- 1Department of General Surgery, Qilu Hospital, Shandong University, Jinan, China
- 2Department of Hepatobiliary Surgery, The Second Hospital of Shandong University, Jinan, China
Background: The relationship between baseline C-reactive protein (CRP) level and the prognosis of cancer patients receiving immune checkpoint inhibitor (ICI) treatment remains controversial. The aim of this meta-analysis was to clarify whether baseline CRP level can serve as a biomarker to predict the efficiency of ICI therapy.
Methods: All associated articles published in the Cochrane Library, EMBASE, and PubMed databases from the inception of the database to December 30, 2021, were retrieved. Progression-free survival (PFS) and overall survival (OS) outcomes were meta-analyzed using the random-effects model and adjusted using the trim-and-fill method because of publication bias.
Results: Thirty-three studies (6,124 patients) conducted between 2013 and 2021 were identified. The pooled outcomes implied that high baseline CRP level patients had significantly worse OS (adjusted pooled value for univariate and multivariate analysis outcomes: HR = 1.48, 95% CI = 1.41–1.56; HR = 1.46, 95% CI = 1.34–1.59) and PFS (adjusted pooled value for univariate and multivariate analysis outcomes: HR = 1.29, 95% CI = 1.15–1.45; HR = 1.20, 95% CI = 1.02–1.40) than low baseline CRP level patients, irrespective of cancer or ICI type. Further analysis indicated that 1 mg/dl was appropriate as a cutoff value for determining the low or high level of baseline CRP to predict the OS or PFS of cancer patients receiving ICI treatment (univariate analysis: HR = 1.56, 95% CI = 1.24–1.97, P = 0.909; multivariate analysis: HR = 1.58, 95% CI = 1.23–2.03, P = 0.521).
Conclusions: High baseline CRP level (>1 mg/dl) may be an indicator for worse OS and PFS of cancer patients treated with ICIs. More high-quality prospective studies are warranted to assess the predictive value of CRP for ICI treatment.
Highlights
● Our study provides a comprehensive review and meta-analysis and indicates that high baseline CRP level (>1 mg/dl) may be a good predictor for recurrence and worse survival of cancer patients who received ICI treatment.
Introduction
Recently, immune checkpoint inhibitors (ICIs) have been more and more commonly applied in clinical use for cancer patients. ICIs mainly contain monoclonal antibodies against programmed cell death 1 (PD-1), its primary ligand (PD-L1), and cytotoxic T lymphocyte-associated protein 4 (CTLA-4) (1, 2). ICIs operate predominantly by deregulating the function of T cells and other immune cells and have shown remarkable effectiveness in the treatment of multiple solid malignancies (3–8). However, some tumors showed intrinsic resistance to ICIs which has gravely restricted the efficiency of ICIs (9). The exact resistance mechanism remains to be determined and may comprise multiple drivers in the light of contemporary findings (2). Therefore, in the area of ICI treatment, it is critical to identify predictors which can specifically anticipate the curative benefits of ICIs. With these predictors, we can guarantee the application of ICIs and presume the concrete resistance mechanisms to furnish orientation toward the subsequent resolution of the resistance mechanism.
Persistent inflammation is increasingly recognized to cause or contribute to immunosuppression (10, 11), which will impair the effect of ICIs or even lead to resistance in cancer patients. Thus, inflammatory biomarkers, including C-reactive protein (CRP), may be reliable prognostic biomarkers for cancer patients receiving ICI treatment. CRP was initially identified in 1930 as a serum protein, which is synthesized in the liver and vigorously responds to the capsular (“C”) polysaccharide of pneumococcus (12). There have previously been plenty of meta-analyses corroborating the correlation between elevated baseline CRP level and poor outcomes of patients with various cancers (13–16). In contrast, there is no confirmatory evidence-based medical research on whether the predictive effect of CRP is applicable to cancer patients treated with ICIs. Numerous studies have demonstrated the predictive value of CRP on ICI treatment (17), yet some studies also exist which argue that CRP lacks predictive power; thus, the existence and definite magnitude of the predictive power of CRP on the prognosis of cancer patients treated with ICIs are controversial and remain to be determined.
Herein, according to the 33 implemented studies, a meta-analysis was conducted. The corrected consolidated hazard ratio (HR) with 95% confidence interval (95% CI) was used to verify the correlations between pretreatment CRP level and overall survival (OS) and progression-free survival (PFS) of cancer patients to assess whether CRP could serve as a predictive biomarker for cancer patients receiving ICI treatment.
Methods
This study was undertaken according to the Preferred Reporting Items for Systematic Reviews and Meta-Analyses (PRISMA) guidelines (18), and the selection criteria were established following the PICOS model (population, intervention, comparison, outcome, and study design).
Search Strategies and Selection Criteria
Suitable studies were searched from the Cochrane Library literature, PubMed, and EMBASE databases from the inception of the database until December 30, 2021, and the language was restricted to English. Overall, 33 studies conducted between 2013 and 2021 were obtained. Carcinoma, neoplasm, malignancy, cancer, C-reactive protein, CRP, immune checkpoint inhibitor, ICIs, avelumab, durvalumab, tremelimumab, pembrolizumab, camrelizumab, ipilimumab, tislelizumab, SHR-1210, toripalimab, penpulimab, nivolumab, atezolizumab, PD-1, PD-L1, and CTLA-4 were employed as the literature search keywords. The detailed search strategy and retrieval methods are presented in Supplementary Table 1.
The selection criteria were as follows: 1) patients were diagnosed with cancer and treated with ICIs; 2) correlations between CRP and prognostic outcomes, such as OS or PFS, were assessed in the form of the HR with 95% CI; 3) published in English; and 4) no duplicate publication of data. For republished studies, only the studies with the most comprehensive data were collected.
Data Extraction and Quality Assessment
Three independent reviewers (C-LH, L-JY, and HL) evaluated the availability of each study, and disagreements were discussed and addressed with B-WT. The following data were retrieved for each study: study ID (last name of the first author plus publication year), country, study period, data collection, ICIs, cancer type, sample size, outcome, cutoff value, and HR and 95% CI for OS and PFS derived from univariate analysis or multivariate analysis.
We appraised study quality using the Newcastle-Ottawa Scale criteria (19). Studies are divided into high- (scores greater than 7), medium- (scores within 5 to 7), and low-quality (scores less than 5) studies.
Statistical Analysis
Data analysis was conducted by Stata 12.0 (Stata Corp LP, College Station, TX). P <0.05 was regarded as statistically significant, and the random-effects model was used. Gross variation was categorized by dissimilarity (I2); amounts greater than 25%, 50%, to 75% were considered minor, moderate, to large. The OS and PFS were compared between high baseline CPR group and low baseline CPR group through pooled HR and 95% CI. If removal of a study outcome in the sensitivity analysis results in a significant bias of the pooled HR and 95% CI, the outcome will be eliminated. A funnel plot is a visual tool for testing publication bias wherein the plot should resemble a symmetrical inverted funnel when lacking publication bias (20); otherwise, it is asymmetrical. We identified significant publication bias through funnel plots and ultimately obtained the adjusted pooled HR and 95% CI by the trim-and-fill method to eliminate publication bias. Subgroup analyses were performed by cancer type, sample size, country, and ICI type to determine the potential sources of heterogeneity.
