In-silico molecular modelling, MM/GBSA binding free energy and molecular dynamics simulation study of novel pyrido fused imidazo[4,5-c]quinolines as potential anti-tumor agents
- 1Department of Chemistry, School of Advanced Sciences, Vellore Institute of Technology, Vellore, Tamil Nadu, India
- 2Department of Biotechnology, School of Bio Sciences and Technology, Vellore Institute of Technology, Vellore, Tamil Nadu, India
- 3Division of Chemistry, School of Advanced Sciences, Vellore Institute of Technology, Chennai campus, Chennai, Tamil Nadu, India
With an alarming increase in the number of cancer patients and a variety of tumors, it is high time for intensive investigation on more efficient and potent anti-tumor agents. Though numerous agents have enriched the literature, still there exist challenges, with the availability of different targets and possible cross-reactivity. Herein we have chosen the phosphoinositide 3-kinase (PI3K) as the target of interest and investigated the potential of pyrido fused imidazo[4,5-c]quinoline derivatives to bind strongly to the active site, thereby inhibiting the progression of various types of tumors. The AutoDock, Glide and the Prime-MM/GBSA analysis are used to execute the molecular docking investigation and validation for the designed compounds. The anti-tumor property evaluations were carried out by using PASS algorithm. Based on the GLIDE score, the binding affinity of the designed molecules towards the target PI3K was evaluated. The energetics associated with static interactions revealed 1j as the most potential candidate and the dynamic investigations including RMSD, RMSF, Rg, SASA and hydrogen bonding also supported the same through relative stabilization induced through ligand interactions. Subsequently, the binding free energy of the Wortmannin and 1j complex calculated using MM-PBSA analysis. Further evaluations with PASS prediction algorithm also supported the above results. The studies reveal that there is evidence for considering appropriate pyrido fused imidazo[4,5-c]quinoline compounds as potential anti-tumor agents.
Introduction
Many heterocyclic molecules particularly fused heterocyclic compounds are privileged structures that are being utilized extensively in various drug discovery programs. These molecules exhibit a broad spectrum of pharmacological properties with variations arising from the nature and position of the substituent in the heterocyclic scaffolds. Moreover, fused heterocyclic molecules with multiple rings formed via sharing of two atoms and a bond between the rings, are recognized as promising fluorescent probes materials. Fused heterocycles like indole, quinoline and isoquinoline possess unique properties with variations in their structural motifs and modified electronic environment. Compounds possessing such fused heterocyclic units exhibit a wide spectrum of biological and physical properties (Grzybowski et al., 2014; Sharma et al., 2021; Salehian et al., 2021). Recently, luminescent properties along with the possible applications of amino substituted benzimidazo[1,2-a]quinoline were reported through experimental and computational experiments (Hranjec et al., 2017). Pyrido fused imidazo[4,5-c]quinolones are a class of such fused heterocyclic structures that originated from the fusion of imidazo[1,2-a] pyridines with quinoline frameworks. Imidazo[4,5-c]quinoline is considered as a privileged scaffold with a wide range of pharmacological activity such as PI3K/PKB-pathway inhibitor (Stauffer et al., 2008) (I) TNF-α inhibitor (Izumi et al., 2003) (II). Further, there are many immunomodulator drugs such as Imiquimod (III) and Resiquimod (IV) contains imidazo[4,5-c]quinolone moiety (Figure 1) that are available in the market (Dockrell and Kinghorn, 2001). Recently Siraiva et al have developed a novel fluorescent benzoimidazo[4,5-c]quinoline nucleoside, exhibiting distinct pH dependent photophysical properties (Siraiwa et al., 2016). Recently, Szostak et al reported the importance and synthetic route to quinolone moiety as fused heterocyclic compounds (Zhang et al., 2020). These molecules are reported to serve as bio-probes for nucleic acid structure and genetic analyses (Jones et al., 2015).
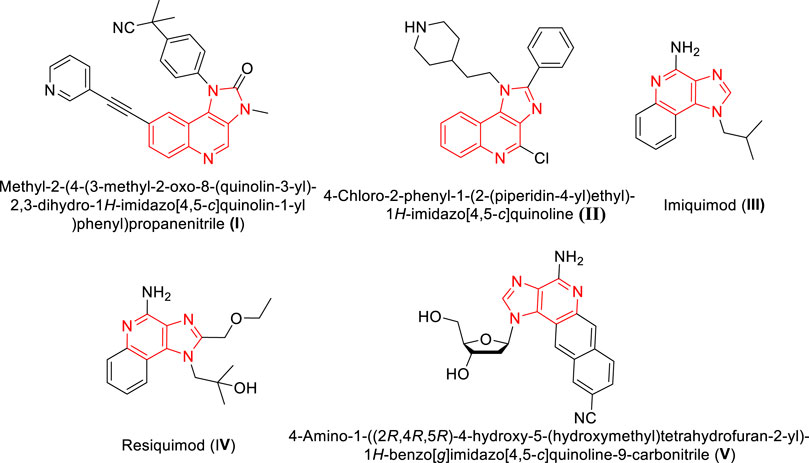
FIGURE 1. Structures of imidazo[4,5-c]quinolone moieties with phamacological and photophysical significance.
Though numerous small-molecular fluorescent probes are available as promising probes in chemical biology, the search for simple fluorogenic scaffolds with the possibility of rational design and predictable photophysical properties for high-throughput screening is still under intense investigation.
All these were proven to be possible with structurally diverse quinoline-based fluorophores and were successfully implemented for live cell imaging applications. (a)
With an alarming increase in the number of cancer patients and variety of tumors, it is high time for intensive investigation on more efficient and potent anti-cancer agents. Though numerous agents have enriched the literature, still there exist challenges, with the availability of different targets and cross-reactivity. Herein we have chosen the phosphoinositide 3-kinase (PI3K) as the target of interest and investigated the potential of pyrido fused imidazo[4,5-c]quinoline derivatives to bind strongly to the active site, thereby inhibiting the progression of various types of tumors. For instance, Phosphoinositide 3-kinases (PI3Ks) are broadly classified into three classes namely, Class I (A and B), II (A and B) and III, amongst these class I PI3Ks were extensively studied for their dysregulation in several cancer types. (b) Specifically, the isoform of PI3K-γ has extended its role in uncontrolled growth and metastases in various cancer types including breast cancer and hematological malignancies (Schmid et al., 2013). Literature evidence has identified the role of PI3K-γ as a molecular switch between immune stimulants and suppressors during inflammation and cancer (Kaneda et al., 2016). Thus, in the present study, a specific isoform of PI3K-γ was targeted to identify potential and selective inhibitors. Furthermore, limiting PI3K-γ expression to the hematopoietic system may reduce the toxicity of specific inhibitors compared to pan-PI3K inhibitors. There are many literature reports indicating the potential of imidazo[4,5-c]quinoline derivatives towards protein kinase inhibition particularly those involved in malignant inducing pathological processes (Alemi et al., 2022; Zaryouh et al., 2022). It is known that alterations in the enzymatic activity of PI3K induce multiple diseases, ranging from cancer to chronic inflammation (Brauer et al., 2012; Shymanets et al., 2015). PI3K signalling plays key roles in cellular responses including proliferation, protein synthesis and vesicular trafficking. It is also reported that imidazo[4,5-c]quinolines reduce the cellular toxicity in host systems and paves way for its selectivity over human kinases (Nguyen et al., 2021; Wright et al., 2021). Additionally, we think that the fusion of two significant bioactive compounds, such as imidazo[1,2-a]pyridines and quinolines, results in an intriguing bioactive property. Recently, we have reported the anti-tumor activities of substituted 2H-indazole derivatives (Panchangam et al., 2019) and novel C,N-cyclometalated 2H-indazole-ruthenium(II) and -iridium(III) complexes targeting triple negative breast cancer cells (Rao et al., 2020; Panchangam et al., 2021).
In continuation of our ongoing drug discovery programme for finding new leads for different diseases through computational approach, (c) we have investigated the potential of pyrido fused imidazo[4,5-c]quinoline derivatives as inhibitor for tumor progression through various in silico approaches including docking, molecular dynamic and pharmacokinetic evaluations.
Methods and methods
Compilation of dataset
The 3 dimensional structure of PI3K protein in complex with wortmannin (PDB ID: 1E7U) was obtained from Brookhaven Protein Data Bank and used for docking studies after certain refinements. All the water molecules and other bound ligands were deleted from the PDB structure followed by the addition of hydrogen atoms to the protein molecule. AutoDockTools-1.5.6 was utilized to assign kollman charges (Chandra Singh and Kollman, 1984) to all atoms of the protein. Conventional procedures were employed to refine protein structures. ChemDraw Ultra 8.0 tool was used to draw the structures of pyrido fused imidazo[4,5-c]quinoline derivatives and the reference (Wortmannin) employed in future processing as shown in Figure 2. Wortmannin is a steroid metabolite present in fungus and is well investigated as a potential inhibitor for PI3K. The PDB 1E7U structure taken from the database is available as bound with Wortmannin. Moreover, the substitutions were chosen based on the electron donating or withdrawing potential at ortho- and para positions of the aromatic ring so as to observe push-pull mechanism along the single rotatable bond that connects the pyrido fused imidazo[4,5-c]quinoline. The geometry of ligands was optimized following DFT calculations with B3LYP functional and 6-311++g** basis set followed by the assignment of Gasteiger partial atomic charges (Frisch et al., 2010). AutoTors was used to define possible flexibility and torsions. The binding pocket was identified using online active site identifier tool and a grid box was constructed. AutoGrid program pre-calculates Grid maps. Using Lamarckian genetic algorithm along with AMBER force field-based energy assessments, various possible conformational states of ligands were explored. Docking calculations were performed with default parameters. The binding energy was evaluated using the following scoring function.
