Genome-Wide DNA Methylation Analysis Reveals a Conserved Epigenetic Response to Seasonal Environmental Variation in the Staghorn Coral Acropora cervicornis
- 1Environmental Epigenetics Laboratory, Institute of Environment, Florida International University, Miami, FL, United States
- 2Sociedad Ambiente Marino, San Juan, PR, United States
- 3Genomes and Disease, Centre for Research in Molecular Medicine and Chronic Diseases (CIMUS), Universidad de Santiago de Compostela – IDIS, Santiago de Compostela, Spain
- 4Department of Biology, University of Puerto Rico, San Juan, PR, United States
- 5Seagrass Ecosystems Research Laboratory, Coastlines and Oceans Division, Institute of Environment, Department of Biological Sciences, Florida International University, Miami, FL, United States
- 6Department of Biology, Pennsylvania State University, State College, University Park, PA, United States
Epigenetic modifications such as DNA methylation have been shown to participate in plastic responses to environmental change in a wide range of organisms, including scleractinian corals. Unfortunately, the current understanding of the links between environmental signals, epigenetic modifications, and the subsequent consequences for acclimatory phenotypic changes remain obscure. Such a knowledge gap extends also to the dynamic nature of epigenetic changes, hampering our ability to ascertain the magnitude and extent of these responses under natural conditions. The present work aims to shed light on these subjects by examining temporal changes in genome-wide patterns of DNA methylation in the staghorn coral Acropora cervicornis in the island of Culebra, PR. During a 17-month period, a total of 162 polymorphic loci were identified using Methylation-Sensitive Amplified Polymorphism (MSAP). Among them, 83 of these restriction fragments displayed changes in DNA methylation that were significantly correlated to seasonal variation as determined mostly by changes in sea water temperature. Remarkably, the observed time-dependent variation in DNA methylation patterns is consistent across coral genets, coral source sites and site-specific conditions studied. Overall, these results are consistent with a conserved epigenetic response to seasonal environmental variation. These findings highlight the importance of including seasonal variability into experimental designs investigating the role of epigenetic mechanisms such as DNA methylation in responses to stress.
Introduction
Hermatypic (i.e., reef-building) corals play a critical role as ecosystem foundation species. Hence, it is not surprising that continuous reductions in their populations for the last 30 years have caused the collapse of many coral reef ecosystems worldwide, and a drastic deterioration in the ones still remaining (Pandolfi et al., 2003; Hoegh-Guldberg et al., 2007; Birkeland, 2019). Among the different potential drivers for this decrease, the increase in average temperature in the upper layers of the ocean (Hansen et al., 2012; Abraham et al., 2013) and changes in ocean chemistry (Feely et al., 2009) caused by human-driven global change (Rosenzweig et al., 2008; Hoegh-Guldberg and Bruno, 2010) are considered among the most important factors. It is well known that corals are particularly sensitive to water temperature fluctuations (Cai et al., 2016; Hume et al., 2016), with current conditions provoking frequent bleaching events in reefs worldwide when temperature increases 1–2°C above normal summer maximum (Hoegh-Guldberg, 1999; Hughes et al., 2003). This susceptibility, along with the fast-paced progression of global change has generated concerns about the ability of corals to acclimatize and adapt to these conditions.
Temperature and light represent the main environmental factors responsible for the collapse (i.e., bleaching) of the coral holobiont (the unit formed by the symbiosis between the coral animal and its associated microorganisms, including dinoflagellate algae of the family Symbiodinaceae). In addition, seasonal changes in these parameters also drive subsequent variation in coral physiology (Scheufen et al., 2017). Contrary to the case of random environmental variation, the predictability of seasonal fluctuations can be conducive to the development and inheritance of plastic transcriptional profiles mediating phenotypic responses, regulated by epigenetic mechanisms [e.g., seasonal DNA methylation changes in bivalve mollusks (Suarez-Ulloa et al., 2019), plants (Ito et al., 2019) and birds (Viitaniemi et al., 2019)]. Indeed, seasonality produces dramatic physiological adjustments in corals, including changes in symbiont’s abundance and pigmentation (Fitt et al., 2000; Thornhill et al., 2006), modifications of microbial community composition (Sharp et al., 2017), as well as the alteration of transcriptional profiles (Edge et al., 2008; Brady et al., 2011; Parkinson et al., 2018; Brener-Raffalli et al., 2019). On one hand, the different sensitivities to heat stress and bleaching displayed by winter and summer coral phenotypes (Berkelmans and Willis, 1999; Scheufen et al., 2017) seem to support the notion that these changes could prepare corals to respond to increased temperature and light stress during the summer months. On the other hand, recent experiments in A. cervicornis have failed to find additional support for this idea (Parkinson et al., 2018). Nevertheless, even if these adjustments were to occur, they may fall short when facing altered seasonal regimes and unprecedented stress events caused by global change. Consequently, understanding the shared mechanisms underlying thermal and seasonal acclimatization in corals will improve our capacity to model coral population trajectories, and enhance coral preconditioning and assisted evolution approaches (van Oppen et al., 2015).
As sessile organisms, corals rely exclusively on phenotypic plasticity to respond to their environment (López-Maury et al., 2008), a response that is largely mediated by the interaction between the coral’s genome and intrinsic and extrinsic environmental signals modulating its expression (West-Eberhard, 2003). Although the role of this plasticity is mostly observed during the life of an organism (IntraGenerational Plasticity, IGP), it has been suggested that parents can “prime” their offspring to better respond to changes in their specific environments (InterGenerational Plasticity, ItGP) (e.g., thermal response in fishes; Salinas and Munch, 2012) and even produce phenotypes that will persist for generations even in the absence of the initial stressor triggering that phenotype (TransGenerational Plasticity TGP) (Perez and Lehner, 2019). Based on the current evidence for the inheritance of acquired epigenetic marks, it seems plausible that epigenetic mechanisms play a critical role providing a mechanistic framework for the acquisition and intergenerational inheritance of phenotypes optimized to the prevailing environmental conditions (Vandegehuchte et al., 2009; Navarro-Martín et al., 2011; Marsh and Pasqualone, 2014; Vignet et al., 2015; Eirin-Lopez and Putnam, 2019), increasing the resilience and resistance of corals to global change. However, in order to disentangle the role of epigenetic mechanisms on IGP, ItGP, and TGP, there is an urgent need to better understand how these epigenetic mechanisms interact with environmental factors.
Epigenetic mechanisms display extremely dynamic responses to environmental changes (Cortessis et al., 2012), serving as a “sensory” interface between the environmental condition and the genome function. Therefore, understanding exposure-response relationships of these molecular mechanisms could potentially allow the quantification of the effects of the environment on phenotypic variation (Cortessis et al., 2012; Suarez-Ulloa et al., 2015; Etchegaray and Mostoslavsky, 2016), increasing our capacity to predict population responses after environmental change. While increasing evidence points to a relevant role of DNA methylation and other epigenetic mechanisms in plastic responses to environmental change in corals (Putnam et al., 2016; Dixon et al., 2018; Liew et al., 2018; Dimond and Roberts, 2020) and other marine organisms (Ryu et al., 2018; Eirin-Lopez and Putnam, 2019), there is limited understanding of the factors influencing dynamic epigenetic changes under non-stressed conditions, confounding the ability to determine the magnitude and extent of epigenetic responses under natural conditions (Suarez-Ulloa et al., 2019). In addition, solid baseline data of “natural response” to seasonal and diel cycles in most ecologically important organisms is lacking (Suarez-Ulloa et al., 2019). This gap can be bridged by developing seasonal monitoring of coral epigenetic signatures, helping disentangle the molecular underpinnings of such epigenetic responses, their involvement in seasonal acclimatization, and their capacity to respond to factors driving global change in the Anthropocene. The present work aims to do so by characterizing temporal changes in DNA methylation patterns using the staghorn coral A. cervicornis as model system.
Methods
Study Site, Experimental and Sampling Design
A total of n = 200 staghorn coral (Acropora cervicornis) fragments (naturally generated by hurricanes Irma and Maria between August and October 2017) were collected from 4 reefs around Culebra Island, Puerto Rico (Figure 1). Naturally occurring fragments were used to minimize the effect of sampling on standing colonies of A. cervicornis. Therefore, sampling effort was not standardized among sites, and analyses on genotypic diversity of the sample pool cannot be extrapolated to compare natural levels of sexual recruitment among sites. Fragments were stabilized by immediate outplanting into two natural reefs located in the Canal Luis Peña No-Take Marine Reserve: Luis Peña (LP: 18°18′45.0″N, 65°20′08.4″W) and Carlos Rosario (CR: 18°19′30.2″N, 65°19′52.7″W) reefs. At the time of outplanting, genotyping information was not available. Thus, in order to homogenize the distribution of putative genets and avoid biases from local adaptation in the site-specific response, fragments from different sources were further subdivided before fixing them to the substrate using nails and plastic ties at two different depths (5 and 15 m). This yielded an equal representation of putative genets at both depths. The outplanting sites were named LP shallow (LPs), LP deep (LPd), CR shallow (CRs), and CR deep (CRd). Coral fragments were organized into 5 × 5 m plots containing 20 fragments per plot, for a total of 5 plots per site (n = 100 fragments per site, total = 400 fragments outplanted). The size of outplanted fragments ranged between 10 and 30 cm in length.
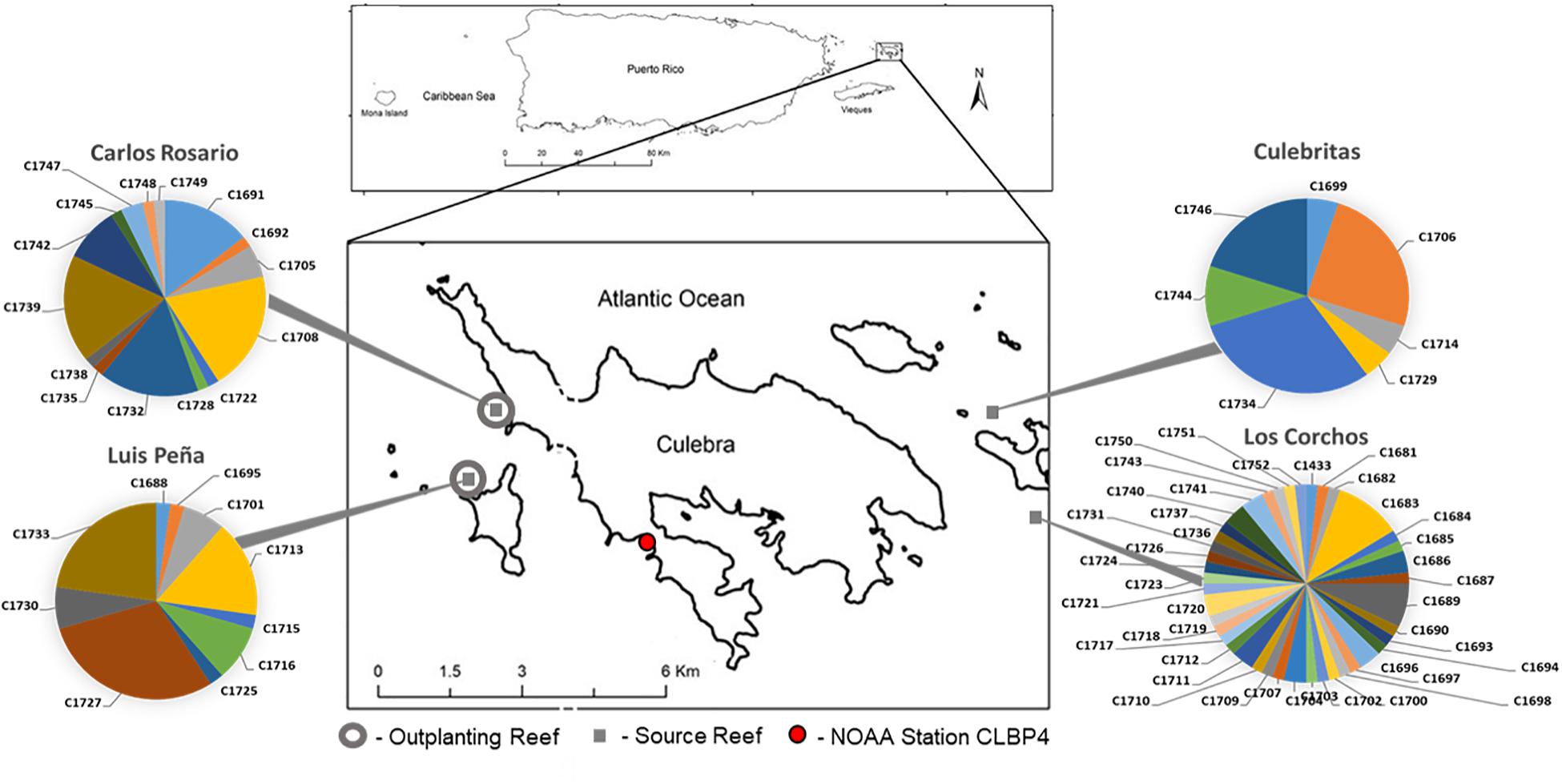
Figure 1. Field experiment locations in Culebra, Puerto Rico. Four source sites are denoted with gray squares, Luis Peña (LP: 18°18′45.0″N, 65°20′08.4″W), Carlos Rosario (CR: 18°19′30.2″N, 65°19′52.7″W), Culebritas (18°19′19.4″N 65°14′14.5″W) and Los Corchos (18°18′33.0″N 65°13′44.3″W). The genotypic composition was obtained using 6 microsatellite loci as in Baums et al. (2005a) and is shown in pie charts. Outplant sites (gray circles) consisted of five 5 m × 5 m plots located at each site at two depths (5 and 15 m). A total of n = 20 fragments from each source site were outplanted in each plot for a total of n = 400 fragments. Temperature records were gathered from NOAA Data Buoy Center, Station CLBP4 (red circle) located 3.8 and 4 km from LP and CR respectively.
