- School of Life Science, North China University of Science and Technology, Tangsha, China
Growth-regulating factor (GRF) is a multigene family that plays a vital role in the growth and development of plants. In the past, the GRF family of many plants has been studied. However, there is not a report about identification and evolution of GRF in foxtail millet (Setaria italia). Here, we identified 10 GRF genes in foxtail millet. Seven (70.00%) were regulated by Sit-miR396, and there were 19 optimal codons in GRFs of foxtail millet. Additionally, we found that WGD or segmental duplication have affected GRFs in foxtail millet between 15.07 and 45.97 million years ago. Regarding the GRF gene family of land plants, we identified a total of 157 GRF genes in 15 representative land plants. We found that GRF gene family originated from Group E, and the GRF gene family in monocots was gradually shrinking. Also, more loss resulted from the small number of GRF genes in lower plants. Exploring the evolution of GRF and functional analysis in the foxtail millet help us to understand GRF better and make a further study about the mechanism of GRF. These results provide a basis for the genetic improvement of foxtail millet and indicate an improvement of the yield.
Introduction
Growth-regulating factor (GRF) is a plant-specific transcription factor that plays an important role in plant growth and development. The first member of the identified GRF family is OsGRF1, which plays a regulatory role in gibberellin (GA)-induced stem elongation (van der Knaap et al., 2000). GRF transcription factor has two conserved domains in its N-terminal region: QLQ (Gln, Leu, and Gln) and WRC (Trp, Arg, and Cys) (Rodriguez et al., 2016). The QLQ domain interacts with GRF interacting factor (GIF), and the resulting complex acts as a transcriptional co-activator (Wang et al., 2014). The WRC domain consists of a functional nuclear localization signal and a DNA binding motif (zinc finger structure), which is mainly involved in DNA binding. The C-terminal of some GRF proteins also consists of other domains, including TQL (Thr, Gln, and Leu), GGPL (Gly, Gly, Pro, and Leu), and FFD (Phe, Phe, and Asp) (Cao et al., 2016).
The GRF gene family is a small family; therefore, the functions of each member of the GRF family in the studied species can be studied more comprehensively. Studies have found that GRF genes are often expressed strongly in actively growing and developing tissues, such as germinating seeds, ears, shoots, flower buds, and young leaves (Kim et al., 2003; Choi et al., 2004; Zhang et al., 2008; Wang et al., 2014; Zhang et al., 2017). In addition, studies have shown that most GRFs are regulated by miRNA396. For example, in Arabidopsis, seven miRNA396 target genes were predicted, and six AtGRFs were confirmed in the experiment (Jones-Rhoades and Bartel, 2004; Liu et al., 2009). With the completion of many plant genome sequences, GRF family members of some plants have been studied, such as Arabidopsis (Kim et al., 2003), rice (Choi et al., 2004), maize (Zhang et al., 2008), Brachypodium distachyon (Filiz et al., 2014), Brassica rapa (Wang et al., 2014), Brassica napus (Ma et al., 2017), Solanu lycopersicum (Khatun et al., 2017), Nicotiana tabacum (Zhang et al., 2017), Cucurbitaceae (Baloglu, 2014), Manihot esculenta (Shang et al., 2018), apple (Zheng et al., 2018), mulberry (Rukmangada et al., 2018), and so on. However, studies on GRF genes in foxtail millet and the evolutionary trajectory of GRF genes have not been available.
Foxtail millet is one of the oldest food crops in many regions of the world, especially in China and India, where it is still widely cultivated as a staple food. Although the genome of foxtail millet is small, it has a high inbreeding rate, strong C4 photosynthesis, and high nutritional value, which is usually higher than other grains, containing a large number of minerals, such as essential amino acids, carbohydrates, and vitamins (Li and Brutnell, 2011; Pandey et al., 2013; Jia et al., 2013; Ji et al., 2015; Li et al., 2018). With the sequencing and continuous updating of the foxtail millet genome, now the foxtail millet genome is about 515 Mb (Bennetzen et al., 2012; Zhang et al., 2012; Han et al., 2014; Yang et al., 2020). Together with other gramineous plants, foxtail millet was affected by a whole-genome duplication or tetraploidy approximately 100 million years ago (Wang et al., 2015). This event resulted in thousands of duplicated genes in the existing genome, providing evolutionary power for genetic and functional innovation. Studying GRFs in foxtail millet helps to improve crop genetics and contributes to in-depth study of GRF function and food production. In this study, we conducted a series of informatics analysis on the exploration and functional prediction of GRF using a more comprehensive bioinformatics method to lay the foundation for further study of GRF functions.
Materials and Methods
Acquisition of Members of the GRF Gene Family
We selected 20 plants (5 dicots, 7 monocots, 1 basal angiosperm, 1 Pteridophyta, 1 Bryophyta, and 5 green algae) for GRF evolution analysis, in which the genome-wide of Aegilops tauschii was obtained from the literature (Luo et al., 2017). The remaining 19 species were obtained from the JGI database (http://genome.jgi.doe.gov/) [Arabidopsis thaliana Araport11, Carica papaya ASGPBv0.4, Populus trichocarpa v3.1, Vitis vinifera v2.1, Solanum lycopersicum ITAG3.2, Zea mays Ensembl-18, Sorghum bicolor Rio v2.1, Setaria italica v2.2, Brachypodium distachyon Bd21-3 v1.1, Hordeum vulgare r1, Oryza sativa v7.0, Amborella trichopoda v1.0, Selaginella moellendorfii v1.0, Physcomitrella patens v3.3, Chlamydomonas reinhardtii v5.6, Volvox carteri v2.1, Coccomyxa subellipsoidea C-169 v2.0, Micromonas sp. RCC299 v3.0, and Ostreococcus lucimarinus v2.0]. We downloaded the WRC (PF08879) and QLQ (PF08880) domains from the Pfam database (Bateman et al., 2013). The HMMER (version 3.2.1) software (Mistry et al., 2013) was used to identify GRF candidate members in 20 species. In addition, we also used local BLAST to screen GRF family members of all species again. Finally, we used Pfam (http://pfam.xfam.org), CDD (https://www.ncbi.nlm.nih.gov/cdd), and SMART databases (http://smart.embl-heidelberg.de/) to confirm GRF members that contain WRC and QLQ domains.
Phylogenetic Analysis of GRF Family
Full-length amino acid sequences of GRF in all species were aligned in MAFFT (version 7.037b) (Katoh and Standley, 2013) using auto strategy and were then manually adjusted in BioEdit (Hall, 1999). JTT + I + G + F model was determined to be the best model via ProtTest (version 3.4.2) (Darriba et al., 2011). PhyML 3.1 was used to construct ML trees with the above model and 1000 nonparametric bootstrap replicates (Guindon et al., 2010).
The amino acid sequences of 10 GRFs of foxtail millet were aligned by ClustalW (Thompson et al., 1994). We employed MEGA 7.0 to construct the phylogenetic trees of GRFs in foxtail millet by using the NJ method with the following parameters: Pairwise deletion and 1000 bootstrap replications (Kumar et al., 2016).
Characterization of GRFs in Foxtail Millet
The chromosome distribution of GRFs in foxtail millet was drawn by MapChart software (Voorrips, 2002). The online website MEME (http://meme-suite.org/) was employed to analyze GRF proteins in foxtail millet to identify as the conservative motifs (Bailey et al., 2009). The maximum number of motifs was set to be 5, and the remaining parameters were default. Isoelectric point value and theoretical molecular weight of GRF proteins in foxtail millet were calculated using the ProtParam tool of ExPaSy (https://web.expasy.org/protparam/) (Gasteiger et al., 2005). The subcellular localization of GRFs in foxtail millet was predicted by Plant-mPLoc database (Chou and Shen, 2010). Using the SOPMA website (https://npsa-prabi.ibcp.fr/cgi-bin/npsa_automat.pl?page=npsa_sopma.html) to predict the secondary structure of GRF proteins in foxtail millet, the parameters were default. Using the Phyre2 website (http://www.sbg.bio.ic.ac.uk/∼phyre2/html/page.cgi?id=index) to predict the three-dimensional structure of GRF proteins, the parameters were default. The gene structure of GRFs in foxtail millet was analyzed and drawn using GSDS 2.0 (http://gsds.cbi.pku.edu.cn/) (Hu et al., 2014) and CFVisual (version 2.1) (https://github.com/ChenHuilong1223/CFVisual) (Chen et al., 2021). Multiple sequence alignment of the amino acid sequences was performed by ClustalX (Wilm et al., 2007) and conserved regions were visualized using DNAMAN 8.0. In order to reduce errors, nine coding sequences that meet requirements were screened, based on literature criteria (Eyre-Walker, 1991). Afterward, the codon bias analysis of these sequences was performed via CodonW software (https://sourceforge.net/projects/codonw/).
Selection Pressure, Gene Duplication, and Collinearity Analysis
The amino acid sequences of GRFs in foxtail millet were aligned using MAFFT (version 7.037b), and the amino acid alignments were translated into coding sequence alignments via PAL2NAL (http://www.bork.embl.de/pal2nal/) (Suyama et al., 2006). After that, we employed the codeml program in PAML 4.9 h software (Yang, 1997) to calculate the selection pressure of each branch of the GRF phylogenetic tree. We chose the branch model to achieve this (Yang et al., 1998), which was based on the free ratio model and one ratio model (prob = 1.517e-04).
MCScanX software (Wang et al., 2012) was used to analyze the duplications of the GRF family of foxtail millet, and 34,584 protein sequences from foxtail millet (Bennetzen et al., 2012) were analyzed using all-vs-all BLAST search with e-value < 1e-05 (Camacho et al., 2009). The putative WGDs/segmental duplications of GRF genes located on chromosomes of foxtail millet are connected by red lines. Ks (synonymous substitution rate) and Ka (nonsynonymous substitution rate) values of WGDs/segmental duplications were calculated based on the coding sequence alignments using the method of Nei and Gojobori as implemented in KaKs_calculator 2.0 (Nei and Gojobori, 1986; Wang et al., 2010). The Ks value was translated into duplication time in millions of years based on the rate of
Orthologous pairs of GRF members among foxtail millet, Arabidopsis, and rice were identified using OrthoFinder software (version 2.2.6) (Emms and Kelly, 2015) and OrthoMCL (version 2.0.9) (Li et al., 2003). The results were visualized using Circos (version 0.69–6) (Krzywinski et al., 2009).