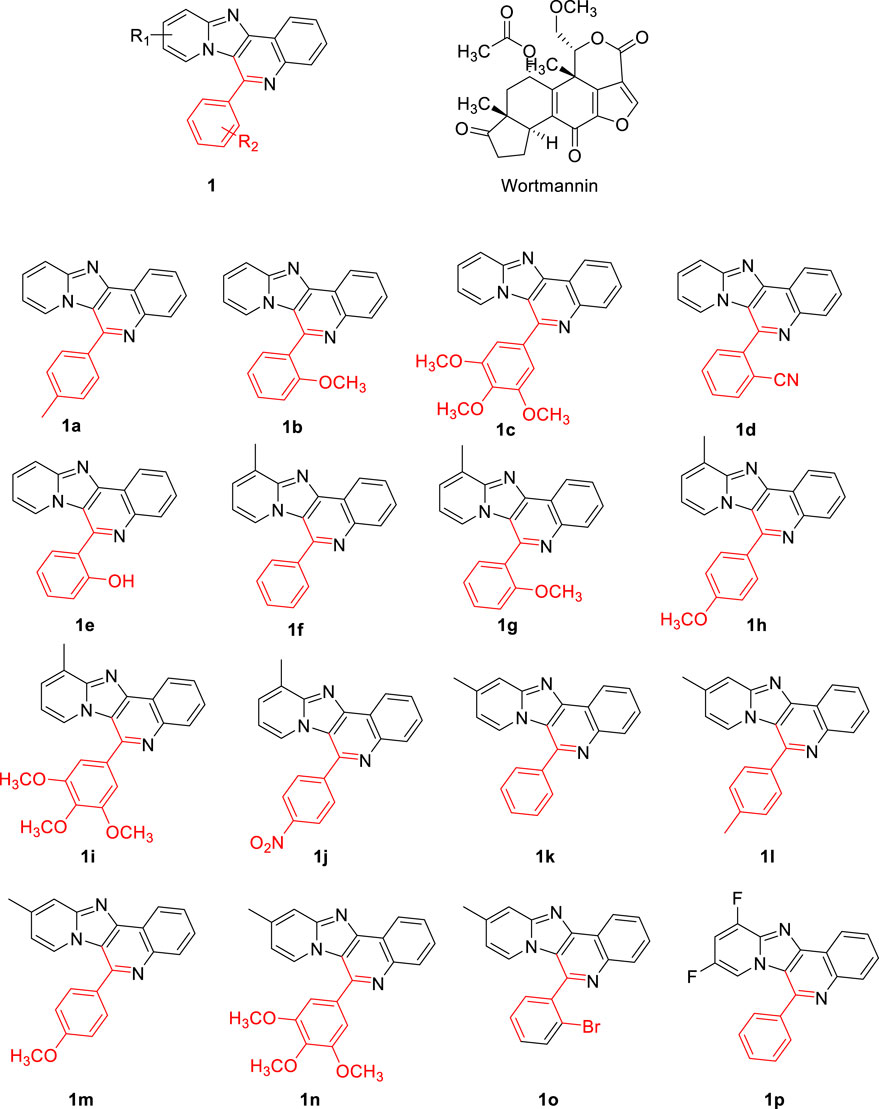
FIGURE 2. The chemical structures of the evaluation of proposed pyrido fused imidazo[4,5-c]quinoline derivatives.
The free energy changes associated with the binding of flexible ligand to rigid target proteins were calculated with the above equation that includes contributing parameters like van der Waals dispersion/repulsion (ΔGvdw), electrostatic (ΔGelec) and hydrogen bonding interaction (ΔGhbond), torsional constraints (ΔGtor) and desolvation effects (ΔGsol).
Drug-likeliness, molecular docking and binding energy calculations
The designed compound’s pharmacodynamic and pharmacokinetic properties have been assessed through using QikProp module (Wang et al., 2015). Lipinski rule of five (Ro5), Central Nervous System activity (CNS), and Human Oral Absorption (HOA) properties have been used to identify drug-like lead compounds (Hodgson, 2001). Moreover, the drug design tool MolAICal was employed to filter out the Pan-Assay Interference Compounds (PAINS) from the proposed candidates (Bai et al., 2021). The designed compounds have been attributed to docking analysis. It is an appropriate approach for investigating the mechanisms of interaction between the ligand and protein of interest. Molecular docking analysis was carried out using Autodock tools 1.5.6 and Glide algorithm of Schrodinger to calculate and retrieve the binding pose of the ligand molecules investigated in our study. In particular, the glide algorithm is of very much useful to discriminate the binders from non-binders. (d)
The free energy of the binding (ΔG bind) for the ligand molecules were then calculated by employing Prime MM-GBSA algorithm using the docked pose retrieved from Glide algorithm. (c) The binding pose of the complex structures were visually inspected by utilizing ligand interaction diagram tool of Schrodinger to gain insight into the binding mode.
The formula corresponds to the (ΔG bind) is given below:
Where, ΔG(solv) is the difference in GBSA solvation energy of the protein-ligand complex and the sum of the solvation energies for unliganded protein and ligand. ΔE(MM) is a difference in the minimized energies between protein-ligand complex and the sum of the energies of the unligated protein and ligand. ΔG(SA) is a difference in surface area energies of the complex and the sum of the surface area energies for the unligated protein and ligand. It also calculates the ligand strain energy by placing ligand in a solution which was auto-generated by VSGB 2.0 solvation model.
The molecular docking is performed on the protein with PDB ID 1E7U. The autogrid was generated centring 23.65, 61.82 and 19.28 (x, y, z coordinate) with grid size of 60, 52, 52 (x, y, z). The docking calculations were carried out by general algorithm parameters with 10 runs of population size 150. The maximum number of evaluations was set to be 25,000 000 (long) with the rate of gene mutation 0.02 and the rate of crossover mode 0.8.
Molecular dynamic simulations
The GROMACS 2018 version was used to analyse the dynamic characteristics of molecules from within the biomolecular system. First, the Prodrg server was used to implement the GROMOS force field and prepare the ligand topology (Sargsyan et al., 2017). The SPC (single point charge) water model included within the dodecahedral box was used to solvated both complexes. Additionally, the steepest decent algorithm was used to carry out energy minimization for each complex. Using the particle mesh ewald (PME) method and the LINCS algorithm respectively, the electrostatic and bond length computations were enforced (Schüttelkopf and Van Aalten, 2004; Wang et al., 2010). The SHAKE algorithm was used to measure the restricted hydrogen bond length (Amiri et al., 2007). Each compound was equilibrated for 100 ps using the NVT and NPT isothermal-isobaric ensemble. Subsequently, a 100 ns MD simulation with a 2 fs integrative step was performed. By utilising the GROMACS toolbox to calculate the development of RMSD and hydrogen bonds, the conformational stability of the protein-ligand complex was investigated in the current study.
Molecular mechanics Poisson–Boltzmann surface area (MM-PBSA) studies
MM-PBSA calculations helps in integrating the high-throughput molecular dynamics (MD) simulations with binding free energies of the protein-ligand interaction, that includes interaction free energies such as van der waals, electrostatic, polar salvation, SASA and binding energies. In this study we have adopted g_mmpbsa package developed by Kumari et al. (Kumari et al., 2014) We executed g_mmpbsa in single step calculation method by using the protein-ligand MD trajectory files along with parameter file. Using MmPbsaStat.py python script provided by g_mmpbsa package van der waals, electrostatic, polar salvation, SASA and binding energies are determined. In addition, we have predicted the contribution energy of each residue by executing MmPbSaDecomp.py of g_mmpbsa package.
Results and discussion
Molecular docking and MM-GBSA calculations
The Glide module of Schrödinger software was employed to estimate the binding affinities of each ligand molecule towards the PI3K target. The binding poses of each small molecule were ranked and scored using XP GScore. The results of molecular docking for 16 compounds were tabulated in Table 1. The binding energy calculations were performed with Molecular Mechanics-Generalized Born Surface Area (MM-GBSA) protocol using VSGB solvation model. MM-GBSA rescoring analysis was carried out to eliminate false positive predictions. Wortmannin was used as control while performing docking calculations. The XP scores of the small molecules varied from −5.68 to −2.56 kcal mol−1. The free binding energy of all the complexes and individual contributions for the total energy values are given in Table 1. From Table 1, it is clear that compound 1j was found to have better XP GScore than other molecules when compared with reference compound (wortmannin: −4.37 kcal mol−1).