Results
Literature Selection and Study Characteristics
The extraction procedure of relevant literature is shown in Figure 1. Initially, 539 records were selected, leaving 373 studies after eliminating duplicates. Following initial screening by title and abstract, 286 papers were excluded. Then, 54 studies were removed from the full-text review due to lack of expected outcomes, duplicated data, or unavailability of full text, resulting in a pool of 33 suitable studies published between 2013 and 2021 (21–53). All studies covered a range of 11 countries, 9 types of cancer, 6 types of ICIs, and 6,124 individuals, as detailed in Table 1. There were only 2 studies with NOS scores of 4, while the rest had NOS scores between 5 and 7 (Supplementary Table 2).
Relationship Between Baseline CRP and OS in Cancer Patients Treated With ICIs
The cutoff value of high and low baseline CRP level groups was ascertained according to the study-specific cutoff values. The cutoff values of CRP in all 33 studies are listed in Supplementary Table 3, and the cutoff value of most of the studies was not higher than 1 mg/dl. Among these 33 studies, 3 studies did not report the cutoff value of CRP, 11 studies used 1 mg/dl as the cutoff value, 4 studies used median CRP level as the cutoff value, while the other studies used cutoff values according to the study-specific consideration. In terms of median CRP level, 10 studies with 2,501 patients had median CRP level <1 mg/dl, and 8 studies with 1,541 patients had median CRP level >1 mg/dl (Supplementary Table 4).
When comparing the high baseline CRP level group with the low baseline CRP level group, the pooled outcomes of univariate and multivariate analyses of worse OS were 1.61 (95% CI = 1.43–1.81) and 1.83 (95% CI = 1.48–2.25), respectively (Figure 2). After sensitivity analysis, we identified that the outcomes of the studies of Chasseuil et al. (35) and Carbone et al. (41) caused significant bias in the pooled HR and 95% CI, and therefore had to be discarded. The results before and after removing the biased outcomes can be observed in Supplementary Figures 1-5. The funnel plots indicated that there existed obvious publication bias in the available data, and many unpublished negative results were likely missing (Supplementary Figures 6, 7), so we finally utilized the trim-and-fill method to obtain the corrected pooled values for HR and 95% CI of OS. The adjusted pooled value for univariate analysis outcomes is 1.48 (95% CI = 1.41–1.56), and the corrected pooled value for multivariate analysis outcomes is 1.46 (95% CI = 1.34–1.59) (Figures 3, 4). Further analysis indicated that 1 mg/dl was appropriate as the cutoff value for determining low or high level of the baseline CRP to predict the OS of cancer patients receiving ICI treatment (univariate analysis group: HR = 2.34, 95% CI = 1.77–3.08, P = 0.355; multivariate analysis group: HR = 1.92, 95% CI = 1.43–2.56, P = 0.256) (Supplementary Figure 8).
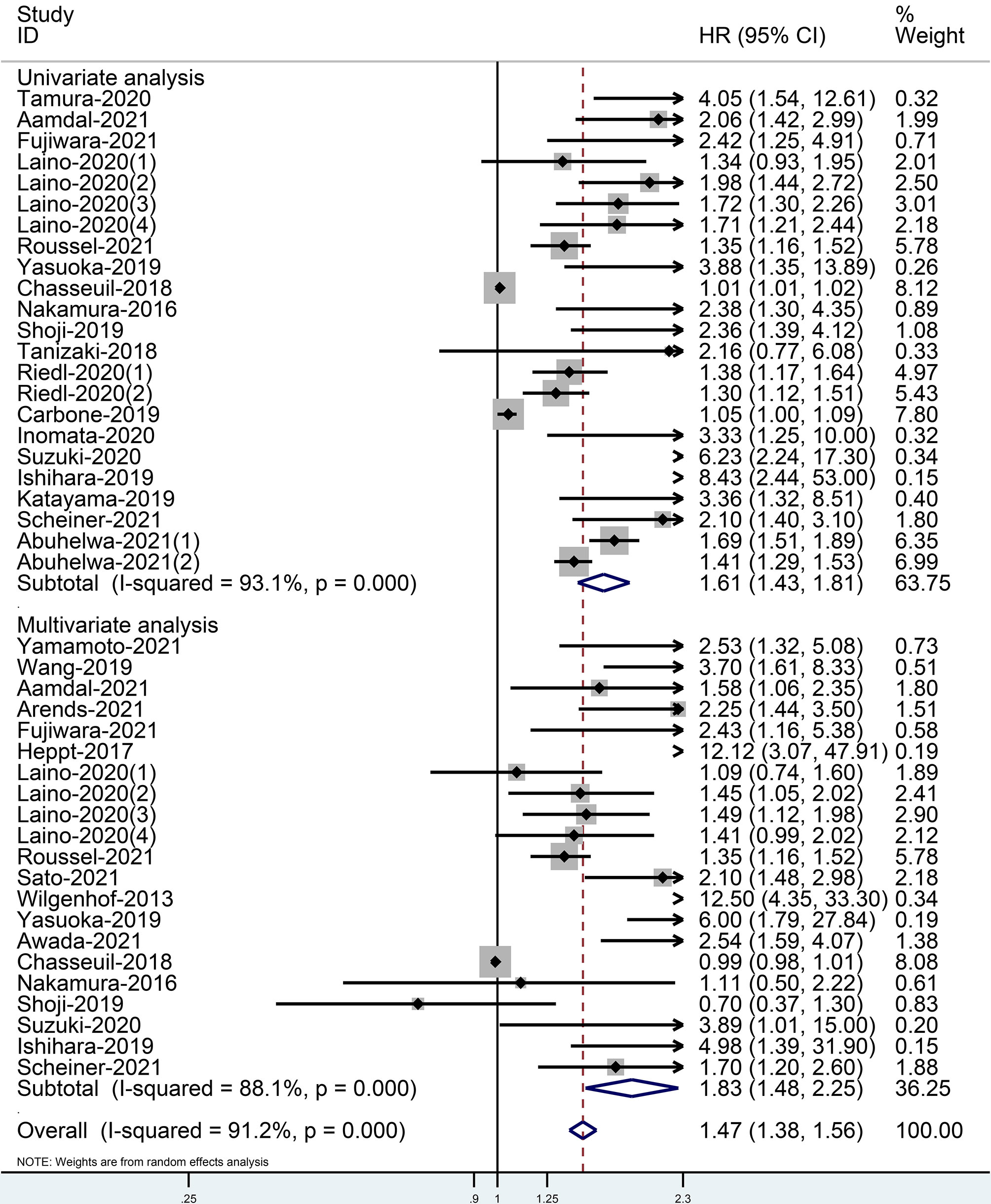
Figure 2 Forest plot of pooled HR and 95% CI about the relationship of baseline CRP levels and OS categorized by univariate and multivariate analysis outcomes in cancer patients treated with ICIs (squares = HR, horizontal lines = 95% CI, rhombus = summarized HR and its 95% CI).
