Vertical Niche Partitioning of Archaea and Bacteria Linked to Shifts in Dissolved Organic Matter Quality and Hydrography in North Atlantic Waters
- 1Centro Oceanográfico de A Coruña, Instituto Español de Oceanografia-Centro Superior de Investigaciones Cientificas (IEO-CSIC), A Coruña, Spain
- 2Instituto de Investigacións Mariñas, Centro Superior de Investigaciones Cientificas (CSIC), Vigo, Spain
- 3Institut de Ciènces del Mar, Centro Superior de Investigaciones Cientificas (CSIC), Barcelona, Spain
Understanding the factors that modulate prokaryotic assemblages and their niche partitioning in marine environments is a longstanding challenge in marine microbial ecology. This study analyzes amplicon sequence variant (ASV) diversity and co-occurrence of prokaryotic (Archaea and Bacteria) communities through coastal-oceanic gradients in the NW Iberian upwelling system and adjacent open-ocean (Atlantic Ocean). Biogeographic patterns were investigated in relation with environmental conditions, mainly focusing on the optical signature of the dissolved organic matter (DOM). Alpha- and beta-diversity were horizontally homogeneous [with the only exception of Archaea (∼1700 m depth), attributed to the influence of Mediterranean water, MW], while beta-diversity was significantly vertically stratified. Prokaryotic communities were structured in four clusters (upper subsurface, lower subsurface, intermediate, and deep clusters). Deep (>2000 m) archaeal and bacterial assemblages, and intermediate (500-2000 m) Bacteria (mainly SAR202 and SAR406), were significantly related to humic-like DOM (FDOM-M), while intermediate Archaea were additionally related to biogeochemical attributes of the high-salinity signature of MW. Lower subsurface (100-500 m) Archaea (particularly one ASV belonging to the genus Candidatus Nitrosopelagicus) were mainly related to the imprint of high-salinity MW, while upper subsurface (≤100 m) archaeal assemblages (particularly some ASVs belonging to Marine Group II) were linked to protein-like DOM (aCDOM254). Conversely, both upper and lower subsurface bacterial assemblages were mainly linked to aCDOM254 (particularly ASVs belonging to Rhodobacteraceae, Cyanobacteria, and Flavobacteriaceae) and nitrite concentration (mainly members of Planctomycetes). Most importantly, our analysis unveiled depth-ecotypes, such as the ASVs MarG.II_1 belonging to the archaeal deep cluster (linked to FDOM-M) and MarG.II_2 belonging to the upper subsurface cluster (related to FDOM-T and aCDOM254). This result strongly suggests DOM-mediated vertical niche differentiation, with further implications for ecosystem functioning. Similarly, positive and negative co-occurrence relationships also suggested niche partitioning (e.g., between the closely related ASVs Thaum._Nit._Nit._Nit._1 and _2) and competitive exclusion (e.g., between Thaum._Nit._Nit._Nit._4 and _5), supporting the finding of non-randomly, vertically structured prokaryotic communities. Overall, differences between Archaea and Bacteria and among closely related ASVs were revealed in their preferential relationship with compositional changes in the DOM pool and environmental forcing. Our results provide new insights on the ecological processes shaping prokaryotic assembly and biogeography.