The characterization of depth-associated changes in dissolved oxygen, pH, salinity and pressure (tides) was performed by deploying YSI EXO2 multiparameter sondes (YSI, Yellow Springs, OH, United States) and photosynthetically active radiation (PAR) sensors (Sea Bird, Bellevue, WA) at the two studied depths in Luis Peña reef. Sensors were deployed for a month in September 2018 and January 2019 in order to capture summer and winter seasonal peaks. Daily water temperature (3 m below Mean Lower Low Water) records were gathered from NOAA Data Buoy Center, Station CLBP4 located in Culebra, PR, approximately 3.8 and 4 km from LP and CR, respectively (Figure 1). Regional light data was obtained from the integration of 25 climatological models (CMIP5 IPCC) for Puerto Rico (San Juan PR, 18°26′24.0″N 66°07′48.0″W).
Tissue samples were clipped from coral fragments using bone cutters at the beginning of the experiment (April 2018), and subsequently stored in 95% non-denatured ethanol for DNA genotyping. Tissue samples were further collected from selected fragments at LP and CR reefs after 3, 5, 6, 9, 12, and 17 months-post-outplanting (hereafter referred to as T + month post outplanting), resampling fragments when possible. Repetitive samples were collected from grown branches, discarding the actively growing tip (generally without symbionts). The selection of specific fragments for sampling was determined based on the availability of healthy branches not previously disturbed. Coral samples were immediately flash-frozen, shipped on dry ice to Florida International University and stored at −80°C. In order to assess seasonal variation of healthy corals during the study period, only corals that survived the 17-month period and were sampled at least 4 times were included in DNA methylation analysis. This ensured replication at each sampling event. Overall, a total of n = 205 samples from the four outplanting sites were analyzed for DNA methylation (n = 55 for LPs, n = 38 for LPd, n = 64 for CRs, and n = 48 for CRd).
Coral Genotyping and Genomic DNA Isolation
We define a collection of fragments sharing the same multilocus genotype as belonging to the same “genet”, and each of the fragments is referred to as a “ramet.” Coral host genotyping was based on DNA isolated using a standard phenol-chloroform protocol (Sambrook and Russell, 2006) from the samples collected at the beginning of the experiment. A panel of 6 microsatellite loci was applied (Baums et al., 2005a). Since these markers were demonstrated to be highly heterozygous, the probability of wrongfully identifying ramets as clonemates of the same genet is consequently extremely low (Baums et al., 2005b). Only samples sharing the same alleles at all six loci were classified as ramets of the same genet. The descriptors of coral genotypic structure at the sampled sites, genotypic richness, diversity and evenness were calculated following (Stoddart and Taylor, 1988) and (Baums et al., 2006). Briefly, genotypic richness was calculated as the number of genets (Ng) over the number of colonies sampled (N). Genotypic diversity refers to the diversity of genets in a population. Here, it was calculated as the observed over the expected genotypic diversity (Baums et al., 2006). Observed genotypic diversity (Go) was calculated as per the equation (Stoddart and Taylor, 1988):
where gi is the relative frequency of each genet. Expected genotypic diversity (Ge) was equal to the total number of colonies analyzed (N), assuming a population with only sexual reproduction. This index of genotypic diversity, therefore, indicates the contribution of sexual reproduction to the population (Baums et al., 2006). Evenness was calculated as the fraction of the observed genotypic diversity (Go) over the number of genets (Ng).
Coral holobiont’s genomic DNA (82.0 ± 41.1 ng/μL, final concentration) was purified from flash-frozen tissue using the Quick DNA/RNA Mini-Prep kit (Zymo Research, Irvine, CA, United States) with some modifications: Briefly, coral fragments were pulverized in liquid nitrogen and approximately 100 mg of the resulting powder was resuspended in 2 mL vials containing 500 mg of Zirconia/Silica beads (0.5 mm diameter) and 1 mL of DNA/RNA Shield (Zymo Research, Irvine, CA, United States). Coral host cells were lysed using two pulses of 30 s in a vortex, in an attempt to leave most of the symbiont cells intact, thus enriching host DNA. However, a significant contribution of symbiont DNA to the final sample was assumed. After centrifugation (12,000 × g for 5 min), the supernatant was carefully transferred to a new tube and DNA isolation continued following the manufacturer’s instructions. DNA quality was assessed by gel electrophoresis for integrity and spectrophotometric analysis (NanoVue GE Healthcare, Chicago, IL, United States) for quality as described elsewhere (Rivera-Casas et al., 2017). DNA concentration was measured using a Qubit 2.0 Fluorometer (Thermo Fisher, Waltham, MA, United States) following the instructions provided by the manufacturer. Samples with concentrations under 40 ng/μL or low quality (i.e., ethanol contamination) were processed using a DNA Clean & Concentrator kit (Zymo Research, Irvine, CA, United States) until requirements were met.
Symbiodiniaceae ITS2 Amplicon Sequencing and Analysis
The ITS2 region was sequenced in coral samples in order to assess changes in symbiont community composition throughout the experiment. Accordingly, a total of n = 30 samples, consisting of 10 randomly selected coral fragments from the 4 outplanting and three representative time points (T3, T12 and T17), were used in the analysis. The isolated genomic DNA was quantified using the Qubit 2.0 DNA HS Assay (ThermoFisher, Waltham, MA, United States) and the quality assessed by the Tapestation genomic DNA Assay (Agilent Technologies, Santa Clara, CA, United States). Library preparation and sequencing was performed by Admera Health (South Plainfield, NJ, United States). Briefly, ITS2 spacer regions of the ribosomal DNA of the family Symbiodinaceae were amplified from 50 ng of isolated genomic DNA via PCR, using Symbiodinaceae-specific primers [ITS2alg-F, 5′-GTGAATTGCAGAACTCCGTG-3′; ITS2alg-R, 3′-TTCGTATATTCATTCGCCTCC-5′ (Pochon et al., 2001)] modified to include Illumina® adapters. The resulting libraries were quantified and assessed for quality before sequencing as detailed above, and barcoded for multiplexing using Illumina® 8-nt dual-indices. An equimolar pooling of the libraries was performed based on QC values and sequenced on an Illumina® MiSeq (Illumina, San Diego, CA, United States) with a read length configuration of 2 × 250 for 0.1 M pairs of reads per sample (500K in each direction).
Symbiodinaceae community composition was analyzed using the SymPortal Pipeline (Hume et al., 2019). Briefly, untrimmed demultiplexed forward and reverse sequences (fastq) were submitted directly into SymPortal for quality control and taxonomic assignment as described in Hume et al. (2019). Identified sequence variants per sample was used to characterize ITS2 type profiles (Hume et al., 2019). The abundance of ITS2 type profile and sequencing reads representative of putative Symbiodiniaceae taxa were used to evaluate changes in symbiont communities through time (T3, T12, T17). Differences of ITS2 profiles between collection times was evaluated by Permutational multivariate analysis of variance (PERMANOVA) with the adonis function in vegan (Oksanen et al., 2019), using fragment identity as strata in the model, and performing 9,999 permutations of residuals from Bray-Curtis dissimilarities.
Genome-Wide DNA Methylation Analysis
Genome-wide DNA methylation was assessed on coral host-enriched-DNA samples using an amplified polymorphism approach specific for DNA methylation states (Methylation Sensitive Amplified Polymorphism, MSAP (Reyna-Lopez et al., 1997; Xiong et al., 2013; Covelo-Soto et al., 2015). This method is based on the use of isoesquizomeric endonucleases, HpaII and MspI, with shared sequence targets (CCGG sites) but differential sensitivities to their DNA methylation. More precisely, HpaII cleavage is blocked by either internal cytosine methylation of the target site (i.e., 5′-CmCGG-3′/3′-GGmCC-5′) or its hypermethylation (i.e., 5′-mCmCGG-3′/3′-GGmCmC-5′). MspI, on the other hand, is sensitive to external cytosine methylation, including hemimethylation (i.e., 5′-mCCGG-3′/3′-GGCC-5′) and hypermethylation states. This allows the establishment of global cytosine methylation patterns by comparing both amplified restriction profiles (Díaz-Freije et al., 2014). Accordingly, coral genomic DNA was digested using EcoRI/HpaII and EcoRI/MspI endonuclease mixes in parallel reactions. In the same step, the resulting fragments were ligated to EcoRI and HpaII/MspI adapters (Table 1). Digestion-ligation reactions were performed for 2 h at 37°C in a solution consisting of 200 ng DNA, 4 U of EcoRI (NEB, Ipswich, MA, United States), 1 U of either HpaII (NEB, Ipswich, MA, United States) or MspI (NEB, Ipswich, MA, United States), 1 U T4 DNA ligase (Thermo Fisher Scientific, Waltham, MA, United States), 1X ligase buffer (Thermo Fisher Scientific, Waltham, MA, United States) and 1X CutSmart Buffer (NEB, Ipswich, MA, United States). The resulting restriction fragments were selectively amplified through two consecutive PCR reactions. First, a pre-selective reaction containing 2 μL of diluted (1:7) restriction-ligation product, 20 pM of each HpaII/MspI and EcoRI primers combination (Table 1), 1X PCR buffer, 0.5 mM dNTPs (Thermo Fisher Scientific, Waltham, MA, United States), 2.5 mM MgCl2, and 1 U DreamTAQ DNA polymerase (Thermo Fisher Scientific, Waltham, MA, United States). Second, a selective reaction used 0.5 μL of 1:9 of the pre-selective PCR product, 0.83 pM of each labeled selective primer (Table 1), 1X PCR buffer, 0.5 mM dNTPs, 2.5 mM MgCl2 and 1 U DreamTaq DNA polymerase. PCR conditions were identical to the original protocol (Reyna-Lopez et al., 1997), and the amplified products (2 per enzyme/sample combination, 4 selective combinations multiplexed, Table 1) were diluted to 1:10 for 6-FAM and 1:5 for 6-HEX prior to multiplexing and run on an ABI Prism 310 Genetic Analyzer (Applied Biosystems, Foster City, CA, United States) with a MapMarker 1000 ROX marker at Florida International University’s DNA Core facility.
Data and Statistical Analysis
MSAP restriction profiles were scored to a binary matrix for each primer combination with GeneMapper v.3.7 (Applied Biosystems, Foster City, CA, United States), retaining fragments between 50 and 500 bp and above 25 Relative Fluorescent Units for 6-HEX and 50 for 6-FAM. The matrices were filtered utilizing a 5% error rate (loci with one methylation state in more than 95% of the samples) and a 2% occurrence of any DNA methylation state to remove uninformative loci and analyzed using the R-package msap (Pérez-Figueroa, 2013). For a given animal, loci were scored according to the presence or absence of EcoRI-HpaII and EcoRI-MspI bands as either Non-Methylated (NMT, 0/0), Hemimethylated (HMM, 1/0), Internal Cytosine Methylated (ICM, 0/1) or Hypermethylated (HPM, 0/0). Hypermethylation was assumed on 0/0 loci due to the low genetic diversity on our dataset, which comprised the repetitive sampling of ramets of 7 genets. Loci were further classified as susceptible (MSL) or not susceptible to methylation (NML). The resulting data matrix of scored methylation states was subjected to further analysis.