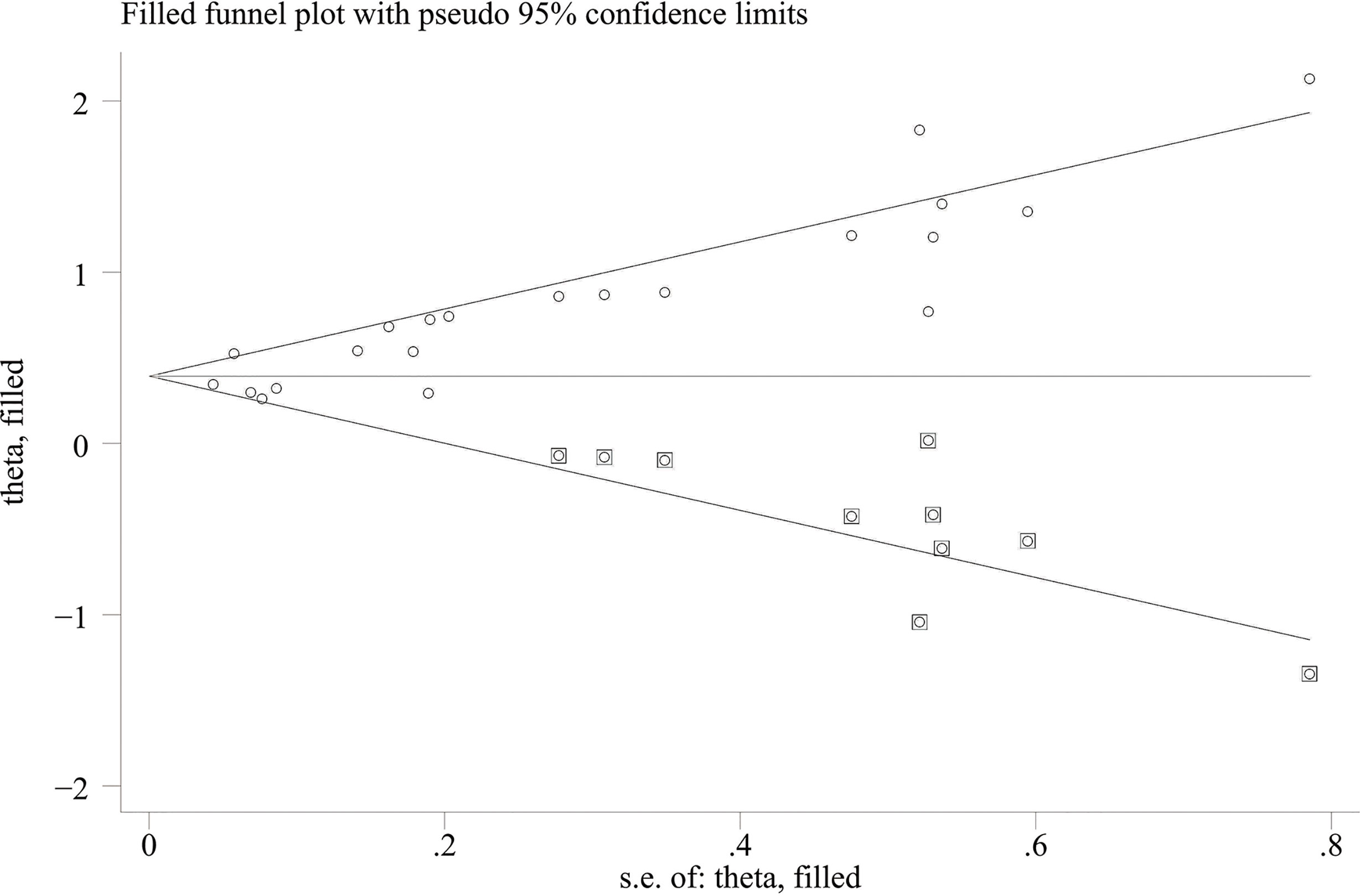
Figure 3 Trimming chart of univariate analysis outcomes of baseline CRP levels and OS [round dots = the observed studies, square dots = the missing studies imputed by the trim–and–fill method, solid lines that create a triangular area indicate the 95% CI (under the fixed–effect model), and the horizontal solid line represents the overall effect size.
Relationship Between Baseline CRP and PFS in Cancer Patients Treated With ICIs
When the high baseline CRP level group was compared with the low baseline CRP level group, the merged outcomes of univariate and multivariate analyses for worse PFS were 1.34 (95% CI = 1.15–1.55) and 1.34 (95% CI = 1.15–1.56), respectively (Figure 5). Sensitivity analysis revealed that both consequences of the study of Chasseuil et al. (35) contributed to a significant bias and, thus, must be discarded (Supplementary Figures 9-12). The funnel plot derived from the outcomes on PFS also showed plenty of missing negative results; thus, the trim-and-fill method was applied to rectify the pooled HR and 95% CI (Supplementary Figures 13, 14). The final corrected pooled outcomes for the PFS on univariate and multivariate analyses were 1.29 (95% CI = 1.15–1.45) and 1.20 (95% CI = 1.02–1.40), respectively (Figures 6, 7). Further analysis indicated that 1 mg/dl was an appropriate cutoff value for baseline CRP to predict PFS in cancer patients receiving ICI treatment (univariate analysis: HR = 1.56, 95% CI = 1.24–1.97, P = 0.909; multivariate analysis: HR = 1.58, 95% CI = 1.23–2.03, P = 0.521) (Supplementary Figure 15).
