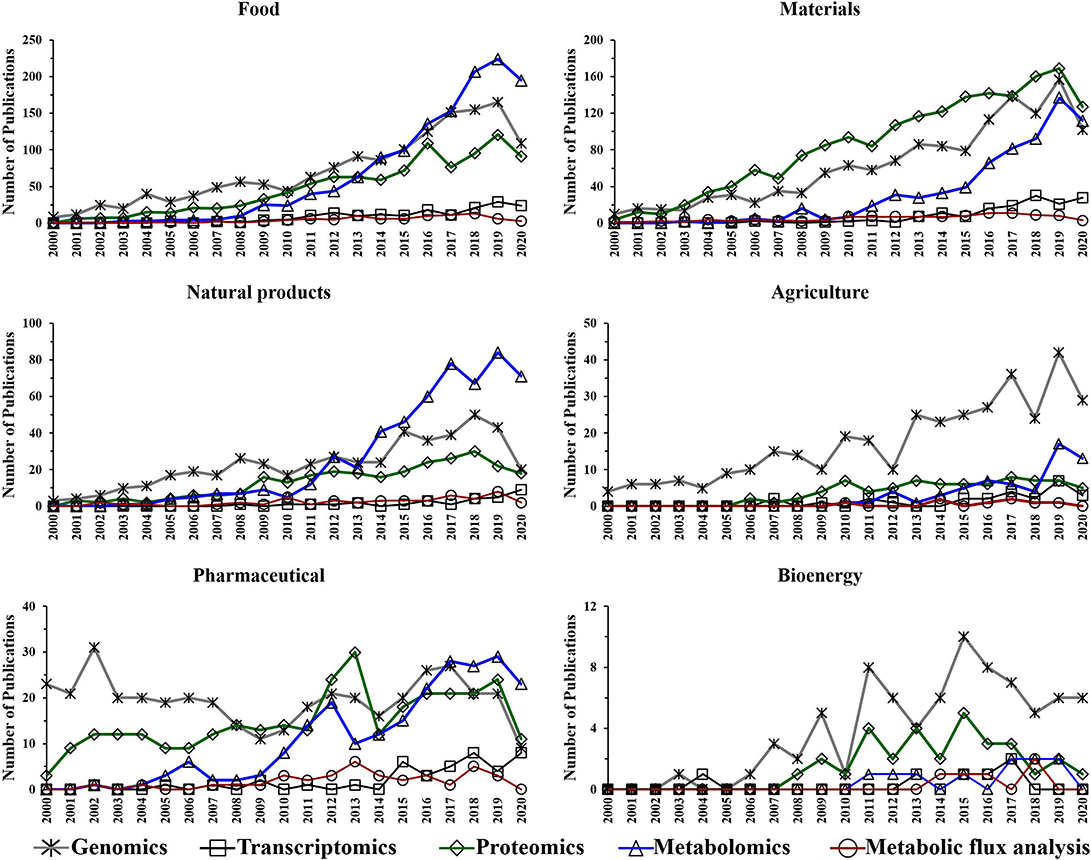
Figure 5. Number of annual publications that utilize “omics” technologies for biotechnology research areas during the period of 2000–2020. Search criteria: the individual “omics” technology and the individual biotechnology research area were selected. The search was conducted using the Web of Science platform.
Omics-based technologies serve as the connective tissue that links biotechnology to these fields of research. For example, the advancement in genomics technologies have improved biotechnology platforms, which have led to developments in pharmaceutical, bioenergy, food, materials, and agriculture research (Oksman-Caldentey and Saito, 2005; Crommelin et al., 2013; Misra et al., 2013; de Pablo et al., 2019; Rexroad et al., 2019). Figure 4 shows a steady growth in the number of genomics publications in biotechnology research, which might be due to the advancement in DNA sequencing, resulting in reduced cost and increased throughput (Pagani et al., 2012). Transcriptomics also appears to show a slight increase in the application of biotechnology-based research during the last decade (Figure 4).
Interestingly, while the utilization of proteomics in biotechnology seems to be significantly increased in the period of 2004–2006, it has generally plateaued during the following years (Figure 4). However, Figure 4 suggests a growing trend in the application of metabolomics studies to biotechnology-based research during the last decade. Additionally, metabolic flux analysis has also shown a slight growing trend in the last decade.
The performed search also suggests an increased utilization of genomics, proteomics and metabolomics during the last two decades in the following research fields: food, materials, and natural products (Figure 5). This is not surprising as there is a growing need for more phenotypic information. Consequently, scientists are using these “omics” techniques to facilitate their research. The improvements in proteomics and metabolomics analytical capabilities may also have contributed to the potential growth in their utilization over the last decade for those fields. Genomics has shown an upward trend in the number of publications for agriculture over the last 20 years (Figure 5).
The utilization of metabolic flux analysis in all research areas has shown a prospective slight growth trend during the last decade (Figure 5). The number of metabolic flux analysis publications, however, is relatively low in the perspective areas for similar reasons to that described for biotechnology.
Conclusions
The DBTL cycle is becoming an increasingly adopted framework in metabolic engineering experiments as it provides a systematic and efficient approach to strain development. However, the DBTL cycle is limited by the Learning process since it requires high quality and large “omics” data sets to increase the accuracy and robustness of Learn methods. The DBTL cycle relies heavily on “omics” technologies during the testing phase of the cycle, and can be integrated into ALE experiments. “Omics” technologies have played major roles in the metabolic engineering of biofuels, bioproducts, and crop development. Proteomics and metabolomics are routinely applied to the analysis of engineered biosynthetic pathways in microbial hosts. Genomics sequencing information appears to be a key component in the development of next generation mRNA and DNA vaccines against virus's such as SARS-CoV-2. Whereas, transcriptomics, proteomics, and metabolomics analyses are being used to guide the development of therapeutic drugs for COVID-19. In the last 20 years, genomics has shown a steady growth in the number of biotechnology publications, however, the emergence of transcriptomics, proteomics, and metabolomics in this field of research is a testament to the development of robust “omics” technologies and methods.
Author Contributions
BA conducted the data curation, data analysis, and literature review. EEKB conducted the data analysis and literature review. All authors contributed to the article and approved the submitted version.
Funding
This work was funded by the DOE Joint BioEnergy Institute (http://www.jbei.org) supported by the U.S. Department of Energy, Office of Science, Office of Biological and Environmental Research, through contract DE-AC02-05CH11231 between Lawrence Berkeley National Laboratory and the U.S. Department of Energy.
Conflict of Interest
The authors declare that the research was conducted in the absence of any commercial or financial relationships that could be construed as a potential conflict of interest.
Footnotes
1. ^UniProt. Available online at: https://www.uniprot.org/ (accessed September 30, 2020).
References
Abid, F., Zahid, M. A., Abedin, Z. U., Nizami, S. B., Abid, M. J., Kazmi, S. Z. H., et al. (2018). Omics Approaches in Marine Biotechnology: The Treasure of Ocean for Human Betterments. London: Elsevier Inc.