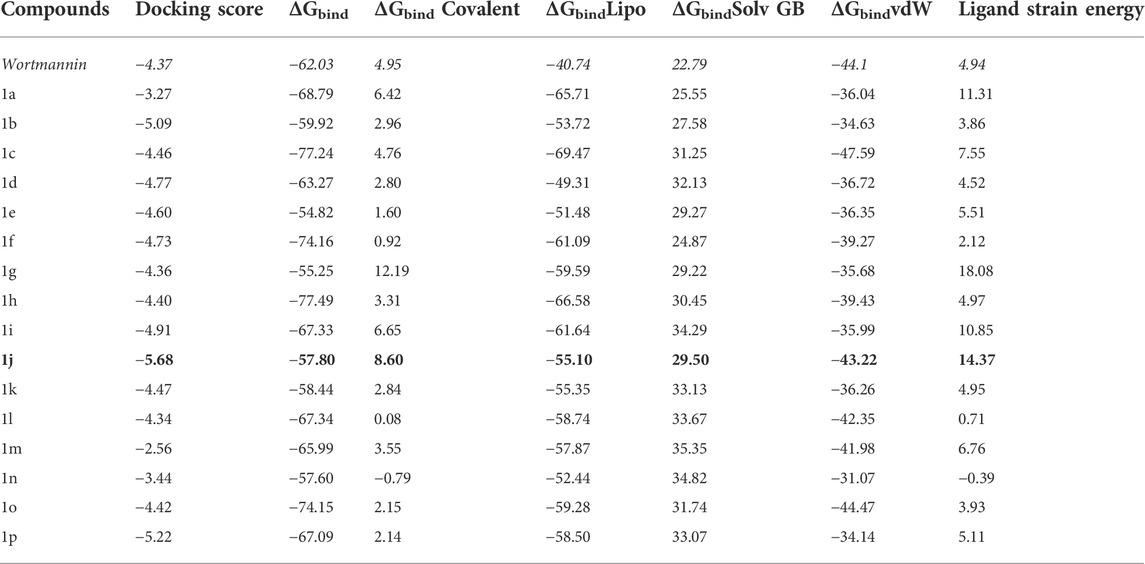
TABLE 1. The free energy (∆G) parameters and the binding score of various pyrido fused imidazo[4,5-c]quinoline derivatives with PI3K as obtained from Glide docking module. All the energies are reported in kcal mol−1.
The interaction pattern of the compound 1j were analysed to gain insights into the binding characteristics of small molecules. The binding pattern of the reference and the compound 1j were highlighted in Figure 3. From Figure 3 it is evident that the reference compound Wortmannin was capable of forming hydrogen bond interaction with the LYS 807 residue. On the other hand, the hit molecule 1j was found to interact with the target protein via two hydrogen bond interactions with residue ASP 950. The increased hydrogen bond formation by the hit molecule indicates its enhanced binding affinity towards the target protein.
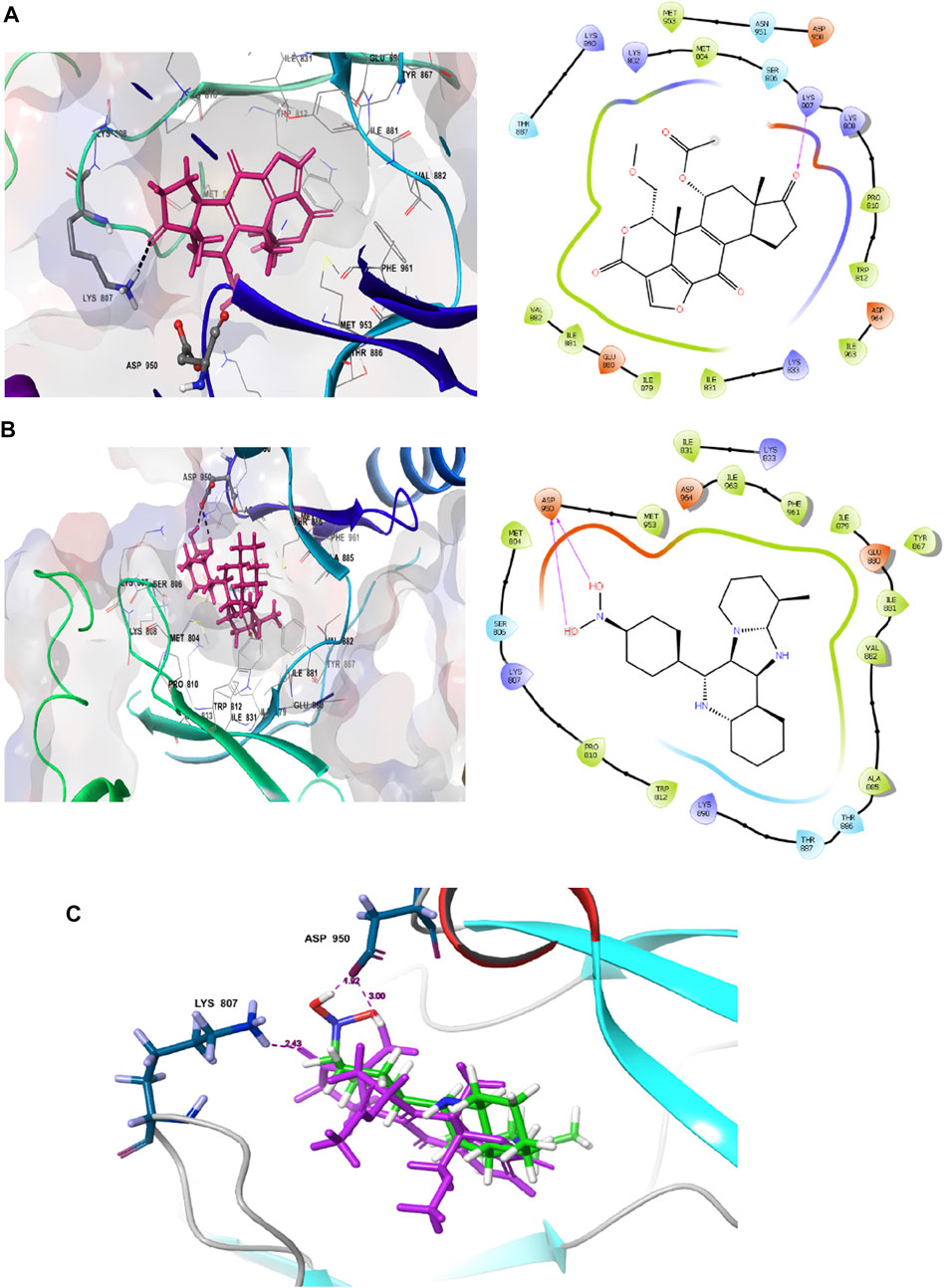
FIGURE 3. Ligand interaction analysis of the (A) Wortmannin, (B) compound 1j and (C) The superimposed view of wortmannin (green) and 1j (violet) with target receptor.
Molecular docking is employed in the advanced drug development process to accurately calculate the binding efficacy of derivatives. Additionally, the Autodock tools 1.5.6 algorithms were employed to calculate the total binding affinity of 16 molecules to the active site of the target P13K. The binding affinity and other features offered by AutoDock algorithm are summarised in Table 2. The binding affinities of all 16 ligands ranged from −6.32 to −7.65 kcal mol−1. In particular, 1j shows good binding affinity to the target site compared to other compounds. Docked conformation of other pyrido fused imidazo[4,5-c]quinolines inhibitors with PI3K along with geometry optimized coordinates are shown in Supplementary Figure S1 (supporting information). The interaction between the hit compound and the aminoacid residues in the active site was also identified and demonstrated using the BIOVIA discovery studio visualizer as shown in Figure 4.