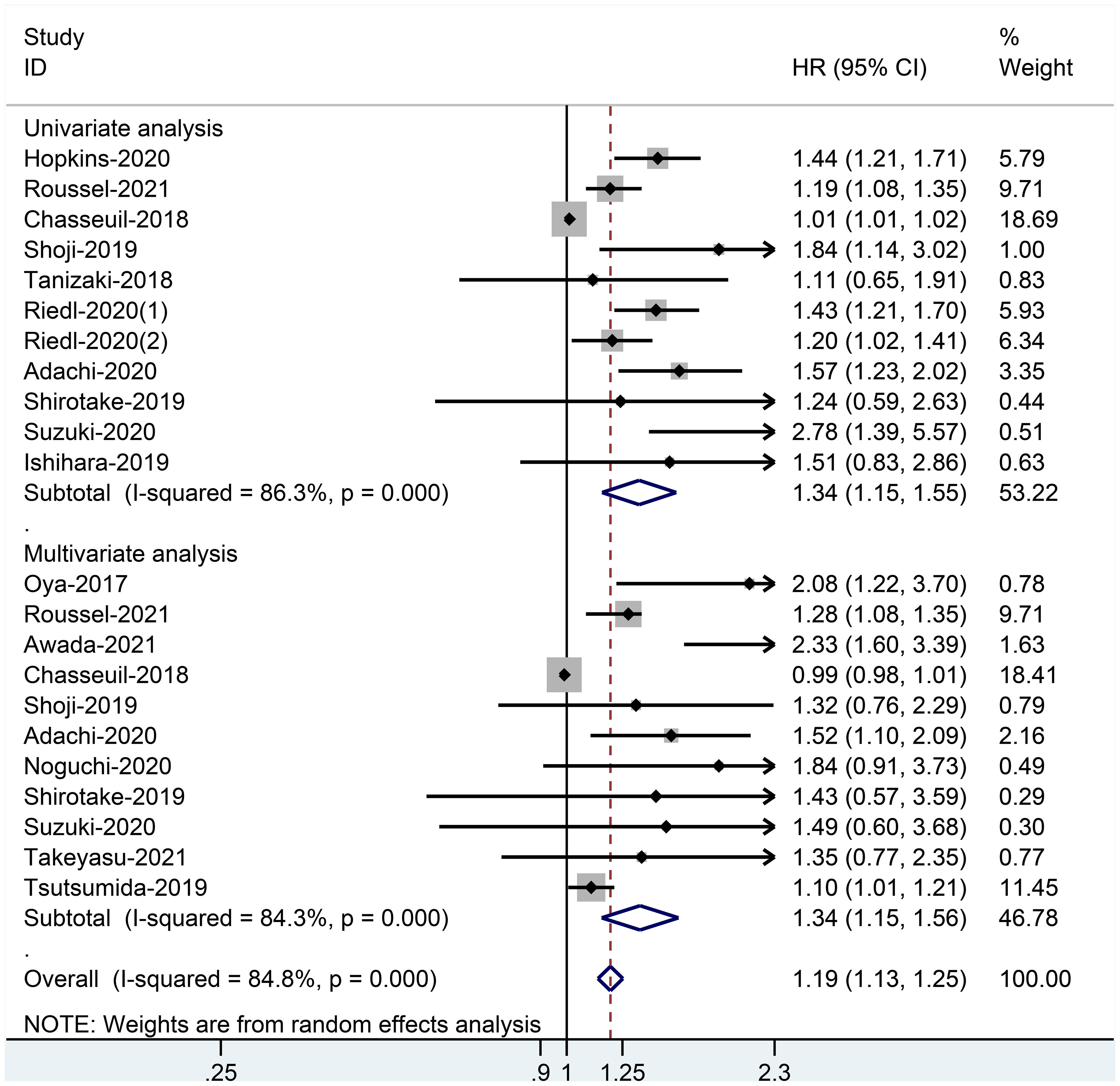
Figure 5 Forest plot of pooled HR and 95% CI about the relationship of baseline CRP levels and PFS categorized by univariate and multivariate analysis outcomes in cancer patients treated with ICIs.
Subgroup Analysis
Further OS and PFS subgroup analyses were undertaken to appraise the correlation of CPR with cancer type, sample size, country, and ICIs (Table 2). Overall, the heterogeneity of the diverse subgroups dropped significantly compared with the whole group. Outcomes of the multivariate analysis component had more credibility, while the univariate analysis section was mainly employed for reference. As far as OS is concerned, high baseline CRP level was markedly correlated with worse OS in sample size <100 group (univariate analysis group: HR = 2.99, 95% CI = 2.90–4.71, P = 0.001; multivariate analysis group: HR = 4.13, 95% CI = 2.20–7.74, P = 0.006). The OS values of subgroups stratified by cancer type, country, or ICI type were not significantly different (1 < HR < 3 in the multivariate analysis group). No significant differences were observed in PFS for all subgroups (1 < HR < 2).
Discussion
To our knowledge, this is the first meta-analysis investigating the correlation of baseline CRP level and outcomes of cancer patients treated with ICIs. The quality of the whole covered literature is generally favorable. As far as the pooled and rectified results are considered, baseline CRP level does correlate with OS and PFS in cancer patients managed with ICIs. The present studies also showed that patients with high levels of CRP or elevated CRP during treatment also had a worse OS and PFS compared with patients with low levels of CRP or decreased CRP (22, 28, 36, 46, 54, 55). Therefore, CRP is an excellent biomarker to predict the potency of ICI treatment, and more investigations are warranted to exploit the predictive value of CRP.
The clinical reference cutoff value for CRP is set as 0.5 mg/dl in some studies (40), but the cutoff value of high baseline CRP level remains controversial and various cutoff values have served in the literature. Our study permitted different cutoff values and the most popular applied cutoff value is still 1.0 mg/dl (21, 24, 29, 34, 35, 42, 43, 47, 49–51). The principal cause of the discrepancy in cutoff values is that some studies employed the median CRP level as the cutoff value, just to equalize the number of individuals in the high CRP and low CRP groups. If it is applied to the clinic, a uniform cutoff is mandatory, and 1.0 mg/dl deserves to be under consideration. We have conducted a further analysis about the median CRP level of cancer patients in all studies, and available data revealed that the majority of the patients have median CRP level <1 mg/dl. Therefore, 1 mg/dl may be a popular and suitable cutoff value for CRP. Of course, the CRP level may differ in different types of cancer, so more high–quality prospective clinical studies are warranted to determine the most suitable cutoff value of baseline CRP for different cancers.
The reason why CRP levels are closely associated with the prognosis of cancer patients receiving ICI treatment remains unclear. It may be attributed essentially to several mechanisms according to a comprehensive review of the related literature and clinical experience. It has previously been observed that CRP can directly suppress T cell (56) and dendritic cell (57), thereby influencing the effect of ICIs by impairing the intrinsic and acquired immunity of cancer patients. At the same time, CRP can promote inflammatory response (58), which will suppress immune function, and facilitate cancer multiplication and transmigration (59). CRP can also foster the formation of the tumor microenvironment (TME) (60, 61), which might impair the effect of ICIs. What is more, CRP is an indicator of body damage (62). It means that, compared with patients with low pretreatment CRP level, patients with high pretreatment CRP level perhaps have worse health and cancer circumstances, which will also affect the effectiveness of ICIs.
Subgroup analysis will inevitably decrease the number of studies, and it is more plausible that the joint outcomes would be biased. Given the magnitude of the P–value ascending, it seems that OS–related heterogeneity is strongly affected by potentially country–specific differences, which may be ethnic differences and concrete lifestyles. PFS–related heterogeneity is significantly influenced by cancer type and ICI category. It might be because of the various resistance mechanisms, proliferation, and migration rates of different cancers, and varying efficacy of specific ICIs.
Overall, CRP is an independent and desirable predictive biomarker of OS and PFS in cancer patients receiving ICI treatment, and quantifying CRP through blood examination is convenient and less invasive. The combination of CRP and other predictors to form a predictive model is also a feasible idea that merits investigation (53, 63, 64). In addition, for cancer patients with high CRP levels, the handling of inflammation and TME should be of particular attention to reduce resistance to ICIs (17). Inflammation can be addressed by causal therapy, and TME might be addressed by TME modulation that is being investigated (65). As confirmed by clinical literature, statin therapy can diminish CRP levels (66). Therefore, statin therapy might be also applied to high CRP cancer patients to improve ICI treatment. What is more, a recent study revealed that moderation–vigorous physical activity (MVPA) could reduce CRP level in breast cancer patients (67). Whether MVPA may be recommended to cancer patients with high CRP level before immunotherapy deserves further studies.