Ahmed, Z., Zeeshan, S., Foran, D. J., Kleinman, L. C., Wondisford, F. E., and Dong, X. Q. (2020). Integrative clinical, genomics and metabolomics data analysis for mainstream precision medicine to investigate COVID-19. BMJ Innov. 7, 1–5. doi: 10.1136/bmjinnov-2020-000444
Aichler, M., and Walch, A. (2015). MALDI imaging mass spectrometry: current frontiers and perspectives in pathology research and practice. Lab. Investig. 95, 422–431. doi: 10.1038/labinvest.2014.156
Aizat, W. M., Ismail, I., and Noor, N. M. (2018). “Recent development in omics studies,” in Advances in Experimental Medicine and Biology, eds W. M. Aizat, H-H. Goh and S. N. Baharum (New York, NY: Springer New York LLC), 1–9. doi: 10.1007/978-3-319-98758-3_1
Alfaro, M. P., Sepulveda, J. L., and Lyon, E. (2019). “Molecular testing for targeted therapies and pharmacogenomics,” in Accurate Results in the Clinical Laboratory, eds A. Dasgupta and J. L. Sepulveda (Amsterdam: Elsevier), 349–363. doi: 10.1016/b978-0-12-813776-5.00022-4
Aliferis, K. A., and Chrysayi-tokousbalides, M. (2011). Metabolomics in pesticide research and development : review and future perspectives. Metabolomics 7, 35–53. doi: 10.1007/s11306-010-0231-x
Ando, D., and García Martín, H. (2019). “Genome-scale 13C fluxomics modeling for metabolic engineering of Saccharomyces cerevisiae,” in Methods in Molecular Biology, ed E. E. K. Baidoo (New York, NY: Humana Press Inc.), 317–345. doi: 10.1007/978-1-4939-8757-3_19
Babar, M. M., Afzaal, H., Pothineni, V. R., Zaidi, N. U. S. S., Ali, Z., Zahid, M. A., et al. (2018). “Omics approaches in industrial biotechnology and bioprocess engineering,” in Omics Technologies and Bio-engineering: Volume 2: Towards Improving Quality of Life, eds D. Barh and V. Azevedo (London: Elsevier), 251–269. doi: 10.1016/B978-0-12-815870-8.00014-0
Babele, P. K., and Young, J. D. (2019). Applications of stable isotope-based metabolomics and fluxomics toward synthetic biology of cyanobacteria. Wiley Interdiscip. Rev. Syst. Biol. Med. 13:e1472. doi: 10.1002/wsbm.1472
Baidoo, E. E. K., and Teixeira Benites, V. (2019). Mass spectrometry-based microbial metabolomics: techniques, analysis, and applications. Methods Mol. Biol. 1859, 11–69. doi: 10.1007/978-1-4939-8757-3_2
Bairoch, A., and Boeckmann, B. (1994). The SWISS-PROT protein sequence data bank: current status. Nucleic Acids Res. 22, 3578–3580.
Bashiardes, S., Zilberman-Schapira, G., and Elinav, E. (2016). Use of metatranscriptomics in microbiome research. Bioinform. Biol. Insights 10, 19–25. doi: 10.4137/BBI.S34610
Batrakou, D. G., Müller, C. A., Wilson, R. H. C., and Nieduszynski, C. A. (2020). DNA copy-number measurement of genome replication dynamics by high-throughput sequencing: the sort-seq, sync-seq and MFA-seq family. Nat. Protoc. 15, 1255–1284. doi: 10.1038/s41596-019-0287-7
Benson, D. A., Cavanaugh, M., Clark, K., Karsch-Mizrachi, I., Lipman, D. J., Ostell, J., et al. (2013). GenBank. Nucleic Acids Res. 41, 36–42. doi: 10.1093/nar/gks1195
Bergh, B. Van Den (2018). Experimental design, population dynamics, and diversity in microbial experimental evolution. Microbiol. Mol. Biol. Rev. 82, 1–54. doi: 10.1128/MMBR.00008-18
Bernatchez, J. A., and McCall, L. I. (2020). Insights gained into respiratory infection pathogenesis using lung tissue metabolomics. PLoS Pathog. 16:e1008662. doi: 10.1371/journal.ppat.1008662
Bhatia, S. (2018). “History, scope and development of biotechnology,” in Introduction to Pharmaceutical Biotechnology, eds S. Bhatia and D. Goli (Bristol: IOP Publishing Ltd), 1–61. doi: 10.1088/978-0-7503-1299-8ch1
Bian, Y., Zheng, R., Bayer, F. P., Wong, C., Chang, Y. C., Meng, C., et al. (2020). Robust, reproducible and quantitative analysis of thousands of proteomes by micro-flow LC–MS/MS. Nat. Commun. 11, 1–12. doi: 10.1038/s41467-019-13973-x
Boguszewicz, Bieleń, A., Mrochem-Kwarciak, J., Skorupa, A., Ciszek, M., Heyda, A., et al. (2019). NMR-based metabolomics in real-time monitoring of treatment induced toxicity and cachexia in head and neck cancer: a method for early detection of high risk patients. Metabolomics 15, 1–16. doi: 10.1007/s11306-019-1576-4
Brown, C. G., and Clarke, J. (2016). Nanopore development at Oxford Nanopore Rapid, semi-automated protein terminal characterization using ISDetect. Nat. Publ. Gr. 34, 481–482. doi: 10.1038/nbt.3622
Brunk, E., George, K. W., Alonso-Gutierrez, J., Thompson, M., Baidoo, E., Wang, G., et al. (2016). Characterizing strain variation in engineered E. coli using a multi-omics-based workflow. Cell Syst. 2, 335–346. doi: 10.1016/j.cels.2016.04.004
Bryson, S., Li, Z., Pett-Ridge, J., Hettich, R. L., Mayali, X., Pan, C., et al. (2016). Proteomic stable isotope probing reveals taxonomically distinct patterns in amino acid assimilation by coastal marine bacterioplankton. mSystems 1, 1–16. doi: 10.1128/msystems.00027-15
Carbonell, P., Jervis, A. J., Robinson, C. J., Yan, C., Dunstan, M., Swainston, N., et al. (2018). An automated design-build-test-learn pipeline for enhanced microbial production of fine chemicals. Commun. Biol. 1:66. doi: 10.1038/s42003-018-0076-9
Cardoso, S., Afonso, T., Maraschin, M., and Rocha, M. (2019). WebSpecmine: a website for metabolomics data analysis and mining. Metabolites 9:237. doi: 10.3390/metabo9100237
Catherman, A. D., Skinner, O. S., and Kelleher, N. L. (2014). Top down proteomics: facts and perspectives. Biochem. Biophys. Res. Commun. 445, 683–693. doi: 10.1016/j.bbrc.2014.02.041
Chatham, J. C., and Blackband, S. J. (2001). Nuclear magnetic resonance spectroscopy and imaging in animal research. ILAR J. 42, 189–208. doi: 10.1093/ilar.42.3.189
Chekmeneva, E., Dos Santos Correia, G., Chan, Q., Wijeyesekera, A., Tin, A., Young, J. H., et al. (2017). Optimization and application of direct infusion nanoelectrospray HRMS method for large-scale urinary metabolic phenotyping in molecular epidemiology. J. Proteome Res. 16, 1646–1658. doi: 10.1021/acs.jproteome.6b01003
Chen, B. J., Lam, T. C., Liu, L. Q., and To, C. H. (2017). Post-translational modifications and their applications in eye research. Mol. Med. Rep. 15, 3923–3935. doi: 10.3892/mmr.2017.6529
Chen, G. Q. (2016). Omics meets metabolic pathway engineering. Cell Syst. 2, 362–363. doi: 10.1016/j.cels.2016.05.005
Chen, Y., Guenther, J. M., Gin, J. W., Chan, L. J. G., Costello, Z., Ogorzalek, T. L., et al. (2019). Automated “cells-to-peptides” sample preparation workflow for high-throughput, quantitative proteomic assays of microbes. J. Proteome Res. 18, 3752–3761. doi: 10.1021/acs.jproteome.9b00455
Chistoserdova, L. (2010). Recent progress and new challenges in metagenomics for biotechnology. Biotechnol. Lett. 32, 1351–1359. doi: 10.1007/s10529-010-0306-9
Chong, J., Soufan, O., Li, C., Caraus, I., Li, S., Bourque, G., et al. (2018). MetaboAnalyst 4.0: towards more transparent and integrative metabolomics analysis. Nucleic Acids Res. 46, W486–W494. doi: 10.1093/nar/gky310
Clomburg, J. M., Crumbley, A. M., and Gonzalez, R. (2017). Industrial biomanufacturing: the future of chemical production. Science 355:aag0804. doi: 10.1126/science.aag0804
Craig, R., and Beavis, R. C. (2004). TANDEM: Matching proteins with tandem mass spectra. Bioinformatics 20, 1466–1467. doi: 10.1093/bioinformatics/bth092
Crommelin, D. J. A., Sindelar, R. D., and Meibohm, B. (2013). Pharmaceutical Biotechnology: Fundamentals and Applications, 4th Edn. New York, NY: Springer.