Expression and Regulation Analysis of GRFs in Foxtail Millet
We obtained the sequence of foxtail millet miRNA396 from the literature (Yadav et al., 2016), and then used psRNATargetsoftware (http://plantgrn.noble.org/psRNATarget/) to predict the binding site of miR396 in GRF genes of foxtail millet (Dai et al., 2018). PlantCARE (http://bioinformatics.psb.ugent.be/webtools/plantcare/html/) was used to analyze the 1 Kb sequence upstream of GRF genes in foxtail millet (Lescot et al., 2002). We utilized an in-house Python script to extract GRF TPM values of foxtail millet from Illumina RNA-seq data reported previously (Yang et al., 2020). The heatmap was drawn via Morpheus software (https://software.broadinstitute.org/morpheus/) based on the transformed data of log2 (TPM+1) values. The String database (version 11.0) (https://string-db.org/) was used to predict interaction proteins of GRFs with the minimum required interaction score set to be high confidence (0.700) (Szklarczyk et al., 2015). The agriGO V2.0 was used for GO analysis of GRFs in foxtail millet (Tian et al., 2017).
Quantitative Real-Time PCR Analysis
The total RNA was extracted using RNAprep Pure Plant Plus Kit (TIANGEN) from three tissues: imbibed 3-day seed, 2-week-old seedling, and 2-week-old seedling root. First-strand cDNA was synthesized using Fastking RT Kit (with gDNase) (TIANGEN). The SuperReal PreMix Plus (SYBR Green) (TIANGEN) was used for real-time-qPCR analysis with 7900HT Fast Real-Time PCR System (American Applied Biosystems). The Primers were designed by Primer Premier6.0 and synthesized by GENEWIZ Biotechnology Co., Ltd. (Supplementary Table S1). EF-1α was the reference gene (Kumar et al., 2013).
Results
Genome-wide Identification and Classification of GRF Genes in Plants
We identified a total of 157 GRF genes in 20 species (Figure 1, Supplementary Table S2). No GRF gene has been identified in green algae (C.reinhardtii, V. carteri, C. subellipsoidea C-169, M. sp. RCC299, and O. lucimarinus). In land plants, the least GRF genes (two) have been identified in P. patens, four GRF genes have been identified in S. moellendorfii, and six GRF genes have been identified in A. trichopoda. The number of GRFs in monocots (Z.mays, S. bicolor, S. italica, B. distachyon, H. vulgare, and O. sativa) ranges from 10 to 16, while the number of GRFs in dicots (A.thaliana, C. papaya, P. trichocarpa, V. vinifera, and S. lycopersicum) ranges from 7 to 19.
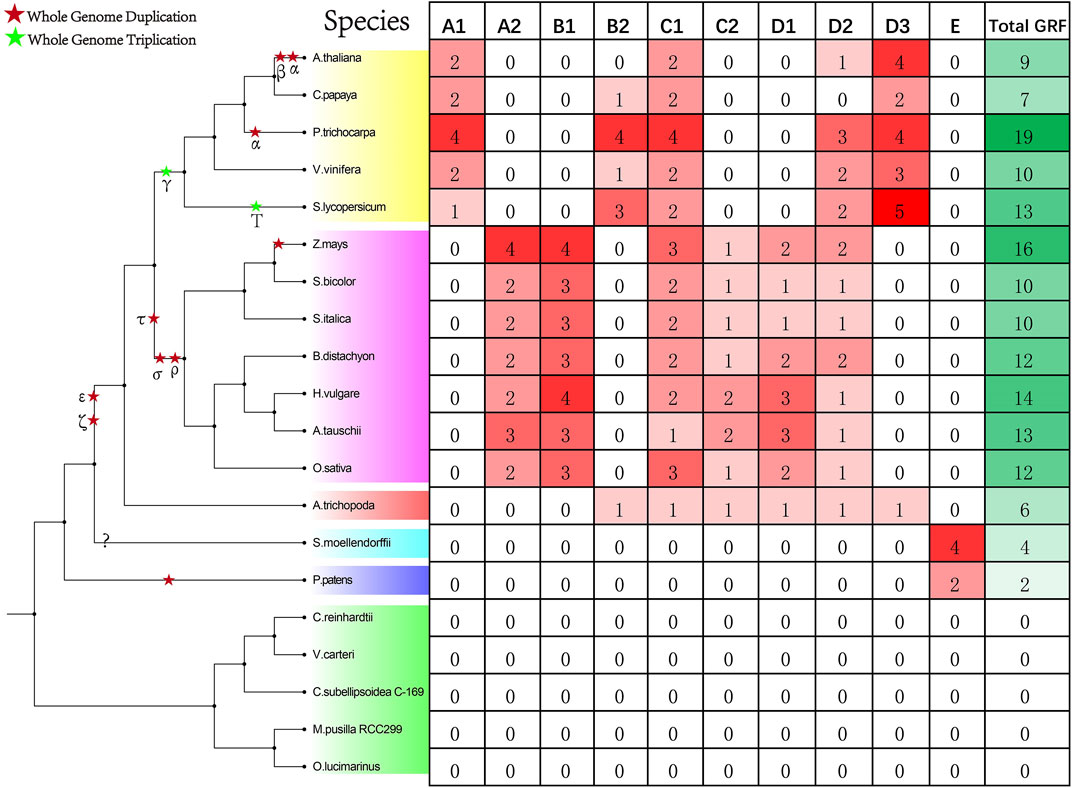
FIGURE 1. The number of GRF families in the collected species. The left of this figure shows the evolutionary relationships of the species; the right of this figure shows the number detail of the GRF family of each species.
According to previous research and the phylogenetic tree topology (Song et al., 2018), phylogenetic analysis showed that 157 GRF genes could be clustered into five categories: A, B, C, D, and E (Figure 2). A class is subdivided into A1 and A2 subclass, and B class is subdivided into B1 and B2 subclass. C class is subdivided into C1 and C2 subclass, and D class is subdivided into D1, D2, and D3 subclass. According to statistics, the genes of the A1 subclass are dicots GRFs. The genes of the A2 subclass and B1 subclass are monocots GRFs. The genes of the B2 subclass and D3 subclass contain some dicots GRFs and one basal angiosperm GRF. The genes of the C2 subclass and D1 subclass contain some monocots GRFs and one basal angiosperm GRF. In addition, the genes of ancient E class are GRFs of all ancient species (P. patens and S. moellendorfii) (Figure 1).
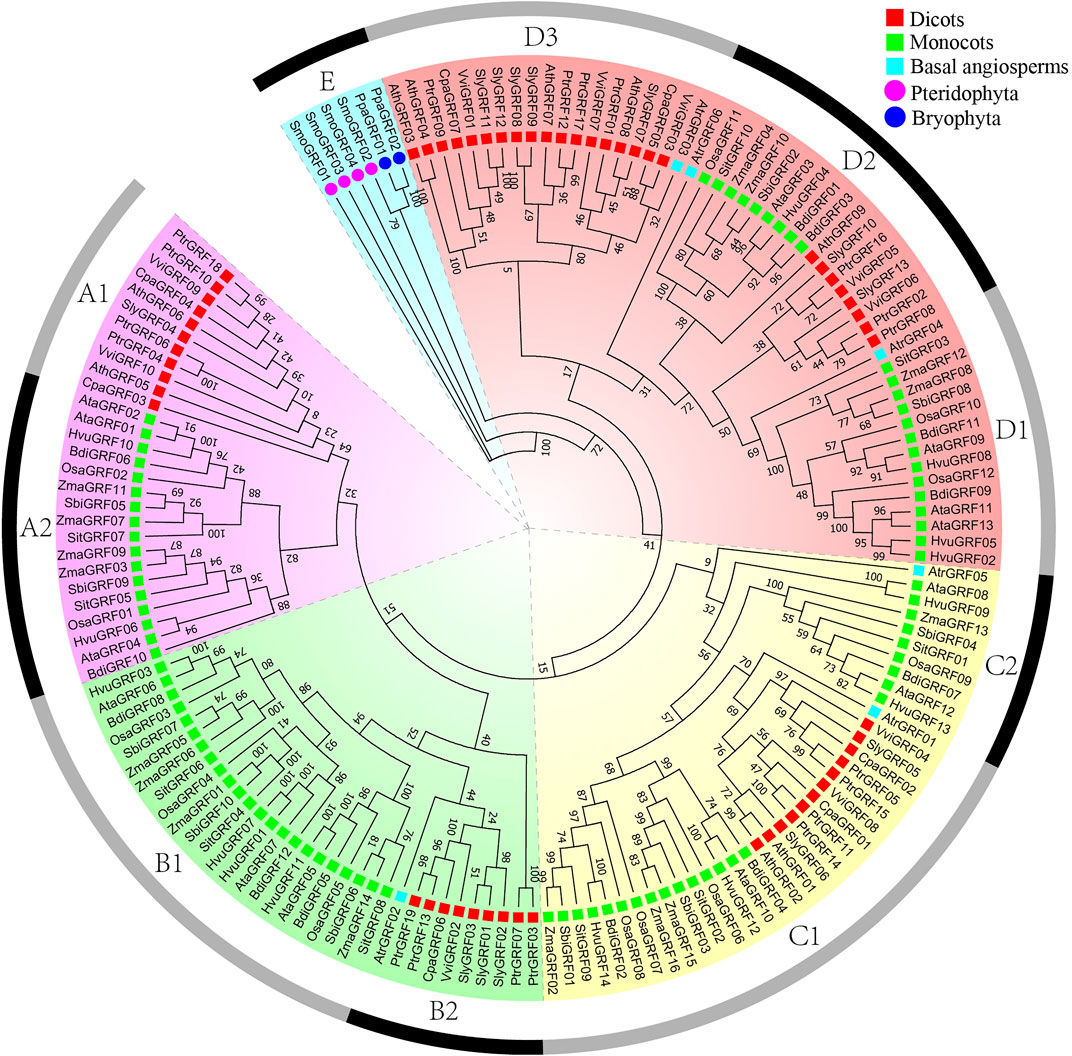
FIGURE 2. Phylogenetic trees of GRF genes. Phylogenetic analysis of plant GRF proteins using PhyML 3.1 under JTT + I + G + F model with 1000 nonparametric bootstrap replicates.