There are some limitations to be considered. First, some researchers do not publish adverse outcomes as they consider them meaningless, leading to a few unavoidable publication biases. Hence, we proceeded with the trim–and–fill method to acquire adjusted results to clarify this association. Secondly, because we only included studies published in English, we may miss some relevant studies. The present application with ICIs is predominately administered in limited types of cancers (68). The value of CRP in predicting the prognosis of breast cancer (69), prostate cancer (14), pancreatic cancer (70), colorectal cancer (71), and other cancers has been reported. However, these studies did not focus on the efficacy of ICIs. What is more, most of the selected papers were retrospective and from Japan. More prospective studies are needed to assess the predictive value of CRP for different cancer patients in different countries.
Conclusion
In summary, regardless of cancer type, high baseline CRP level is significantly correlated to worse OS and PFS in cancer patients treated with ICIs. Our study indicates that the baseline CRP level is a useful predictor and 1 mg/dl may be a suitable cutoff value to identify cancer patients who may benefit from ICI treatment, thereby helping to lead decisions in personalized treatments.
Data Availability Statement
The original contributions presented in the study are included in the article/Supplementary Material. Further inquiries can be directed to the corresponding author.
Author Contributions
All authors had full access to the data in the study and took responsibility for the integrity and authenticity of the data. C–LH formulated the study objective, conceptualized the study, performed the statistical analysis, and interpreted the results. G–XM and Z–ND designed the protocol of the systematic reviews. Z–RD, Z–QC, and J–GH provided essential guidance to the protocols and modified them. C–LH, L–JY, and HL, respectively, evaluated the availability of each study and disagreements were discussed and addressed with B–WT. L–SY and J–SX performed the methodology, data collection, and data validation. C–LH and TL guided the task of formal statistical analysis and analysis of the data. C–LH and TL contributed to outlining the manuscript and drafting the manuscript. C–LH and TL verified the underlying data. TL supervised and coordinated the study. All authors have read and approved the final version.
Funding
This work was supported by the Taishan Scholars Program for Young Expert of Shandong Province (tsqn20161064), the National Natural Science Foundation of China (81874178 and 82073200), Major Basic Research of Shandong Provincial Natural Science Foundation (Grant No. ZR202105070027), and Founds for Independent Cultivation of Innovative Team from Universities in Jinan (Grant No. 2020GXRC023).
Conflict of Interest
The authors declare that the research was conducted in the absence of any commercial or financial relationships that could be construed as a potential conflict of interest.
Publisher’s Note
All claims expressed in this article are solely those of the authors and do not necessarily represent those of their affiliated organizations, or those of the publisher, the editors and the reviewers. Any product that may be evaluated in this article, or claim that may be made by its manufacturer, is not guaranteed or endorsed by the publisher.
Supplementary Material
The Supplementary Material for this article can be found online at: https://www.frontiersin.org/articles/10.3389/fimmu.2022.827788/full#supplementary-material
Abbreviations
CRP, C–reactive protein; ICIs, immune checkpoint inhibitors; PFS, progression–free survival; OS, overall survival; PD–1, programmed cell death 1; PD–L1, programmed cell death ligand 1; CTLA–4, cytotoxic T lymphocyte–associated protein 4; PRISMA, Preferred Reporting Items for Systematic Reviews and Meta–Analyses; NOS, Newcastle–Ottawa Scale; HR, hazard ratio; 95% CI, 95% confidence interval; GPS, Glasgow prognostic score; Oct, October; No., number; NR, not reported; UC, urothelial cancer; ESCC, esophageal squamous cell carcinoma; HNSCC, head and neck squamous cell carcinoma; NSCLC, non–small cell lung cancer, RCC, renal cell carcinoma; GC, gastric cancer; SGC, salivary gland carcinoma; HCC, hepatocellular carcinoma; Pembro, pembrolizumab; Nivo, nivolumab; Ipi, ipilimumab; Camre, camrelizumab; Durva, durvalumab; Atezo, atezolizumab; TME, tumor microenvironment; MVPA, moderation–vigorous physical activity.
References
1. Ritchie G, Gasper H, Man J, Lord S, Marschner I, Friedlander M, et al. Defining the Most Appropriate Primary End Point in Phase 2 Trials of Immune Checkpoint Inhibitors for Advanced Solid Cancers: A Systematic Review and Meta–Analysis. JAMA Oncol (2018) 4(4):522–8. doi: 10.1001/jamaoncol.2017.5236
2. Bagchi S, Yuan R, Engleman EG. Immune Checkpoint Inhibitors for the Treatment of Cancer: Clinical Impact and Mechanisms of Response and Resistance. Annu Rev Pathol (2021) 16:223–49. doi: 10.1146/annurev–pathol–042020–042741
3. Hodi FS, O'Day SJ, McDermott DF, Weber RW, Sosman JA, Haanen JB, et al. Improved Survival With Ipilimumab in Patients With Metastatic Melanoma. N Engl J Med (2010) 363(8):711–23. doi: 10.1056/NEJMoa1003466
4. Borghaei H, Paz–Ares L, Horn L, Spigel DR, Steins M, Ready NE, et al. Nivolumab Versus Docetaxel in Advanced Nonsquamous Non–Small–Cell Lung Cancer. N Engl J Med (2015) 373(17):1627–39. doi: 10.1056/NEJMoa1507643
5. Motzer RJ, Tannir NM, McDermott DF, Arén Frontera O, Melichar B, Choueiri TK, et al. Nivolumab Plus Ipilimumab Versus Sunitinib in Advanced Renal–Cell Carcinoma. N Engl J Med (2018) 378(14):1277–90. doi: 10.1056/NEJMoa1712126