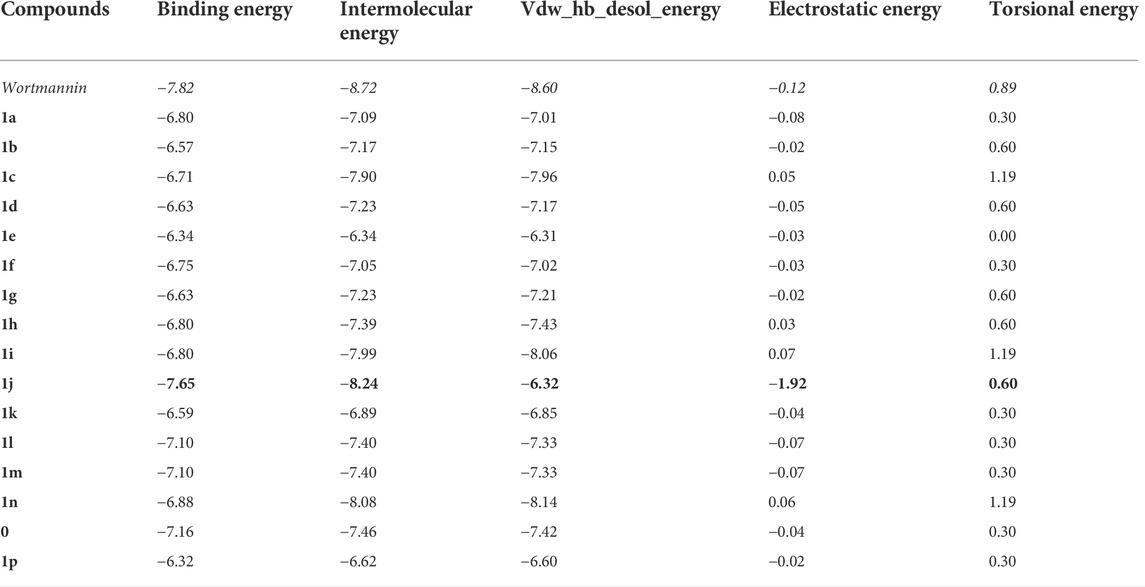
TABLE 2. The binding energy (∆GBE) and intermolecular energy (∆Gintermol) ofpyrido fused imidazo[4,5-c]quinoline derivativeswith PI3K as obtained from AutoDock Tools are given below. All the energies are reported in kcal mol−1.
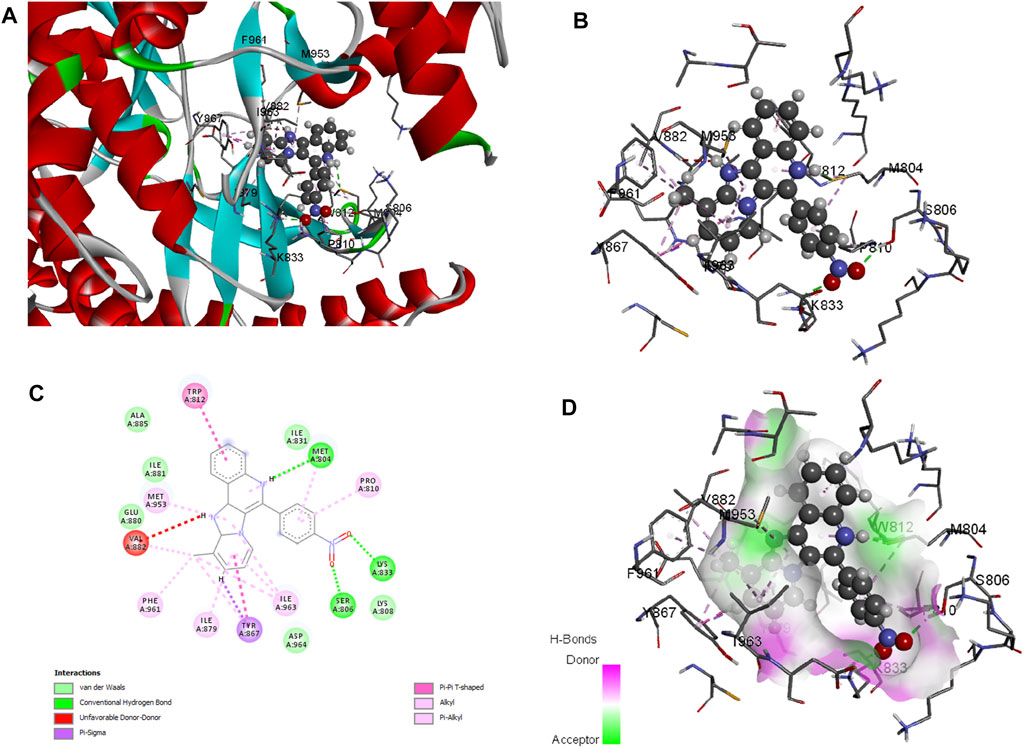
FIGURE 4. Docked conformation of compound 1j with crystal structure of phosphoinositide 3-kinase. (Pdb id: 1E7U) is shown. 1j is represented as ball and stick. (A) 3D Ribbon representation of the binding cavity and its interacting residues with 1j. (B) 3D stick representation of the binding cavity and its interacting residues with 1j. (C) 2D representation of the binding cavity residues along with the nature of interactions. (D) Binding cavity highlighting hydrogen bonding interactions with the residues with 1j.
Based on the above evaluations, compound 1j was found to exhibit lowest free energy values upon interacting with PI3K protein, and the resulting complex was stabilized by van der Waals energy contribution though the ligand strain energy contribution was observed to be high. Thus, our pipelines comprised of multiple docking and MM-GBSA analysis will provide clear view of anti-cancer activity of compound 1j through in silico study. Figures 3A,B represents the 2D docking of Wortmannin and 1j with PI3K protein respectively using Glide module of Schrodinger suite. The interaction pattern also provides the insight into the binding pattern of the molecule. It depicts that compound 1j is able to exhibit more intermolecular hydrogen bonding interaction than Wortmannin in the binding pocket of PI3K protein. Thus, we hypothesize that the increased interaction of ligand molecules with PI3K facilitates the effective binding of the designed molecule. Ligand interaction analysis of all compounds with target receptor are shown in Supplementary Figure S2 (supporting information).
Molecular dynamic simulation
Detailed information on the drug-receptor interactions including binding affinity and orientation of the potent therapeutic molecules to the binding pocket were obtained from molecular docking investigations. Based on the binding affinity score, 1j identified as the most potent inhibitor for PI3K protein towards tumor management. To investigate the binding interactions of any biological molecules in a biological process it is essential to understand the associated mechanisms which in turn can be achieved by molecular dynamic simulation (MDS) method. In the present study, the contacts made between the ligands 1j and Wortmannin with protein PDB ID:1E7U were analyzed by static and dynamic studies. Molecular dynamics (MD) simulation was performed using GROMACS 2018 package for native protein 1E7U, 1j–1E7U complex, and Wortmannin-1E7U complex. To minimize the steric factors, energy minimization was carried out with the geometry of the complex 1j-1E7U through 50000 steps. The calculated average energy was found to be −498.24 × 103 kcal mol−1 while that for the complex Wortmannin-1E7U was calculated to be −472.48 × 103 kcal mol−1. This was followed by NVT and NPT to equilibrate the system. After a successful setup of the parameters, MD simulation was carried out for a 100 ns timescale period. In the present study we have determined Root mean square deviation (RMSD), Root mean square fluctuations (RMSF), Radius of gyration (Rg), Solvent accessible surface areas (SASA), and Hydrogen bonds (Hb) and further the results were analysed to dissect the actual mechanisms involved in the above interactions. These results are depicted by plotting graphs for respective analysis using Qtgrace.
RMSD
The Root mean square deviation (RMSD) is used to study the conformational stability of biological molecules (Zaki et al., 2021). The structural changes in 1E7U upon interacting with Wortmannin and 1j ligands were determined by invoking RMSD analysis and the same is depicted in Figure 5. The overall stability and structural convergence of the two complexes Wortmannin-1E7U and 1j-1E7U alongside native system were analyzed for ∼100 ns Initially, the Wortmannin–1E7U complex showed deviation till 0.60 nm and maintained its stability with minimal fluctuations till ∼68 ns and thereafter sudden instability was observed till ∼80 ns with maximum peak difference ∼1.12 nm (1.62 – 0.5 nm) and later became stable till 100 ns with minimal deviations. The overall average RMSD is calculated to be 0.6769 nm (Wortmannin–1E7U). Whereas complex 1j–1E7U maintained stability throughout the simulation with a lower average of 0.2867 nm RMSD. Similar pattern of deviation was observed even in the case of native protein.
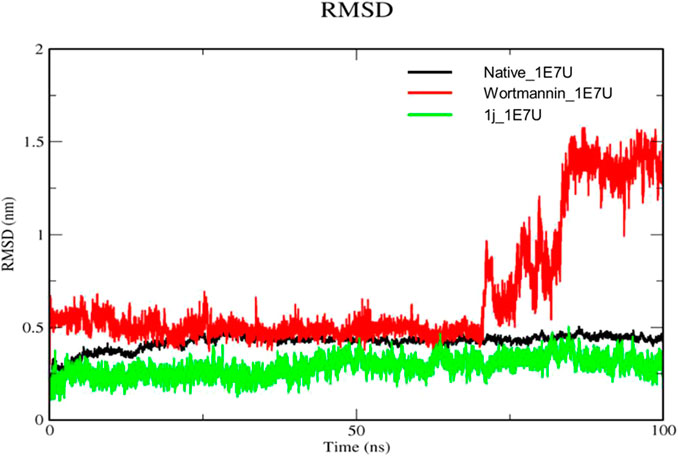
FIGURE 5. RMSD analysis for the native structure of 1E7U (black) and its complex with Wortmannin (red) and 1j (green).
RMSF
The root mean square fluctuation (RMSF) analysis represents the flexibility of each residue in the protein. Here we have examined the positional fluctuations of each protein residue in the native and its complexes with respective ligands (Baildya et al., 2021). Native protein 1E7U showed average fluctuations 0.173 nm whereas complex Wortmannin–1E7U was calculated to be 0.183 nm and complex 1j–1E7U evaluated as 0.185 nm. It can be observed from Figure 6 that residues in the binding site ASN951, ASP864, THR887, LYS890, THR886, TRP812, GLU880, ILE 881, ASN 951 and ILE963 display lower fluctuations.