Gain and Loss of GRF Genes in Plants
Based on the comparison between the species tree and the plant GRF gene tree, we used Notung software to analyze the gain and loss of GRF genes. The results show that the ancestors of land plants contained 11 GRF genes (Figure 3). The loss is more serious in lower plants. Among them, 10 GRF genes of P. patens and S. moellendorfii have been lost, and 1 GRF gene and 3 GRF genes have been obtained, respectively. The ancestor of angiosperms contains 15 GRF genes with 9 GRF genes being lost and 0 GRF genes being gained. This results in 6 existing GRF genes in A. trichopoda. There are 17 GRF genes in common ancestors of dicots and monocots. After 12 GRF genes were gained and 4 GRF genes were lost, 25 GRF genes exist in dicots ancestor species, after 20 GRF genes were gained and 10 GRF genes were lost, 25 GRF genes exist in monocots ancestor species. This indicates that the GRF gene family in the ancestor species of angiosperms has expanded after the divergence of monocots and dicots. After comparing the gain and loss of dicots with that of monocots, we found that the GRF gene family in monocots was gradually shrinking. For example, the ancestor of foxtail millet, sorghum, and maize has undergone 14 losses and 7 gains, which result in the reduction from 27 GRF genes to 20 GRF genes. Although the number of existing species of dicots is less than that of ancestors, it does not show a gradual shrinking phenomenon. For example, the ancestor of V. vinifera, P. pilosa, A. thaliana, and C. papaya has undergone 2 losses and 4 gains, but the number of GRF genes of the ancestor increased from 25 to 27.
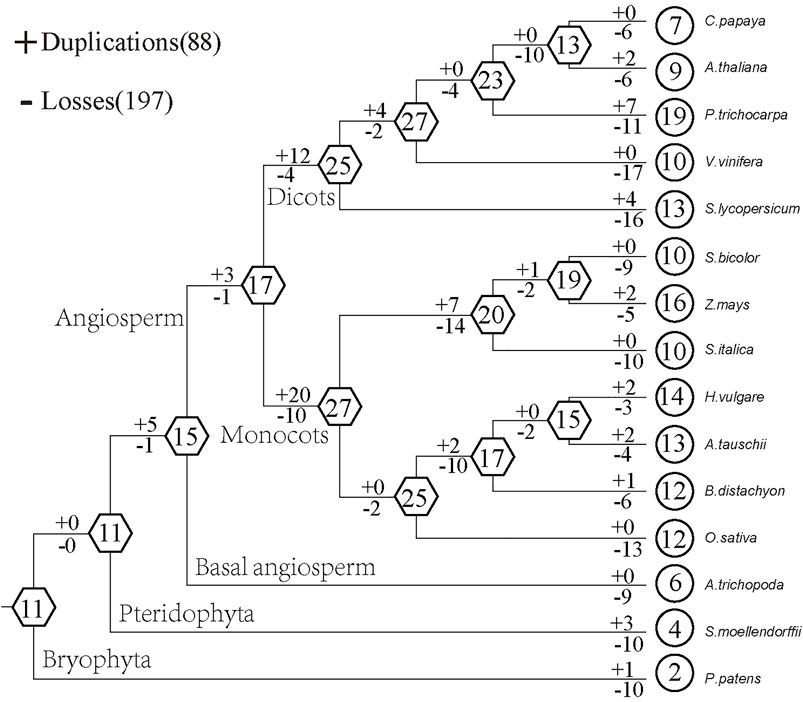
FIGURE 3. Schematic diagram of gain and loss of GRF gene family in plants. The numbers in the hexagons and circles represent the number of GRF genes in ancestors and existing species, and the + and − signs represent the gain and loss of genes, respectively.
Strong Collinearity Between Foxtail Millet GRFs and Related Species and Weaker Positive Selection
Combining with the results of internal collinearity in foxtail millet, we found that 6 pairs of GRF genes (seven genes, accounting for 70.00%) are in the collinearity block. Estimates of divergence time indicate that the divergence time of fragment duplication ranges from 15.07 Mya to 45.97 Mya, and GRFs are subject to purification options (Supplementary Table S3). Unfortunately, we did not find a tandem repeat gene pair.
In addition, we used a self-made Python script (https://github.com/ChenHuilong1223) to draw the GRF collinearity relationship between foxtail millet and other closely related species. We identified 15 pairs of collinearity genes in the collinearity region of the genome of foxtail millet and rice. Chromosome 1 of foxtail millet has the most collinearity GRF gene pairs (40.00%) with rice. Among them, there are collinearity GRF genes with chromosome 2, 4, and 6 of rice, respectively (Figure 4A). Similarly, 15 collinearity gene pairs (33.33%) were identified in foxtail millet and sorghum. Chromosome 1 of foxtail millet has the most collinearity GRF gene pairs with sorghum. Among them, there are collinearity GRF genes with chromosome 4, 6, and 10 of sorghum (Figure 4B). An abundance of collinearity gene pairs indicate that GRF of foxtail millet has strong collinearity with closely related species.
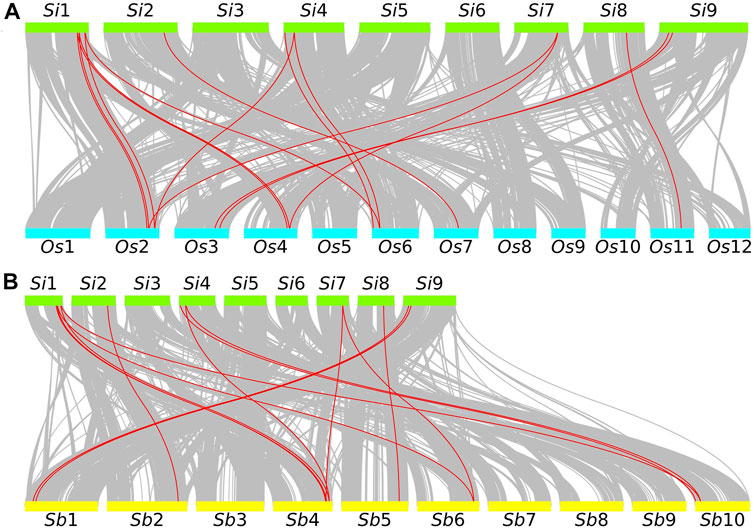
FIGURE 4. Collinearity analysis of foxtail millet GRF and related species. (A) The green rectangular color block represents the foxtail millet chromosome. Si represents foxtail millet. The number represents the chromosome number. The blue rectangular color block represents the rice chromosome. Os represents rice. The number represents the chromosome number. (B) The green rectangular color block represents the foxtail millet chromosome. Si represents foxtail millet. The number represents the chromosome number. The yellow rectangle represents the sorghum chromosome. Sb represents sorghum. The number represents the chromosome number.
The GRF phylogeny tree of foxtail millet shows that these 10 GRF genes can be assigned to A, B, C, and D class in the phylogenetic tree (Figure 5A). After selection pressure analysis, two (11.76%) of the 17 branches in the GRF gene tree of foxtail millet were detected to be positively selected. Therefore, this indicates that the GRF of foxtail millet has received weaker positive selection during the evolution process.
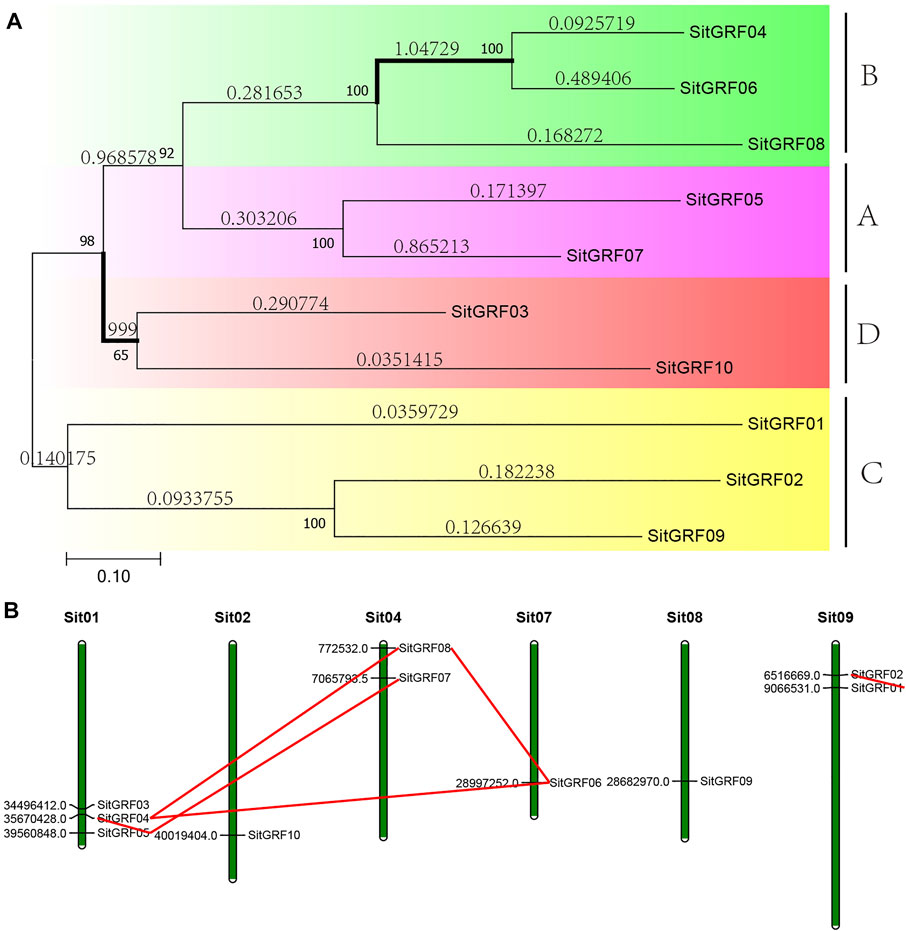
FIGURE 5. Phylogenetic tree and chromosome location of the GRF family of foxtail millet. (A) Phylogenetic analysis of foxtail millet GRF proteins using MEGA 7.0 via the neighbor-joining (NJ) method with 1000 bootstrap replicates. Bold branches suggest that they may be positively selected. (B) Chromosomal distribution and gene duplications of foxtail millet GRF genes. Green bars represent chromosomes of foxtail millet. The putative whole genome duplication (WGD) or segmental duplication genes are linked by a red line.