6. Rosenberg JE, Hoffman–Censits J, Powles T, van der Heijden MS, Balar AV, Necchi A, et al. Atezolizumab in Patients With Locally Advanced and Metastatic Urothelial Carcinoma Who Have Progressed Following Treatment With Platinum–Based Chemotherapy: A Single–Arm, Multicentre, Phase 2 Trial. Lancet (2016) 387(10031):1909–20. doi: 10.1016/s0140–6736(16)00561–4
7. Overman MJ, Lonardi S, Wong KYM, Lenz HJ, Gelsomino F, Aglietta M, et al. Durable Clinical Benefit With Nivolumab Plus Ipilimumab in DNA Mismatch Repair–Deficient/Microsatellite Instability–High Metastatic Colorectal Cancer. J Clin Oncol (2018) 36(8):773–9. doi: 10.1200/jco.2017.76.9901
8. Topalian SL, Hodi FS, Brahmer JR, Gettinger SN, Smith DC, McDermott DF, et al. Safety, Activity, and Immune Correlates of Anti–PD–1 Antibody in Cancer. N Engl J Med (2012) 366(26):2443–54. doi: 10.1056/NEJMoa1200690
9. Seliger B. The Role of the Lymphocyte Functional Crosstalk and Regulation in the Context of Checkpoint Inhibitor Treatment–Review. Front Immunol (2019) 10:2043. doi: 10.3389/fimmu.2019.02043
10. Lesokhin AM, Ansell SM, Armand P, Scott EC, Halwani A, Gutierrez M, et al. Nivolumab in Patients With Relapsed or Refractory Hematologic Malignancy: Preliminary Results of a Phase Ib Study. J Clin Oncol (2016) 34(23):2698–704. doi: 10.1200/jco.2015.65.9789
11. Morou A, Brunet–Ratnasingham E, Dubé M, Charlebois R, Mercier E, Darko S, et al. Altered Differentiation is Central to HIV–Specific CD4(+) T Cell Dysfunction in Progressive Disease. Nat Immunol (2019) 20(8):1059–70. doi: 10.1038/s41590–019–0418–x
12. Tillett WS, Francis T. Serological Reactions in Pneumonia With a Non–Protein Somatic Fraction of Pneumococcus. J Exp Med (1930) 52(4):561–71. doi: 10.1084/jem.52.4.561
13. Zhou L, Cai X, Liu Q, Jian ZY, Li H, Wang KJ. Prognostic Role of C–Reactive Protein In Urological Cancers: A Meta–Analysis. Sci Rep (2015) 5:12733. doi: 10.1038/srep12733
14. Liu ZQ, Chu L, Fang JM, Zhang X, Zhao HX, Chen YJ, et al. Prognostic Role of C–Reactive Protein in Prostate Cancer: A Systematic Review and Meta–Analysis. Asian J Androl (2014) 16(3):467–71. doi: 10.4103/1008–682x.123686
15. Wang X, Liu S, Zhao X, Fang E, Zhao X. The Value of C–Reactive Protein as an Independent Prognostic Indicator for Disease–Specific Survival in Patients With Soft Tissue Sarcoma: A Meta–Analysis. PLoS One (2019) 14(7):e0219215. doi: 10.1371/journal.pone.0219215
16. Chen Y, Cong R, Ji C, Ruan W. The Prognostic Role of C–Reactive Protein in Patients With Head and Neck Squamous Cell Carcinoma: A Meta–Analysis. Cancer Med (2020) 9(24):9541–53. doi: 10.1002/cam4.3520
17. Jimenez RV, Szalai AJ. Therapeutic Lowering of C–Reactive Protein. Front Immunol (2020) 11:619564. doi: 10.3389/fimmu.2020.619564
18. Moher D, Liberati A, Tetzlaff J, Altman DG. Preferred Reporting Items for Systematic Reviews and Meta–Analyses: The PRISMA Statement. PLoS Med (2009) 6(7):e1000097. doi: 10.1371/journal.pmed.1000097
19. Stang A. Critical Evaluation of the Newcastle–Ottawa Scale for the Assessment of the Quality of Nonrandomized Studies in Meta–Analyses. Eur J Epidemiol (2010) 25(9):603–5. doi: 10.1007/s10654–010–9491–z
20. Sterne JA, Egger M. Funnel Plots for Detecting Bias in Meta–Analysis: Guidelines on Choice of Axis. J Clin Epidemiol (2001) 54(10):1046–55. doi: 10.1016/s0895–4356(01)00377–8
21. Yamamoto Y, Yatsuda J, Shimokawa M, Fuji N, Aoki A, Sakano S, et al. Prognostic Value of Pre–Treatment Risk Stratification and Post–Treatment Neutrophil/Lymphocyte Ratio Change for Pembrolizumab in Patients With Advanced Urothelial Carcinoma. Int J Clin Oncol (2021) 26(1):169–77. doi: 10.1007/s10147–020–01784–w
22. Tamura D, Jinnouchi N, Abe M, Ikarashi D, Matsuura T, Kato R, et al. Prognostic Outcomes and Safety in Patients Treated With Pembrolizumab for Advanced Urothelial Carcinoma: Experience in Real–World Clinical Practice. Int J Clin Oncol (2020) 25(5):899–905. doi: 10.1007/s10147–019–01613–9
23. Wang X, Zhang B, Chen X, Mo H, Wu D, Lan B, et al. Lactate Dehydrogenase and Baseline Markers Associated With Clinical Outcomes of Advanced Esophageal Squamous Cell Carcinoma Patients Treated With Camrelizumab (SHR–1210), a Novel Anti–PD–1 Antibody. Thorac Cancer (2019) 10(6):1395–401. doi: 10.1111/1759–7714.13083
24. Aamdal E, Jacobsen KD, Straume O, Kersten C, Herlofsen O, Karlsen J, et al. Ipilimumab in a Real–World Population: A Prospective Phase IV Trial With Long–Term Follow–Up. Int J Cancer (2022) 150(1):100–11. doi: 10.1002/ijc.33768
25. Arends R, Guo X, Baverel PG, González–García I, Xie J, Morsli N, et al. Association of Circulating Protein Biomarkers With Clinical Outcomes of Durvalumab in Head and Neck Squamous Cell Carcinoma. Oncoimmunology (2021) 10(1):1898104. doi: 10.1080/2162402x.2021.1898104
26. Fujiwara M, Yuasa T, Urasaki T, Komai Y, Fujiwara R, Numao N, et al. Effectiveness and Safety Profile of Pembrolizumab for Metastatic Urothelial Cancer: A Retrospective Single–Center Analysis in Japan. Cancer Rep (Hoboken) (2021) 4(6):e1398. doi: 10.1002/cnr2.1398
27. Heppt MV, Heinzerling L, Kähler KC, Forschner A, Kirchberger MC, Loquai C, et al. Prognostic Factors and Outcomes in Metastatic Uveal Melanoma Treated With Programmed Cell Death–1 or Combined PD–1/Cytotoxic T–Lymphocyte Antigen–4 Inhibition. Eur J Cancer (2017) 82:56–65. doi: 10.1016/j.ejca.2017.05.038
28. Laino AS, Woods D, Vassallo M, Qian X, Tang H, Wind–Rotolo M, et al. Serum Interleukin–6 and C–Reactive Protein are Associated With Survival in Melanoma Patients Receiving Immune Checkpoint Inhibition. J Immunother Cancer (2020) 8(1):e000842. doi: 10.1136/jitc–2020–000842
29. Oya Y, Yoshida T, Kuroda H, Mikubo M, Kondo C, Shimizu J, et al. Predictive Clinical Parameters for the Response of Nivolumab in Pretreated Advanced non–Small–Cell Lung Cancer. Oncotarget (2017) 8(61):103117–28. doi: 10.18632/oncotarget.21602