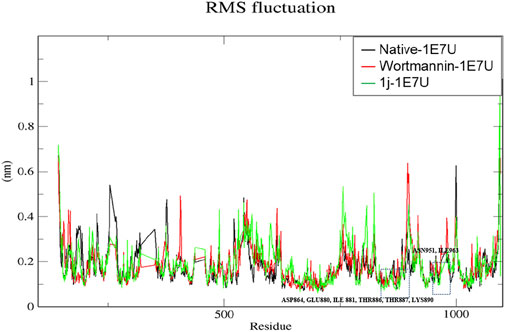
FIGURE 6. RMSF analysis for the native structure of 1E7U and its complex with wortmannin (red) and 1j (green).
Radius of gyration (Rg)
The Radius of gyration (Rg) is a measurement of the structural compactness of protein. The distance between the axis of the structure and the point of the atom during the rotation where maximum energy is transferred gives Rg (Khare et al., 2021). The ligand induced conformational changes alter the Rg upon binding to the target. In the present study, we have measured the Rg of native protein and the protein complexes Wortmannin–1E7U and 1j–1E7U to understand the compactness of the protein upon ligand interactions and the same is depicted in Figure 7. The Rg for native protein was calculated and the average Rg was found to be 2.8322 nm, whereas for the complexes Wortmannin–1E7U and 1j–1E7U were calculated to be 2.8563 nm, and 2.8208 nm respectively. There was a significant decrease in the Rg from 2.95 to 2.77 nm till ∼38 ns and maintained lower than native and Wortmannin complex with a difference of 0.0241 nm indicating that the compactness marginally increased upon interacting with 1j.
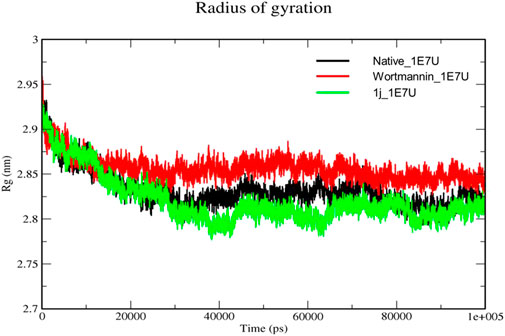
FIGURE 7. Radius of gyration analysis for the native structure of 1E7U (black) and its complexes with Wortmannin (red) and 1j (green).
Solvent-accessible surface area (SASA)
The solvent-accessible surface area (SASA) is a method used to calculate polar and non-polar molecular surface area to understand the residues interacting with the surrounding solvent (Anuar et al., 2021). From Figure 8, the SASA for the native 1E7U, Wortmannin–1E7U complex, and 1j-1E7U complex where native protein average SASA was calculated to be 350.49, 352.038 and 347.148 nm2 respectively. It is observed that the 1j-1E7U complex possess lower SASA in comparison to the native and Wortmannin complex, thus signifying the conformational changes induced by 1j.
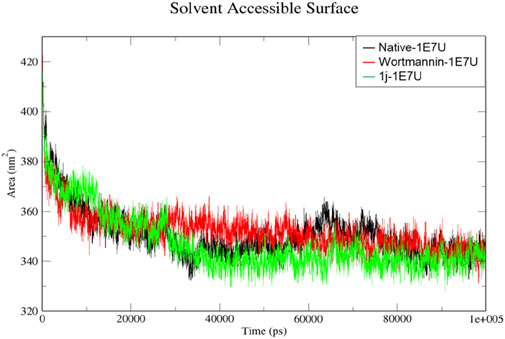
FIGURE 8. SASA analysis for the native structure of 1E7U (black) and its complex with Wortmannin (red) and 1j (green).
Hydrogen bond
Hydrogen bonding is a geometrical analysis that is performed to understand the interactions of bio-molecules. Bio-molecules maintain their structural stability through various interactions and one of the crucial interactions is hydrogen bonding (Vora et al., 2020). In this study, we have examined the interactions of the native 1E7U with ligands Wortmannin and 1j during 100 ns MD simulation and the same is depicted in Figure 9. The Wortmannin complex showed three hydrogen bonds out of which two are continuous throughout the simulation and the 1j complex was observed to have seven hydrogen bonds out of which four were seen throughout the simulation and one hydrogen bond was formed after 60 ns and maintained contact till 100 ns The overall analysis depicts that 1j is making more number of hydrogen contacts with 1E7U to maintain stability.
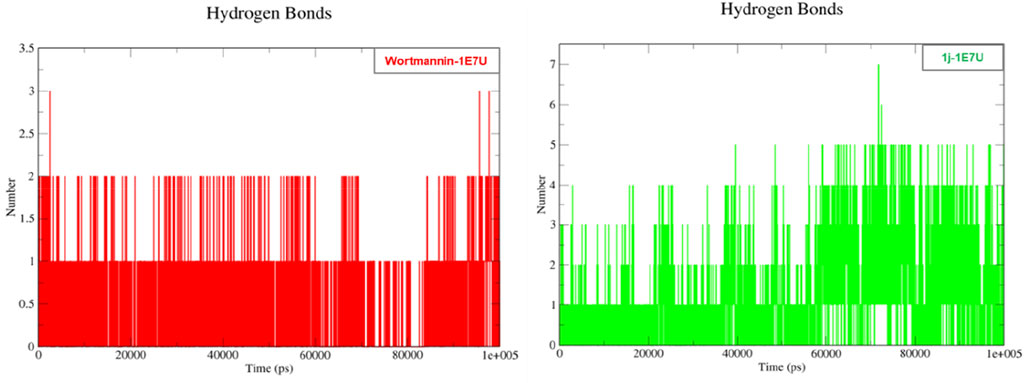
FIGURE 9. Hydrogen bond analysis throughout the 100 ns - 1E7U with Wortmannin (red), 1E7U with 1j (green).
The protein undergoes structural changes at different degrees to perform various cellular functions. Even small conformation changes can impact the functionality of the protein-ligand (Kurkcuoglu and Doruker, 2016). The protein 1E7U has five domains represented in Figure 10. Conformational changes brought up by ligands Wortmannin and 1J in 1E7U during the 100 ns MD simulation has been depicted in Figure 10. The complex structures were extracted for every 10 ns and superimposed to examine conformational changes during the simulation. It is observed that both the ligands Wortmatnnin (red) and 1J (green) were inducing conformational changes to the native protein 1E7U. In Figure 11, it is observed that all the five domains such as 1) phosphoinositide 3-kinase C2 (PI3K_C2) (sky blue), 2) phosphoionsidtide 3-Kinase (PIK-domain) (bright green), 3) PI3-kinase family (PI3K_rbd), 4) PIK3 catalytic subunit gamma adaptor-binding domain (PIK3CG_ABD domain) (dark blue) and 5) phosphatidylinositol 3 and 4-kinase (PI3_PI4 kinase domain) (red) were showing minimal conformational changes. Figure 12 represents the conformation changes in the 1J-1E7U complex throughout the simulations.
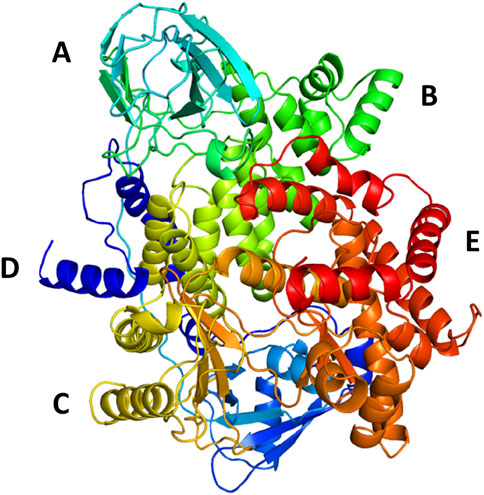
FIGURE 10. 3D - Domain organization of the 1E7U (A)- phosphoinositide 3-kinase C2 (PI3K_C2) (skyblue); (B)-phosphoionsidtide 3-Kinase (PIK-domain)(bright green), (C)-PI3-kinase family (PI3K_rbd)(brass), (D)-PIK3 catalytic subunit gamma adaptor-binding domain (PIK3CG_ABD domain) (dark blue) and (E)-phosphatidylinositol 3 and 4-kinase (PI3_PI4 kinase domain) (red).