Introduction
Marine heterotrophic microbes depend upon dissolved organic matter (DOM) quantity and quality to fulfill their metabolic requirements, as they recycle dissolved organic carbon through the microbial loop (Azam et al., 1983). Many recent studies showed the relationship between the structure and function of microbial communities and environmental forcing (Lindh and Pinhassi, 2018; Auladell et al., 2019; Mena et al., 2020). Indeed, several investigations have displayed vertical stratification [water mass- (Agogué et al., 2011) or depth realm-specificity (Dobal-Amador et al., 2016; Guerrero-Feijóo et al., 2017)] of microbial populations in the Atlantic Ocean attributed to the vertical variability of the hydrographic features in the pelagic environment (Agogué et al., 2011; Dobal-Amador et al., 2016; Zorz et al., 2019). However, only a few studies have taken into account the potential role of DOM quality (Amaral et al., 2016; Dobal-Amador et al., 2016; Guerrero-Feijóo et al., 2017), characterized by its optical properties, as a key parameter in modeling aquatic microbial communities due to two main limitations. On the one hand, our knowledge about the composition of the DOM, especially at intermediate and deep waters, is pretty limited (Bergauer et al., 2018). On the other, concurrent data about prokaryotic diversity and DOM quantity and quality indicators are extraordinarily scarce in oceanographic surveys (Dobal-Amador et al., 2016; Guerrero-Feijóo et al., 2017), so virtually all the available information comes from mesocosm and/or experimental approaches (e.g., Omori et al., 2020; Varela et al., 2020; Gutiérrez-Barral et al., 2021). These empirical methods are of great value since they provide important information on the behavior of different populations under controlled conditions. However, their results are only cautiously comparable with natural populations due to the so-called “bottle effect” (Zobell and Anderson, 1936).
The seasonal pattern of water column stratification, typical of temperate regions, is masked in the Atlantic Ocean off the western Galician coast by frequent upwelling pulses (the so-called NW Iberian upwelling system) between March and September-October (Casas et al., 1997), interrupted by periods of relaxation of winds or downwelling (Bode et al., 2019). As a result, there is an accumulation of autotrophically synthetized organic compounds which support the export offshore and sinking fluxes of organic matter (Bode et al., 1998; Álvarez-Salgado et al., 2008). Comparatively, in the Cantabrian coast (Bay of Biscay), located in the eastern limit of the upwelling region, these upwelling events usually last for a shorter time and reach lower intensities than off the Galician coast (Lavin et al., 2006). The few previous exploratory studies on the Galician coast indicated that bacterial community structure, assessed by automated rRNA intergenic spacer analysis (ARISA) fingerprinting (Dobal-Amador et al., 2016) and by terminal restriction fragment length polymorphism (T-RFLP) (Guerrero-Feijóo et al., 2017), was related to hydrographic parameters and DOM quality indices. However, the low diversity resolution provided by those methods did not allow testing for specific relationships among particular taxa and DOM. Moreover, to the best of our knowledge, there is no previous concurrent characterization of DOM quantity and quality and prokaryotic community composition in the Bay of Biscay.
High-throughput sequencing (HTS) technologies revealed that nominal estimates of marine microbial richness are at least one order of magnitude higher than previously estimated (Pedrós-Alió et al., 2018). Besides, Amplicon Sequence Variants (ASVs) can be resolved exactly down to the level of single-nucleotide differences over the sequenced gene region (Callahan et al., 2017), giving rise to improved diversity estimates based on their finer resolution. Thus, even if we do not know the identity of a given ASV, we can be sure that it is certainly different from all the others in the dataset. This feature allows for a more accurate analysis of diversity patterns and co-occurrence among the components of microbial assemblages (Needham et al., 2017), as well as identifying relationships among particular ASVs and environmental variables, revealing different ecological patterns within a specific taxon (i.e., ecotypes), which might potentially affect organic matter cycling.
It has been recently suggested that abiotic factors have a more limited effect than biotic interactions on determining plankton community structure (Lima-Mendez et al., 2015; Milici et al., 2016). Then, ecosystem function and dynamics would depend upon the association of taxa playing ecologically distinct roles and their interactions with other taxa and the environment across space (Koeppel et al., 2008). Negative co-occurrence (i.e., the presence of one phylotype implies the absence of the other), might result from differences in habitat preference (ecotypes) or competitive exclusion (Milici et al., 2016). Conversely, positive co-occurrence suggests collaborative phylogenetic associations (mutualism) (Coutinho et al., 2015) or, alternatively, that organisms can avoid competition by their dissimilar physiologies, resources requirements and/or capability of response to environmental forcing. If so, they do not share ecological niche (niche partitioning) and thus they can share habitat (Barberán et al., 2012).