Characterization and Structure of GRFs in Foxtail Millet
We have identified 10 GRF genes in the foxtail millet genome. The amino acid lengths of GRFs in foxtail millet are between 232 and 590 amino acids. The molecular weights (MW) are between 34,981.10 and 61,607.68 Da. The predicted isoelectric point (PI) values are between 4.95 and 9.54. Prediction of subcellular location indicates that all 10 GRFs may be located in the nucleus (Supplementary Table S4).
The chromosome location shows that there is no GRF gene on chromosomes 3, 5, and 6 of the foxtail millet, no clustering phenomenon and scattered distribution on those chromosomes (Figure 5B). The remaining chromosomes have one to three GRF genes with the most being on chromosome 1.
Regarding the composition of the secondary structure of GRF in foxtail millet, a random coil occupies the largest proportion (49.63–67.83%). Alpha helix occupies the second largest proportion (18.26–36.57%), and extended strand occupies the third largest proportion (4.56–12.03%). Beta turn makes up the smallest proportion (3.04–6.08%) (Supplementary Table S5). In addition, we predicted the three-dimensional structure of the GRFs in foxtail millet. The results showed that the three-dimensional structure of the GRFs in foxtail millet is simple with no complicated spiral folding structures. The three-dimensional structure of the 10 GRFs is very similar (Figure 6).
The results of the amino acid sequence alignment of GRFs in foxtail millet indicate that all GRFs in foxtail millet contain QLQ and WRC (Figure 7C). The WRC domain contains a C3H motif spanning three cysteines and one histidine. The motif is CX9CX10CX2H, and the motif of the QLQ domain is QX3LX2Q. We also found that five GRF proteins contain FFD and TQL domains and are highly conserved (Figure 7D).
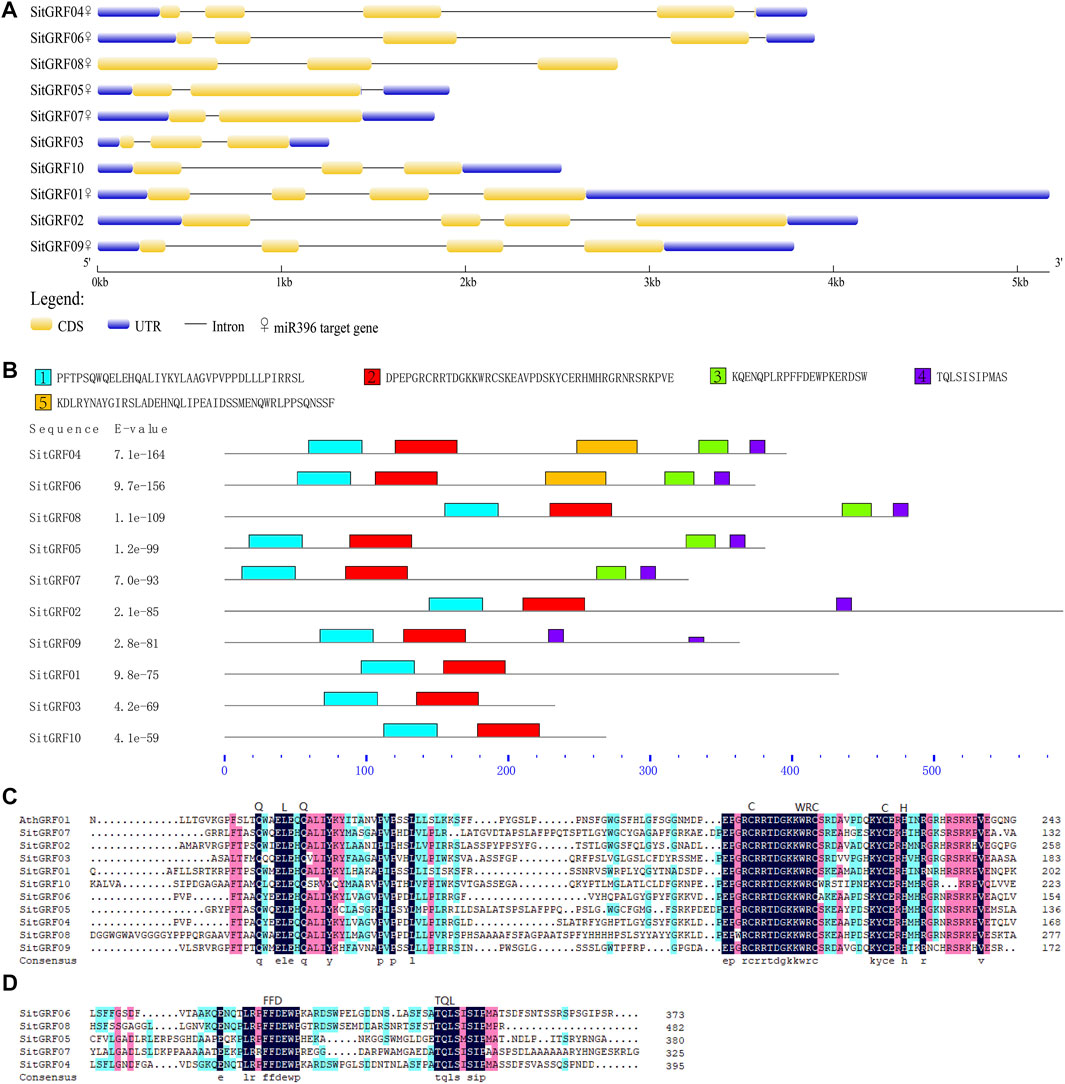
FIGURE 7. Characterization of foxtail millet GRFs. (A) Exon-intron organization and miR396 binding information of foxtail millet GRF family. (B) Distributions of conserved motifs in foxtail millet GRF genes. Five putative motifs are indicated in different colored boxes. (C) Comparison of the amino acid sequences of foxtail millet GRF QLQ and WRC domains. (D) Multiple sequence alignment of the amino acid sequences of foxtail millet GRF other domains.
We used MEME software to predict the GRF motifs in foxtail millet (Figure 7B). The results indicated that the conservative motifs 1 through 4 correspond to the corresponding domains. For example, motif 1 corresponds to QLQ, and motif 2 corresponds to WRC. In addition, we predicted that motif 5 exists in most members (seven) of the GRF family in foxtail millet.
In the structure of GRFs in foxtail millet, each GRF has two to four coding sequences (Figure 7A). The number of introns varies from one to four. It is noteworthy that SitGRF08 has no UTR, and the 3′ UTR of SitGRF01 is particularly long. However, SitGRF03 has the shortest length of the gene structure.
We also conducted the analysis of codon preference, and the results showed that the GRF gene family of foxtail millet is biased toward the use of G or C nucleotides. The ENC value is between 32.68 and 61.00, and 35.00 is the strength of codon preference. The closer to 61.00, the weaker the codon preference is, and vice versa. It shows that the overall preference of GRFs in foxtail millet is relatively weak (Supplementary Table S6). We also identified 19 optimal codons: UUU, UUG, AUU, GUA, UCU, CCG, ACG, GCA, UAA, CAU, CAG, AAU, AAG, GAU, GAA, UGC, CGC, AGU, and GGG (Supplementary Table S7). These results are helpful in the application of transgenic technology on foxtail millet.
cis-acting Elements and miRNA
We predicted cis-acting elements of the putative promoter region (upstream 1000 bp) of each GRF in foxtail millet (Figures 8A,B, Supplementary Table S8). We found that all gene promoters contained hormone-related cis-acting elements, and only four gene promoters were predicted to contain stress-related cis-acting elements. Seven hormone-related cis-acting elements were identified in the promoter region of GRFs in foxtail millet. These seven hormone-related cis-acting elements are ABRE (cis-acting element involved in abscisic acid responsiveness) (Hobo et al., 1999), CGTCA/TGACG-motif (cis-acting element involved in MeJA-responsiveness) (Rouster et al., 1997), GARE-motif/P-box (gibberellin-responsive cis-acting element) (Gubler and Jacobsen, 1992), TCA-element (cis-acting element involved in salicylic acid responsiveness) (Shah and Klessig, 1996), and TGA-element (auxin-responsive cis-acting element) (Khan et al., 2012). Three stress-related cis-acting elements were identified in the promoter region of GRFs in foxtail millet. The three stress-related cis-acting elements are ARE (cis-acting element essential for anaerobic induction), LTR (cis-acting element involved in low-temperature responsiveness), and MBS (MYB binding site involved in drought-inducibility) (Yoshida et al., 1998).