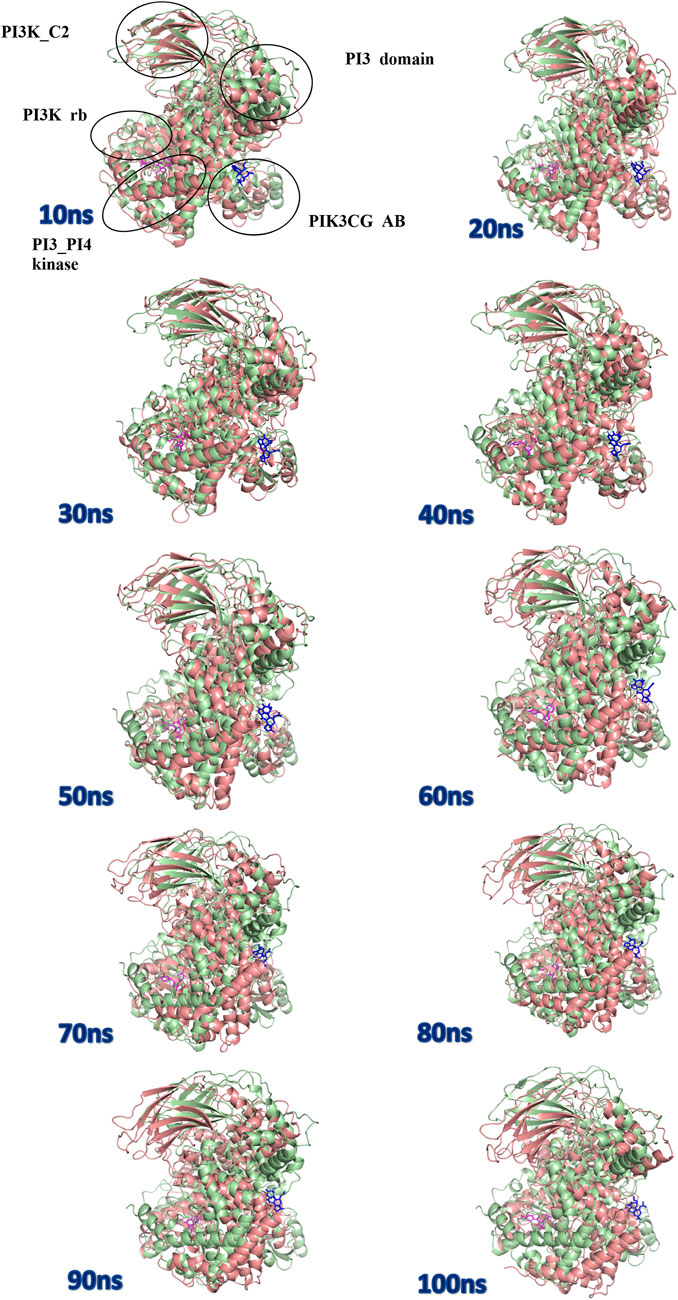
FIGURE 11. Superposition of complex structures of Wortmannin–1E7U and 1j–1E7U after simulation for 10, 20, 30, 40, 50, 60, 70, 80, 90, and 100 ns.
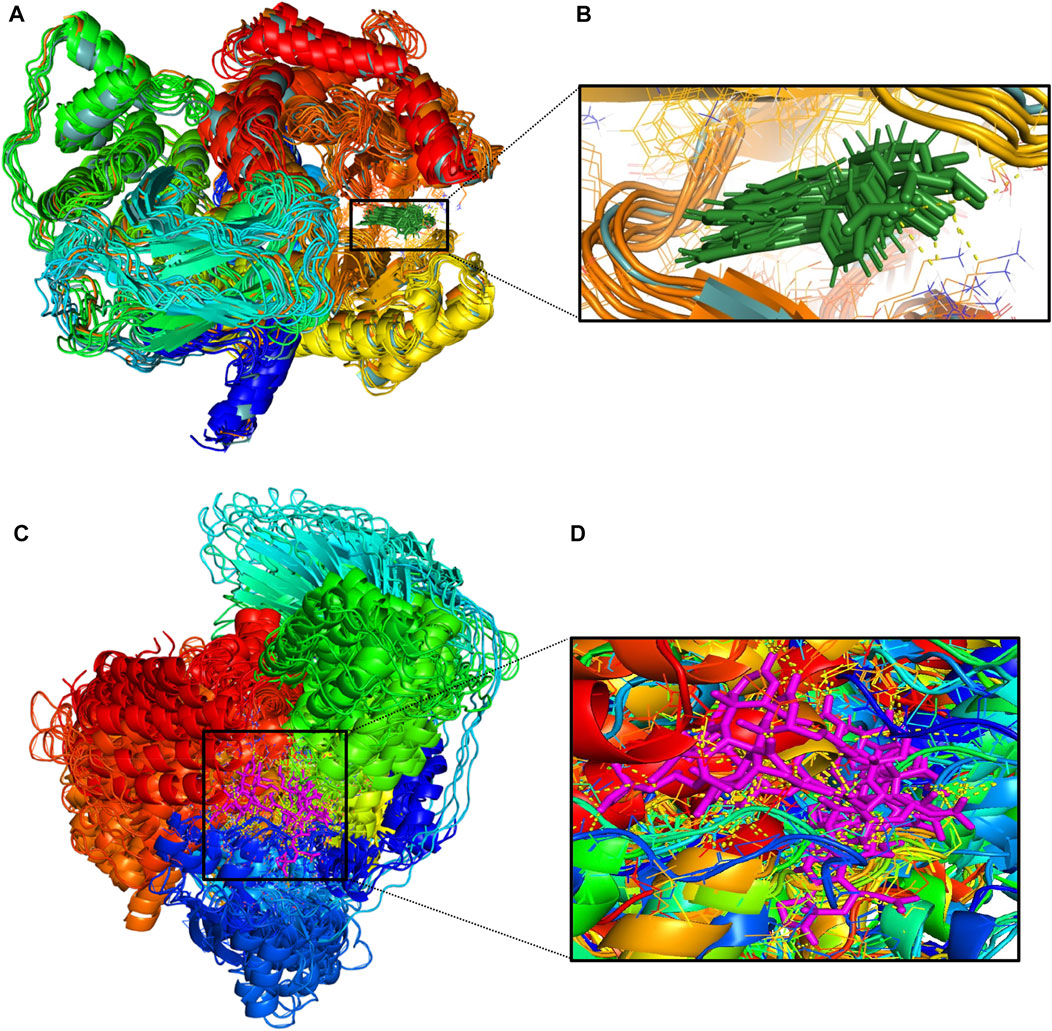
FIGURE 12. (A)-Conformational changes in 1E7U-1J (protein-ligand) complex; (B)-(green color for ligand) (C)-Conformational changes in 1E7U-Wortmannin (protein-ligand) (D)-(pink color for ligand) interacting with with target protien in the active site extracted from molecular dynamics trajectory at different time interims to depict the complex stability. MM-PBSA based Binding free energy Calculations.
To validate the results of our study, we have carried out the MM-PBSA analysis to estimate the binding free energy of the reference and the hit complexes. Of note, shreds of literature evidence highlight that the results of MM-PBSA analysis (ΔGbind) correlate well with the experimental studies. (e) The inclusion of each energy component in the free energy calculations provides insight into the ligand binding mechanism. The results of MM-PBSA calculations for the standard drug (Wortmannin) and screened hit (1j) molecule are tabulated in Table 3. From Table 3, it is clear that the overall binding free energy of the hit molecule was −49.28 kcal mol−1 which is much lower than the reference molecule. Furthermore, the energy terms such as van der Waals energy, electrostatic energy, SASA energy and polar solvation energy are crucial contributors to the overall binding free energy of the complexes. In the present study, it is evident from the table that van der Waals energy and solvation energy was found to be the key driving energies that significantly contributed to the binding free energy of wortmannin and compound 1j.

TABLE 3. The binding free energy of the Wortmannin and 1j complex calculated using MM-PBSA analysis.
ADME screening
In the present investigation, the drug ability of the small molecules were screened using Absorption Distribution Metabolism and Excretion properties incorporated in QikProp module of Schrödinger suite. The important descriptors such as stars, HOA and Lipinski’s rule of five were subjected for considerations. The star value describes the violations of the descriptors by comparing with 95% of known inhibitors. The HOA highlights the human oral absorption, where the compound with HOA >2 was identified as efficient drug molecule. Further, the Lipinski’s rule of five includes molecular weight (<500), hydrogen bond acceptor (HBA≤ 10), donor (HBD ≤5), and octanol/water coefficient (QPlogPo/W < 5). The results from ADME analysis were illustrated in Table 4. Of note, compound 1j was found to satisfy the above-mentioned criteria and therefore identified as hit compound for further analysis.
Anticancer activity-prediction of activity spectra for substance
The anti-cancer activity of the compound was further assessed by employing PASS prediction algorithm and the results are given in Table 5. It is evident from the table that the probability of actives was higher when compared with the probability of inactive ones among all the investigated compounds. Among the studied compounds, the compound such as 1p, and 1j, exhibit higher active scores (greater than 0.5). This indicates that there is a greater possibility of these compounds to have experimental activity (Stepanchikova et al., 2003). Additionally, all these compounds were predicted to have anti-cancerous activity including breast cancer.