This study aimed to explore the biotic and abiotic interactions potentially driving prokaryotic community assembly along two coastal-oceanic gradients in the North Atlantic Ocean off the Iberian Peninsula (0-5000 m depth). We hypothesize that the studied areas, with different hydrographic features and exposed to different levels of upwelling intensity, would presumably support different prokaryotic communities. Accordingly, it is hypothesized that distinctive DOM quantity and quality and hydrographic conditions will drive prokaryotic niche partitioning, and the differentiation of ecotypes for closely related ASVs/phylotypes.
Materials and Methods
Sampling and Hydrography
Seawater samples were collected during MODUPLAN cruise (4th to 24th August 2014) on board R/V Sarmiento de Gamboa along two transects (9 stations each) off the Galicia and Cantabria coasts [Fisterra (43°N, 9°W to 43°N, 14°W) and Santander (43.30°N, 3.47°W to 44.50°N, 3.47°W); Figure 1], using 12-L Niskin bottles attached to a CTD-rosette sampler. Sampling depths were selected based on distinctive temperature, salinity, and dissolved oxygen concentration signals through the water column (0-5000 m) (Prieto et al., 2013). Seawater samples for microbial analyses and for DOM characterization were collected in acid-washed polycarbonate carboys (Nalgene, Thermo Fisher Scientific) and 250-mL acid-washed glass bottles, respectively.
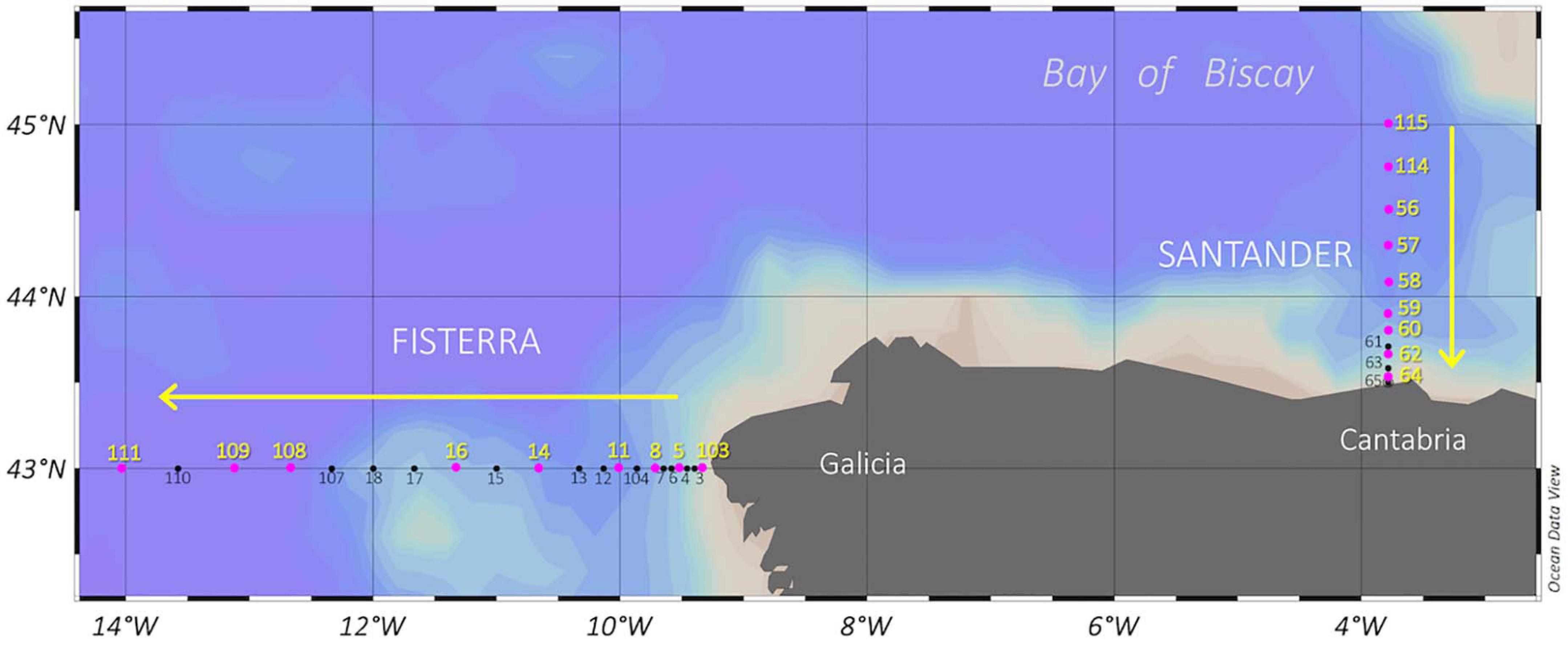
Figure 1. Sampling stations during MODUPLAN cruise along Fisterra and Santander sections, off Galicia coast and in the Bay of Biscay, respectively, off the NW Iberian Peninsula. The hidrographic parameters were determined in all stations (black dots), while pink dots indicate biological stations where, additionally, prokaryotic community composition and DOM were characterized. Fisterra transect took place from August 04, 2014 to August 12, 2014, while Santander transect took place from August 14, 2014 to August 21, 2014. Arrows indicate sampling direction.