Cupples, A. M. (2005). Principles and applications of soil microbiology, second edition. J. Environ. Qual. 34, 731–732. doi: 10.2134/JEQ2005.0731DUP
Dayon, L., Hainard, A., Licker, V., Turck, N., Kuhn, K., Hochstrasser, D. F., et al. (2008). Relative quantification of proteins in human cerebrospinal fluids by MS/MS using 6-plex isobaric tags. Anal. Chem. 80, 2921–2931. doi: 10.1021/ac702422x
de Pablo, J. J., Jackson, N. E., Webb, M. A., Chen, L. Q., Moore, J. E., Morgan, D., et al. (2019). New frontiers for the materials genome initiative. NPJ Comput. Mater. 5, 1–23. doi: 10.1038/s41524-019-0173-4
Doellinger, J., Schneider, A., Hoeller, M., and Lasch, P. (2020). Sample preparation by easy extraction and digestion (SPEED)–a universal, rapid, and detergent-free protocol for proteomics based on acid extraction. Mol. Cell. Proteomics 19, 209–222. doi: 10.1074/mcp.TIR119.001616
Doran, M. L., Mykytczuk, N., Bieniek, A., Methé, A., and Merritt, T. J. S. (2017). Evaluation of quenching and extraction procedures for performing metabolomics in Acidithiobacillus ferrooxidans. Metabolomics 13, 1–10. doi: 10.1007/s11306-017-1298-4
Dourou, M., Aggeli, D., Papanikolaou, S., and Aggelis, G. (2018). Critical steps in carbon metabolism affecting lipid accumulation and their regulation in oleaginous microorganisms. Appl. Microbiol. Biotechnol. 102, 2509–2523. doi: 10.1007/s00253-018-8813-z
Dragosits, M., and Mattanovich, D. (2013). Adaptive laboratory evolution–principles and applications for biotechnology. Microb. Cell Fact. 12:64. doi: 10.1186/1475-2859-12-64
Dührkop, K., Fleischauer, M., Ludwig, M., Aksenov, A. A., Melnik, A. V., Meusel, M., et al. (2019). SIRIUS 4: a rapid tool for turning tandem mass spectra into metabolite structure information. Nat. Methods 16, 299–302. doi: 10.1038/s41592-019-0344-8
Duong, V. A., Park, J. M., and Lee, H. (2020). Review of three-dimensional liquid chromatography platforms for bottom-up proteomics. Int. J. Mol. Sci. 21:1524. doi: 10.3390/ijms21041524
Durot, M., Bourguignon, P. Y., and Schachter, V. (2009). Genome-scale models of bacterial metabolism: reconstruction and applications. FEMS Microbiol. Rev. 33, 164–190. doi: 10.1111/j.1574-6976.2008.00146.x
Ellis, D. I., and Goodacre, R. (2012). Metabolomics-assisted synthetic biology. Curr. Opin. Biotechnol. 23, 22–28. doi: 10.1016/j.copbio.2011.10.014
Eng, J. K., McCormack, A. L., and Yates, J. R. (1994). An approach to correlate tandem mass spectral data of peptides with amino acid sequences in a protein database. J. Am. Soc. Mass Spectrom. 5, 976–989. doi: 10.1016/1044-0305(94)80016-2
Esvelt, K. M., and Wang, H. H. (2013). Genome-scale engineering for systems and synthetic biology. Mol. Syst. Biol. 9:641. doi: 10.1038/msb.2012.66
Fatima, S., Hameed, A., Noman, M., Ahmed, T., Shahid, M., Tariq, M., et al. (2018). Lignocellulosic biomass : a sustainable bioenergy source for future lignocellulosic biomass : a sustainable bioenergy source for the future. Protein Pept. Lett. 25, 1–16. doi: 10.2174/0929866525666180122144504
Feng, X., Page, L., Rubens, J., Chircus, L., Colletti, P., Pakrasi, H. B., et al. (2010). Bridging the gap between fluxomics and industrial biotechnology. J. Biomed. Biotechnol. 2010:460717. doi: 10.1155/2010/460717
Ferranti, P. (2018). The future of analytical chemistry in foodomics. Curr. Opin. Food Sci. 22, 102–108. doi: 10.1016/j.cofs.2018.02.005
Fiehn, O. (2001). Combining genomics, metabolome analysis, and biochemical modelling to understand metabolic networks. Comp. Funct. Genomics 2, 155–168. doi: 10.1002/cfg.82
Fiehn, O. (2002). Metabolomics - The link between genotypes and phenotypes. Plant Mol. Biol. 48, 155–171. doi: 10.1023/A:1013713905833
Folegatti, P. M., Ewer, K. J., Aley, P. K., Angus, B., Becker, S., Belij-Rammerstorfer, S., et al. (2020). Safety and immunogenicity of the ChAdOx1 nCoV-19 vaccine against SARS-CoV-2: a preliminary report of a phase 1/2, single-blind, randomised controlled trial. Lancet 396, 467–478. doi: 10.1016/S0140-6736(20)31604-4
Frese, K. S., Katus, H. A., and Meder, B. (2013). Next-generation sequencing: From understanding biology to personalized medicine. Biology (Basel) 2, 378–398. doi: 10.3390/biology2010378
Gardinassi, L. G., Souza, C. O. S., Sales-Campos, H., and Fonseca, S. G. (2020). Immune and metabolic signatures of COVID-19 revealed by transcriptomics data reuse. Front. Immunol. 11:1636. doi: 10.3389/fimmu.2020.01636
Geiselman, G. M., Zhuang, X., Kirby, J., Tran-Gyamfi, M. B., Prahl, J. P., Sundstrom, E. R., et al. (2020). Production of ent-kaurene from lignocellulosic hydrolysate in Rhodosporidium toruloides. Microb. Cell Fact. 19, 1–12. doi: 10.1186/s12934-020-1293-8
Gibson, B., Dahabieh, M., Krogerus, K., Jouhten, P., Magalhães, F., Pereira, R., et al. (2020). Adaptive laboratory evolution of ale and lager yeasts for improved brewing efficiency and beer quality. Annu. Rev. 11, 23–44. doi: 10.1146/annurev-food-032519-051715
Gilbert, D., and Heiner, M. (2015). Advances in Computational Methods in Systems Biology. Amsterdam: Elsevier.