Epigenetic variation on MSL was analyzed with Permutational Multivariate Analysis of Variance [PERMANOVA] (Anderson, 2001), considering genet, outplant site and collection time as grouping variables in the model genet × fragment × time + site as implemented on the R-package vegan [adonis function (Oksanen et al., 2019)]. Fragment identity was included in the model and as a strata to assess the effect of repeated measurements. A Euclidean distance matrix was generated with 9,999 permutations. Pairwise PERMANOVA (Martinez-Arbizu, 2019) with Holm’s correction (Holm, 1979) was performed to evaluate variables with significant effects on DNA methylation. Statistical significance of each MSL was assessed by means of multiple comparisons between the experimental groups by Fisher’s exact tests with Benjamini and Hochberg multi-test corrections (Benjamini and Hochberg, 2000; adjusted p < 0.05, pFDR < 0.05), identifying loci with non-random distribution of DNA methylation states for each experimental variable. Using these significant MSL, pairwise distances between all analyzed coral fragments with Gower’s Coefficient of Similarity were computed. The resulting distance matrix was clustered with UPGMA (unweighted pair group method with arithmetic mean) and visualized as a heatmap with ComplexHeatmap (Gu et al., 2016).
A Discriminant Analysis of Principal Components (DAPC, Pritchard et al., 2000; Jombart et al., 2010; Grünwald and Goss, 2011) was performed to assess the epigenetic discrimination between groups using adegenet (Jombart, 2008). The number of principal components (PCs) retained for the analysis was evaluated with two rounds of cross-validation [Xval.dapc function, (Jombart and Collins, 2015)]. All discriminant functions (K-1 = 5) were retained in the analysis. Correlation between the independent variables (Temperature and light) and DAPC coordinates of temporal variation in DNA methylation was evaluated. Appropriate Lag shifts were calculated [ccf function, (Brockwell and Davis, 2009)] to determine the cross correlation between each of the univariate series. Next, the lag corrected series (Lag corrected + 1 shift for temperature) were input into a matrix of Pearson’s r rank correlation coefficients using rcorr in the Hmisc library (Harrell and Harrell, 2019).
Non-Metric Multidimensional Scaling Analysis (NMDS) was performed utilizing Gower’s distances, and environmental parameters were fitted as vectors in the ordination (envfit function) with vegan (Oksanen et al., 2019) to represent their effect on DNA methylation. Monthly mean values, maximum, standard deviations and differences for each environmental factor were employed as vectors. For temperature and light irradiance long-term data sets, a coefficient of variation of the previous 3 months (CV3) to each sampling month was calculated and employed as an additional vector to evaluate a possible response to the relative change in the parameter and not the actual magnitude. Significance and coefficient of determination was calculated for each of these parameters.
Fragment Sequencing and Identification
Preselective products from 10 samples with high band representation for each selective (SL1-4) and enzyme (Hpall and Mspl) combination were pooled and amplified with non-labeled selective primers. Resulting products (n = 8) were cleaned with a DNA Clean & Concentrator kit (Zymo Research, Irvine, CA, United States), quality checked with a TapeStation D1000 ScreenTape (Agilent Technologies Inc., CA, United States) on a Tapestation 4200 system and multiplexed with a Native barcoding expansion kit (EXP-NBD104, Oxford Nanopore Technologies). Libraries for Oxford NanoPore sequencing were constructed with a ligation library kit (SQK-LSK109, Oxford Nanopore Technologies, Oxford, United Kingdom) and sequenced to a total of 20GB on MinION R9.4 flowcells. The resulting sequences were basecalled and demultiplexed with the MinKNOW software, trimmed with Porechop1 to eliminate PCR adapters, and mapped to the genomes of A. digitifera (Shinzato et al., 2011) and Symbiodinium microadriaticum (Aranda et al., 2016) using Minimap2 (Li, 2018).
Results
Abiotic Characterization and Seasonality
Hourly data (n = 824) was recorded for temperature, photosynthetic active radiation (PAR), dissolved oxygen (DO), and salinity at two sites at a depth of 5 and 15 m (LPs and LPd, respectively) during two monthly deployments to capture peak summer and winter signals in sites representative of studied depths (Supplementary Table 1). Greater values (two tailed t-test p < 0.05) for pH, PAR and Salinity were observed at LPs as opposed to LPd. However, as expected, both depths showed greater values of temperature and PAR as well as lower pH, DO and salinity during the summer (two tailed t-test p < 0.05). Temperature daily mean for each month was analyzed for seasonality, revealing a trend for the period through 2018 and 2019 (Mann-Kendall trend test p = 0.007). This is graphically confirmed (Supplementary Figure 1) by applying a moving average to the data set to extract the seasonal component from the trend and error terms assuming an additive model because the variance structure remained homogeneous throughout the periods observed (decompose function in the Stats package R). Solar Radiation is reported as W/m–2 with peak values in April and lowest values reported in December.
Genotypic Composition of Source Reefs
A total of n = 81 A. cervicornis host genets were identified in 186 of the 200 initial fragments analyzed (14 samples failed): 45 from Los Corchos (LC), 15 from Carlos Rosario (CR), 14 from Luis Peña (LP), and 7 from Culebritas (CUL) (Figure 1). All genets were exclusive to their corresponding sampling site, and three to four prevalent genets accounted for 67–75% of the collected fragments at each site, with the exception of LC, where most genets had only one or two ramets. The genotypic structure was subsequently described for each site (Table 2), resulting in an overall genotypic richness [number of genets (Ng)/number of samples (N)] of 0.38 ± 0.21 for all sites combined. LC showed the highest richness amongst all sampled sites. Genotypic diversity [observed genotypic diversity (Go)/expected genotypic diversity (Ge)] followed the same pattern with combined values of 0.24 ± 0.16 and 0.47 for LC. For evenness (Go/Ng) however, both sites in the east of the island (LC and CUL) showed similar values (around 0.68) while the sites on the west were lower (around 0.5).
Symbiodinaceae Community Composition and Dynamic
In order to evaluate symbiotic community dynamics through the duration of the study, ITS2 amplicon sequences for the Symbiodinaceae family were analyzed. The 30 samples generated 5,415,404 sequencing reads, producing 2,707,680 sequences after quality filtering into the SymPortal pipeline (50%). A total of 57 operational taxonomic units were identified from ITS2 sequences, with the majority of filtered ITS2 sequences being of the genus Symbiodinium (formerly Clade A), and a minor representation of genuses Brevolium (formerly Clade B) and Cladocopium (formerly Clade C) (Supplementary Figure 2). Four ITS2 type profiles were identified across samples, all uniquely composed by Symbiodinium spp. sequences. ITS2 profile shifts were observed in some of the samples. However, no significant dynamic changes were evidenced between collection times (PERMANOVA; F = 0.2552, p = 0.6646; Supplementary Table 2).
Global Genome-Wide DNA Methylation Variability
A total of 7 genets were selected among those represented by the transplanted fragments for DNA methylation analyses. Genet selection was based on the number of ramets of each genet surviving the 17-month period, allowing appropriate replication between outplanting sites and source sites. The availability of a minimum of 3 ramets of each genet per outplanting site at the end of the 17-month period were used as criteria for selection. Selected genets were n = 3 from CR (C1708, C1732 and C1739), n = 2 from LP (C1727 and C1733), and n = 2 from CUL (C1706 and C1734), representing most of the highly represented genets at each source site (Figure 1). Unfortunately, no genet from LC satisfied the criteria to be included in the DNA methylation analyses.
MSAP analyses were performed to assess changes in whole-genome DNA methylation profiles of corals depending on their outplant site, genet and/or collection time. The four combinations of primers tested yielded a total of n = 199 loci after quality-filtering, among which 192 were categorized as methylation-susceptible loci (MSL, 96%) and the remaining 7 were non-methylated (NML, 4%) loci. Primer combinations SL2 and SL4 (Table 1) showed the highest number of methylation-susceptible loci with 93 (46.7%) and 81 (40.7%), respectively. The overall epigenetic diversity within methylation-susceptible loci, based on the occurrence of the different DNA methylation states by means of Shannon’s diversity index (SDI), was 0.33 ± 0.22, while non-methylated loci showed a Shannon diversity index of 0.22 ± 0.08. A total of 162 (84%) of the methylation-susceptible loci were characterized as polymorphic, showing at least two occurrences for each DNA methylation state, either NMT, ICM, HMM or HPM. These polymorphic loci were subsequently used for further analyses aimed to describe the influence of collection time, outplant sites, and genet on the DNA methylation patterns.
The results indicate a dynamic fluctuation in coral DNA methylation states over time (Table 3 and Supplementary Figure 3). Accordingly, HPM and NMT trended upward from July 2018 (T3, i.e., 3 months post-outplanting) to April 2019 (T12), then decreased by September 2019 (T17). ICM and HMM showed the opposite trend, with an absolute minimum value by T12 and a subsequent increase by September 2019 (T17). This variation of DNA methylation patterns over time was significant, as revealed by PERMANOVA (Supplementary Table 3, F = 4.1524, p < 0.0001). Further post hoc analyses (Table 4) revealed significantly different DNA methylation patterns between all pairwise sampling time comparisons except for October 2018 with September 2018 (T5-T6, F = 1.6992, p = 0.0994) and October 2018 with Jan 2019 (T6-T9, F = 1.2757, Adjusted p = 0.1782) respectively.
The contribution of genet and outplanting sites to the variability observed in DNA methylation states was also evaluated using PERMANOVA analyses (Supplementary Table 3). While no significant differences were observed between outplanting sites (F = 0.8735, p = 0.6637), genets influenced DNA methylation significantly (F = 2.3315, p = 0.0131). Accordingly, post hoc analyses (Table 5) revealed significant pairwise differences of genet C1739 with C1733 (F = 3.2225, Adjusted p = 0.0084) and marginally significant with C1732 (F = 2.539, Adjusted p = 0.0494) and C1727 (F = 2.449, Adjusted p = 0.0494). Additional marginal significance was found between genets C1732 and C1708 (F = 2.4780, Adjusted p = 0.0494), and between C1734 and C1708 (F = 2.4824, Adjusted p = 0.0440). It is interesting to note that most genet pairs showing significant differences in DNA methylation originated from the same source reefs or from reefs located near each other (i.e., CR and LP), making it less likely that similarities in DNA methylation patterns displayed by most genets were determined by epigenetic memory or local adaptation. Fragment (ramet) identity also had a significant effect on DNA methylation patterns (F = 1.1037, p = 0.0131).
Seasonal Influence on Global DNA Methylation Patterns
Considering the significant fluctuation observed on DNA methylation patterns throughout the studied time series, detailed analyses were performed to ascertain the exact contribution of seasonality to such variation. First, Fisher’s exact test analyses were conducted to identify significant MSL, resulting in n = 83 MSL with both significant differences among experimental times (Adjusted p < 0.05) and low probability of false positives (pFDR < 0.05). As shown in Figure 2, the clustering analyses of identified loci organized the samples into two major groups based on similar distribution of DNA methylation profiles, discriminating between cold (T12) and warm (T3, T5 and T17) months. Samples from T6 and T9 showed a scattered distribution across these two clusters, while most T17 specimens constituted a well-defined sub-cluster within the warm group.