Temperature and salinity profiles were recorded in situ alongside the bottle samples collection using CTD sensors. Samples for the analysis of dissolved oxygen concentration were collected in 115-mL Pyrex “iodine titration” flasks with flared necks and ground glass stoppers. Oxygen concentration was determined by the Winkler potentiometric method (Langdon, 2010). At the same depths, unfiltered samples for the determination of dissolved inorganic nutrients (nitrate, nitrite, and phosphate) were collected in rinsed 15-mL polyethylene tubes and frozen at -20°C until the analysis by standard colorimetric methods on a segmented flow analyzer (Bran-Luebbe), following the procedures of Hansen and Koroleff (2007).
Upwelling intensity (UI, m3 s–1 km–1) was obtained from the Ekman transport derived from surface winds for the studied areas1. Positive values of UI indicate net upwelling periods when surface water is transported offshore, while negative values indicate an accumulation of surface water against the coast (downwelling). The squared Brunt-Väisälä frequency (N2), a proxy for water column stratification, was computed from the CTD data by the function swN2 within oce package (Kelley and Richards, 2019) in R Core Team (2017). The mixed layer depth (MLD) was estimated as the depth where seawater density was 0.125 kg m–3 higher than the surface value.
DOM Optical Signature
All DOM samples above 200 m were filtered under positive N2 pressure using an acid-clean all-glass system and combusted (450°C, 4 h) GFF filters. Water samples for the analysis of dissolved organic carbon (DOC) were collected in combusted (450°C, 4 h) glass ampoules, and acidified with H3PO4 to pH < 2. The ampoules were heat-sealed. DOC concentrations were determined using a Shimadzu TOC-VCSH analyzer by high-temperature Pt-catalytic oxidation (Álvarez-Salgado and Miller, 1998). Samples were calibrated daily with potassium hydrogen phthalate (99.95-100.05%, p.a., Merck) and the precision of the measurements was 1 μmol C L–1. The accuracy of the system was checked with the reference samples supplied by D.A. Hansell (University of Miami, United States). The fluorescence of DOM (FDOM) was measured on board (within 5 h after sampling) at two pairs of fixed excitation/emission wavelengths: (i) 320/410 nm (FDOM-M), characteristic of marine humic-like substrates, and (ii) 280/350 nm (FDOM-T), characteristic of protein-like compounds, using a Perkin Elmer LS55 following Nieto-Cid et al. (2006). Samples were calibrated against quinine sulfate and results are given in quinine sulfate units (QSU). UV-visible absorption spectra of the chromophoric DOM (CDOM) were acquired using a double beam Perkin Elmer lambda 850 spectrophotometer equipped with 10-cm path length quartz cuvettes for sample and reference (ultrapure water, Milli-Q, Millipore Advantage A10). Spectral scans were recorded from 250 to 700 nm at 1 nm intervals and constant temperature, using the sample average absorbance between 600 and 700 nm to correct for offsets (Green and Blough, 1994). Absorbance was converted into Naeperian absorption coefficients (m–1) at several wavelengths along the spectra (Green and Blough, 1994): aCDOM254 (absorption coefficient at 254 nm), aCDOM340 (absorption coefficient at 340 nm), and aCDOM365 (absorption coefficient at 365 nm). Differences between these indices lay on the nature of the colored DOM, as the intensification of the conjugation/aromaticity increases with the absorption wavelength (Stedmon and Nelson, 2015; Catalá et al., 2018). The spectral slope between 275 and 295 nm [s(275-295)] provides information on shifts in molecular mass and DOM aromaticity (Helms et al., 2008; Catalá et al., 2018).