Giraudeau, P. (2020). NMR-based metabolomics and fluxomics: developments and future prospects. Analyst 145, 2457–2472. doi: 10.1039/d0an00142b
Gonzalez, P., and Pierron, F. (2015). “Omics in aquatic ecotoxicology: the ultimate response to biological questions?” in Aquatic Ecotoxicology: Advancing Tools for Dealing With Emerging Risks, eds C. Amiard-Triquet, J.-C. Amiard and C. Mouneyrac (London: Elsevier Inc.), 183–203. doi: 10.1016/B978-0-12-800949-9.00008-5
Gordon, D. E., Jang, G. M., Bouhaddou, M., Xu, J., Obernier, K., White, K. M., et al. (2020). A SARS-CoV-2 protein interaction map reveals targets for drug repurposing. Nature 583, 459–468. doi: 10.1038/s41586-020-2286-9
Gross, T., Mapstone, M., Miramontes, R., Padilla, R., Cheema, A. K., Macciardi, F., et al. (2018). Toward reproducible results from targeted metabolomic studies: perspectives for data pre-processing and a basis for analytic pipeline development. Curr. Top. Med. Chem. 18, 883–895. doi: 10.2174/1568026618666180711144323
Guo, X., Fillmore, T. L., Gao, Y., and Tang, K. (2016). Capillary electrophoresis-nanoelectrospray ionization-selected reaction monitoring mass spectrometry via a true sheathless metal-coated emitter interface for robust and high-sensitivity sample quantification. Anal. Chem. 88, 4418–4425. doi: 10.1021/acs.analchem.5b04912
Guo, Y., Sheng, Q., Li, J., Ye, F., Samuels, D. C., and Shyr, Y. (2013). Large scale comparison of gene expression levels by microarrays and RNAseq using TCGA data. PLoS ONE 8:e71462. doi: 10.1371/journal.pone.0071462
Hagen, A., Poust, S., De Rond, T., Fortman, J. L., Katz, L., Petzold, C. J., et al. (2016). Engineering a polyketide synthase for in vitro production of adipic acid. ACS Synth. Biol. 5, 21–27. doi: 10.1021/acssynbio.5b00153
Hamann, E., Gruber-Vodicka, H., Kleiner, M., Tegetmeyer, H. E., Riedel, D., Littmann, S., et al. (2016). Environmental Breviatea harbour mutualistic Arcobacter epibionts. Nature 534, 254–258. doi: 10.1038/nature18297
Hansen, B. T., Jones, J. A., Mason, D. E., and Liebler, D. C. (2001). Salsa: a pattern recognition algorithm to detect electrophile-adducted peptides by automated evaluation of CID spectra in LC-MS-MS analyses. Anal. Chem. 73, 1676–1683. doi: 10.1021/ac001172h
Hayoun, K., Gouveia, D., Grenga, L., Pible, O., Armengaud, J., and Alpha-Bazin, B. (2019). Evaluation of sample preparation methods for fast proteotyping of microorganisms by Tandem mass spectrometry. Front. Microbiol. 10:1985. doi: 10.3389/fmicb.2019.01985
Hinzke, T., Kouris, A., Hughes, R. A., Strous, M., and Kleiner, M. (2019). More is not always better: evaluation of 1D and 2D-LC-MS/MS methods for metaproteomics. Front. Microbiol. 10:238. doi: 10.3389/fmicb.2019.00238
Horinouchi, T., Maeda, T., and Furusawa, C. (2018). Understanding and engineering alcohol-tolerant bacteria using OMICS technology. World J. Microbiol. Biotechnol. 34, 1–9. doi: 10.1007/s11274-018-2542-4
Iwamoto, N., and Shimada, T. (2018). Recent advances in mass spectrometry-based approaches for proteomics and biologics: great contribution for developing therapeutic antibodies. Pharmacol. Ther. 185, 147–154. doi: 10.1016/j.pharmthera.2017.12.007
Jackson, L. A., Anderson, E. J., Rouphael, N. G., Roberts, P. C., Makhene, M., Coler, R. N., et al. (2020). An mRNA vaccine against SARS-CoV-2—preliminary report. N. Engl. J. Med. 383, 1920–1931. doi: 10.1056/nejmoa2022483
Jakočiunas, T., Klitgaard, A. K., Kontou, E. E., Nielsen, J. B., Thomsen, E., Romero-Suarez, D., et al. (2020). Programmable polyketide biosynthesis platform for production of aromatic compounds in yeast. Synth. Syst. Biotechnol. 5, 11–18. doi: 10.1016/j.synbio.2020.01.004
Jeong, K., Kim, S., and Pevzner, P. A. (2013). UniNovo: a universal tool for de novo peptide sequencing. Bioinformatics 29, 1953–1962. doi: 10.1093/bioinformatics/btt338
Johansen, E. (2018). Use of natural selection and evolution to develop new starter cultures for fermented foods. Annu. Rev. Food Sci. Technol. 9, 411–428. doi: 10.1146/annurev-food-030117-012450
Kambale, R., Rahman, H., and Omics, S. (2019). Fluorescence Activated Cell Sorting Single-Cell Omics Approaches in Plants. London: Elsevier.
Kanehisa, M., Furumichi, M., Tanabe, M., Sato, Y., and Morishima, K. (2017). KEGG: new perspectives on genomes, pathways, diseases and drugs. Nucleic Acids Res. 45, D353–D361. doi: 10.1093/nar/gkw1092
Kanehisa, M., and Subramaniam (2002). The KEGG database. Novartis Found. Symp. 247, 91–103. doi: 10.1002/0470857897.ch8
Kang, A., George, K. W., Wang, G., Baidoo, E., Keasling, J. D., and Lee, T. S. (2016). Isopentenyl diphosphate (IPP)-bypass mevalonate pathways for isopentenol production. Metab. Eng. 34, 25–35. doi: 10.1016/j.ymben.2015.12.002
Kang, A., Mendez-Perez, D., Goh, E. B., Baidoo, E. E. K., Benites, V. T., Beller, H. R., et al. (2019). Optimization of the IPP-bypass mevalonate pathway and fed-batch fermentation for the production of isoprenol in Escherichia coli. Metab. Eng. 56, 85–96. doi: 10.1016/j.ymben.2019.09.003
Karpievitch, Y. V., Polpitiya, A. D., Anderson, G. A., Smith, R. D., and Dabney, A. R. (2010). Liquid chromatography mass spectrometry-based proteomics: biological and technological aspects. Ann. Appl. Stat. 4, 1797–1823. doi: 10.1214/10-AOAS341
Kell, D. B. (2004). Metabolomics and systems biology: making sense of the soup. Curr. Opin. Microbiol. 7, 296–307. doi: 10.1016/j.mib.2004.04.012
Kirpich, A. S., Ibarra, M., Moskalenko, O., Fear, J. M., Gerken, J., Mi, X., et al. (2018). SECIMTools: a suite of metabolomics data analysis tools. BMC Bioinformatics 19:151. doi: 10.1186/s12859-018-2134-1
Kleiner, M. (2019). Metaproteomics: much more than measuring gene expression in microbial communities. mSystems 4, 1–6. doi: 10.1128/msystems.00115-19
Kleiner, M., Dong, X., Hinzke, T., Wippler, J., Thorson, E., Mayer, B., et al. (2018). Metaproteomics method to determine carbon sources and assimilation pathways of species in microbial communities. Proc. Natl. Acad. Sci. U.S.A. 115, E5576–E5584. doi: 10.1073/pnas.1722325115
Kleiner, M., Thorson, E., Sharp, C. E., Dong, X., Liu, D., Li, C., et al. (2017). Assessing species biomass contributions in microbial communities via metaproteomics. Nat. Commun. 8:1558. doi: 10.1038/s41467-017-01544-x