30. Roussel E, Kinget L, Verbiest A, Debruyne PR, Baldewijns M, Van Poppel H, et al. C–Reactive Protein and Neutrophil–Lymphocyte Ratio are Prognostic in Metastatic Clear–Cell Renal Cell Carcinoma Patients Treated With Nivolumab. Urol Oncol (2021) 39(4):239.e217–39.e225. doi: 10.1016/j.urolonc.2020.12.020
31. Sato S, Oshima Y, Matsumoto Y, Seto Y, Yamashita H, Hayano K, et al. The New Prognostic Score for Unresectable or Recurrent Gastric Cancer Treated With Nivolumab: A Multi–Institutional Cohort Study. Ann Gastroenterol Surg (2021) 5(6):794–803. doi: 10.1002/ags3.12489
32. Wilgenhof S, Four SD, Vandenbroucke F, Everaert H, Salmon I, Liénard D, et al. Single–Center Experience With Ipilimumab in an Expanded Access Program for Patients With Pretreated Advanced Melanoma. J Immunother (2013) 36(3):215–22. doi: 10.1097/CJI.0b013e31828eed39
33. Yasuoka S, Yuasa T, Nishimura N, Ogawa M, Komai Y, Numao N, et al. Initial Experience of Pembrolizumab Therapy in Japanese Patients With Metastatic Urothelial Cancer. Anticancer Res (2019) 39(7):3887–92. doi: 10.21873/anticanres.13539
34. Awada G, Jansen Y, Schwarze JK, Tijtgat J, Hellinckx L, Gondry O, et al. A Comprehensive Analysis of Baseline Clinical Characteristics and Biomarkers Associated With Outcome in Advanced Melanoma Patients Treated With Pembrolizumab. Cancers (Basel) (2021) 13(2):168. doi: 10.3390/cancers13020168
35. Chasseuil E, Saint–Jean M, Chasseuil H, Peuvrel L, Quéreux G, Nguyen JM, et al. Blood Predictive Biomarkers for Nivolumab in Advanced Melanoma. Acta Derm Venereol (2018) 98(4):406–10. doi: 10.2340/00015555–2872
36. Nakamura Y, Kitano S, Takahashi A, Tsutsumida A, Namikawa K, Tanese K, et al. Nivolumab for Advanced Melanoma: Pretreatment Prognostic Factors and Early Outcome Markers During Therapy. Oncotarget (2016) 7(47):77404–15. doi: 10.18632/oncotarget.12677
37. Niwa K, Kawakita D, Nagao T, Takahashi H, Saotome T, Okazaki M, et al. Multicentre, Retrospective Study of the Efficacy and Safety of Nivolumab for Recurrent and Metastatic Salivary Gland Carcinoma. Sci Rep (2020) 10(1):16988. doi: 10.1038/s41598–020–73965–6
38. Shoji F, Takeoka H, Kozuma Y, Toyokawa G, Yamazaki K, Ichiki M, et al. Pretreatment Prognostic Nutritional Index as a Novel Biomarker in non–Small Cell Lung Cancer Patients Treated With Immune Checkpoint Inhibitors. Lung Cancer (2019) 136:45–51. doi: 10.1016/j.lungcan.2019.08.006
39. Tanizaki J, Haratani K, Hayashi H, Chiba Y, Nakamura Y, Yonesaka K, et al. Peripheral Blood Biomarkers Associated With Clinical Outcome in Non–Small Cell Lung Cancer Patients Treated With Nivolumab. J Thorac Oncol (2018) 13(1):97–105. doi: 10.1016/j.jtho.2017.10.030
40. Riedl JM, Barth DA, Brueckl WM, Zeitler G, Foris V, Mollnar S, et al. C–Reactive Protein (CRP) Levels in Immune Checkpoint Inhibitor Response and Progression in Advanced Non–Small Cell Lung Cancer: A Bi–Center Study. Cancers (Basel) (2020) 12(8):2319. doi: 10.3390/cancers12082319
41. Carbone F, Grossi F, Bonaventura A, Vecchié A, Minetti S, Bardi N, et al. Baseline Serum Levels of Osteopontin Predict Clinical Response to Treatment With Nivolumab in Patients With non–Small Cell Lung Cancer. Clin Exp Metastasis (2019) 36(5):449–56. doi: 10.1007/s10585–019–09984–z
42. Adachi Y, Tamiya A, Taniguchi Y, Enomoto T, Azuma K, Kouno S, et al. Predictive Factors for Progression–Free Survival in non–Small Cell Lung Cancer Patients Receiving Nivolumab Based on Performance Status. Cancer Med (2020) 9(4):1383–91. doi: 10.1002/cam4.2807
43. Inomata M, Hirai T, Seto Z, Tokui K, Taka C, Okazawa S, et al. Clinical Parameters for Predicting the Survival in Patients With Squamous and Non–Squamous–Cell NSCLC Receiving PD–1 Inhibitor Therapy. Pathol Oncol Res (2020) 26(1):327–33. doi: 10.1007/s12253–018–0473–x
44. Noguchi G, Nakaigawa N, Umemoto S, Kobayashi K, Shibata Y, Tsutsumi S, et al. C–Reactive Protein at 1 Month After Treatment of Nivolumab as a Predictive Marker of Efficacy in Advanced Renal Cell Carcinoma. Cancer Chemother Pharmacol (2020) 86(1):75–85. doi: 10.1007/s00280–020–04088–y
45. Shirotake S, Takamatsu K, Mizuno R, Kaneko G, Nishimoto K, Oya M, et al. Serum Lactate Dehydrogenase Before Nivolumab Treatment Could be a Therapeutic Prognostic Biomarker for Patients With Metastatic Clear Cell Renal Cell Carcinoma. Anticancer Res (2019) 39(8):4371–7. doi: 10.21873/anticanres.13606
46. Suzuki K, Terakawa T, Furukawa J, Harada K, Hinata N, Nakano Y, et al. C–Reactive Protein and the Neutrophil–to–Lymphocyte Ratio are Prognostic Biomarkers in Metastatic Renal Cell Carcinoma Patients Treated With Nivolumab. Int J Clin Oncol (2020) 25(1):135–44. doi: 10.1007/s10147–019–01528–5
47. Takeyasu Y, Yoshida T, Shibaki R, Matsumoto Y, Goto Y, Kanda S, et al. Differential Efficacy of Pembrolizumab According to Metastatic Sites in Patients With PD–L1 Strongly Positive (TPS ≥ 50%) NSCLC. Clin Lung Cancer (2021) 22(2):127–33.e123. doi: 10.1016/j.cllc.2020.10.002
48. Tsutsumida A, Fukushima S, Yokota K, Yoshikawa S, Yamasaki O, Tanemura A, et al. Japanese Real–World Study of Sequential Nivolumab and Ipilimumab Treament in Melanoma. J Dermatol (2019) 46(11):947–55. doi: 10.1111/1346–8138.15073
49. Ishihara H, Tachibana H, Takagi T, Kondo T, Fukuda H, Yoshida K, et al. Predictive Impact of Peripheral Blood Markers and C–Reactive Protein in Nivolumab Therapy for Metastatic Renal Cell Carcinoma. Targeting Oncol (2019) 14(4):453–63. doi: 10.1007/s11523–019–00660–6
50. Katayama Y, Yamada T, Tanimura K, Yoshimura A, Takeda T, Chihara Y, et al. Impact of Bowel Movement Condition on Immune Checkpoint Inhibitor Efficacy in Patients With Advanced non–Small Cell Lung Cancer. Thorac Cancer (2019) 10(3):526–32. doi: 10.1111/1759–7714.12969
51. Scheiner B, Pomej K, Kirstein MM, Hucke F, Finkelmeier F, Waidmann O, et al. Prognosis of Patients With Hepatocellular Carcinoma Treated With Immunotherapy – Development and Validation of the CRAFITY Score. J Hepatol (2022) 76(2):353–63. doi: 10.1016/j.jhep.2021.09.035