Conclusions
Herein we have reported the potential application of a series of synthesized pyrido fused imidazo[4,5-c]quinoline derivatives (Rao and Chanda, 2021) as anti-tumor drug candidates via in silico evaluation. The initial pharmacokinetic and pharmacodynamics evaluations have revealed the drug likeliness of all the proposed candidates. Further investigation on their potential as drug-like candidate was evaluated based on their ability to inhibit the PI3K family of enzymes via in silico evaluation. Based on the scores obtained from docking investigations 1j was identified to exhibit the highest activity through its binding interactions with the active site residues of PI3K enzyme. The energetics associated with static interactions also revealed 1j as the most potential candidate while the dynamic investigations including RMSD, RMSF, Rg, SASA and hydrogen bonding also supported the same through relative stabilization induced through ligand interactions. It can be concluded that the presence of a strong electron-withdrawing nitro group at the para position of the attached phenyl ring of 1j could have played a key role in the observed activity. Molecular dynamic simulations have revealed average stabilization energy of −498.24 × 103 kcal mol−1 upon interaction with PI3K. Moreover, the RMS fluctuations indicate the interacting residues as ASN951, ASP864, THR887, LYS890, THR886, TRP812, GLU880, ILE 881, ASN 951 and ILE963, while the hydrogen bond analysis revealed the presence of seven hydrogen bonds. All the above evaluations revealed the highest potency of 1j as anticancer drug. Further evaluations with PASS prediction algorithm also supported the above results as promising anticancer agents. Therefore, we propose that the possibility of these candidates as potential therapeutic agents can further be tuned by modulating their molecular structures by adding a variety of electron donating or accepting moieties appropriately in the imidazo quinoline backbone to possess enhanced biological activities, which could be made possible through detailed electronic structure evaluations. In vitro and in vivo evaluations may further be needed to support the above claims. The same procedure may further be implemented with other fused heterocyclic to enrich the library of available anti-cancer candidates.
Data availability statement
The original contributions presented in the study are included in the article/Supplementary Material, further inquiries can be directed to the corresponding author.
Author contributions
All authors read and approved the final manuscript.
Acknowledgments
The authors thank the Chancellor and Vice Chancellor of Vellore Institute of Technology for providing opportunity to carry out this study.
Conflict of interest
The authors declare that the research was conducted in the absence of any commercial or financial relationships that could be construed as a potential conflict of interest.
Publisher’s note
All claims expressed in this article are solely those of the authors and do not necessarily represent those of their affiliated organizations, or those of the publisher, the editors and the reviewers. Any product that may be evaluated in this article, or claim that may be made by its manufacturer, is not guaranteed or endorsed by the publisher.
Supplementary material
The Supplementary Material for this article can be found online at: https://www.frontiersin.org/articles/10.3389/fchem.2022.991369/full#supplementary-material
References
Alemi, F., Raei Sadigh, A., Malakoti, F., Elhaei, Y., Ghaffari, S. H., Maleki, M., et al. (2022). Molecular mechanisms involved in DNA repair in human cancers: An overview of PI3k/Akt signaling and PIKKs crosstalk. J. Cell. Physiol. 237, 313–328. doi:10.1002/jcp.30573
Amiri, S., Sansom, M. S., and Biggin, P. C. (2007). Molecular dynamics studies of AChBP with nicotine and carbamylcholine: The role of water in the binding pocket. Protein Eng. Des. Sel. 20, 353–359. doi:10.1093/protein/gzm029
Anuar, N. F. S. K., Wahab, R. A., Huyop, F., Amran, S. I., Hamid, A. A. A., Halim, K. B. A., et al. (2021). Molecular docking and molecular dynamics simulations of a mutant Acinetobacter haemolyticus alkaline-stable lipase against tributyrin. J. Biomol. Struct. Dyn. 39, 2079–2091. doi:10.1080/07391102.2020.1743364
Bai, Q., Tan, S., Xu, T., Liu, H., Huang, J., and Yao, X. (2021). MolAICal: A soft tool for 3D drug design of protein targets by artificial intelligence and classical algorithm. Brief. Bioinform. 22, bbaa161. doi:10.1093/bib/bbaa161
Baildya, N., Khan, A. A., Ghosh, N. N., Dutta, T., and Chattopadhyay, A. P. (2021). Screening of potential drug from Azadirachta Indica (Neem) extracts for SARS-CoV-2: An insight from molecular docking and MD-simulation studies. J. Mol. Struct. 1227, 129390. doi:10.1016/j.molstruc.2020.129390
Brauer, H., Strauss, J., Wegner, W., Muller-Tidow, C., Horstmann, M., and Jucker, M. (2012). Leukemia-associated mutations in SHIP1 inhibit its enzymatic activity, interaction with the GM-CSF receptor and Grb2, and its ability to inactivate PI3K/AKT signaling. Cell. Signal. 24, 2095–2101. doi:10.1016/j.cellsig.2012.07.017
Chandra Singh, U., and Kollman, P. A. (1984). An approach to computing electrostatic charges for molecules. J. Comput. Chem. 5, 129–145. doi:10.1002/jcc.540050204
Czaplińska, B., Malarz, K., Mrozek-Wilczkiewicz, A., Slodek, A., Korzec, M., and Musiol, R. (2020). Theoretical and experimental investigations of large Stokes shift fluorophores based on a quinoline scaffold. Molecules 25, 2488. doi:10.3390/molecules25112488
Dockrell, D. H., and Kinghorn, G. R. (2001). Imiquimod and resiquimod as novel immunomodulators. J. Antimicrob. Chemother. 48, 751–755. doi:10.1093/jac/48.6.751
Frisch, M. J. T., Schlegel, H. B., Scuseria, G. E., Robb, M. A., Cheeseman, J. R., Scalmani, G., et al. (2010). Revision B01. Wallingford: Gaussian Inc.
Fruman, D. A., and Rommel, C. (2014). PI3K and cancer: Lessons, challenges and opportunities. Nat. Rev. Drug Discov. 13, 140–156. doi:10.1038/nrd4204
Grzybowski, M., Hugues, V., Blanchard-Desce, M., and Gryko, D. T. (2014). Two-photon-induced fluorescence in new π-expanded diketopyrrolopyrroles. Chem. Eur. J. 20, 12493–12501. doi:10.1002/chem.201402569
Hodgson, J. (2001). ADMET—Turning chemicals into drugs. Nat. Biotechnol. 19, 722–726. doi:10.1038/90761
Hranjec, M., Horak, E., Babić, D., Plavljanin, S., Srdović, Z., Steinberg, I. M., et al. (2017). Fluorescent benzimidazo[1, 2-a]quinolines: Synthesis, spectroscopic and computational studies of protonation equilibria and metal ion sensitivity. New J. Chem. 41, 358–371. doi:10.1039/c6nj02268e
Izumi, T., Sakaguchi, J., Takeshita, M., Tawara, H., Kato, K., Dose, H., et al. (2003). 1H-Imidazo[4, 5-c]quinoline derivatives as novel potent TNF-α suppressors: Synthesis and structure–activity relationship of 1-2-and 4-substituted 1H-imidazo[4, 5-c]quinolines or 1H-imidazo[4, 5-c]pyridines. Bioorg. Med. Chem. 11, 2541–2550. doi:10.1016/s0968-0896(03)00178-0
James, N., and Ramanathan, K. (2018). Ligand-based pharmacophore screening strategy: A pragmatic approach for targeting HER proteins. Appl. Biochem. Biotechnol. 186, 85–108. doi:10.1007/s12010-018-2724-4
Jones, R. A., Panda, S. S., and Hall, C. D. (2015). Quinine conjugates and quinine analogues as potential antimalarial agents. Eur. J. Med. Chem. 97, 335–355. doi:10.1016/j.ejmech.2015.02.002
Kaneda, M. M., Messer, K. S., Ralainirina, N., Li, H., Leem, C. J., Gorjestani, S., et al. (2016). PI3Kγ is a molecular switch that controls immune suppression. Nature 539, 437–442. doi:10.1038/nature19834
Khare, N., Maheshwari, S. K., and Jha, A. K. (2021). Screening and identification of secondary metabolites in the bark of Bauhinia variegata to treat Alzheimer’s disease by using molecular docking and molecular dynamics simulations. J. Biomol. Struct. Dyn. 39, 5988–5998. doi:10.1080/07391102.2020.1796798
Kumari, R., Kumar, R., and Lynn, A. (2014). g_mmpbsa—a GROMACS tool for high-throughput MM-PBSA calculations. J. Chem. Inf. Model. 54, 1951–1962. doi:10.1021/ci500020m