DNA Extraction, Sequencing, and Bioinformatics
A total of 127 seawater samples for DNA extraction were collected at 18 stations (9 stations per section) throughout the water column. Prokaryotic cells were concentrated by filtering ∼10 L of 200-μm prefiltered seawater through sterile, 0.22 μm pore-size Sterivex filters (Millipore, United States), and preserved with 1.8 mL of lysis buffer (40 mM EDTA, 50 mM Tris-HCl, 0.75 M sucrose). The cartridges were flash-frozen in liquid nitrogen and stored at -80°C. DNA extraction was performed according to Massana et al. (1997) with slight modifications (Guerrero-Feijóo et al., 2017). Cell lysis was performed by 45-min digestion with lysozyme (1 mg mL–1 final concentration) at 37°C, followed by 60-min proteinase K digestion (0.2 mg mL–1 final concentration) with sodium dodecyl sulfate (SDS) (10%) at 55°C. Then, DNA was extracted twice in phenol:chlorophorm:IAA (25:24:1) and once in chlorophorm:IAA (24:1). The extracted DNA was concentrated using an Amicon Ultracel 100k filter unit (Millipore). Concentration and purity were determined according to the absorbance A260/A280 ratio using a Nanodrop spectrophotometer (Thermo Fisher Scientific, United States). Nucleic acid extracts were stored at -20°C until further analysis.
The V3 to V4 regions of the 16S rRNA gene were amplified by polymerase chain reaction (PCR), using the primer pairs 349F-806R (Klindworth et al., 2013) for Archaea, and 341F-805R (Herlemann et al., 2011) for Bacteria. The 20-μL PCR mixture contained 2 μL of the corresponding primer set (1 μL and 10 μM each), 2 μL 10 × PCR Buffer (Invitrogen), 1.2 μL MgCl2 (25 mM), 0.4 μL dNTP (10 mM), 1.25 U Taq polymerase (Platinum, Invitrogen), and 1 μL of DNA templates (approximately 20 ng), completed with sterilized ultrapure water. PCR amplification was performed in a Mastercycler (Eppendorf), implementing the same cycling conditions for archaeal and bacterial DNA samples (94°C, 5 min; 30 cycles of 94°C, 1 min; 57.5°C, 1 min; 72°C, 2 min, and 72°C, 10 min). All PCR products were checked for quality control on 1% agarose gel electrophoresis, purified with 5-Prime ArchivePure purification kit (Thermo Fisher Scientific) and kept at -20°C until further analysis.
The purified PCR-products were subjected to Illumina Mi-seq high-throughput sequencing (2 × 250 bp, IMGM Laboratories, Germany2). Paired Illumina reads were processed through the high-performance supercomputing resources of the Centro Tecnolóxico de Supercomputación de Galicia (CESGA) as follows: primers and spurious sequences were trimmed using cutadapt (Martin, 2011). Exact amplicon sequence variants (ASVs) were differentiated by implementing dada2 (Callahan et al., 2016) in R Core Team (2017). The approach is threshold free, inferring exact variants up to one nucleotide of difference using the Q scores in a probability model. Sequences were aligned against SILVA 132 16S rRNA database (Quast et al., 2013) as reference. Finally, singletons (ASVs found only once in the final ASV table) were excluded, as they have been shown to likely be the result of PCR or sequencing errors (Huse et al., 2010).