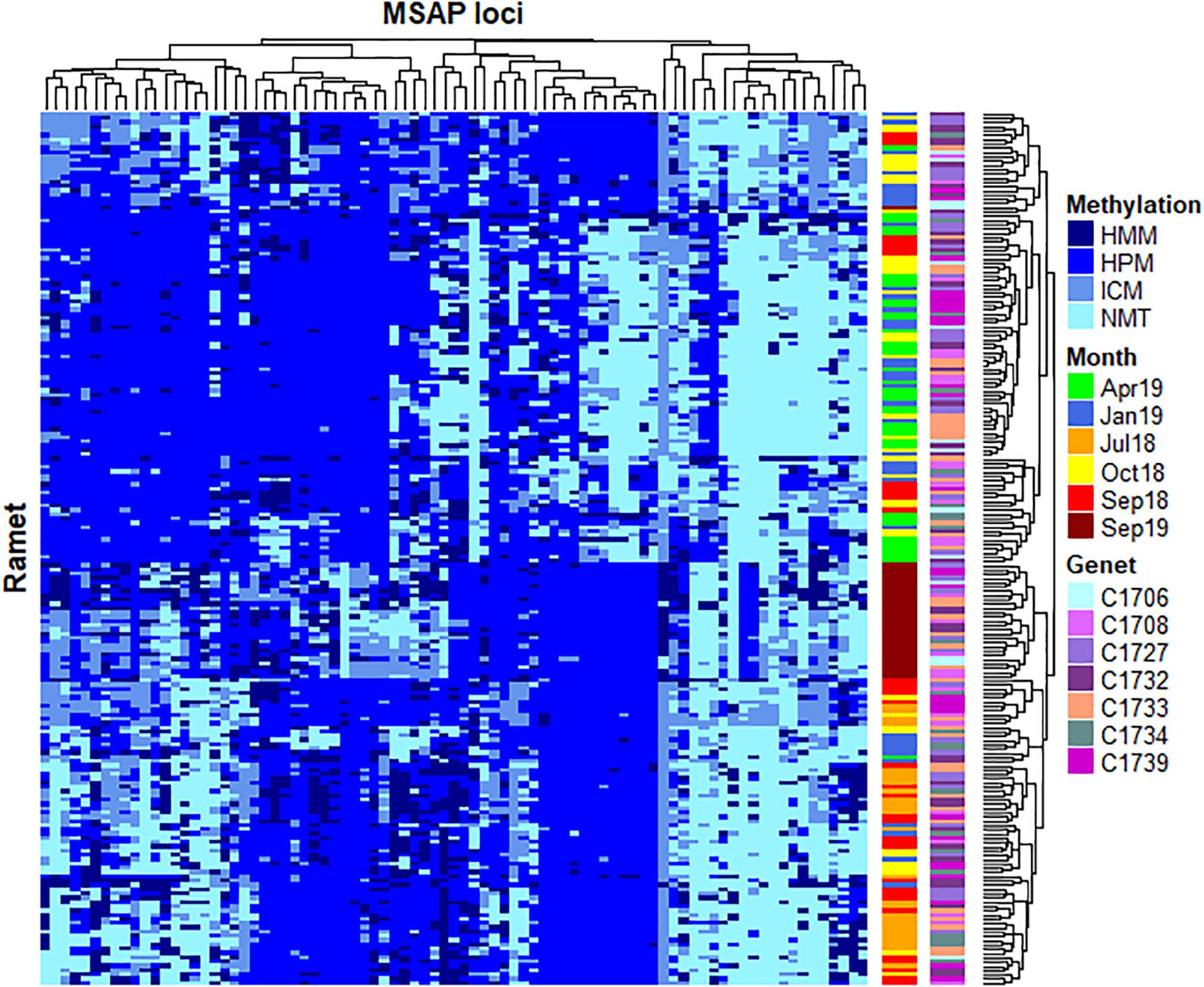
Figure 2. Heatmap representing temporal changes in 83 loci showing a significant non-random distribution of DNA methylation patterns (p < 0.05, pFDR < 0.05). Two distinctive clusters separate DNA methylation between cold (April 2019) and warm (July 2018, September 2018 and September 2019) months. Samples from October 2018 and January 2019 show a scattered distribution across these two clusters, while most September 2019 specimens constituted a well-defined sub-cluster within the warm group. No clear clustering is observed for specific genets. Rows (samples) and columns (MSAP loci) were clustered using Gower’s Coefficient of Similarity. The methylation status of each locus is indicated in the right margin of the figure: HMM, hemimethylated; HPM, hypermethylated; ICM, internal cytosine methylation; NMT, non-methylated (unmethylated).
In order to further assess epigenetic discrimination among sampling times, a Discriminant Analysis of Principal Components (DAPC) analysis was employed (Figure 3). As evidenced by the first discriminant function (LD1, x-axis, horizontal, Figures 3A,B), T17 samples constituted a well-defined cluster with distinct epigenetic signatures respective to the remaining samples. In contrast, the second discriminant function (LD2, y-axis, verticals Figures 3A,C) split the samples into warm (T3, T5 and T17) and cold (T6, T9 and T12) months, with each of these sampling times forming a discrete cluster. Along this axis, T17 occupied a position between T5 and T6 corresponding to the same period in the previous year. Analysis of the individual contribution of each locus to the group separation (Jombart and Collins, 2015; Figures 3D,E) resulted in the identification of different groups of loci mediating the separation of each discriminant function. Marked differences in the frequency of occurrence of each DNA methylation status in these loci through time (Figures 3F,G) were observed, with loci contributing to LD1 showing stable frequencies with a drastic change at T17, while LD2 loci showed a variable temporal response. These differences could indicate the occurrence of different overlapping responses mediated by DNA methylation changes.
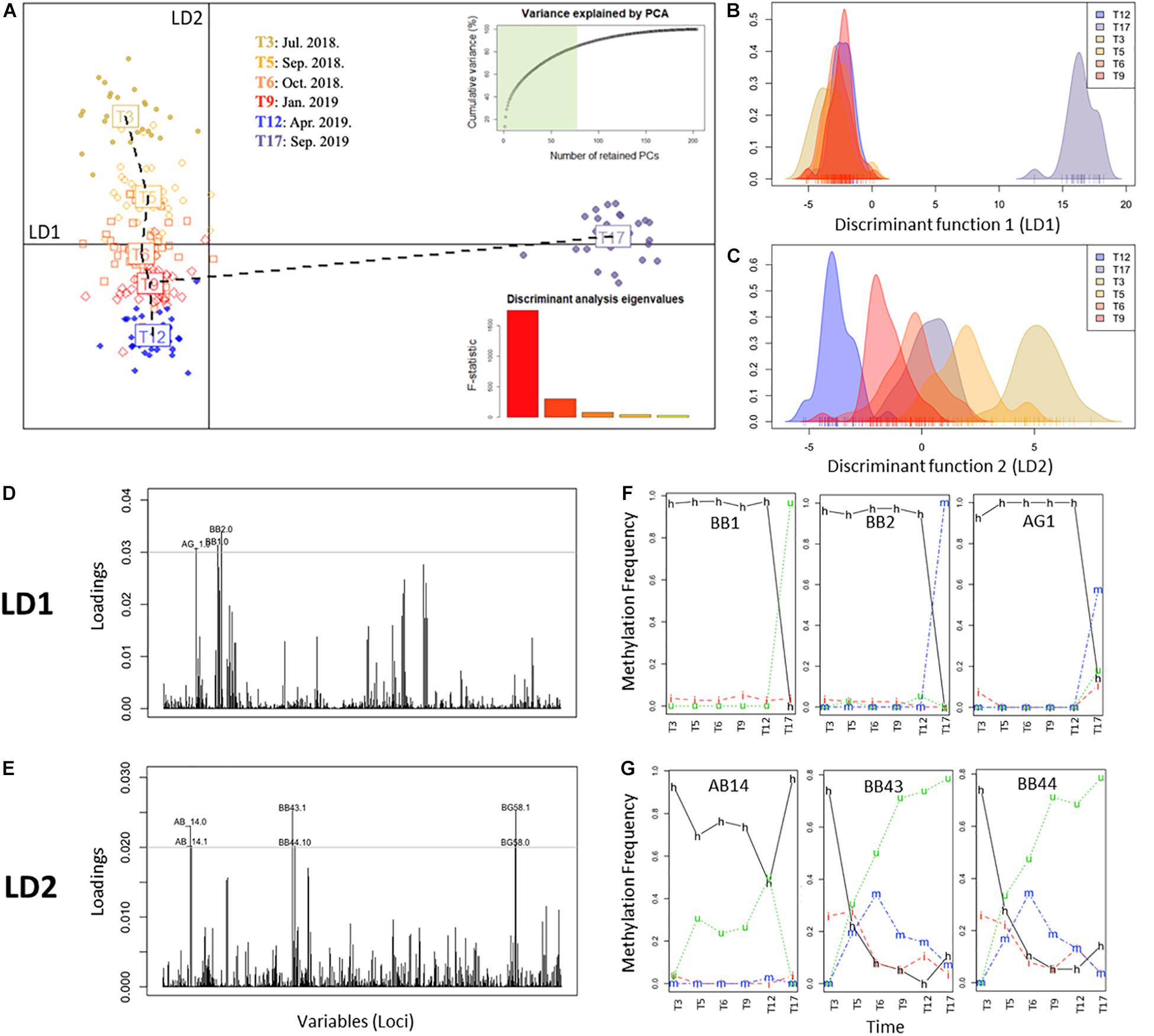
Figure 3. Discriminant analysis of principal components (DAPC) of complete MSAP profiles representing the different groups (i.e., time points). (A) Scatterplot of monthly clusters resolved by DAPC. Barplot shows the significance of each of the five discriminant functions retained. In the upper-left corner the variance explained by the 77 PCs retained for the study. Horizontal line: x-axis, first discriminant function (LD1) and vertical line: y-axis, second discriminant function (LD2). (B,C) Density of methylation profiles of each A. cervicornis fragment against discriminant function 1 (LD1) and discriminant function 2 (LD2), respectively. (D,E) Loading plot of MSAP loci that most contributed to LD1 and LD2 respectively. (F,G) Temporal variation of the frequency of each methylation status of loci with high contribution to LD1 (BB1, BB2, AG1) and LD2 (AB14, BB43, BB44), respectively. Methylation status is indicated in the lines of the figure: h, hemimethylated; m, hypermethylated; i, internal cytosine methylation; u, non-methylated (unmethylated).
To further investigate this, discriminant 3 (LD3) was also evaluated (Figure 4), in spite of its lower discriminant power (hence significant; F = 76.29, p < 0.0001). In this function, the marked separation of T17 was no longer evident and a clearer seasonal pattern emerged (Figures 4A,B). Remarkably, LD3 pattern correlated significantly to Temperature (+1 lag, r = 0.91, p = 0.0310), but not with irradiance (r = −0.76, p = 0.0783) that showed significance only for α = 0.1. Although LD3 has lower discriminant power (Figure 4B), the temporal changes of DNA methylation status in the main contributing loci showed a dynamic variation as in LD2 (Figure 4C). Altogether, these results show an orderly transition of DNA methylation profiles during the months after the introduction of corals in their new environment, apparently driven by a warm-cold seasonality, but experiencing a pronounced change from T12 to T17 maybe related with a heat-stress event throughout this period.
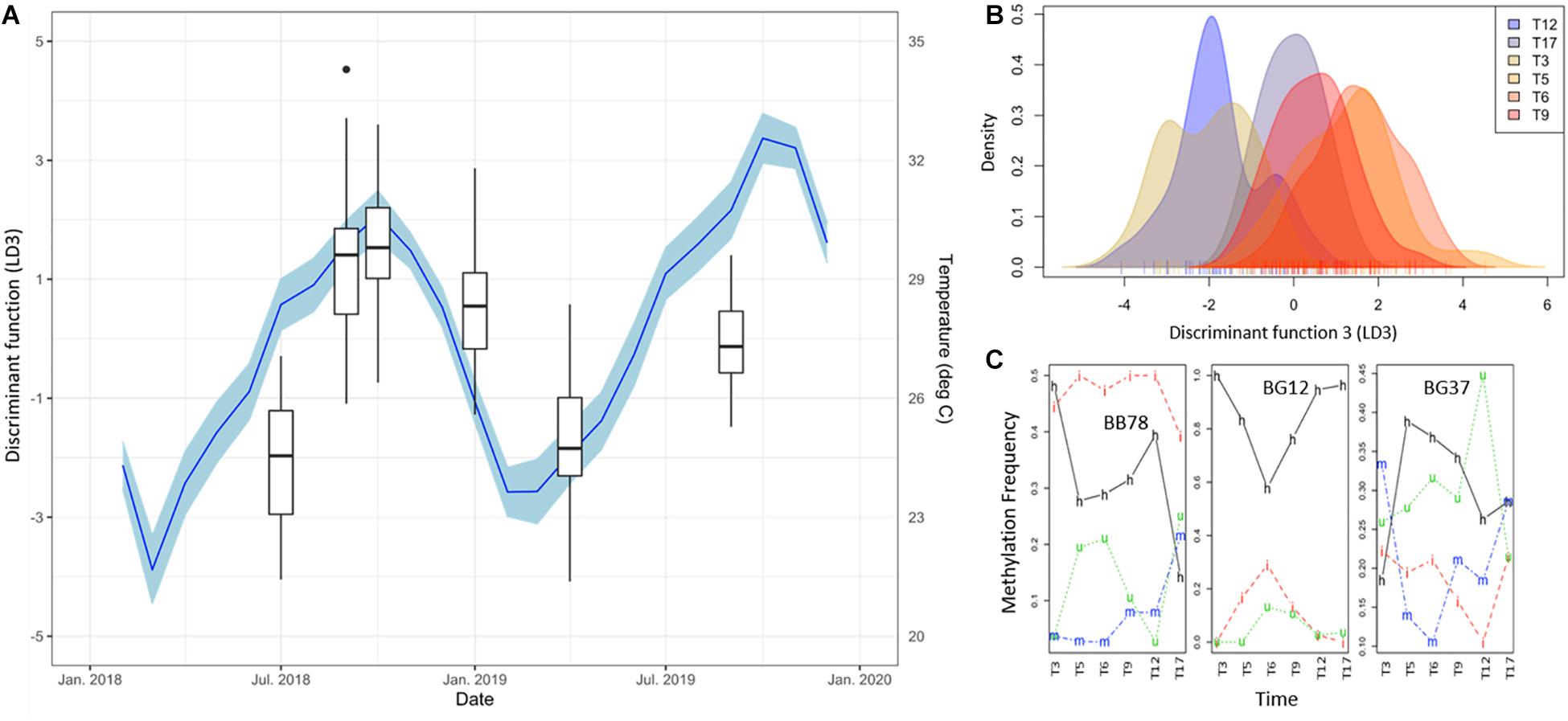
Figure 4. (A) Time series of DNA methylation pattern separation as given by the DAPC discriminant function 3 (LD3, Box Plots). Black dots indicate outliers. Monthly average of daily mean temperature (NOAA Buoy CLBP4, blue line), lagg corrected + 1 as calculated with the ccf function in R, is shown as additional y-axes. Blue shadings indicate 95% confidence intervals of temperature variability. Note the clear seasonal variation of the DNA methylation patterns evidenced by this discriminant function and its significant correlation with temperature changes (Pearson’s rank correlation: r = 0.91, p = 0.0310). (B) Density of DNA methylation profiles of each A. cervicornis fragment against discriminant function 3 (LD3). (C) Temporal variation of the frequency of each methylation status of three loci with high contribution to LD3 (BB78, BG12, BG37). Methylation status is indicated in the lines of the figure: h, hemimethylated; m, hypermethylated; i, internal cytosine methylation; u, non-methylated (unmethylated).