Kurkcuoglu, Z., and Doruker, P. (2016). Ligand docking to intermediate and close-to-bound conformers generated by an elastic network model based algorithm for highly flexible proteins. PLoS One 11, e0158063. doi:10.1371/journal.pone.0158063
Liu, P., Cheng, H., Roberts, T. M., and Zhao, J. (2009). Targeting the phosphoinositide 3-kinase pathway in cancer. J. Nat. Rev. Drug. Discov. 8, 627–644. doi:10.1038/nrd2926
Nguyen, T., Deenick, E. K., and Tangye, S. G. (2021). Phosphatidylinositol 3-kinase signaling and immune regulation: Insights into disease pathogenesis and clinical implications. Expert Rev. Clin. Immunol. 17, 905–914. doi:10.1080/1744666x.2021.1945443
Panchangam, R. L., Manickam, V., and Chanda, K. (2019). Assembly of fully substituted 2H-indazoles catalyzed by Cu2O rhombic dodecahedra and evaluation of anticancer activity. ChemMedChem 14, 262–272. doi:10.1002/cmdc.201800707
Panchangam, R. L., Rao, R. N., Balamurali, M. M., Hingamire, T. B., Shanmugam, D., Manickam, V., et al. (2021). Antitumor effects of Ir(III)-2H-indazole complexes for triple negative breast cancer. Inorg. Chem. 60, 17593–17607. doi:10.1021/acs.inorgchem.1c02193
Park, S., Kwon, D. I., Lee, J., and Kim, I. (2015). When indolizine meets quinoline: Diversity-oriented synthesis of new polyheterocycles and their optical properties. ACS Comb. Sci. 17, 459–469. doi:10.1021/acscombsci.5b00031
Rajasekhar, S., Das, S., Karuppasamy, R., Balamurali, M. M., and Chanda, K. J. (2022). Identification of novel inhibitors for Prp protein of Mycobacterium tuberculosis by structure based drug design, and molecular dynamics simulations. J. Comput. Chem. 43, 619–630. doi:10.1002/jcc.26823
Rajasekhar, S., Karuppasamy, R., and Chanda, K. (2021). Exploration of potential inhibitors for tuberculosis via structure‐based drug design, molecular docking, and molecular dynamics simulation studies. J. Comput. Chem. 42, 1736–1749. doi:10.1002/jcc.26712
Rao, R. N., and Chanda, K. (2021). An expeditious microwave assisted one-pot sequential route to pyrido fused imidazo[4, 5-c] quinolines in green media. New J. Chem. 45, 3280–3289. doi:10.1039/d0nj05835a
Rao, R. N., Panchangam, R. L., Manickam, V., Balamurali, M. M., and Chanda, K. (2020). Synthesis and antitumor activity evaluation of cyclometalated 2H‐ indazole ruthenium(II) and iridium(III) complexes. ChemPlusChem 85, 1800–1812. doi:10.1002/cplu.202000516
Salehian, F., Nadri, H., Jalili-Baleh, L., Youseftabar-Miri, L., Bukhari, S. N. A., Alireza Foroumadi, A., et al. (2021). A review: Biologically active 3, 4-heterocycle-fused coumarins. Eur. J. Med. Chem. 212, 113034. doi:10.1016/j.ejmech.2020.113034
Sargsyan, K., Grauffel, C., and Lim, C. (2017). How molecular size impacts RMSD applications in molecular dynamics simulations. J. Chem. Theory Comput. 13, 1518–1524. doi:10.1021/acs.jctc.7b00028
Schmid, M. C., Franco, I., Kang, S. W., Hirsch, E., Quilliam, L. A., and Varner, J. A. (2013). PI3-Kinase γ promotes rap1a-mediated activation of myeloid cell integrin α4β1, leading to tumor inflammation and growth. PloSone 8, e60226. doi:10.1371/journal.pone.0060226
Schüttelkopf, A. W., and Van Aalten, D. M. (2004). Prodrg: A tool for high-throughput crystallography of protein–ligand complexes. Acta Crystallogr. D. Biol. Crystallogr. 60, 1355–1363. doi:10.1107/s0907444904011679
Sharma, P., LaRosa, C., Antwi, J., Govindarajan, R., and Werbovetz, K. A. (2021). Imidazoles as potential anticancer agents: An update on recent studies. Molecules 26, 4213. doi:10.3390/molecules26144213
Shymanets, A., Prajwal, L., Vadas, O., Czupalla, C., LoPiccolo, J., Brenowitz, M., et al. (2015). Different inhibition of Gβγ-stimulated class IB phosphoinositide 3-kinase (PI3K) variants by a monoclonal antibody. Specific function of p101 as a Gβγ-dependent regulator of PI3Kγ enzymatic activity. Biochem. J. 469, 59–69. doi:10.1042/bj20150099
Siraiwa, S., Suzuki, A., Katoh, R., and Saito, Y. (2016). Design and synthesis of a novel fluorescent benzo[g]imidazo[4, 5-c]quinoline nucleoside for monitoring base-pair-induced protonation with cytosine: Distinguishing cytosine via changes in the intensity and wavelength of fluorescence. Org. Biomol. Chem. 14, 3934–3942. doi:10.1039/c6ob00494f
Srivastava, H. K., and Sastry, G. N. (2012). Molecular dynamics investigation on a series of HIV protease inhibitors: Assessing the performance of MM-PBSA and MM-GBSA approaches. J. Chem. Inf. Model. 52, 3088–3098. doi:10.1021/ci300385h
Stauffer, F., Maira, S. M., Furet, P., and Echeverria, C. G. (2008). Imidazo[4, 5-c]quinolines as inhibitors of the PI3K/PKB-pathway. Bioorg. Med. Chem. Lett. 18, 1027–1030. doi:10.1016/j.bmcl.2007.12.018
Stepanchikova, A. V., Lagunin, A. A., Filimonov, D. A., and Poroikov, V. V. (2003). Prediction of biological activity spectra for substances: Evaluation on the diverse sets of drug-like structures. Curr. Med. Chem. 10, 225–233. doi:10.2174/0929867033368510
Vora, J., Patel, S., Athar, M., Sinha, S., Chhabria, M. T., Jha, P. C., et al. (2020). Pharmacophore modeling, molecular docking and molecular dynamics simulation for screening and identifying anti-dengue phytocompounds. J. Biomol. Struct. Dyn. 38, 1726–1740. doi:10.1080/07391102.2019.1615002
Wan, S., Knapp, B., Wright, D. W., Deane, C. M., and Coveney, P. V. (2015). Rapid, precise, and reproducible prediction of peptide–MHC binding affinities from molecular dynamics that correlate well with experiment. J. Chem. Theory Comput. 11, 3346–3356. doi:10.1021/acs.jctc.5b00179
Wang, H., Dommert, F., and Holm, C. (2010). Optimizing working parameters of the smooth particle mesh Ewald algorithm in terms of accuracy and efficiency. J. Chem. Phys. 133, 034117. doi:10.1063/1.3446812
Wang, Y., Xing, J., Xu, Y., Zhou, N., Peng, J., Xiong, Z., et al. (2015). In silicoADME/T modelling for rational drug design. Q. Rev. Biophys. 48, 488–515. doi:10.1017/s0033583515000190
Wright, S. C. E., Vasilevski, N., Serra, V., Rodon, J., and Eichhorn, P. J. A. (2021). Mechanisms of resistance to PI3K inhibitors in cancer: Adaptive responses, drug tolerance and cellular plasticity. Cancers (Basel) 13, 1538. doi:10.3390/cancers13071538
Zaki, M. E. A., Al-Hussain, S. A., Masand, V. H., Akasapu, S., Bajaj, S. O., El-Sayed, N. N. E., et al. (2021). Identification of anti-SARS-CoV-2 compounds from food using QSAR-based virtual screening, molecular docking, and molecular dynamics simulation analysis. Pharmaceuticals 14, 357. doi:10.3390/ph14040357
Zaryouh, H., De Pauw, I., Baysal, H., Peeters, M., Vermorken, J. B., Lardon, F., et al. (2022). Recent insights in the PI3K/Akt pathway as a promising therapeutic target in combination with EGFR‐targeting agents to treat head and neck squamous cell carcinoma. Med. Res. Rev. 42, 112–155. doi:10.1002/med.21806
Keywords: PI3K, computational chemistry, cancer, heterocyclic, molecular dynamic (MD)
Citation: Dasmahapatra U, Kumar CK, Das S, Subramanian PT, Murali P, Isaac AE, Ramanathan K, MM B and Chanda K (2022) In-silico molecular modelling, MM/GBSA binding free energy and molecular dynamics simulation study of novel pyrido fused imidazo[4,5-c]quinolines as potential anti-tumor agents. Front. Chem. 10:991369. doi: 10.3389/fchem.2022.991369
Received: 11 July 2022; Accepted: 29 August 2022;
Published: 30 September 2022.
Edited by:
Qifeng Bai, Lanzhou University, ChinaReviewed by:
Xiaohua Zhang, Lawrence Livermore National Laboratory (DOE), United StatesMichal Szostak, Rutgers University, Newark, United States
Copyright © 2022 Dasmahapatra, Kumar, Das, Subramanian, Murali, Isaac, Ramanathan, MM and Chanda. This is an open-access article distributed under the terms of the Creative Commons Attribution License (CC BY). The use, distribution or reproduction in other forums is permitted, provided the original author(s) and the copyright owner(s) are credited and that the original publication in this journal is cited, in accordance with accepted academic practice. No use, distribution or reproduction is permitted which does not comply with these terms.
*Correspondence: Balamurali MM, mmbala@gmail.com; Kaushik Chanda, chandakaushik1@gmail.com
†These authors have contributed equally to this work