Kleiner, M., Wentrup, C., Lott, C., Teeling, H., Wetzel, S., Young, J., et al. (2012). Metaproteomics of a gutless marine worm and its symbiotic microbial community reveal unusual pathways for carbon and energy use. Proc. Natl. Acad. Sci. U.S.A. 109, 1173–1182. doi: 10.1073/pnas.1121198109
Koberstein, J. N. (2020). A sort-seq approach to the development of single fluorescent protein biosensors. bioRxiv. doi: 10.1101/2020.08.21.261578
Koh, H., Fermin, D., Choi, K. P., Ewing, R., and Choi, H. (2018). iOmicsPASS: a novel method for integration of multi-omics data over biological networks and discovery of predictive subnetworks. bioRxiv 374520. doi: 10.1101/374520
Kono, N., and Arakawa, K. (2019). Nanopore sequencing: review of potential applications in functional genomics. Dev. Growth Differ. 61, 316–326. doi: 10.1111/dgd.12608
Krisp, C., Yang, H., Van Soest, R., and Molloy, M. P. (2015). Online peptide fractionation using a multiphasic microfluidic liquid chromatography chip improves reproducibility and detection limits for quantitation in discovery and targeted proteomics. Mol. Cell. Proteomics 14, 1708–1719. doi: 10.1074/mcp.M114.046425
Kumar Awasthi, M., Ravindran, B., Sarsaiya, S., Chen, H., Wainaina, S., Singh, E., et al. (2020). Metagenomics for taxonomy profiling: tools and approaches. Bioengineered 11, 356–374. doi: 10.1080/21655979.2020.1736238
Kuo, W. P., Jenssen, T., Butte, A. J., Ohno-machado, L., and Kohane, I. S. (2002). Analysis of matched mRNA measurements from two different microarray technologies. Bioinformatics 18, 405–412. doi: 10.1093/bioinformatics/18.3.405
Langley, R. J., Tipper, J. L., Bruse, S., Baron, R. M., Tsalik, E. L., Huntley, J., et al. (2014). Integrative “Omic” analysis of experimental bacteremia identi fi es a metabolic signature that distinguishes human sepsis from systemic inflammatory response Syndromes. 190, 445–455. doi: 10.1164/rccm.201404-0624OC
Langley, R. J., Tsalik, E. L., Velkinburgh, J. C. Van, Glickman, S. W., Rice, B. J., Wang, C., et al. (2013). An integrated clinico-metabolomic model improves prediction of death in sepsis. Sci. Transl. Med. 5:195ra95. doi: 10.1126/scitranslmed.3005893
Lee, S., and Kim, P. (2020). Current status and applications of adaptive laboratory evolution in industrial microorganisms. J. Microbiol. Biotechnol. 30, 793–803. doi: 10.4014/jmb.2003.03072
Lewis, N. E., Nagarajan, H., and Palsson, B. O. (2012). Constraining the metabolic genotype-phenotype relationship using a phylogeny of in silico methods. Nat. Rev. Microbiol. 10, 291–305. doi: 10.1038/nrmicro2737
Li, C., Li, K., Li, K., Xie, X., and Lin, F. (2019). SWPepNovo: an efficient de novo peptide sequencing tool for large-scale MS/MS spectra analysis. Int. J. Biol. Sci. 15, 1787–1801. doi: 10.7150/ijbs.32142
Li, Y., Zhang, Z., Liu, X., Li, A., Hou, Z., Wang, Y., et al. (2015). A novel approach to the simultaneous extraction and non-targeted analysis of the small molecules metabolome and lipidome using 96-well solid phase extraction plates with column-switching technology. J. Chromatogr. A 1409, 277–281. doi: 10.1016/j.chroma.2015.07.048
Link, H., Fuhrer, T., Gerosa, L., Zamboni, N., and Sauer, U. (2015). Real-time metabolome profiling of the metabolic switch between starvation and growth. Nat. Methods 12, 1091–1097. doi: 10.1038/nmeth.3584
Liu, H., Wang, Z., Xu, W., Zeng, J., Li, L., Li, S., et al. (2020). Bacillus pumilus LZP02 promotes rice root growth by improving carbohydrate metabolism and phenylpropanoid biosynthesis. Mol. Plant Microbe Interact. 33, 1222–1231. doi: 10.1094/MPMI-04-20-0106-R
Liu, X., Zhou, L., Shi, X., and Xu, G. (2019). New advances in analytical methods for mass spectrometry-based large-scale metabolomics study. Trends Anal. Chem. 121:115665. doi: 10.1016/j.trac.2019.115665
Liu, Y., and Nielsen, J. (2019). Recent trends in metabolic engineering of microbial chemical factories. Curr. Opin. Biotechnol. 60, 188–197. doi: 10.1016/j.copbio.2019.05.010
Long, C. P., and Antoniewicz, M. R. (2018). How adaptive evolution reshapes metabolism to improve fitness: recent advances and future outlook applications of adaptive laboratory evolution (ALE). Curr. Opin. Chem. Eng. 22, 209–215. doi: 10.1016/j.coche.2018.11.001
Luo, X., Reiter, M. A., d'Espaux, L., Wong, J., Denby, C. M., Lechner, A., et al. (2019). Complete biosynthesis of cannabinoids and their unnatural analogues in yeast. Nature 567, 123–126. doi: 10.1038/s41586-019-0978-9
MacLean, B., Tomazela, D. M., Shulman, N., Chambers, M., Finney, G. L., Frewen, B., et al. (2010). Skyline: an open source document editor for creating and analyzing targeted proteomics experiments. Bioinformatics 26, 966–968. doi: 10.1093/bioinformatics/btq054
MacPherson, K. A., Starr, B., Wong, E. D., Dalusag, K. S., Hellerstedt, S. T., Lang, O. W., et al. (2017). Outreach and online training services at the saccharomyces genome database. Database 2017, 1–9. doi: 10.1093/database/bax002
Mahajan, K. R., and Ontaneda, D. (2017). The role of advanced magnetic resonance imaging techniques in multiple sclerosis clinical trials. Neurotherapeutics 14, 905–923. doi: 10.1007/s13311-017-0561-8
Manier, S. K., Keller, A., Schäper, J., and Meyer, M. R. (2019). Untargeted metabolomics by high resolution mass spectrometry coupled to normal and reversed phase liquid chromatography as a tool to study the in vitro biotransformation of new psychoactive substances. Sci. Rep. 9:2741. doi: 10.1038/s41598-019-39235-w
Migaud, M., Gandotra, S., Chand, H. S., Gillespie, M. N., Thannickal, V. J., and Langley, R. J. (2020). Metabolomics to predict antiviral drug efficacy in COVID-19. Am. J. Respir. Cell Mol. Biol. 63, 396–398. doi: 10.1165/rcmb.2020-0206LE
Misra, B. B., Bassey, E., Bishop, A. C., Kusel, D. T., Cox, L. A., and Olivier, M. (2018). High-resolution gas chromatography/mass spectrometry metabolomics of non-human primate serum. Rapid Commun. Mass Spectrom. 32, 1497–1506. doi: 10.1002/rcm.8197
Misra, N., Panda, P. K., and Parida, B. K. (2013). Agrigenomics for microalgal biofuel production: an overview of various bioinformatics resources and recent studies to link omics to bioenergy and bioeconomy. Omics 17, 537–549. doi: 10.1089/omi.2013.0025
Mohanram, S., and Kumar, P. (2019). Rhizosphere microbiome: revisiting the synergy of plant-microbe interactions. Ann. Microbiol. 69, 307–320. doi: 10.1007/s13213-019-01448-9
Mousavi, F., Bojko, B., and Pawliszyn, J. (2015). Development of high throughput 96-blade solid phase microextraction-liquid chromatrography-mass spectrometry protocol for metabolomics. Anal. Chim. Acta 892, 95–104. doi: 10.1016/j.aca.2015.08.016