Quantification of Prokaryotic Cells by CARD-FISH
Catalyzed reporter deposition fluorescence in situ hybridization (CARD-FISH) was implemented to quantify the abundance of major prokaryotic groups (Bacteria, Thaumarchaeota, and Euryarchaeota) at selected stations (8, 11, 16, and 111 in Fisterra, and 60, 57 and 115 in Santander), as previously described in Guerrero-Feijóo et al. (2017). Briefly, immediately after sampling, 20–80 mL of seawater were preserved with paraformaldehyde (2% final concentration) and stored at 4°C in the dark. After 12–18 h, samples were filtered onto 0.2 μm polycarbonate filters (Millipore GTTP, 25 mm filter diameter) supported by nitrocellulose filters (Millipore, HAWP, 0.45 μm). Filters were washed twice with 10 mL of Milli-Q water, dried and stored in microcentrifuge vials at –20°C. Then, filters were cut in sections and hybridized with horseradish peroxidase (HRP)-labeled oligonucleotide probes (Supplementary Table 1), followed by tyramide-Alexa488 signal amplification. Cells were counter-stained with a DAPI-mix (DAPI final concentration 2 μg mL–1). Quantification of stained cells was carried out under a Nikon Eclipse 80i epifluorescence microscope equipped with Hg lamp and appropriate filter sets for DAPI and Alexa448. A minimum of 500 DAPI-stained cells were counted per sample.
Statistical Analysis
All statistical analyses were performed in R Core Team (2017). Whether significant differences existed for the environmental variables (temperature, salinity and oxygen concentration, DOC concentration, and DOM optical properties), as well as the relative contributions of prokaryotic groups assessed by CARD-FISH, was tested between transects (Fisterra and Santander) through Student’s t-test, and among depth realms (epi- [<200 m], meso- [200-1000 m], and bathypelagic [>1000 m]) and different sampling depth ranges (≤100 m, 250-700 m, 800-1200 m, 1800-2000 m, 2300-2750 m, ≥3000 m) by One-Way ANOVA and Tukey’s HSD tests. After removing collinear variables, determined by regression analysis (R2 > 0.7, p < 0.001), the final environmental matrix for further analysis was composed by aCDOM254, FDOM-T, FDOM-M, s(275-295), salinity (SAL), and nitrite concentration (NO2–).
To compare the diversity of samples obtained from different sampling efforts (Magurran, 2004), reads were resampled by rarefaction at the minimum number of reads per sample (9718 reads for Archaea and 2002 reads for Bacteria). The percentage of coverage accounted by rarefied data with respect to bulk diversity was calculated by the Good’s coverage estimator (Good, 1953).
From rarefied data, ASV richness [observed (SObs), and estimated by the non-parametric model Chao1 (SChao1) (Chao, 1984)], and Shannon and Simpson diversity indices were calculated. Venn diagrams were built using the R package VennDiagram (Chen and Boutros, 2011). The spatial variability of archaeal and bacterial ASV richness and diversity were explored by determining their correlation with (a) the distance to the coast (km) (horizontal variability) and (b) depth (m) (vertical variability), with MINE function (Reshef et al., 2011). For the significant correlations, linear and quadratic models were fitted, and the best-fitted model was selected according to the lowest Akaike’s information criterion (AIC; Burnham and Anderson, 2002). The rate of horizontal and vertical change for ASV richness and diversity was derived from the slope of the best fitted models. The correlation between ASV richness and diversity with respect to the environmental variables was determined by Spearman’s correlation test.