52. Abuhelwa AY, Bellmunt J, Kichenadasse G, McKinnon RA, Rowland A, Sorich MJ, et al. Enhanced Bellmunt Risk Score for Survival Prediction in Urothelial Carcinoma Treated With Immunotherapy. Clin Genitourin Cancer (2021). doi: 10.1016/j.clgc.2021.11.010
53. Hopkins AM, Kichenadasse G, Garrett–Mayer E, Karapetis CS, Rowland A, Sorich MJ. Development and Validation of a Prognostic Model for Patients With Advanced Lung Cancer Treated With the Immune Checkpoint Inhibitor Atezolizumab. Clin Cancer Res (2020) 26(13):3280–6. doi: 10.1158/1078–0432.Ccr–19–2968
54. Ishihara H, Takagi T, Kondo T, Fukuda H, Tachibana H, Yoshida K, et al. Predictive Impact of an Early Change in Serum C–Reactive Protein Levels in Nivolumab Therapy for Metastatic Renal Cell Carcinoma. Urol Oncol (2020) 38(5):526–32. doi: 10.1016/j.urolonc.2019.12.008
55. Takamatsu K, Mizuno R, Baba Y, Yasumizu Y, Tanaka N, Shirotake S, et al. On–Treatment C–Reactive Protein Control Could Predict Response to Subsequent Anti–PD–1 Treatment in Metastatic Renal Cell Carcinoma. Int J Clin Oncol (2021) 26(8):1500–5. doi: 10.1007/s10147–021–01930–y
56. Yoshida T, Ichikawa J, Giuroiu I, Laino AS, Hao Y, Krogsgaard M, et al. C Reactive Protein Impairs Adaptive Immunity in Immune Cells of Patients With Melanoma. J Immunother Cancer (2020) 8(1):e000234. doi: 10.1136/jitc–2019–000234
57. Bilen MA, Martini DJ, Liu Y, Lewis C, Collins HH, Shabto JM, et al. The Prognostic and Predictive Impact of Inflammatory Biomarkers in Patients Who Have Advanced–Stage Cancer Treated With Immunotherapy. Cancer (2019) 125(1):127–34. doi: 10.1002/cncr.31778
58. Sproston NR, Ashworth JJ. Role of C–Reactive Protein at Sites of Inflammation and Infection. Front Immunol (2018) 9:754. doi: 10.3389/fimmu.2018.00754
59. Whiteside TL. The Tumor Microenvironment and its Role in Promoting Tumor Growth. Oncogene (2008) 27(45):5904–12. doi: 10.1038/onc.2008.271
60. Greten FR, Grivennikov SI. Inflammation and Cancer: Triggers, Mechanisms, and Consequences. Immunity (2019) 51(1):27–41. doi: 10.1016/j.immuni.2019.06.025
61. Hart PC, Rajab IM, Alebraheem M, Potempa L. A. C–Reactive Protein and Cancer–Diagnostic and Therapeutic Insights. Front Immunol (2020) 11:595835. doi: 10.3389/fimmu.2020.595835
62. Potempa LA, Rajab IM, Olson ME, Hart PC. C–Reactive Protein and Cancer: Interpreting the Differential Bioactivities of Its Pentameric and Monomeric, Modified Isoforms. Front Immunol (2021) 12:744129. doi: 10.3389/fimmu.2021.744129
63. Takamori S, Takada K, Shimokawa M, Matsubara T, Fujishita T, Ito K, et al. Clinical Utility of Pretreatment Glasgow Prognostic Score in non–Small–Cell Lung Cancer Patients Treated With Immune Checkpoint Inhibitors. Lung Cancer (2021) 152:27–33. doi: 10.1016/j.lungcan.2020.11.026
64. Brown JT, Liu Y, Shabto JM, Martini DJ, Ravindranathan D, Hitron EE, et al. Baseline Modified Glasgow Prognostic Score Associated With Survival in Metastatic Urothelial Carcinoma Treated With Immune Checkpoint Inhibitors. Oncologist (2021) 26(5):397–405. doi: 10.1002/onco.13727
65. Casey SC, Amedei A, Aquilano K, Azmi AS, Benencia F, Bhakta D, et al. Cancer Prevention and Therapy Through the Modulation of the Tumor Microenvironment. Semin Cancer Biol (2015) 35 (Suppl):S199–s223. doi: 10.1016/j.semcancer.2015.02.007
66. Albert MA, Danielson E, Rifai N, Ridker PM, Investigators, P. Effect of Statin Therapy on C–Reactive Protein Levels – The Pravastatin Inflammation/CRP Evaluation (PRINCE): A Randomized Trial and Cohort Study. Jama–Journal Am Med Assoc (2001) 286(1):64–70. doi: 10.1001/jama.286.1.64
67. Sabiston CM, Wrosch C, Castonguay AL, Sylvester BD. Changes in Physical Activity Behavior and C–Reactive Protein in Breast Cancer Patients. Ann Behav Med (2018) 52(7):545–51. doi: 10.1093/abm/kax010
68. Stephen ZR, Zhang M. Recent Progress in the Synergistic Combination of Nanoparticle–Mediated Hyperthermia and Immunotherapy for Treatment of Cancer. Adv Healthc Mater (2021) 10(2):e2001415. doi: 10.1002/adhm.202001415
69. Han Y, Mao F, Wu Y, Fu X, Zhu X, Zhou S, et al. Prognostic Role of C–Reactive Protein in Breast Cancer: A Systematic Review and Meta–Analysis. Int J Biol Markers (2011) 26(4):209–15. doi: 10.5301/jbm.2011.8872
70. Stevens L, Pathak S, Nunes QM, Pandanaboyana S, Macutkiewicz C, Smart N, et al. Prognostic Significance of Pre–Operative C–Reactive Protein and the Neutrophil–Lymphocyte Ratio in Resectable Pancreatic Cancer: A Systematic Review. HPB (Oxford) (2015) 17(4):285–91. doi: 10.1111/hpb.12355
Keywords: predictive potential, C-reactive protein, prognosis, immune checkpoint inhibitors, cancer, meta-analysis
Citation: Han C-L, Meng G-X, Ding Z-N, Dong Z-R, Chen Z-Q, Hong J-G, Yan L-J, Liu H, Tian B-W, Yang L-S, Xue J-S and Li T (2022) The Predictive Potential of the Baseline C-Reactive Protein Levels for the Efficiency of Immune Checkpoint Inhibitors in Cancer Patients: A Systematic Review and Meta-Analysis. Front. Immunol. 13:827788. doi: 10.3389/fimmu.2022.827788
Received: 02 December 2021; Accepted: 14 January 2022;
Published: 08 February 2022.
Edited by:
Shyamasree Ghosh, National Institute of Science Education and Research (NISER), IndiaReviewed by:
Laercio Lopes DaSilva, MetroWest Medical Center, United StatesWaliza Ansar, Behala College, India
Copyright © 2022 Han, Meng, Ding, Dong, Chen, Hong, Yan, Liu, Tian, Yang, Xue and Li. This is an open-access article distributed under the terms of the Creative Commons Attribution License (CC BY). The use, distribution or reproduction in other forums is permitted, provided the original author(s) and the copyright owner(s) are credited and that the original publication in this journal is cited, in accordance with accepted academic practice. No use, distribution or reproduction is permitted which does not comply with these terms.
*Correspondence: Tao Li, litao7706@163.com