Contribution of Coral Host vs. Symbiont to MSAP-Amplified Loci
Considering the limitations to separate symbiont and host DNA efficiently, additional analyses were performed to evaluate the contribution of the symbionts to the methylation pattern observed. Therefore, MSAP products were sequenced and aligned against the genomes of the closely related acroporid coral A. digitifera (the A. cervicornis genome was not available at the time of this analysis) and a representative symbiont (S. microadriaticum, formerly clade A). All MSAP selective-enzyme combinations (n = 8) produced a total of 30,519,266 reads after trimming. From those, 27,696,330 reads mapped to the coral genome (90.75%), while only 388,363 reads mapped to the symbiont genome (1.27%). This result indicates that although contamination with symbiont DNA is present, its contribution to MSAP loci is negligible.
Environmental Parameters Driving Seasonal Variability in Global DNA Methylation Patterns
Given the observed seasonal trend in DNA methylation and its link with regional temperature and light irradiance patterns, further analyses were performed to evaluate such relationship. Accordingly, the contribution of different environmental parameters was assessed by conducting non-metric multidimensional scaling (NMDS), fitting vectors to the ordination using the function envfit. Considering the abiotic data available and the lack of difference between the DNA methylation response among outplanting sites, two separate analyses were implemented. First, only samples from T5 (September 2018) and T12 (April 2019) for sites LPs and LPd (where site-specific environmental data was collected) were included (Figure 5A). This dataset allowed the evaluation of the contribution of temperature, pH, DO, salinity, and PAR to DNA methylation patterns. Results revealed that temperature, pH and DO correlated significantly with the NMDS ordination of the DNA methylation patterns driven by collection time (Figure 5B and Supplementary Table 4), while surprisingly PAR did not. Despite clear abiotic differences between depths, these parameters correlate to DNA methylation differences across sampling time points instead of sampling sites, indicating that seasonal variation in these environmental parameters was more relevant than site specific conditions in modulating DNA methylation patterns.
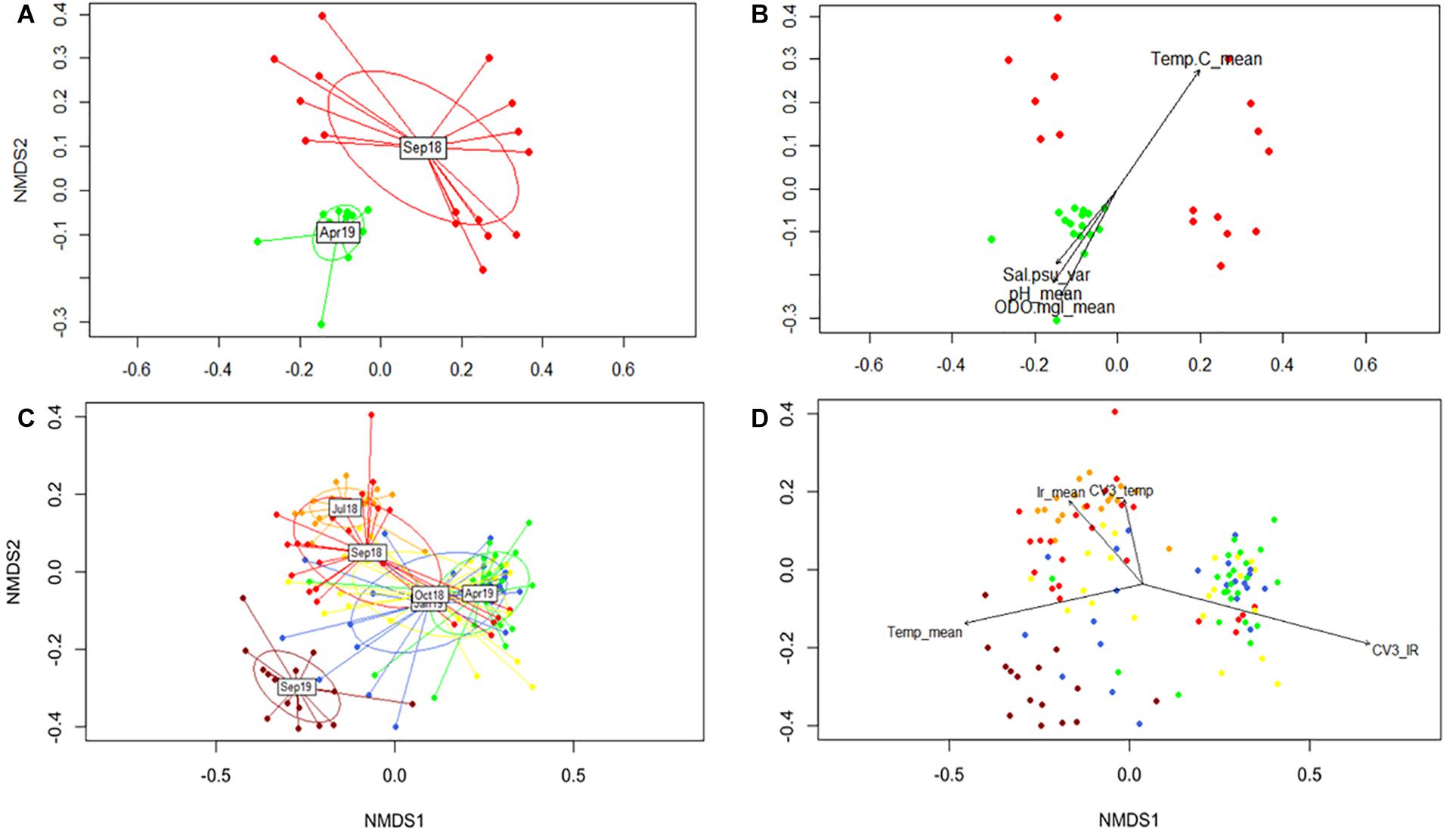
Figure 5. Non-metric multidimensional scaling (NMDS) of global DNA methylation patterns using Gower distances. (A) Temporal separation for T5 and T12 for LP sites. (C) Representation of all sampling times for both shallow sites (LP and CR). (B,D) Significant correlations of environmental parameters to DNA methylation patterns (P < 0.05). Colors correspond to collection times.
The second analysis fitted regional temperature and light irradiance to the ordination of all sampling times, but only for shallow sites in both reefs. This was performed to determine the influence of these parameters during the duration of the experiment without introducing errors derived by differences in irradiance between depths. We tested the contribution of monthly averages together with the coefficient of variation of the previous 3 months (CV3) for each variable. The NMDS ordination with all the data corroborated the DAPC analysis by showing T17 as an independent cluster (Figure 5C). All vectors analyzed showed a significant correlation with the ordination (Figure 5D and Supplementary Table 5), with temperature mean and CV3 of the irradiance showing the highest coefficients of determination (R2). Interestingly, it seemed that light and temperature were sensed differently by DNA methylation mechanisms, with rapid responses to temperature and a potentially lagged response to light (Figure 5D).
Discussion
This work constitutes the first attempt to characterize seasonal epigenetic changes in stony corals, providing support for the role of DNA methylation during seasonal acclimatization in the coral A. cervicornis. The results presented in this work suggest that DNA methylation profiles in this species vary following a season-dependent trend, with similar temporal changes in DNA methylation patterns in all inspected coral fragments, regardless of their genotype, source reef or outplanting site. This concurs with the frequently proposed notion of a seasonal variation in the phenotype of corals, including the presence of winter and summer ecotypes (Scheufen et al., 2017), likely driven by observed transcriptional changes (DeSalvo et al., 2008; Kenkel et al., 2013). These findings underscore the importance of including seasonal variability in environmental epigenetic studies in marine (specially sessile) organisms (Parkinson et al., 2018).
Variability and Seasonal Trends in Environmental Abiotic Parameters
Describing changes in environmental conditions is a prerequisite for the establishment of a seasonal dependence in any organismal response. Since DNA methylation data did not differ among sites, it was possible to use data from NOAA’s weather buoy (CLBP4) to describe changes in temperature for all study sites, and data derived from climatological models (CMIP5 IPCC) for light irradiance. A limited in situ dataset was used to corroborate the responsiveness of DNA methylation to regional seasonal environmental variation, therefore validating the use of regional data and models to describe the general seasonal patterns as evidenced in temperature correlation with DNA methylation patterns with both datasets (Figure 5). Given the resolution of the regional light dataset with a limited sensitivity to differences in depth, it is not possible to categorically invoke interactive effects with depth and season based on the obtained data. Nonetheless, these results strongly support the interest of future research to understand the interactive effects of seasonality and depth differences on global DNA methylation.
Genotypic Composition of Source Reefs
Genotypic variation is correlated with diverse stress responses, disease resistance, epigenetic patterns and reproductive output in Caribbean Acroporids (Baums et al., 2013; Parkinson and Baums, 2014; Drury et al., 2019; Durante et al., 2019). In this experiment, fragments were collected using an opportunistic sampling approach that favors the collection of dominant genets. It is thus encouraging that multiple genets were collected at each site, indicating that the genotypic diversity of A. cervicornis around Culebra is not low (Figure 1 and Table 2). Genets were restricted to one collection site each, and thus there was no evidence of long-distance dispersal of asexually derived fragments. This is not surprising, considering that asexual fragmentation (Tunnicliffe, 1981; Drury et al., 2019), restricts dispersion to a few hundred meters under natural conditions (including hurricane impacts), restricting genet distributions. Therefore, genotypic diversity observed on each site was mostly based in sexual recruitment.
Temporal Differences Dominate Patterns of DNA Methylation
Epigenetic landmarks, such as histone variants and DNA methylation, influence phenotypic plasticity in response to changes in environmental conditions and are, therefore, predictors of the general state of the organism in the face of environmental alterations and natural cycles (Rivière, 2014). Emerging evidence suggests that these mechanisms play an important role during responses to environmental changes, likely by regulating gene expression and maintaining DNA integrity throughout the entire lifespan of an organism (Roberts and Gavery, 2012; Dimond and Roberts, 2016; Liew et al., 2018; Rodriguez-Casariego et al., 2018). Recent studies on marine invertebrates [reviewed in Eirin-Lopez and Putnam (2019)] have shown that DNA methylation exerts a role on phenotypic acclimatization (Putnam et al., 2016; Liew et al., 2018; Durante et al., 2019) by modulating gene expression (Dixon et al., 2018). Moreover, epigenetic marks acquired throughout the lifespan of coral can be inherited intergenerationally, promoting acclimatized phenotypes in the offspring and thus increasing their fitness (Liew et al., 2020; Putnam et al., 2020). In addition, seasonal patterns of DNA methylation have been observed in vertebrates (Stevenson and Prendergast, 2013; Viitaniemi et al., 2019), invertebrates (Pegoraro et al., 2016; Suarez-Ulloa et al., 2019) and plants (Finnegan et al., 1998; Bastow et al., 2004; Ito et al., 2019). Based on these elements, it is not surprising that DNA methylation could play an active role during coral responses to seasonal variation.