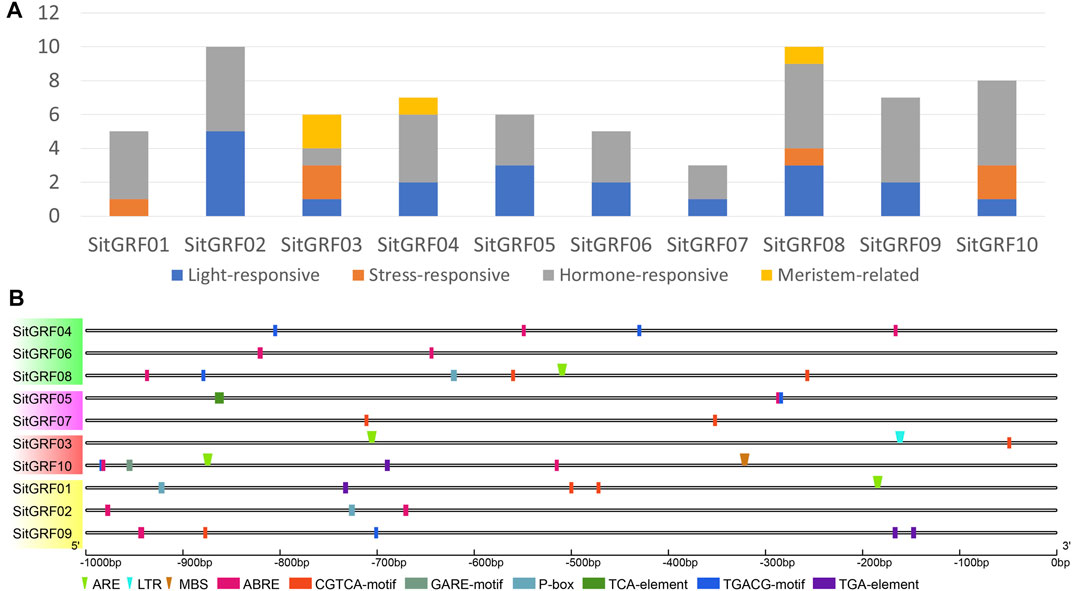
FIGURE 8. Promoter analysis of foxtail millet GRF genes. (A) Distribution of four kinds of cis-regulatory elements in the promoter of foxtail millet GRF genes. (B) The 1 Kb promoter sequences of corresponding GRF genes were used to analyze hormone-related cis-elements and stress-related elements. Rectangle indicates stress-related elements, and the inverted triangle indicates hormone-related elements. Different cis-elements were indicated by different colored symbols and placed in their relative position on the promoter.
According to previous studies, most GRFs are regulated by miR396 (Jones-Rhoades and Bartel, 2004; Liu et al., 2009; Zheng et al., 2018). Therefore, we predicted the GRF target gene of Sit-miR396 (Figure 7A, Supplementary Table S9). The results show that seven GRF target genes are predicted, and they all have a cleavage effect on each GRF gene.
Tissue Expression Analysis of GRF Genes in Foxtail Millet at Different Periods
By analyzing the expression pattern of GRF genes in foxtail millet in different tissues (Figure 9, Supplementary Table S10), the results showed that the expression of GRFs in foxtail millet was the strongest in seed, panicle, and stem tissues. The weakest expression patterns of GRF genes in foxtail millet were in different periods and were found during the third day of imbibed seeds. Some genes displayed higher expression, such as SitGRF06 (68.12 TPM) and SitGRF10 (29.86 TPM), followed by SitGRF08 (16.66 TPM). In the seedling stage on the 14th day, the expression of seven GRF genes (70.00%) all increased, while SitGRF06, SitGRF10, and SitGRF07 decreased. When the top first leaf of a 2-week-old seedling is fully extended, the gene expression changed little while the expression of SitGRF01, SitGRF09, SitGRF08, and SitGRF10 increased. In immature panicles, SitGRF02, SitGRF03, SitGRF04, SitGRF05, and SitGRF06 were significantly increased. The overall performance of the GRF family decreased gradually in the panicle at the pollination stage and at the grain-filling stage. However, SitGRF03 and SitGRF10 increased in the panicle at the grain-filling stage. In the flag leaf and the fourth leaf, the expression of seven GRF genes was extremely low while SitGRF07, SitGRF08, and SitGRF10 had higher expression. Additionally, the overall expression of the GRF family was extremely low and nine GRF genes (except SitGRF07) had high expression values in the stem tissue. Through observation, we found that SitGRF08 and SitGRF10 maintained high expression in the organization of each period, and SitGRF07 expressed lower in the organization of each period.
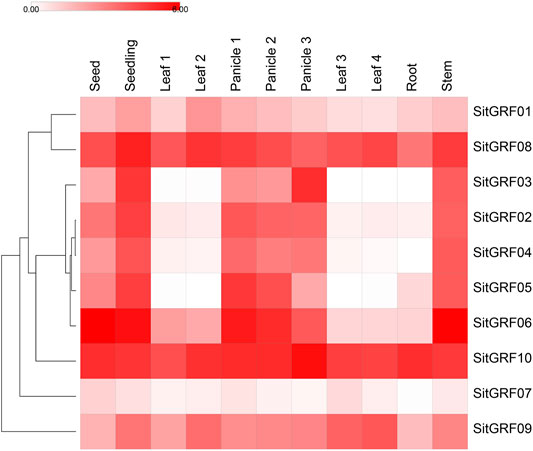
FIGURE 9. Expression of foxtail millet GRF genes in the different organs and periods. The shade of the color corresponds to the expression value (log2 (TPM+1)). Seed represents 3 days imbibed seeds; seedling represents 2-week-old whole seedling. Leaf 1 represents the top first fully extended leaf of a 2-week-old seedling. Leaf 2 represents the top second leaf of 30-day-old plants. Panicle 1 represents an immature panicle. Panicle 2 represents a panicle at the pollination stage. Panicle 3 represents a panicleat at the grain-filling stage. Leaf 3 represents a flag leaf. Leaf 4 represents the fourth leaf. Root represents the root. Stem represents the stem.
In order to definitely test the tissue expression patterns of SitGRF genes, qRT-PCR was used to detect the relative expression level of five SitGRF genes (SitGRF02, SitGRF06, SitGRF08, SitGRF09, and SitGRF10) in imbibed 3-day seed, 2-week-old seedling, and the root of 2-week-old seedling. The other five SitGRF genes were not detected due to the lack of screening specific primers across the introns. The results of qRT-PCR showed that among 15 pairs of comparison (five genes and three tissues), the expression trend of 13 pairs (86.67%) was consistent with the transcriptome data (Figure 10). For example, among the three tissues, SitGRF08 and SitGRF09 expressed the highest in the seedling and the lowest in the root, SitGRF10 expressed the highest in the germinated seed and the lowest in the seedling, In the meanwhile, both SitGRF02 and SitGRF06 expressed higher in the seedling than in the germinated seed. Only the expression pattern in the root of SitGRF02 and SitGRF06 was inconsistent. In qRT-PCR, SitGRF02 and SitGRF06 expressed the highest in the root, while in transcriptome, they were the lowest in the root.

FIGURE 10. Tissue specific expression analysis of SitGRF genes in germinated seed, 2-week-old seedling, and root. The bars represent the mean values of three replicates ± s.d. Significant differences in means are indicated by a, b, c, p < 0.01, according to one-way ANOVA test. a represents the comparation between germinated seed and seedling. b represents the comparation between seedling and root. c represents the comparation between germinated seed and root.
Protein Interaction Analysis
In order to further explore the mechanism of action of proteins expressed by the GRF genes of foxtail millet, we looked for an interaction protein for each GRF in the String database (Figure 11). Under high confidence (0.7) conditions, GRFs in foxtail millet have interaction proteins, except SitGRF04. Among them, SitGRF10 had the most interaction proteins. Each of SitGRF06 and SitGRF07 has only one interaction protein. These interaction proteins provide clues to the function and mechanism of each GRF. For example, both SitGRF01 and SitGRF08 could interact with auxin response factors, implying that they may participate in the network regulation mechanism of auxin response factors. We also found that SitGRF01, SitGRF02, SitGRF05, SitGRF07, SitGRF08, and SitGRF09 can interact with Si037326m.
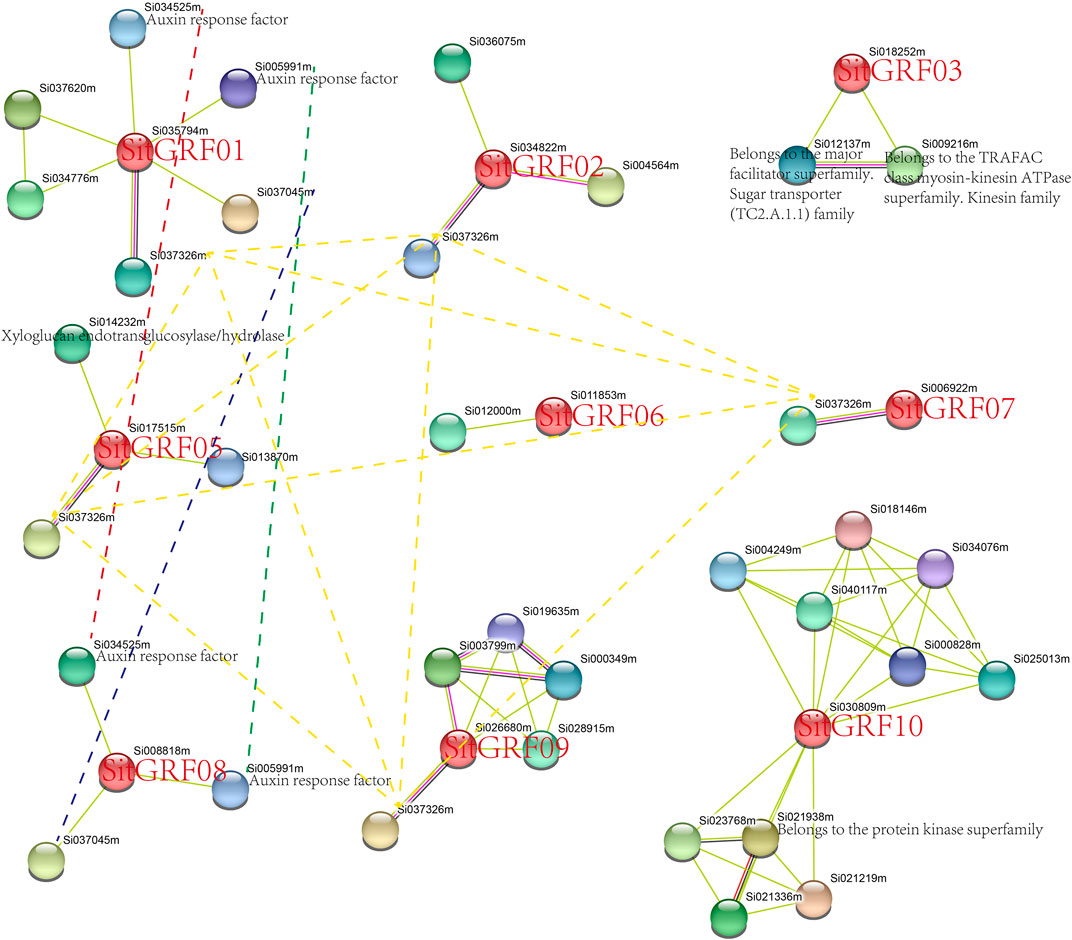
FIGURE 11. Protein interaction analysis of foxtail millet GRFs. The same protein is connected by a dotted line. The unannotated protein indicates uncharacterized protein in the String database.