Mulligan, M. J., Lyke, K. E., Kitchin, N., Absalon, J., Gurtman, A., Lockhart, S., et al. (2020). Phase I/II study of COVID-19 RNA vaccine BNT162b1 in adults. Nature 586, 589–593. doi: 10.1038/s41586-020-2639-4
Nakamura, Y. (2018). Rice starch biotechnology: rice endosperm as a model of cereal endosperms. Starch 70, 1–20. doi: 10.1002/star.201600375
Nesvizhskii, A. I., and Aebersold, R. (2005). Interpretation of shotgun proteomic data: the protein inference problem. Mol. Cell. Proteomics 4, 1419–1440. doi: 10.1074/mcp.R500012-MCP200
Nguyen, T. T. M., An, Y. J., Cha, J. W., Cha, J. W., Ko, Y. J., Lee, H., et al. (2020). Real-time in-organism NMR metabolomics reveals different roles of AMP-activated protein kinase catalytic subunits. Anal. Chem. 92, 7382–7387. doi: 10.1021/acs.analchem.9b05670
Nielsen, J., and Jewett, M. C. (2007). The role of metabolomics in systems biology. Top. Curr. Genet. 18, 1–10. doi: 10.1007/4735_2007_0228
Oksman-Caldentey, K.-M., and Saito, K. (2005). Integrating genomics and metabolomics for engineering plant metabolic pathways. Curr. Opin. Biotechnol. 16, 174–179. doi: 10.1016/j.copbio.2005.02.007
Opgenorth, P., Costello, Z., Okada, T., Goyal, G., Chen, Y., Gin, J., et al. (2019). Lessons from two design–build–test–learn cycles of dodecanol production in Escherichia coli aided by machine learning. ACS Synth. Biol. 8, 1337–1351. doi: 10.1021/acssynbio.9b00020
Oyetunde, T., Bao, F. S., Chen, J. W., Martin, H. G., and Tang, Y. J. (2018). Leveraging knowledge engineering and machine learning for microbial bio-manufacturing. Biotechnol. Adv. 36, 1308–1315. doi: 10.1016/j.biotechadv.2018.04.008
Pagani, I., Liolios, K., Jansson, J., Chen, I. M. A., Smirnova, T., Nosrat, B., et al. (2012). The Genomes OnLine Database (GOLD) v.4: status of genomic and metagenomic projects and their associated metadata. Nucleic Acids Res. 40, 571–579. doi: 10.1093/nar/gkr1100
Pan, M., and Barrangou, R. (2020). Combining omics technologies with CRISPR-based genome editing to study food microbes. Curr. Opin. Biotechnol. 61, 198–208. doi: 10.1016/j.copbio.2019.12.027
Pappireddi, N., Martin, L., and Wühr, M. (2019). A review on quantitative multiplexed proteomics. Chembiochem 20, 1210–1224. doi: 10.1002/cbic.201800650
Perfetto, L., Briganti, L., Calderone, A., Perpetuini, A. C., Iannuccelli, M., Langone, F., et al. (2016). SIGNOR: a database of causal relationships between biological entities. Nucleic Acids Res. 44, D548–D554. doi: 10.1093/nar/gkv1048
Perkins, D. N., Pappin, D. J. C., Creasy, D. M., and Cottrell, J. S. (1999). Probability-based protein identification by searching sequence databases using mass spectrometry data. Electrophoresis 20, 3551–3567. doi: 10.1002/(SICI)1522-2683(19991201)20:18<3551::AID-ELPS3551>3.0.CO;2-2
Phaneuf, P. V, Yurkovich, J. T., Heckmann, D., Wu, M., Sandberg, T. E., King, Z. A., et al. (2020). Causal mutations from adaptive laboratory evolution are outlined by multiple scales of genome annotations and condition-specificity. BMC Genomics. 21:514. doi: 10.1186/s12864-020-06920-4
Pinu, F. R., Beale, D. J., Paten, A. M., Kouremenos, K., Swarup, S., Schirra, H. J., et al. (2019). Systems biology and multi-omics integration: viewpoints from the metabolomics research community. Metabolites 9, 1–31. doi: 10.3390/metabo9040076
Ramirez-Gaona, M., Marcu, A., Pon, A., Guo, A. C., Sajed, T., Wishart, N. A., et al. (2017). YMDB 2.0: a significantly expanded version of the yeast metabolome database. Nucleic Acids Res. 45, D440–D445. doi: 10.1093/nar/gkw1058
Rathahao-Paris, E., Alves, S., Boussaid, N., Picard-Hagen, N., Gayrard, V., Toutain, P. L., et al. (2019). Evaluation and validation of an analytical approach for high-throughput metabolomic fingerprinting using direct introduction–high-resolution mass spectrometry: applicability to classification of urine of scrapie-infected ewes. Eur. J. Mass Spectrom. 25, 251–258. doi: 10.1177/1469066718806450
Ren, J. L., Zhang, A. H., Kong, L., and Wang, X. J. (2018). Advances in mass spectrometry-based metabolomics for investigation of metabolites. RSC Adv. 8, 22335–22350. doi: 10.1039/c8ra01574k
Rexroad, C., Vallet, J., Matukumalli, L. K., Reecy, J., Bickhart, D., Blackburn, H., et al. (2019). Genome to phenome: improving animal health, production, and well-being–a new USDA blueprint for animal genome research 2018–2027. Front. Genet. 10:327. doi: 10.3389/fgene.2019.00327
Roberts, L. D., Souza, A. L., Gerszten, R. E., and Clish, C. B. (2012). Targeted metabolomics. Curr. Protoc. Mol. Biol. 1, 1–24. doi: 10.1002/0471142727.mb3002s98
Robinson, C. J., Carbonell, P., Jervis, A. J., Yan, C., Hollywood, K. A., Dunstan, M. S., et al. (2020). Rapid prototyping of microbial production strains for the biomanufacture of potential materials monomers. Metab. Eng. 60, 168–182. doi: 10.1016/j.ymben.2020.04.008
Robinson, D. G., Chen, W., Storey, J. D., and Gresham, D. (2014). Design and analysis of bar-seq experiments. G3 Genes Genomes Genet. 4, 11–18. doi: 10.1534/g3.113.008565
Roh, K., Nerem, R. M., and Roy, K. (2016). Biomanufacturing of therapeutic cells: state of the art, current challenges, and future perspectives. Annu. Rev. Chem. Biomol. Eng. 7, 455–478. doi: 10.1146/annurev-chembioeng-080615-033559
Rohlhill, J., Sandoval, N. R., and Papoutsakis, E. T. (2017). Sort-seq approach to engineering a formaldehyde-inducible promoter for dynamically regulated Escherichia coli growth on methanol. ACS Synth. Biol. 6, 1584–1595. doi: 10.1021/acssynbio.7b00114
Roukos, D. H. (2012). Biotechnological, genomics and systems-synthetic biology revolution: Redesigning genetic code for a pragmatic systems medicine. Expert Rev. Med. Devices 9, 97–101. doi: 10.1586/erd.11.68
Roumpeka, D. D., Wallace, R. J., Escalettes, F., Fotheringham, I., and Watson, M. (2017). A review of bioinformatics tools for bio-prospecting from metagenomic sequence data. Front. Genet. 8:23. doi: 10.3389/fgene.2017.00023
Rühl, M., Kühn, B., Roos, J., Maier, T. J., Steinhilber, D., and Karas, M. (2019). Elucidation of chemical modifier reactivity towards peptides and proteins and the analysis of specific fragmentation by matrix-assisted laser desorption/ionization collision-induced dissociation tandem mass spectrometry. Rapid Commun. Mass Spectrom. 33, 40–49. doi: 10.1002/rcm.8223