The PERMANOVA analysis of all loci susceptible to DNA methylation showed clear differences in DNA methylation patterns between sampled months and genets, while no differences were observed between, sources or outplant sites in this study. This is a remarkable result, considering the significant differences in environmental conditions and habitat type between deep and shallow sites (see Supplementary Table 1), although the seasonal variation is larger for most parameters, including temperature. In corals, several studies have also found clear changes in DNA methylation in response to experimental manipulation in environmental conditions (Putnam et al., 2016; Liew et al., 2018; Cziesielski et al., 2019). On the other hand, a study aimed to evaluate the components of phenotypic divergence between clonemates of A. palmata under natural conditions (Durante et al., 2019), attributed most of the variation in DNA methylation to difference among genets followed by micro-environmental conditions, rather than between study sites. Nonetheless, this study was still able to observe small differences between sites. In the present work, A. cervicornis fragments were transplanted to new locations and only sampled after an acclimation period, hence source-site specific differences in DNA methylation profiles could have been diluted after a rapid acclimation. Still, evidence here suggests that seasonality remains as a stronger modulator of DNA methylation patterns. Coral genotype also exerts a significant effect over DNA methylation variability, although to a lesser extent than the aforementioned temporal influence, as evidenced by PERMANOVA (Supplementary Table 3). This observation is consistent with the dependence of DNA methylation on the presence of CpG sites in the DNA, and is further supported by previous evidence that DNA methylation in corals (or in any other eukaryotic organism) directly relies on sequence features of the genome, also supporting its heritability (Dixon et al., 2014; Liew et al., 2018; Durante et al., 2019).
Coral DNA Methylation Displays Seasonal Trends in Response to Environmental Changes
Seasonal environmental variation, similar to diel cycles, triggers the adjustment of physiological functions in corals (Hill and Ralph, 2005; Ulstrup et al., 2008; Brady et al., 2011; Sorek et al., 2014). The obtained results support the role of DNA methylation on the seasonal acclimatization of A. cervicornis, as evidenced by a clear temporal effect over the MSAP methylation patterns. DNA methylation seems to follow seasonal trends in temperature, light, DO and pH, as evidenced by the significant correlation between DNA methylation ordination and the vectors representing mean-value variation of these parameters and coefficient of variations in the case of light and temperature (Figure 5), hinting a possible lagged response. However, analysis of the complete dataset with specific methylation patterns (DAPC) showed that temperature (1 + lagged) significantly correlate with changes in DNA methylation, while light was significant only under α = 0.1. Yet, interactive effects of light seasonality and depth differences on global DNA methylation require additional analyses. Overall, it seems that seasonal variation in temperature, light, pH and dissolved oxygen modulate DNA methylation patterns.
This seasonal trend, however, seems to be masked by other phenomena occurring in the temporal scale. For example, samples collected during September 2019 have homogeneous DNA methylation profiles, markedly differentiated from the remaining sampling times by the first discriminant function of the DAPC analysis (Figure 3A). However, as revealed by the second and third linear discriminant functions (LD2 & LD3) of the DAPC analysis (Figures 3C, 4), these samples are more related to September and October 2018. Although this may sound incompatible with an annual periodicity in DNA methylation profiles (considering there is just one replicated time point in both years), this change may simply reflect either coral acclimation to the experimental environment within the possibilities of its genetic and epigenetic backgrounds, or more likely, a response to stress.
Under an acclimation scenario, the switch in DNA methylation patterns would be immediate and then progressively undergo a resilience period after which the epigenome would be reprogrammed, resulting in the activation (or repression) of genes previously silenced (or activated) under native conditions. Unfortunately, the DNA methylation trends characterized in the present work do not support this notion. While rapid epigenetic changes were observed by our own previous research in coral (Rodriguez-Casariego et al., 2018), the constant change in DNA methylation patterns observed in the present work is not consistent with a linear progression toward an acclimated state. Indeed, several loci follow a seasonal-like pattern returning to DNA methylation values similar to those measured during the same season in the previous year (Figures 3G, 4). This is especially evident in the loci driving the divergence of T17, which display a rather abrupt change instead of a progressive transition toward an acclimated state (Figure 3F).
A stress response hypothesis, on the other hand, would be consistent with the occurrence of an abnormal event in September 2019, justifying the dramatic change observed in the aforementioned loci. Abiotic monitoring data seem to validate this idea, including extremely high seawater temperatures during the summer of 2019 (+0.5–1.3°C, between July and October) compared to the same period of 2018. Indeed, a moderate bleaching event was observed in the area in subsequent months following an accumulation of 7 Degree Heat Weaks (Weil et al., 2019). Thermal-stress has been linked to significant changes in coral transcriptional profiles (DeSalvo et al., 2008; Voolstra et al., 2009; Kenkel et al., 2013), and to rapid epigenetic responses (Barshis et al., 2013; Palumbi et al., 2014), even at stress levels not high enough to produce bleaching (Rodriguez-Casariego et al., 2018). However, the anticipation of the response observed in T17 to the heat-stress event opens the possibility that DNA methylation could represent an early indicator of a changing thermal environment.
Overall, the evidence of a seasonal-driven response of DNA methylation presented by this work is in agreement with observed seasonal changes in gene expression in A. cervicornis (Parkinson et al., 2018), and phenotypic changes described in the coral holobiont (DeSalvo et al., 2008; Kenkel et al., 2013). Given the proposed role of DNA methylation mediating transcriptional plasticity (Dixon et al., 2014; Dimond and Roberts, 2016), it is not surprising to find such a seasonal response. Previous studies have also highlighted significant responses in the holobiont physiology, supporting seasonal variations (Chen et al., 2005; Ulstrup et al., 2008; Carballo-Bolaños et al., 2019). Bacterial community composition has been also described to follow a certain seasonal pattern in several coral species (Li et al., 2014; Sharp et al., 2017; Cai et al., 2018). Changes in symbiont cell density, pigment composition, and photosynthetic capacity following annual periods have also been reported (Fitt et al., 2000; Warner et al., 2002; Ulstrup et al., 2008). While the proposed role of temperature mediating seasonal changes in coral physiology (Brown et al., 1999; Fitt et al., 2000; Dimond and Carrington, 2007) was confirmed for DNA methylation here, non-conclusive evidence of the significant effect of other environmental factors with seasonal trends like pH, DO and light was obtained and will require further study. Given the marked seasonality observed in calcification rates and photosynthetic production (Hinrichs et al., 2013; Samiei et al., 2016), it is not surprising that these factors would also influence DNA methylation patterns potentially involved in the establishment of these seasonal phenotypes.
Conclusion
The present work provides support for the role of DNA methylation during seasonal acclimatization of the coral A. cervicornis, based on its correlation with seasonal environmental variation independently of genotypic and site-specific differences. The emergence of these patterns, despite the complexity of DNA methylation responses to environmental stress described in marine invertebrates and the limited resolution of the method employed here (when compared to sequencing techniques), support the relevance of this phenomena for epigenetic regulation in corals. Given the ecological importance of coral acclimatization in the Anthropocene and the potential similarities between seasonal adjustments and heat-stress responses, the evidence generated by the present effort constitutes an initial approach to understanding the dynamicity and the potential for intergenerational inheritance of this epigenetic mechanism. Further studies will be instrumental to decipher the extent in which seasonally driven epigenetic patterns are indicative of IGP, ItGP or even TGP, encompassing critical implications on the current understanding of the epigenetic regulation of phenotypic plasticity. Overall, the data generated with this work will serve as a baseline to filter the contribution of seasonal-driven DNA methylation changes in studies addressing epigenetic responses to stressors, and as background for the study of environmental disturbances caused by extreme weather episodes (e.g., hurricanes).
Data Availability Statement
Raw sequence data for ITS2 and MSAP analyses are available at the NCBI Sequence Read Archive under BioProjects with accession numbers PRJNA661294 and PRJNA661530, respectively. Additional datasets utilized in the study can be found in the Github repository https://github.com/eelabfiu/seasonal_PR.
Author Contributions
JR-C designed the work, performed fieldwork, lab experiments, data analyses, and wrote the manuscript. AM-M designed the work, performed fieldwork, lab experiments, data analyses, and wrote the manuscript. DG-S designed the work, performed data analyses, and wrote the manuscript. IO-R performed lab experiments and data analyses. CL performed data analyses. IB performed lab experiments, data analyses, wrote the manuscript, and provided reagents and funding. AS designed the work, performed fieldwork, wrote the manuscript, and provided reagents and funding. JE-L designed the work, performed fieldwork, wrote the manuscript, and provided reagents and funding. All authors contributed to the article and approved the submitted version.
Funding
This work was supported by grants from the National Science Foundation (Grants 1810981 awarded to JE-L, AS, and IB; 1921402 awarded to JE-L, 1537959 awarded to IB; 1547798 awarded to Florida International University as part of the Centers for Research Excellence in Science and Technology Program). DG-S was supported by a postdoctoral contract ED481B/2018/091 (Xunta de Galicia). Corals were collected under Puerto Rico Marine Resources Department’s (DERM) permit # 2018-IC-034 O-VS-PVS15-SJ-00980-20042018.
Conflict of Interest
The authors declare that the research was conducted in the absence of any commercial or financial relationships that could be construed as a potential conflict of interest.
Acknowledgments
We are thankful to all the volunteers at FIU’s Environmental Epigenetics Laboratory, University of Puerto Rico, Rio Piedras and Sociedad Ambiente Marino who assisted during the early stages of this work. Thanks are also due to M. Devlin-Durante who performed the microsatellite genotyping and to FIU’s Seagrass lab for providing access to a submersible PAM sensor. This is contribution #204 from the Coastlines and oceans Division of the Institute of Environment at Florida International University.
Supplementary Material
The Supplementary Material for this article can be found online at: https://www.frontiersin.org/articles/10.3389/fmars.2020.560424/full#supplementary-material
Abbreviations
DO, dissolved oxygen; HMM, hemiMethylated target; HPM, hyperMethylated target; ICM, internal cytosine methylation in target; IGP, intraGenerational plasticity; ItGP, interGenerational plasticity; MSAP, methylation-sensitive amplification polymorphism; MSL, methylation-susceptible loci; NML, loci not susceptible to methylation; NMT, non-methylated target; PAR, photosynthetically active radiation; TGP, transGenerational plasticity.
Footnotes
References
Abraham, J. P., Baringer, M., Bindoff, N. L., Boyer, T., Cheng, L. J., Church, J. A., et al. (2013). A review of global ocean temperature observations: implications for ocean heat content estimates and climate change. Rev. Geophys. 51, 450–483. doi: 10.1002/rog.20022
Anderson, M. J. (2001). A new method for non-parametric multivariate analysis of variance. Austr. Ecol. 26, 32–46. doi: 10.1111/j.1442-9993.2001.01070.pp.x
Aranda, M., Li, Y., Liew, Y. J., Baumgarten, S., Simakov, O., Wilson, M. C., et al. (2016). Genomes of coral dinoflagellate symbionts highlight evolutionary adaptations conducive to a symbiotic lifestyle. Sci. Rep. 6:39734. doi: 10.1038/srep39734
Barshis, D. J., Ladner, J. T., Oliver, T. A., Seneca, F. O., Traylor-Knowles, N., and Palumbi, S. R. (2013). Genomic basis for coral resilience to climate change. Proc. Natl. Acad. Sci. U.S.A. 110, 1387–1392. doi: 10.1073/pnas.1210224110
Bastow, R., Mylne, J. S., Lister, C., Lippman, Z., Martienssen, R. A., and Dean, C. (2004). Vernalization requires epigenetic silencing of FLC by histone methylation. Nature 427, 164–167. doi: 10.1038/nature02269
Baums, I. B., Devlin-Durante, M. K., Polato, N. R., Xu, D., and Giri, S. (2013). Genotypic variation influences reproductive success and thermal stress tolerance in the reef building coral, Acropora palmata. Coral Reefs 32, 703–717. doi: 10.1007/s00338-013-1012-6
Baums, I. B., Hughes, C. R., and Hellberg, M. E. (2005a). Mendelian Microsatellite loci for the caribbean coral Acropora palmata. Mar. Ecol. Prog. Ser. 288, 115–127. doi: 10.3354/meps288115
Baums, I. B., Miller, M. W., and Hellberg, M. E. (2005b). Regionally isolated populations of an imperiled caribbean coral, Acropora palmata. Mol. Ecol. 14, 1377–1390. doi: 10.1111/j.1365-294x.2005.02489.x
Baums, I. B., Miller, M. W., and Hellberg, M. E. (2006). Geographic variation in clonal structure in a reef-building caribbean coral, Acropora palmata. Ecol. Monogr. 76, 503–519. doi: 10.1890/0012-9615(2006)076[0503:gvicsi]2.0.co;2
Benjamini, Y., and Hochberg, Y. (2000). On the adaptive control of the false discovery rate in multiple testing with independent statistics. J. Educ. Behav. Statist. 25, 60–83. doi: 10.2307/1165312
Berkelmans, R., and Willis, B. L. (1999). Seasonal and local spatial patterns in the upper thermal limits of corals on the inshore central great barrier reef. Coral Reefs 18, 219–228. doi: 10.1007/s003380050186
Birkeland, C. (2019). Global status of coral reefs: in combination, disturbances and stressors become ratchets. World Seas Environ. Eval. 2019, 35–56. doi: 10.1016/b978-0-12-805052-1.00002-4
Brady, A. K., Snyder, K. A., and Vize, P. D. (2011). Circadian cycles of gene expression in the coral, Acropora millepora. PLoS One 6:e25072. doi: 10.1371/journal.pone.0025072
Brener-Raffalli, K., Vidal-Dupiol, J., Adjeroud, M., Rey, O., Romans, P., Bonhomme, F., et al. (2019). Gene expression plasticity and frontloading promote Thermotolerance in Pocillopora corals. bioRxiv [Preprint], doi: 10.1101/398602
Brown, B. E., Dunne, R. P., Ambarsari, I., Le Tissier, M. D. A., and Satapoomin, U. (1999). Seasonal fluctuations in environmental factors and variations in symbiotic algae and chlorophyll pigments in four indo-pacific coral species. Mar. Ecol. Prog. Ser. 191, 53–69. doi: 10.3354/meps191053
Cai, L., Tian, R.-M., Zhou, G., Tong, H., Wong, Y. H., Zhang, W., et al. (2018). Exploring coral microbiome assemblages in the south China Sea. Sci. Rep. 8:2428.