Identification of Orthologs with Model Plants and Functional Annotation of GO
The identification of orthologous genes with GRF genes in model plants is helpful for the function prediction of GRF genes in foxtail millet. Through the identification of orthologous gene pairs of GRF with rice and Arabidopsis, it is found that the GRF gene family of foxtail millet and rice can form 10 pairs of orthologous genes. the GRF gene family of foxtail millet and rice have eight orthologous gene pairs. In addition, there are 10 orthologous gene pairs of the GRF gene family between Arabidopsis and rice (Figure 12).
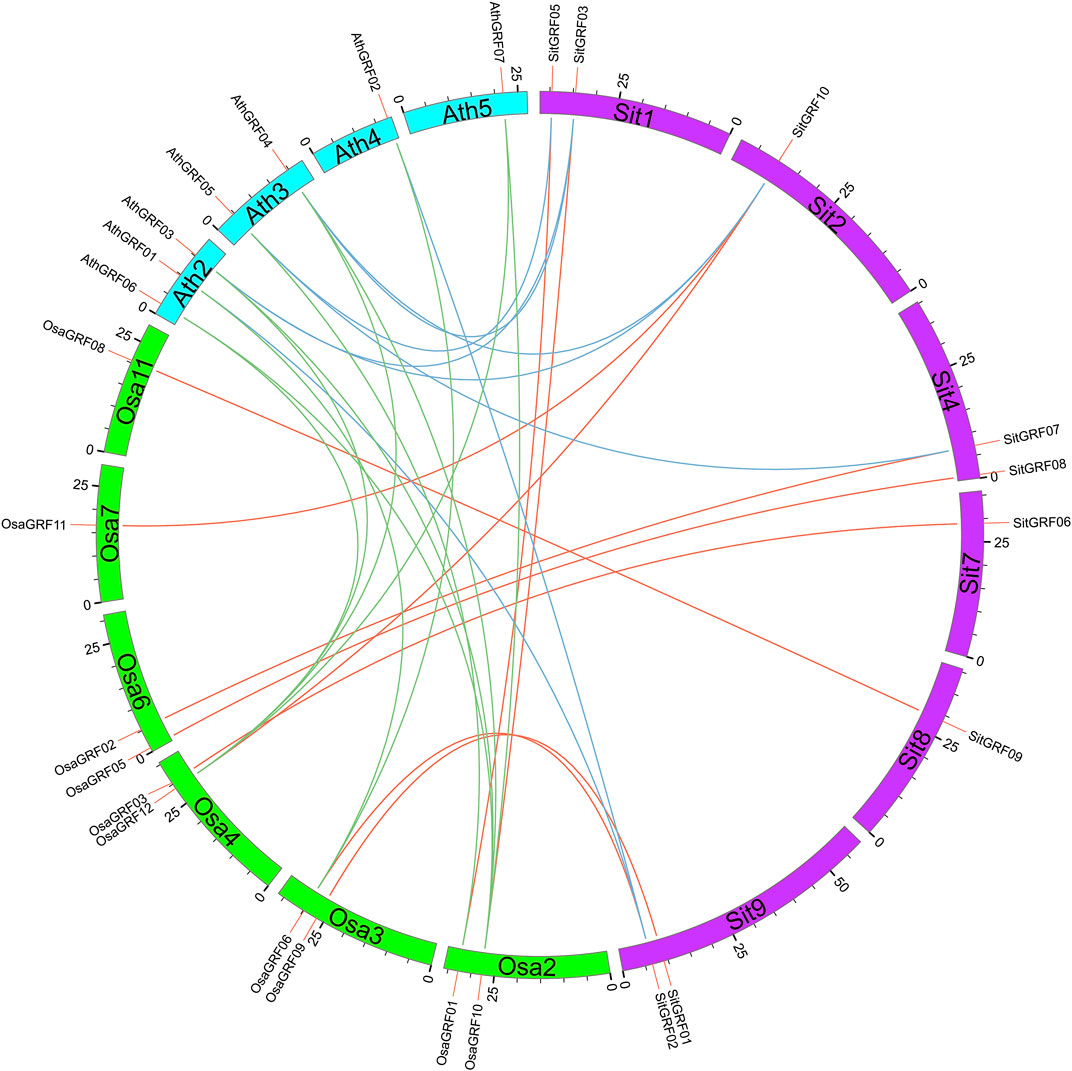
FIGURE 12. Orthologs of GRF genes between foxtail millet and model plants. The orthologous GRF gene pairs between different species are linked by different colors.
We performed GO function annotations and the results reported that all 10 GRFs of foxtail millet can participate in biological pathways, molecular functions, and cellular components (Supplementary Table S11). A total of 75 GO numbers could be annotated. The most annotated entries are in biological pathways (64.00%), such as developmental process, regulation of cellular biosynthetic process, regulation of cellular metabolic process, etc. Molecular functions (ATP binding, purine ribonucleoside triphosphate binding, purine nucleoside binding, etc.) accounted for 24.00%. The cellular component (nucleus, intracellular membrane-bounded organelle, membrane-bounded organelle, etc.) was 12.00%. Overall, GO function annotations found that GRF mainly functions in molecular pathways.
Discussion
In our research, the ancestors of land plants contain at least 11 GRF genes, which are not much different from the number of existing plants. This indicates that GRF genes have not expanded on a large scale. The GRF content of ancient species is very small, and it can always cluster in the ancient E branch in the phylogenetic tree. Thus, the GRF gene family of land plants originated from the E class. The number of GRF in higher plants is significantly elevated than that of ancient species (lower plants), meanwhile, the number of monocots is relatively stable and the number of dicots is more divergent. We found that the ancestors of angiosperms have experienced more gains and the family has expanded. However, the GRF gene family of monocots has been shrinking in the course of evolution, while the GRF gene family of dicots has not shown a gradual shrinking phenomenon. This points to the different evolutionary processes of monocots and dicots. Combining the results of identification and classification, we found that the low number of GRF in lower plants should be caused by excessive loss, rather than a small amount at the beginning.
Our research also showed that GRF in foxtail millet is affected by WGD or segmental duplication between 15.07 Mya and 45.97 Mya. The GRFs of foxtail millet and other closely related species have strong collinearity and homology. They were mainly subjected to purification selection in the past. These evolutionary phenomena indicate that the evolution of GRF is a conservative evolutionary model.
Comparing the qRT-PCR results with transcriptome data showed that 86.67% of the expression trend was consistent. Only 13.33% was inconsistent. This inconsistency has been reported in many literatures (Everaert et al., 2017). Celine Everaert et al. reported that about 85% of the genes showed consistent results between RNA-sequencing and qRT-PCR data. Our result was consistent with that reported. These results indicated that the transcriptome data was reliable.
Data Availability Statement
The RNA-seq data presented in the study are deposited in the Beijing Institute of Genomics Data Center ( https://bigd.big.ac.cn/) repository, accession number CRA001953.
Author Contributions
W.G. conceived the study; H.C. and W.G. conducted experiments; H.C. and W.G. analyzed data; H.C. and W.G. wrote the manuscript. All authors revised and approved the final manuscript.
Conflict of Interest
The authors declare that the research was conducted in the absence of any commercial or financial relationships that could be construed as a potential conflict of interest.
Publisher’s Note
All claims expressed in this article are solely those of the authors and do not necessarily represent those of their affiliated organizations, or those of the publisher, the editors, and the reviewers. Any product that may be evaluated in this article, or claim that may be made by its manufacturer, is not guaranteed or endorsed by the publisher.