Saito, K., and Matsuda, F. (2010). Metabolomics for functional genomics, systems biology, and biotechnology. Annu. Rev. Plant Biol. 61, 463–489. doi: 10.1146/annurev.arplant.043008.092035
Sajed, T., Marcu, A., Ramirez, M., Pon, A., Guo, A. C., Knox, C., et al. (2016). ECMDB 2.0: A richer resource for understanding the biochemistry of E. coli. Nucleic Acids Res. 44, D495–D501. doi: 10.1093/nar/gkv1060
Sandberg, T. E., Salazar, M. J., Weng, L. L., Palsson, B. O., and Feist, A. M. (2019). The emergence of adaptive laboratory evolution as an efficient tool for biological discovery and industrial biotechnology. Metab. Eng. 56, 1–16. doi: 10.1016/j.ymben.2019.08.004
Savitski, M. M., Nielsen, M. L., and Zubarev, R. A. (2006). ModifiComb, a new proteomic tool for mapping substoichiometric post-translational modifications, finding novel types of modifications, and fingerprinting complex protein mixtures. Mol. Cell. Proteomics 5, 935–948. doi: 10.1074/mcp.T500034-MCP200
Scalbert, A., Brennan, L., Fiehn, O., Hankemeier, T., Kristal, B. S., van Ommen, B., et al. (2009). Mass-spectrometry-based metabolomics: limitations and recommendations for future progress with particular focus on nutrition research. Metabolomics 5, 435–458. doi: 10.1007/s11306-009-0168-0
Schulz, S., Becker, M., Groseclose, M. R., Schadt, S., and Hopf, C. (2019). Advanced MALDI mass spectrometry imaging in pharmaceutical research and drug development. Curr. Opin. Biotechnol. 55, 51–59. doi: 10.1016/j.copbio.2018.08.003
Shen, B., Yi, X., Sun, Y., Bi, X., Du, J., Zhang, C., et al. (2020). Proteomic and metabolomic characterization of COVID-19 patient sera. Cell 182, 59–72.e15. doi: 10.1016/j.cell.2020.05.032
Simirgiotis, M. J., Quispe, C., Mocan, A., Villatoro, J. M., Areche, C., Bórquez, J., et al. (2017). UHPLC high resolution orbitrap metabolomic fingerprinting of the unique species Ophryosporus triangularis meyen from the atacama desert, Northern Chile. Rev. Bras. Farmacogn. 27, 179–187. doi: 10.1016/j.bjp.2016.10.002
Sirén, K., Mak, S. S. T., Fischer, U., Hansen, L. H., and Gilbert, M. T. P. (2019). Multi-omics and potential applications in wine production. Curr. Opin. Biotechnol. 56, 172–178. doi: 10.1016/j.copbio.2018.11.014
Smith, A. M., Heisler, L. E., Mellor, J., Kaper, F., Thompson, M. J., Chee, M., et al. (2009). Quantitative phenotyping via deep barcode sequencing. Genome Res. 19, 1836–1842. doi: 10.1101/gr.093955.109
Smith, A. M., Heisler, L. E., St.Onge, R. P., Farias-Hesson, E., Wallace, I. M., Bodeau, J., et al. (2010). Highly-multiplexed barcode sequencing: an efficient method for parallel analysis of pooled samples. Nucleic Acids Res. 38, 1–7. doi: 10.1093/nar/gkq368
Smith, J. C., and Figeys, D. (2006). Proteomics technology in systems biology. Mol. Biosyst. 2:364. doi: 10.1039/b606798k
Solovchenko, A. E., Ismagulova, T. T., Lukyanov, A. A., Vasilieva, S. G., Konyukhov, I. V., Pogosyan, S. I., et al. (2019). Luxury phosphorus uptake in microalgae. J. Appl. Phycol. 31, 2755–2770. doi: 10.1007/s10811-019-01831-8
Somerville, V., Lutz, S., Schmid, M., Frei, D., Moser, A., Irmler, S., et al. (2018). Long read-based de novo assembly of low complex metagenome samples results in finished genomes and reveals insights into strain diversity and an active phage system. bioRxiv 1–18. doi: 10.1101/476747
Song, J. W., Lam, S. M., Fan, X., Cao, W. J., Wang, S. Y., Tian, H., et al. (2020). Omics-driven systems interrogation of metabolic dysregulation in COVID-19 pathogenesis. Cell Metab. 32, 188–202.e5. doi: 10.1016/j.cmet.2020.06.016
Southam, A. D., Weber, R. J. M., Engel, J., Jones, M. R., and Viant, M. R. (2017). A complete workflow for high-resolution spectral-stitching nanoelectrospray direct-infusion mass-spectrometry-based metabolomics and lipidomics. Nat. Protoc. 12, 255–273. doi: 10.1038/nprot.2016.156
Stark, R., Grzelak, M., and Hadfield, J. (2019). RNA sequencing: the teenage years. Nat. Rev. Genet. 20, 631–656. doi: 10.1038/s41576-019-0150-2
Stettin, D., Poulin, R. X., and Pohnert, G. (2020). Metabolomics benefits from orbitrap GC-MS-comparison of low-and high-resolution GC-MS. Metabolites 10, 1–16. doi: 10.3390/metabo10040143
Subramanian, I., Verma, S., Kumar, S., Jere, A., and Anamika, K. (2020). Multi-omics data integration, interpretation, and its application. Bioinform. Biol. Insights 14, 7–9. doi: 10.1177/1177932219899051
Sun, X., and Weckwerth, W. (2012). COVAIN: a toolbox for uni- and multivariate statistics, time-series and correlation network analysis and inverse estimation of the differential Jacobian from metabolomics covariance data. Metabolomics 8, 81–93. doi: 10.1007/s11306-012-0399-3
Sun, X. M., Ren, L. J., Zhao, Q. Y., Ji, X. J., and Huang, H. (2018). Microalgae for the production of lipid and carotenoids: a review with focus on stress regulation and adaptation. Biotechnol. Biofuels 11:272. doi: 10.1186/s13068-018-1275-9
Szklarczyk, D., Gable, A. L., Lyon, D., Junge, A., Wyder, S., Huerta-Cepas, J., et al. (2019). STRING v11: protein-protein association networks with increased coverage, supporting functional discovery in genome-wide experimental datasets. Nucleic Acids Res. 47, D607–D613. doi: 10.1093/nar/gky1131
Tang, J. (2011). Microbial metabolomics. Curr. Genomics 12, 391–403. doi: 10.2174/138920211797248619
Tilocca, B., Britti, D., Urbani, A., and Roncada, P. (2020). Computational immune proteomics approach to target COVID-19. J. Proteome Res. 19, 4233–4241. doi: 10.1021/acs.jproteome.0c00553
Uranukul, B., Woolston, B. M., Fink, G. R., and Stephanopoulos, G. (2019). Biosynthesis of monoethylene glycol in Saccharomyces cerevisiae utilizing native glycolytic enzymes. Metab. Eng. 51, 20–31. doi: 10.1016/j.ymben.2018.09.012
Urgen Cox, J., and Mann, M. (2011). Quantitative, high-resolution proteomics for data-driven systems biology. Annu. Rev. Biochem. 80, 273–299. doi: 10.1146/annurev-biochem-061308-093216
van Doremalen, N., Lambe, T., Spencer, A., Belij-Rammerstorfer, S., Purushotham, J. N., Port, J. R., et al. (2020). ChAdOx1 nCoV-19 vaccine prevents SARS-CoV-2 pneumonia in rhesus macaques. Nature 586, 578–582. doi: 10.1038/s41586-020-2608-y
Van Emon, J. M. (2016). The omics revolution in agricultural research. J. Agric. Food Chem. 64, 36–44. doi: 10.1021/acs.jafc.5b04515
Van Emon, J. M. (2019). Omics in Fruit Nutrition: Concepts and Application. Amsterdam: Elsevier Inc.