Bray-Curtis dissimilarity matrices were calculated after Hellinger transformation of rarefied reads per sample (Legendre and Gallagher, 2001), which gives low weights to variables with low counts and many zeroes (Ramette, 2007; Paliy and Shankar, 2016). An analysis of similarities (ANOSIM) was performed to test the null hypothesis that there were no differences in community assemblages either between sections, or among stations, depth ranges or depth realms within each section. The global R in ANOSIM represents the separation degree between groups; R = 0 indicates no separation (completely random grouping), whereas R = 1 suggests complete separation (Oksanen et al., 2012). SIMPER analysis was implemented to identify the ASVs which mostly accounted for the dissimilarity among samples. Hierarchical clustering analysis was used to examine multivariate similarities among the communities.
Due to the high ASV richness found, relative abundance, co-occurrence and RDA analyses were based on “abundant phylotypes,” those representing more than 1% of the total number of reads in the corresponding data set (Archaea and Bacteria). They can be individual ASVs, or groups of rare ASVs pooled at higher taxonomic-rank levels (e.g., rare ASVs within the same genus, rare genus within the same order, etc.) until reaching relative abundance > 1%. Phylotypes with relative abundance lower than 1% after adding up at phylum level were pooled in “Others.”
A pair-wise analysis was implemented (cooccur package; Griffith et al., 2016) to determine pairs of archaeal and bacterial abundant phylotypes with significant (p < 0.05) associations. The probabilistic model of species co-occurrence (Veech, 2013) determines the probability that the observed frequency of co-occurrence is significantly large and greater than expected (positive association), significantly small and less than expected (negative association), or not significantly different and approximately equal to expected (random association). Finally, redundancy analysis (RDA) was performed to assess how much of the variation in the dissimilarity matrix could be explained by the variation in the final set of non-correlated environmental variables.
Results
Hydrographic Conditions
Sampling depths were chosen according to differences in the hydrographic parameters (Table 1 and Supplementary Figure 1) throughout the water column (0-5000 m). Maximum and minimum temperatures were found at surface and bottom waters, respectively, in both transects. Salinity showed minimum values at the deep bottom in Fisterra and at the surface in Santander, while maximum salinity, higher in Fisterra than in Santander, was found in both sections at ∼1000 m depth, in association with minimum O2 concentrations. In general, significant differences (ANOVA, p < 0.001 and Tukey’s test, p < 0.001) were found for temperature, salinity and O2 concentration among epi-, meso-, and bathypelagic realms in both sections, and among the depth ranges except for the homogeneous bathypelagic realm (i.e., no significant differences were found among 1800-2000 m, 2300-2750 m, and ≥3000 m depth ranges) (Supplementary Figure 1).