Supplementary Material
The Supplementary Material for this article can be found online at: https://www.frontiersin.org/articles/10.3389/fgene.2021.727674/full#supplementary-material
References
Bailey, T. L., Boden, M., Buske, F. A., Frith, M., Grant, C. E., Clementi, L., et al. (2009). MEME Suite: Tools for Motif Discovery and Searching. Nucleic Acids Res. 37 (Suppl. l_2), W202–W208. doi:10.1093/nar/gkp335
Baloglu, M. C. (2014). Genome-wide In Silico Identification and Comparison of Growth Regulating Factor (GRF) Genes in Cucurbitaceae Family. Plant Omics 7 (4), 260–270. doi:10.1097/01.tp.0000332556.64365.e7
Bateman, A., Heger, A., Sonnhammer, E. L. L., Mistry, J., Clements, J., Tate, J., et al. (2013). Pfam: the Protein Families Database. Nucleic Acids Res. 42 (D1), D222–D230. doi:10.1093/nar/gkt1223
Bennetzen, J. L., Schmutz, J., Wang, H., Percifield, R., Hawkins, J., Pontaroli, A. C., et al. (2012). Reference Genome Sequence of the Model Plant Setaria. Nat. Biotechnol. 30 (6), 555–561. doi:10.1038/nbt.2196
Camacho, C., Coulouris, G., Avagyan, V., Ma, N., Papadopoulos, J., Bealer, K., et al. (2009). BLAST+: Architecture and Applications. BMC Bioinformatics 10 (1), 421. doi:10.1186/1471-2105-10-421
Cao, Y., Han, Y., Jin, Q., Lin, Y., and Cai, Y. (2016). Comparative Genomic Analysis of the GRF Genes in Chinese Pear (Pyrus Bretschneideri Rehd), Poplar (Populous), Grape (Vitis vinifera), Arabidopsis and Rice (Oryza Sativa). Front. Plant Sci. 7 (577), 1750. doi:10.3389/fpls.2016.01750
Chai, W., Si, W., Ji, W., Qin, Q., Zhao, M., and Jiang, H. (20182018). Genome-Wide Investigation and Expression Profiling of HD-Zip Transcription Factors in Foxtail Millet (Setaria Italica L.). Biomed. Res. Int. 2018, 8457614. doi:10.1155/2018/8457614
Chen, H. L., Wang, X. Y., and Ge, W. N. (2021). Comparative Genomics of Three-Domain Multi-Copper Oxidase Gene Family in Foxtail Millet (Setaria Italica L.). Comput. Mol. Biol. 11 (4), 1–13. doi:10.5376/cmb.2021.11.0004
Choi, D., Kim, J. H., and Kende, H. (2004). Whole Genome Analysis of the OsGRF Gene Family Encoding Plant-specific Putative Transcription Activators in Rice (Oryza Sativa L.). Plant Cell Physiol. 45 (7), 897–904. doi:10.1093/pcp/pch098
Chou, K.-C., and Shen, H.-B. (2010). Plant-mPLoc: a Top-Down Strategy to Augment the Power for Predicting Plant Protein Subcellular Localization. PloS one 5 (6), e11335. doi:10.1371/journal.pone.0011335
Dai, X., Zhuang, Z., and Zhao, P. X. (2018). psRNATarget: a Plant Small RNA Target Analysis Server (2017 Release). Nucleic Acids Res. 46 (W1), W49–W54. doi:10.1093/nar/gky316
Darriba, D., Taboada, G. L., Doallo, R., and Posada, D. (2011). ProtTest 3: Fast Selection of Best-Fit Models of Protein Evolution. Bioinformatics 27 (8), 1164–1165. doi:10.1093/bioinformatics/btr088
Emms, D. M., and Kelly, S. (2015). OrthoFinder: Solving Fundamental Biases in Whole Genome Comparisons Dramatically Improves Orthogroup Inference Accuracy. Genome Biol. 16 (1), 157. doi:10.1186/s13059-015-0721-2
Everaert, C., Luypaert, M., Maag, J. L. V., Cheng, Q. X., Dinger, M. E., Hellemans, J., et al. (2017). Benchmarking of RNA-Sequencing Analysis Workflows Using Whole-Transcriptome RT-qPCR Expression Data. Sci. Rep. 7 (1), 1–11. doi:10.1038/s41598-017-01617-3
Eyre-Walker, A. C. (1991). An Analysis of Codon Usage in Mammals: Selection or Mutation Bias? J. Mol. Evol. 33 (5), 442–449. doi:10.1007/bf02103136
Filiz, E., Koç, İ., and TombuloğLu, H. (2014). Genome-wide Identification and Analysis of Growth Regulating Factor Genes in Brachypodium Distachyon: In Silico Approaches. Turkish J. Biol. 38, 296–306. doi:10.3906/biy-1308-57
Gasteiger, E., Hoogland, C., Gattiker, A., Duvaud, S. e., Wilkins, M. R., Appel, R. D., et al. (2005). “Protein Identification and Analysis Tools on the ExPASy Server,” in The Proteomics Protocols Handbook. Editor J. M. Walker (Totowa, NJ: Humana Press), 571–607. doi:10.1385/1-59259-890-0:571
Gubler, F., and Jacobsen, J. V. (1992). Gibberellin-responsive Elements in the Promoter of a Barley High-pI Alpha-Amylase Gene. Plant Cell 4 (11), 1435–1441. doi:10.1105/tpc.4.11.1435
Guindon, S., Dufayard, J.-F., Lefort, V., Anisimova, M., Hordijk, W., and Gascuel, O. (2010). New Algorithms and Methods to Estimate Maximum-Likelihood Phylogenies: Assessing the Performance of PhyML 3.0. Syst. Biol. 59 (3), 307–321. doi:10.1093/sysbio/syq010
Hall, T. (1999). BioEdit: A User-Friendly Biological Sequence Alignment Editor and Analysis Program for Windows 95/98/NT[C]. Nucleic Acids Symp. Ser. 41, 95–98. doi:10.1021/bk-1999-0734.ch008
Han, J., Xie, H., Sun, Q., Wang, J., Lu, M., Wang, W., et al. (2014). Bioinformatic Identification and Experimental Validation of miRNAs from Foxtail Millet (Setaria Italica). Gene 546 (2), 367–377. doi:10.1016/j.gene.2014.05.050
Hobo, T., Asada, M., Kowyama, Y., and Hattori, T. (1999). ACGT-containing Abscisic Acid Response Element (ABRE) and Coupling Element 3 (CE3) Are Functionally Equivalent. Plant J. 19 (6), 679–689. doi:10.1046/j.1365-313x.1999.00565.x
Hu, B., Jin, J., Guo, A. Y., Zhang, H., Luo, J., and Gao, G. (2014). GSDS 2.0: an Upgraded Gene Feature Visualization Server. Bioinformatics 31 (8), 1296–1297. doi:10.1093/bioinformatics/btu817
Ji, J. H., Zhou, Y. J., Wu, H. H., and Yang, L. M. (2015). Genome-wide Analysis and Functional Prediction of the Trihelix Transcription Factor Family in rice. Yi Chuan 37, 1228–1241. doi:10.16288/j.yczz.15-196
Jia, G., Huang, X., Zhi, H., Zhao, Y., Zhao, Q., Li, W., et al. (2013). A Haplotype Map of Genomic Variations and Genome-wide Association Studies of Agronomic Traits in Foxtail Millet (Setaria Italica). Nat. Genet. 45, 957–961. doi:10.1038/ng.2673
Jones-Rhoades, M. W., and Bartel, D. P. (2004). Computational Identification of Plant MicroRNAs and Their Targets, Including a Stress-Induced miRNA. Mol. Cell 14 (6), 787–799. doi:10.1016/j.molcel.2004.05.027
Katoh, K., and Standley, D. M. (2013). MAFFT Multiple Sequence Alignment Software Version 7: Improvements in Performance and Usability. Mol. Biol. Evol. 30 (4), 772–780. doi:10.1093/molbev/mst010
Khan, M. R., Hu, J., and Ali, G. M. (2012). Reciprocal Loss of CArG-Boxes and Auxin Response Elements Drives Expression Divergence of MPF2-like MADS-Box Genes Controlling Calyx Inflation. PLoS One 7, e42781. doi:10.1371/journal.pone.0042781
Khatun, K., Robin, A. H. K., Park, J.-I., Nath, U. K., Kim, C. K., Lim, K.-B., et al. (2017). Molecular Characterization and Expression Profiling of Tomato GRF Transcription Factor Family Genes in Response to Abiotic Stresses and Phytohormones. Ijms 18 (5), 1056. doi:10.3390/ijms18051056
Kim, J. H., Choi, D., and Kende, H. (2003). The AtGRF Family of Putative Transcription Factors Is Involved in Leaf and Cotyledon Growth inArabidopsis. Plant J. 36 (1), 94–104. doi:10.1046/j.1365-313x.2003.01862.x
Krzywinski, M., Schein, J., Birol, İ., Connors, J., Gascoyne, R., Horsman, D., et al. (2009). Circos: an Information Aesthetic for Comparative Genomics. Genome Res. 19 (9), 1639–1645. doi:10.1101/gr.092759.109
Kumar, K., Muthamilarasan, M., and Prasad, M. (2013). Reference Genes for Quantitative Real-Time PCR Analysis in the Model Plant Foxtail Millet (Setaria Italica L.) Subjected to Abiotic Stress Conditions. Plant Cell Tiss Organ. Cult 115 (1), 13–22. doi:10.1007/s11240-013-0335-x
Kumar, S., Stecher, G., and Tamura, K. (2016). MEGA7: Molecular Evolutionary Genetics Analysis Version 7.0 for Bigger Datasets. Mol. Biol. Evol. 33 (7), 1870–1874. doi:10.1093/molbev/msw054
Lescot, M., Déhais, P., Thijs, G., Marchal, K., Moreau, Y., Van de Peer, Y., et al. (2002). PlantCARE, a Database of Plant Cis-Acting Regulatory Elements and a portal to Tools for In Silico Analysis of Promoter Sequences. Nucleic Acids Res. 30 (1), 325–327. doi:10.1093/nar/30.1.325
Li, L., Stoeckert, C. J., and Roos, D. S. (2003). OrthoMCL: Identification of Ortholog Groups for Eukaryotic Genomes. Genome Res. 13 (9), 2178–2189. doi:10.1101/gr.1224503
Li, P., and Brutnell, T. P. (2011). Setaria Viridis and Setaria Italica, Model Genetic Systems for the Panicoid Grasses. J. Exp. Bot. 62 (9), 3031–3037. doi:10.1093/jxb/err096
Li, S., Dong, X., Fan, G., Yang, Q., Shi, J., Wei, W., et al. (2018). Comprehensive Profiling and Inheritance Patterns of Metabolites in Foxtail Millet. Front. Plant Sci. 9, 1716. doi:10.3389/fpls.2018.01716
Liu, D., Song, Y., Chen, Z., and Yu, D. (2009). Ectopic Expression of miR396 suppressesGRFtarget Gene Expression and Alters Leaf Growth inArabidopsis. Physiologia Plantarum 136 (2), 223–236. doi:10.1111/j.1399-3054.2009.01229.x