Vavricka, C. J., Hasunuma, T., and Kondo, A. (2020). Dynamic metabolomics for engineering biology: accelerating learning cycles for bioproduction. Trends Biotechnol. 38, 68–82. doi: 10.1016/j.tibtech.2019.07.009
VerBerkmoes, N. C., Denef, V. J., Hettich, R. L., and Banfield, J. F. (2009). Systems biology: functional analysis of natural microbial consortia using community proteomics. Nat. Rev. Microbiol. 7, 196–205. doi: 10.1038/nrmicro2080
Volke, D. C., Rohwer, J., Fischer, R., and Jennewein, S. (2019). Investigation of the methylerythritol 4–phosphate pathway for microbial terpenoid production through metabolic control analysis. Microb. Cell Fact. 18, 1–15. doi: 10.1186/s12934-019-1235-5
Waegele, B., Dunger-Kaltenbach, I., Fobo, G., Montrone, C., Mewes, H. W., and Ruepp, A. (2009). CRONOS: the cross-reference navigation server. Bioinformatics 25, 141–143. doi: 10.1093/bioinformatics/btn590
Walker, C., Ryu, S., and Trinh, C. T. (2019). Exceptional solvent tolerance in Yarrowia lipolytica is enhanced by sterols. Metab. Eng. 54, 83–95. doi: 10.1016/j.ymben.2019.03.003
Wang, C., Cui, Y., and Qu, X. (2018). Mechanisms and improvement of acid resistance in lactic acid bacteria. Arch. Microbiol. 200, 195–201. doi: 10.1007/s00203-017-1446-2
Wang, J. H., Byun, J., and Pennathur, S. (2010). Analytical approaches to metabolomics and applications to systems biology. Semin. Nephrol. 30, 500–511. doi: 10.1016/j.semnephrol.2010.07.007
Wehrs, M., Thompson, M. G., Banerjee, D., Prahl, J. P., Morella, N. M., Barcelos, C. A., et al. (2020). Investigation of Bar-seq as a method to study population dynamics of Saccharomyces cerevisiae deletion library during bioreactor cultivation. Microb. Cell Fact. 19, 1–15. doi: 10.1186/s12934-020-01423-z
Wilmes, P., and Bond, P. L. (2004). The application of two-dimensional polyacrylamide gel electrophoresis and downstream analyses to a mixed community of prokaryotic microorganisms. Environ. Microbiol. 6, 911–920. doi: 10.1111/j.1462-2920.2004.00687.x
Wilmes, P., Heintz-Buschart, A., and Bond, P. L. (2015). A decade of metaproteomics: where we stand and what the future holds. Proteomics 15, 3409–3417. doi: 10.1002/pmic.201500183
Wilson, S. R., Vehus, T., Berg, H. S., and Lundanes, E. (2015). Nano-LC in proteomics: recent advances and approaches. Bioanalysis 7, 1799–1815. doi: 10.4155/bio.15.92
Wolters, D. A., Washburn, M. P., and Yates, J. R. (2001). An automated multidimensional protein identification technology for shotgun proteomics. Anal. Chem. 73, 5683–5690. doi: 10.1021/ac010617e
Wu, T., Liu, J., Li, M., Zhang, G., Liu, L., Li, X., et al. (2020). Improvement of sabinene tolerance of Escherichia coli using adaptive laboratory evolution and omics technologies. Biotechnol. Biofuels 13:79. doi: 10.1186/s13068-020-01715-x
Yan, Q., and Fong, S. S. (2018). Increasing carbon source uptake rates to improve chemical productivity in metabolic engineering. Curr. Opin. Biotechnol. 53, 254–263. doi: 10.1016/j.copbio.2018.06.001
Yang, F., Xiang, W., Li, T., and Long, L. (2018). Transcriptome analysis for phosphorus starvation-induced lipid accumulation in Scenedesmus sp. Sci. Rep. 8:16420. doi: 10.1038/s41598-018-34650-x
Yang, S., Synovec, R. E., Kalyuzhnaya, M. G., and Lidstrom, M. E. (2011). Development of a solid phase extraction protocol coupled with liquid chromatography mass spectrometry to analyze central carbon metabolites in lake sediment microcosms. J. Sep. Sci. 34, 3597–3605. doi: 10.1002/jssc.201100533
Yates, J. R. (2019). Recent technical advances in proteomics. F1000Research 8, 1–8. doi: 10.12688/f1000research.16987.1
Yizhak, K., Benyamini, T., Liebermeister, W., Ruppin, E., and Shlomi, T. (2010). Integrating quantitative proteomics and metabolomics with a genome-scale metabolic network model. Bioinformatics 26, 255–260. doi: 10.1093/bioinformatics/btq183
Zhalnina, K., Louie, K. B., Hao, Z., Mansoori, N., Nunes, U., Shi, S., et al. (2018). substrate preferences drive patterns in rhizosphere microbial community assembly. Nat. Microbiol. 3, 470–480. doi: 10.1038/s41564-018-0129-3
Zhang, Y. H. P., Sun, J., and Ma, Y. (2017). Biomanufacturing: history and perspective. J. Ind. Microbiol. Biotechnol. 44, 773–784. doi: 10.1007/s10295-016-1863-2
Keywords: systems biology, genomics, transcriptomics, metabolomics, proteomics, multi-omics, metabolic engineering, biotechnology
Citation: Amer B and Baidoo EEK (2021) Omics-Driven Biotechnology for Industrial Applications. Front. Bioeng. Biotechnol. 9:613307. doi: 10.3389/fbioe.2021.613307
Received: 01 October 2020; Accepted: 11 January 2021;
Published: 23 February 2021.
Edited by:
Eduard Kerkhoven, Chalmers University of Technology, SwedenReviewed by:
Hyun Uk Kim, Korea Advanced Institute of Science and Technology, South KoreaAdam M. Feist, University of California, San Diego, United States
Copyright © 2021 Amer and Baidoo. This is an open-access article distributed under the terms of the Creative Commons Attribution License (CC BY). The use, distribution or reproduction in other forums is permitted, provided the original author(s) and the copyright owner(s) are credited and that the original publication in this journal is cited, in accordance with accepted academic practice. No use, distribution or reproduction is permitted which does not comply with these terms.
*Correspondence: Edward E. K. Baidoo, eebaidoo@lbl.gov