Cai, W.-J., Ma, Y., Hopkinson, B. M., Grottoli, A. G., Warner, M. E., Ding, Q., et al. (2016). Microelectrode characterization of coral daytime interior pH and carbonate chemistry. Nat. Commun. 7:11144.
Carballo-Bolaños, R., Denis, V., Huang, Y.-Y., Keshavmurthy, S., and Chen, C. A. (2019). Temporal variation and photochemical efficiency of species in symbiodinaceae associated with coral Leptoria phrygia (Scleractinia; Merulinidae) exposed to contrasting temperature regimes. PLoS One 14:e0218801. doi: 10.1371/journal.pone.00218801
Chen, C. A., Yang, Y.-W., Wei, N. V., Tsai, W.-S., and Fang, L.-S. (2005). Symbiont diversity in scleractinian corals from tropical reefs and subtropical non-reef communities in taiwan. Coral Reefs 24, 11–22. doi: 10.1007/s00338-004-0389-7
Cortessis, V. K., Thomas, D. C., Levine, A. J., Breton, C. V., Mack, T. M., Siegmund, K. D., et al. (2012). Environmental epigenetics: prospects for studying epigenetic mediation of exposure-response relationships. Hum. Genet. 131, 1565–1589. doi: 10.1007/s00439-012-1189-8
Covelo-Soto, L., Saura, M., and Morán, P. (2015). Does DNA methylation regulate metamorphosis? The case of the sea lamprey (Petromyzon marinus) as an example. Compara. Biochem. Physiol. Part B Biochem. Mol. Biol. 185, 42–46. doi: 10.1016/j.cbpb.2015.03.007
Cziesielski, M. J., Schmidt-Roach, S., and Aranda, M. (2019). The past, present, and future of coral heat stress studies. Ecol. Evol. 9, 10055–10066. doi: 10.1002/ece3.5576
DeSalvo, M. K., Voolstra, C. R., Sunagawa, S., Schwarz, J. A., Stillman, J. H., Coffroth, M. A., et al. (2008). Differential gene expression during thermal stress and bleaching in the caribbean coral Montastraea faveolata. Mol. Ecol. 17, 3952–3971. doi: 10.1111/j.1365-294x.2008.03879.x
Díaz-Freije, E., Gestal, C., Castellanos-Martínez, S., and Morán, P. (2014). The role of DNA methylation on octopus vulgaris development and their perspectives. Front. Physiol. 5:62. doi: 10.3389/fmicb.2014.00062
Dimond, J., and Carrington, E. (2007). temporal variation in the symbiosis and growth of the temperate scleractinian coral Astrangia poculata. Mar. Ecol. Prog. Ser. 348, 161–172. doi: 10.3354/meps07050
Dimond, J. L., and Roberts, S. B. (2016). Germline DNA methylation in reef corals: patterns and potential roles in response to environmental change. Mol. Ecol. 25, 1895–1904. doi: 10.1111/mec.13414
Dimond, J. L., and Roberts, S. B. (2020). Convergence of DNA methylation profiles of the reef coral Porites astreoides in a novel environment. Front. Mar. Sci. 6:792. doi: 10.3389/fmicb.2014.00792
Dixon, G., Liao, Y., Bay, L. K., and Matz, M. V. (2018). Role of gene body methylation in acclimatization and adaptation in a basal metazoan. Proc. Natl. Acad. Sci. U.S.A. 115, 13342–13346. doi: 10.1073/pnas.1813749115
Dixon, G. B., Bay, L. K., and Matz, M. V. (2014). Bimodal signatures of Germline methylation are linked with gene expression plasticity in the coral Acropora millepora. BMC Genom. 15:1109. doi: 10.1186/1471-2156-11-1109
Drury, C., Greer, J. B., Baums, I., Gintert, B., and Lirman, D. (2019). Clonal diversity impacts coral cover in Acropora cervicornis thickets: potential relationships between density, growth, and polymorphisms. Ecol. Evol. 9, 4518–4531. doi: 10.1002/ece3.5035
Durante, M. K., Baums, I. B., Williams, D. E., Vohsen, S., and Kemp, D. W. (2019). What drives phenotypic divergence among coral clonemates of Acropora palmata? Mol. Ecol. 28, 3208–3224.
Edge, S. E., Morgan, M. B., and Snell, T. W. (2008). Temporal analysis of gene expression in a field population of the Scleractinian coral Montastraea faveolata. J. Exper. Mar. Biol. Ecol. 355, 114–124. doi: 10.1016/j.jembe.2007.12.004
Eirin-Lopez, J. M., and Putnam, H. M. (2019). Marine environmental epigenetics. Annu. Rev. Mar. Sci. 11, 335–368. doi: 10.1146/annurev-marine-010318-095114
Etchegaray, J.-P., and Mostoslavsky, R. (2016). Interplay between metabolism and epigenetics: a nuclear adaptation to environmental changes. Mol. Cell 62, 695–711. doi: 10.1016/j.molcel.2016.05.029
Feely, R. A., Doney, S. C., and Cooley, S. R. (2009). Ocean acidification: present conditions and future changes in a high-CO2 world. Oceanography 22, 36–47. doi: 10.5670/oceanog.2009.95
Finnegan, E. J., Genger, R. K., Kovac, K., Peacock, W. J., and Dennis, E. S. (1998). DNA Methylation and the promotion of flowering by vernalization. Proc. Natl. Acad. Sci. U.S.A. 95, 5824–5829. doi: 10.1073/pnas.95.10.5824
Fitt, W. K., McFarland, F. K., Warner, M. E., and Chilcoat, G. C. (2000). Seasonal patterns of tissue biomass and densities of symbiotic dinoflagellates in reef corals and relation to coral bleaching. Limnol. Oceanogr. 45, 677–685. doi: 10.4319/lo.2000.45.3.0677
Grünwald, N. J., and Goss, E. M. (2011). Evolution and population genetics of exotic and re-emerging pathogens: novel tools and approaches. Annu. Rev. Phytopathol. 49, 249–267. doi: 10.1146/annurev-phyto-072910-095246
Gu, Z., Eils, R., and Schlesner, M. (2016). Complex heatmaps reveal patterns and correlations in multidimensional genomic data. Bioinformatics 32, 2847–2849. doi: 10.1093/bioinformatics/btw313
Hansen, J., Sato, M., and Ruedy, R. (2012). Perception of climate change. Proc. Natl. Acad. Sci. U.S.A. 109, E2415–E2423.
Hill, R., and Ralph, P. J. (2005). Diel and seasonal changes in fluorescence rise kinetics of three Scleractinian corals. Funct. Plant Biol. 32, 549–559. doi: 10.1071/fp05017
Hinrichs, S., Patten, N. L., Allcock, R. J. N., Saunders, S. M., Strickland, D., and Waite, A. M. (2013). Seasonal variations in energy levels and metabolic processes of two dominant Acropora Species (A. Spicifera and A. Digitifera) at ningaloo reef. Coral Reefs 32, 623–635. doi: 10.1007/s00338-013-1027-z
Hoegh-Guldberg, O. (1999). Climate change, coral bleaching and the future of the world’s coral reefs. Mar. Freshw. Res. 50, 839–866.
Hoegh-Guldberg, O., and Bruno, J. F. (2010). The impact of climate change on the world’s marine ecosystems. Science 328, 1523–1528. doi: 10.1126/science.1189930
Hoegh-Guldberg, O., Mumby, P. J., Hooten, A. J., Steneck, R. S., Greenfield, P., Gomez, E., et al. (2007). Coral reefs under rapid climate change and ocean acidification. Science 318, 1737–1742.
Holm, S. (1979). A simple sequentially rejective multiple test procedure. Scand. J. Statist. Theor. Appl. 6, 65–70.
Hughes, T. P., Baird, A. H., Bellwood, D. R., Card, M., Connolly, S. R., Folke, C., et al. (2003). Climate change, human impacts, and the resilience of coral reefs. Science 301, 929–933. doi: 10.1126/science.1085046
Hume, B. C. C., Smith, E. G., Ziegler, M., Warrington, H. J. M., Burt, J. A., LaJeunesse, T. C., et al. (2019). SymPortal: a novel analytical framework and platform for coral algal symbiont next-generation sequencing ITS2 profiling. Mol. Ecol. Resour. 19, 1063–1080. doi: 10.1111/1755-0998.13004
Hume, B. C. C., Voolstra, C. R., Arif, C., D’Angelo, C., Burt, J. A., Eyal, G., et al. (2016). Ancestral genetic diversity associated with the rapid spread of stress-tolerant coral symbionts in response to Holocene climate change. Proc. Natl. Acad. Sci. U.S.A. 113, 4416–4421. doi: 10.1073/pnas.1601910113
Ito, T., Nishio, H., Tarutani, Y., Emura, N., Honjo, M. N., Toyoda, A., et al. (2019). Seasonal stability and dynamics of DNA methylation in plants in a natural environment. Genes. 10:544. doi: 10.3390/genes10070544
Jombart, T. (2008). Adegenet: a R package for the multivariate analysis of genetic markers. Bioinformatics. 24, 1403–1405. doi: 10.1093/bioinformatics/btn129
Jombart, T., and Collins, C. (2015). A tutorial for discriminant analysis of principal components (DAPC) using adegenet 2.0.0. Imp Coll Lond. MRC Cent Outbreak Anal. Model. 43, 1–43.
Jombart, T., Devillard, S., and Balloux, F. (2010). Discriminant analysis of principal components: a new method for the analysis of genetically structured populations. BMC Genet. 11:94. doi: 10.1186/1471-2156-11-94
Kenkel, C. D., Meyer, E., and Matz, M. V. (2013). Gene expression under chronic heat stress in populations of the mustard hill coral (Porites astreoides) from different thermal environments. Mol. Ecol. 22, 4322–4334. doi: 10.1111/mec.12390
Li, H. (2018). Minimap2: pairwise alignment for nucleotide sequences. Bioinformatics 34, 3094–3100. doi: 10.1093/bioinformatics/bty191
Li, J., Chen, Q., Long, L. J., Dong, J. D., Yang, J., and Zhang, S. (2014). Bacterial dynamics within the mucus, tissue and skeleton of the coral Porites lutea during different seasons. Sci. Rep. 4:7320.
Liew, Y. J., Howells, E. J., Wang, X., Michell, C. T., Burt, J. A., Idaghdour, Y., et al. (2020). Intergenerational epigenetic inheritance in reef-building corals. Nat. Clim. Chang. 10, 254–259. doi: 10.1038/s41558-019-0687-2
Liew, Y. J., Zoccola, D., Li, Y., Tambutté, E., Venn, A. A., Michell, C. T., et al. (2018). Epigenome-associated phenotypic acclimatization to ocean acidification in a reef-building coral. Sci. Adv. 4:eaar8028. doi: 10.1126/sciadv.aar8028
López-Maury, L., Marguerat, S. L., and Bähler, J. (2008). Tuning gene expression to changing environments: from rapid responses to evolutionary adaptation. Nat. Rev. Genet. 9, 583–593. doi: 10.1038/nrg2398
Marsh, A. G., and Pasqualone, A. A. (2014). DNA Methylation and temperature stress in an antarctic polychaete, Spiophanes tcherniai. Front. Physiol. 5:173. doi: 10.3389/fmicb.2014.00173
Martinez-Arbizu, P. (2019). PairwiseAdonis: Pairwise Multilevel Comparison Using Adonis. R Package Version 0.01.