Luo, M.-C., Gu, Y. Q., Puiu, D., Wang, H., Twardziok, S. O., Deal, K. R., et al. (2017). Genome Sequence of the Progenitor of the Wheat D Genome Aegilops Tauschii. Nature 551 (7681), 498–502. doi:10.1038/nature24486
Lynch, M., and Conery, J. S. (2000). The Evolutionary Fate and Consequences of Duplicate Genes. Science 290 (5494), 1151–1155. doi:10.1126/science.290.5494.1151
Ma, J.-Q., Jian, H.-J., Yang, B., Lu, K., Zhang, A.-X., Liu, P., et al. (2017). Genome-wide Analysis and Expression Profiling of the GRF Gene Family in Oilseed Rape ( Brassica Napus L.). Gene 620, 36–45. doi:10.1016/j.gene.2017.03.030
Mehanathan, M., Rohit, K., Chandra Bhan, Y., Venkata Suresh, B., Yusuf, K., and Manoj, P. (2014). Identification and Molecular Characterization of MYB Transcription Factor Superfamily in C4 Model Plant Foxtail Millet (Setaria Italica L.). Plos One 9 (10), e109920. doi:10.1371/journal.pone.0109920s
Mistry, J., Finn, R. D., Eddy, S. R., Bateman, A., and Punta, M. (2013). Challenges in Homology Search: HMMER3 and Convergent Evolution of Coiled-Coil Regions. Nucleic Acids Res. 41 (12), e121. doi:10.1093/nar/gkt263
Nei, M., and Gojobori, T. (1986). Simple Methods for Estimating the Numbers of Synonymous and Nonsynonymous Nucleotide Substitutions. Mol. Biol. Evol. 3 (5), 418–426. doi:10.1093/oxfordjournals.molbev.a040410
Pandey, G., Misra, G., Kumari, K., Gupta, S., Parida, S. K., Chattopadhyay, D., et al. (2013). Genome-Wide Development and Use of Microsatellite Markers for Large-Scale Genotyping Applications in Foxtail Millet [Setaria Italica (L.)]. DNA Res. 20 (2), 197–207. doi:10.1093/dnares/dst002
Rodriguez, R. E., Ercoli, M. F., Debernardi, J. M., and Palatnik, J. F. (2016). “Growth-Regulating Factors, A Transcription Factor Family Regulating More Than Just Plant Growth,” in Plant Transcription Factors. Editor D. H. Gonzalez (Boston: Academic Press), 269–280. doi:10.1016/b978-0-12-800854-6.00017-8
Rouster, J., Leah, R., Mundy, J., and Cameron-Mills, V. (1997). Identification of a Methyl Jasmonate-Responsive Region in the Promoter of a Lipoxygenase 1 Gene Expressed in Barley Grain. Plant J. 11 (3), 513–523. doi:10.1046/j.1365-313x.1997.11030513.x
Rukmangada, M. S., Sumathy, R., Sivaprasad, V., and Naik, V. G. (2018). Genome-wide Identification and Characterization of Growth-Regulating Factors in mulberry (Morus spp.). Trees 32 (6), 1695–1705. doi:10.1007/s00468-018-1744-6
Shah, J., and Klessig, D. F. (1996). Identification of a Salicylic Acid-Responsive Element in the Promoter of the Tobacco Pathogenesis-Related Beta-1,3-Glucanase Gene, PR-2d. Plant J. 10 (6), 1089–1101. doi:10.1046/j.1365-313x.1996.10061089.x
Shang, S., Wu, C., Huang, C., Tie, W., Yan, Y., Ding, Z., et al. (2018). Genome-Wide Analysis of the GRF Family Reveals Their Involvement in Abiotic Stress Response in Cassava. Genes 9 (2), 110. doi:10.3390/genes9020110
Song, X., Ma, X., Li, C., Hu, J., Yang, Q., Wang, T., et al. (2018). Comprehensive Analyses of the BES1 Gene Family in Brassica Napus and Examination of Their Evolutionary Pattern in Representative Species. BMC genomics 19 (1), 346–415. doi:10.1186/s12864-018-4744-4
Suyama, M., Torrents, D., and Bork, P. (2006). PAL2NAL: Robust Conversion of Protein Sequence Alignments into the Corresponding Codon Alignments. Nucleic Acids Res. 34 (Suppl. l_2), W609–W612. doi:10.1093/nar/gkl315
Szklarczyk, D., Franceschini, A., Wyder, S., Forslund, K., Heller, D., Huerta-Cepas, J., et al. (2015). STRING V10: Protein-Protein Interaction Networks, Integrated over the Tree of Life. Nucleic Acids Res. 43 (Database issue), D447–D452. doi:10.1093/nar/gku1003
Thompson, J. D., Higgins, D. G., and Gibson, T. J. (1994). CLUSTAL W: Improving the Sensitivity of Progressive Multiple Sequence Alignment through Sequence Weighting, Position-specific gap Penalties and Weight Matrix Choice. Nucleic Acids Res. 22 (22), 4673–4680. doi:10.1093/nar/22.22.4673
Tian, T., Liu, Y., Yan, H., You, Q., Yi, X., Du, Z., et al. (2017). agriGO v2.0: a GO Analysis Toolkit for the Agricultural Community, 2017 Update. Nucleic Acids Res. 45 (W1), W122–W129. doi:10.1093/nar/gkx382
van der Knaap, E., Kim, J. H., and Kende, H. (2000). A Novel Gibberellin-Induced Gene from Rice and its Potential Regulatory Role in Stem Growth. Plant Physiol. 122 (3), 695–704. doi:10.1104/pp.122.3.695
Voorrips, R. E. (2002). MapChart: Software for the Graphical Presentation of Linkage Maps and QTLs. J. Hered. 93 (1), 77–78. doi:10.1093/jhered/93.1.77
Wang, D., Zhang, Y., Zhang, Z., Zhu, J., and Yu, J. (2010). KaKs_Calculator 2.0: A Toolkit Incorporating Gamma-Series Methods and Sliding Window Strategies. Genomics, Proteomics & Bioinformatics 8 (1), 77–80. doi:10.1016/s1672-0229(10)60008-3
Wang, F., Qiu, N., Ding, Q., Li, J., Zhang, Y., Li, H., et al. (2014). Genome-wide Identification and Analysis of the Growth-Regulating Factor Family in Chinese Cabbage (Brassica Rapa L. Ssp. Pekinensis). BMC Genomics 15, 807. doi:10.1186/1471-2164-15-807
Wang, X., Wang, J., Jin, D., Guo, H., Lee, T.-H., Liu, T., et al. (2015). Genome Alignment Spanning Major Poaceae Lineages Reveals Heterogeneous Evolutionary Rates and Alters Inferred Dates for Key Evolutionary Events. Mol. Plant 8 (6), 885–898. doi:10.1016/j.molp.2015.04.004
Wang, Y., Tang, H., DeBarry, J. D., Tan, X., Li, J., Wang, X., et al. (2012). MCScanX: a Toolkit for Detection and Evolutionary Analysis of Gene Synteny and Collinearity. Nucleic Acids Res. 40 (7), e49. doi:10.1093/nar/gkr1293
Wilm, A., Higgins, D. G., Valentin, F., Blackshields, G., McWilliam, H., Wallace, I. M., et al. (2007). Clustal W and Clustal X Version 2.0. Bioinformatics 23 (21), 2947–2948. doi:10.1093/bioinformatics/btm404
Yadav, A., Khan, Y., and Prasad, M. (2016). Dehydration-responsive miRNAs in Foxtail Millet: Genome-wide Identification, Characterization and Expression Profiling. Planta 243 (3), 749–766. doi:10.1007/s00425-015-2437-7
Yang, Z., Nielsen, R., and Hasegawa, M. (1998). Models of Amino Acid Substitution and Applications to Mitochondrial Protein Evolution. Mol. Biol. Evol. 15 (12), 1600–1611. doi:10.1093/oxfordjournals.molbev.a025888
Yang, Z. (1997). PAML: a Program Package for Phylogenetic Analysis by Maximum Likelihood. Bioinformatics 13 (5), 555–556. doi:10.1093/bioinformatics/13.5.555
Yang, Z., Zhang, H., Li, X., Shen, H., Gao, J., Hou, S., et al. (2020). A Mini Foxtail Millet with an Arabidopsis-like Life Cycle as a C4 Model System. Nat. Plants 6 (9), 1167–1178. doi:10.1038/s41477-020-0747-7
Yoshida, H., Haze, K., Yanagi, H., Yura, T., and Mori, K. (1998). Identification of the Cis-Acting Endoplasmic Reticulum Stress Response Element Responsible for Transcriptional Induction of Mammalian Glucose-Regulated Proteins. J. Biol. Chem. 273 (50), 33741–33749. doi:10.1074/jbc.273.50.33741
Zhang, D.-F., Li, B., Jia, G.-Q., Zhang, T.-F., Dai, J.-R., Li, J.-S., et al. (2008). Isolation and Characterization of Genes Encoding GRF Transcription Factors and GIF Transcriptional Coactivators in Maize (Zea mays L.). Plant Sci. 175 (6), 809–817. doi:10.1016/j.plantsci.2008.08.002
Zhang, G., Liu, X., Quan, Z., Cheng, S., Xu, X., Pan, S., et al. (2012). Genome Sequence of Foxtail Millet (Setaria Italica) Provides Insights into Grass Evolution and Biofuel Potential. Nat. Biotechnol. 30 (6), 549–554. doi:10.1038/nbt.2195
Zhang, J., Li, Z., Jin, J., Xie, X., Zhang, H., Chen, Q., et al. (2017). Genome-wide Identification and Analysis of the Growth-Regulating Factor Family in Tobacco (Nicotiana Tabacum). Gene 639, 117–127. doi:10.1016/j.gene.2017.09.070
Keywords: growth-regulating factors, foxtail millet, structure, expression, loss
Citation: Chen H and Ge W (2022) Identification, Molecular Characteristics, and Evolution of GRF Gene Family in Foxtail Millet (Setaria italica L.). Front. Genet. 12:727674. doi: 10.3389/fgene.2021.727674
Received: 19 June 2021; Accepted: 20 December 2021;
Published: 03 February 2022.
Edited by:
Million Tadege, Oklahoma State University, United StatesReviewed by:
Ghulam Qanmber, Cotton Research Institute (CAAS), ChinaLifang Niu, Biotechnology Research Institute (CAAS), China
Copyright © 2022 Chen and Ge. This is an open-access article distributed under the terms of the Creative Commons Attribution License (CC BY). The use, distribution or reproduction in other forums is permitted, provided the original author(s) and the copyright owner(s) are credited and that the original publication in this journal is cited, in accordance with accepted academic practice. No use, distribution or reproduction is permitted which does not comply with these terms.
*Correspondence: Weina Ge, gwn-06@163.com
†These authors have contributed equally to this work.