Navarro-Martín, L., Viñas, J., Ribas, L., Díaz, N., Gutiérrez, A., Di Croce, L., et al. (2011). DNA Methylation of the Gonadal aromatase (cyp19a) promoter is involved in temperature-dependent sex ratio shifts in the European sea bass. PLoS Genet. 7:e1002447. doi: 10.1371/journal.pone.001002447
Oksanen, J., Blanchet, F. G., Friendly, M., Kindt, R., Legendre, P., McGlinn, D., et al. (2019). Vegan: Community Ecology Package. 2018. R Package Version 1.17.14.
Palumbi, S. R., Barshis, D. J., Traylor-Knowles, N., and Bay, R. A. (2014). Mechanisms of reef coral resistance to future climate change. Science 344, 895–898. doi: 10.1126/science.1251336
Pandolfi, J. M., Bradbury, R. H., Sala, E., Hughes, T. P., Bjorndal, K. A., Cooke, R. G., et al. (2003). Global trajectories of the long-term decline of coral reef ecosystems. Science 301, 955–958. doi: 10.1126/science.1085706
Parkinson, J. E., Bartels, E., Devlin-Durante, M. K., Lustic, C., Nedimyer, K., Schopmeyer, S., et al. (2018). Extensive transcriptional variation poses a challenge to thermal stress biomarker development for endangered Corals. Mol. Ecol. 27, 1103–1119. doi: 10.1111/mec.14517
Parkinson, J. E., and Baums, I. B. (2014). The extended phenotypes of marine symbioses: ecological and evolutionary consequences of intraspecific genetic diversity in coral-algal associations. Front. Microbiol. 5:445. doi: 10.3389/fmicb.2014.00445
Pegoraro, M., Bafna, A., Davies, N. J., Shuker, D. M., and Tauber, E. (2016). DNA Methylation changes induced by long and short photoperiods in Nasonia. Genome Res. 26, 203–210. doi: 10.1101/gr.196204.115
Perez, M. F., and Lehner, B. (2019). Intergenerational and transgenerational epigenetic inheritance in animals. Nat. Cell Biol. 21, 143–151. doi: 10.1038/s41556-018-0242-9
Pérez-Figueroa, A. (2013). Msap: a tool for the statistical analysis of Methylation-sensitive amplified polymorphism data. Mol. Ecol. Resour. 13, 522–527. doi: 10.1111/1755-0998.12064
Pochon, X., Pawlowski, J., Zaninetti, L., and Rowan, R. (2001). High genetic diversity and relative specificity among Symbiodinium-like Endosymbiotic dinoflagellates in soritid foraminiferans. Mar. Biol. 139, 1069–1078. doi: 10.1007/s002270100674
Pritchard, J. K., Stephens, M., Rosenberg, N. A., and Donnelly, P. (2000). Association mapping in structured populations. Am. J. Hum. Genet. 67, 170–181. doi: 10.1086/302959
Putnam, H. M., Davidson, J. M., and Gates, R. D. (2016). Ocean acidification influences host DNA Methylation and phenotypic plasticity in environmentally susceptible corals. Evol. Appl. 9, 1165–1178. doi: 10.1111/eva.12408
Putnam, H. M., Ritson-Williams, R., Cruz, J. A., Davidson, J. M., and Gates, R. D. (2020). Environmentally-induced parental or developmental conditioning influences coral offspring ecological performance. Sci. Rep. 10:13664. doi: 10.1038/s41598-020-70605-x
Reyna-Lopez, G. E., Simpson, J., and Ruiz-Herrera, J. (1997). Differences in DNA Methylation patterns are detectable during the dimorphic transition of fungi by amplification of restriction polymorphisms. Mol. Gen. Genet. 253, 703–710. doi: 10.1007/s004380050374
Rivera-Casas, C., Gonzalez-Romero, R., Garduño, R. A., Cheema, M. S., Ausio, J., and Eirin-Lopez, J. M. (2017). Molecular and biochemical methods useful for the epigenetic characterization of chromatin-associated proteins in Bivalve molluscs. Front. Physiol. 8:490. doi: 10.3389/fmicb.2014.00490
Rivière, G. (2014). Epigenetic features in the oyster Crassostrea gigas suggestive of functionally relevant promoter DNA Methylation in invertebrates. Front. Physiol. 5:129. doi: 10.3389/fmicb.2014.00129
Roberts, S. B., and Gavery, M. R. (2012). Is there a relationship between DNA Methylation and phenotypic plasticity in invertebrates? Front. Physiol. 2:116. doi: 10.3389/fphys.2011.00116
Rodriguez-Casariego, J. A., Ladd, M. C., Shantz, A. A., Lopes, C., Cheema, M. S., Kim, B., et al. (2018). Coral epigenetic responses to nutrient stress: histone H2A. X phosphorylation dynamics and DNA Methylation in the Staghorn coral Acropora cervicornis. Ecol. Evol. 8, 12193–12207. doi: 10.1002/ece3.4678
Rosenzweig, C., Karoly, D., Vicarelli, M., Neofotis, P., Wu, Q., Casassa, G., et al. (2008). Attributing physical and biological impacts to anthropogenic climate change. Nature 453, 353–357. doi: 10.1038/nature06937
Ryu, T., Veilleux, H. D., Donelson, J. M., Munday, P. L., and Ravasi, T. (2018). The epigenetic landscape of transgenerational acclimation to ocean warming. Nat. Clim. Chang. 8, 504–509. doi: 10.1038/s41558-018-0159-0
Salinas, S., and Munch, S. B. (2012). Thermal legacies: transgenerational effects of temperature on growth in a vertebrate. Ecol. Lett. 15, 159–163. doi: 10.1111/j.1461-0248.2011.01721.x
Sambrook, J., and Russell, D. W. (2006). Isolation of high-molecular-weight DNA from mammalian cells using proteinase K and phenol. Cold Spring Harb. Protoc. 15, 159–163. doi: 10.1101/pdb.prot4036
Samiei, J. V., Saleh, A., Shirvani, A., Fumani, N. S., Hashtroudi, M., and Pratchett, M. S. (2016). Variation in calcification rate of acroporadowningi relative to seasonal changes in environmental conditions in the northeastern Persian gulf. Coral Reefs 35, 1371–1382. doi: 10.1007/s00338-016-1464-6
Scheufen, T., Krämer, W. E., Iglesias-Prieto, R., and Enríquez, S. (2017). Seasonal variation modulates coral sensibility to heat-stress and explains annual changes in coral productivity. Sci. Rep. 7:4937.
Sharp, K. H., Pratte, Z. A., Kerwin, A. H., Rotjan, R. D., and Stewart, F. J. (2017). Season, but not symbiont state, drives microbiome structure in the temperate Coral Astrangia poculata. Microbiome 5:120.
Shinzato, C., Shoguchi, E., Kawashima, T., and Hamada, M. (2011). Using the Acropora digitifera genome to understand coral responses to environmental change. Nature 476, 320–323. doi: 10.1038/nature10249
Sorek, M., Díaz-Almeyda, E. M., Medina, M., and Levy, O. (2014). Circadian clocks in symbiotic corals: the duet between Symbiodinium algae and their coral host. Mar. Genom. 14, 47–57. doi: 10.1016/j.margen.2014.01.003
Stevenson, T. J., and Prendergast, B. J. (2013). Reversible DNA Methylation regulates seasonal photoperiodic time measurement. Proc. Natl. Acad. Sci. U.S.A. 110, 16651–16656. doi: 10.1073/pnas.1310643110
Stoddart, J. A., and Taylor, J. F. (1988). Genotypic diversity: estimation and prediction in samples. Genetics 118, 705–711.
Suarez-Ulloa, V., Gonzalez-Romero, R., and Eirin-Lopez, J. M. (2015). Environmental Epigenetics: a promising venue for developing next-generation pollution Biomonitoring tools in marine invertebrates. Mar. Pollut. Bull. 98, 5–13. doi: 10.1016/j.marpolbul.2015.06.020
Suarez-Ulloa, V., Rivera-Casas, C., and Michel, M. (2019). Seasonal DNA Methylation variation in the flat tree oyster isognomon Alatus from a mangrove ecosystem in North Biscayne Bay, Florida. J. Shellf. Res. 38:79. doi: 10.2983/035.038.0108
Thornhill, D. J., LaJeunesse, T. C., Kemp, D. W., Fitt, W. K., and Schmidt, G. W. (2006). Multi-year, seasonal genotypic surveys of coral-algal symbioses reveal prevalent stability or post-bleaching reversion. Mar. Biol. 148, 711–722. doi: 10.1007/s00227-005-0114-2
Tunnicliffe, V. (1981). Breakage and propagation of the stony coral Acropora cervicornis. Proc. Natl. Acad. Sci. U.S.A. 78, 2427–2431. doi: 10.1073/pnas.78.4.2427
Ulstrup, K. E., Hill, R., van Oppen, M. J. H., Larkum, A. W. D., and Ralph, P. J. (2008). Seasonal variation in the photo-physiology of homogeneous and heterogeneous Symbiodinium consortia in two Scleractinian corals. Mar. Ecol. Prog. Ser. 361, 139–150. doi: 10.3354/meps07360
van Oppen, M. J. H., Oliver, J. K., Putnam, H. M., and Gates, R. D. (2015). Building coral reef resilience through assisted evolution. Proc. Natl. Acad. Sci. U.S.A. 112, 2307–2313. doi: 10.1073/pnas.1422301112
Vandegehuchte, M. B., Kyndt, T., Vanholme, B., Haegeman, A., Gheysen, G., and Janssen, C. R. (2009). Occurrence of DNA Methylation in daphnia magna and influence of multigeneration Cd exposure. Environ. Intern. 35, 700–706. doi: 10.1016/j.envint.2009.01.002
Vignet, C., Joassard, L., Lyphout, L., Guionnet, T., Goubeau, M., Le Menach, K., et al. (2015). Exposures of zebrafish through diet to three environmentally relevant mixtures of PAHs Produce behavioral disruptions in unexposed F1 and F2 descendant. Environ. Sci. Pollut. Res. Intern. 22, 16371–16383. doi: 10.1007/s11356-015-4157-8
Viitaniemi, H. M., Verhagen, I., Visser, M. E., Honkela, A., van Oers, K., and Husby, A. (2019). Seasonal variation in genome-Wide DNA Methylation patterns and the onset of seasonal timing of reproduction in great tits. Genome Biol. Evol. 11, 970–983. doi: 10.1093/gbe/evz044
Voolstra, C. R., Schnetzer, J., Peshkin, L., Randall, C. J., Szmant, A. M., and Medina, M. (2009). Effects of temperature on gene expression in embryos of the coral Montastraea faveolata. BMC Genom. 10:627. doi: 10.1186/1471-2156-11-627
Warner, M., Chilcoat, G., McFarland, F., and Fitt, W. (2002). Seasonal fluctuations in the photosynthetic capacity of Photosystem II in symbiotic dinoflagellates in the caribbean reef-building coral montastraea. Mar. Biol. 141, 31–38. doi: 10.1007/s00227-002-0807-8
Weil, E., Hernández-Delgado, E., Gonzalez, M., Williams, S., Ramos, S. Suleimán, Figuerola, M., et al. (2019). Spread of the new coral disease ‘SCTLD’ into the Caribbean: implications for Puerto Rico. Reef Encount. 34, 38–43.
West-Eberhard, M. J. (2003). Developmental Plasticity and Evolution. Oxford: Oxford University Press.
Keywords: staghorn coral, DNA methylation, MSAP, stress response, temperature, seasonality, epigenetics
Citation: Rodríguez-Casariego JA, Mercado-Molina AE, Garcia-Souto D, Ortiz-Rivera IM, Lopes C, Baums IB, Sabat AM and Eirin-Lopez JM (2020) Genome-Wide DNA Methylation Analysis Reveals a Conserved Epigenetic Response to Seasonal Environmental Variation in the Staghorn Coral Acropora cervicornis. Front. Mar. Sci. 7:560424. doi: 10.3389/fmars.2020.560424
Received: 08 May 2020; Accepted: 07 September 2020;
Published: 30 September 2020.
Edited by:
Yong Wang, Institute of Deep-Sea Science and Engineering (CAS), ChinaReviewed by:
Alexandre Fellous, Alfred Wegener Institute Helmholtz Centre for Polar and Marine Research (AWI), GermanyMikhail V. Matz, University of Texas at Austin, United States
Hui Huang, South China Sea Institute of Oceanology (CAS), China
Copyright © 2020 Rodríguez-Casariego, Mercado-Molina, Garcia-Souto, Ortiz-Rivera, Lopes, Baums, Sabat and Eirin-Lopez. This is an open-access article distributed under the terms of the Creative Commons Attribution License (CC BY). The use, distribution or reproduction in other forums is permitted, provided the original author(s) and the copyright owner(s) are credited and that the original publication in this journal is cited, in accordance with accepted academic practice. No use, distribution or reproduction is permitted which does not comply with these terms.
*Correspondence: Jose M. Eirin-Lopez, jeirinlo@fiu.edu