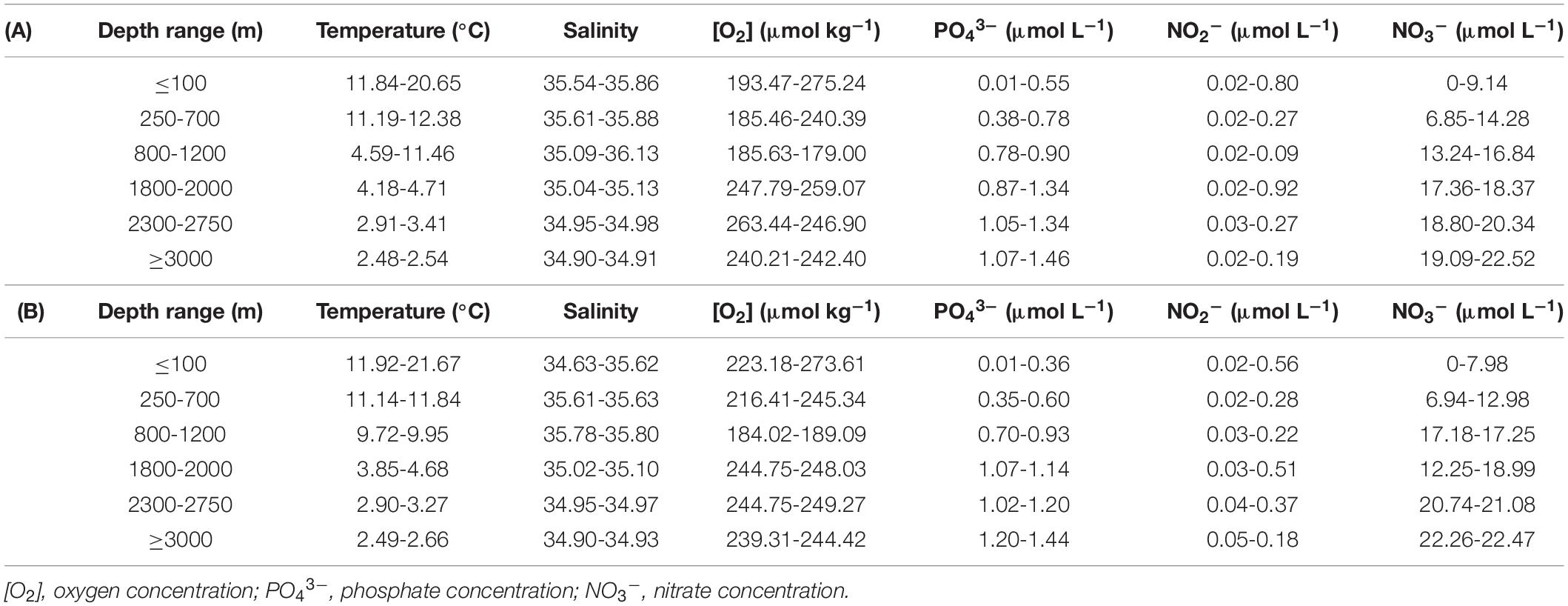
Table 1. Range of variability (minimum-maximum) of the hydrographic features at the different sampling depth ranges during MODUPLAN cruise along (A) Fisterra and (B) Santander sections.
In Fisterra, upwelling conditions before the cruise (Supplementary Figure 2A) resulted in the development of a filament, which exported chlorophyll-enriched waters towards offshore stations (Supplementary Figure 3A). Fisterra section was sampled during the relaxation of this upwelling episode, resulting in the onshore movement of the superficial front between warmer offshore waters (around the 18°C isotherm) and upwelled cold coastal waters. In Santander, the exportation of high-chlorophyll waters from the French shelf partially reached our hydrographical section (Supplementary Figure 3B). Stations closer to the coast were sampled at the end of the cruise, when favorable conditions induced coastal upwelling (Supplementary Figure 2B), shown by the steeply sloping of the isotherms (Supplementary Figure 1A). The squared Brunt-Väissälä frequency (N2) profiles (Supplementary Figure 4) revealed stratified conditions in the upper water column, with maximum stratification (highest N2 values) underneath the mixed layer depth, which was shallow along the two transects (∼5 m at coastal stations, increasing up to only >20 m at offshore stations and station 62 in the Santander section), and above ∼100 m depth. The concentrations of the inorganic nutrients (nitrate, nitrite, and phosphate) showed similar vertical profiles at both sections (Table 1; Supplementary Figure 5; and Supplementary Table 2).
Spatial (Horizontal and Vertical) Variability of DOM
Mean values of protein-like DOM (FDOM-T) (Figure 2B), humic-like substances (FDOM-M) (Figure 2C) and the absorption index at 340 nm (aCDOM340) (Figure 2E) were significantly higher in Santander than in Fisterra (Student’s t-Test, p < 0.05), while no significant differences were found between sections for DOC (Figure 2A), aCDOM254 (Figure 2D) and aCDOM365 (Figure 2F), and the slope s(275-295) (Figure 2G). DOC and the DOM optical indices tended to be higher in coastal than in offshore stations, and to decrease from surface to deep waters, in both sections. Only the fluorescence of marine humic-like substances (FDOM-M) was higher in deep than in subsurface waters, except for coastal stations at Santander section, where it showed maximum values in subsurface waters. One-way ANOVA and post hoc Tukey’s test (Supplementary Figure 6) revealed that meso- and bathypelagic depth realms were similar to each other in terms of the DOM optical signature, but significantly different to the epipelagic realm. The exception was the spectral slope s(275-295), showing no significant differences throughout the water column.
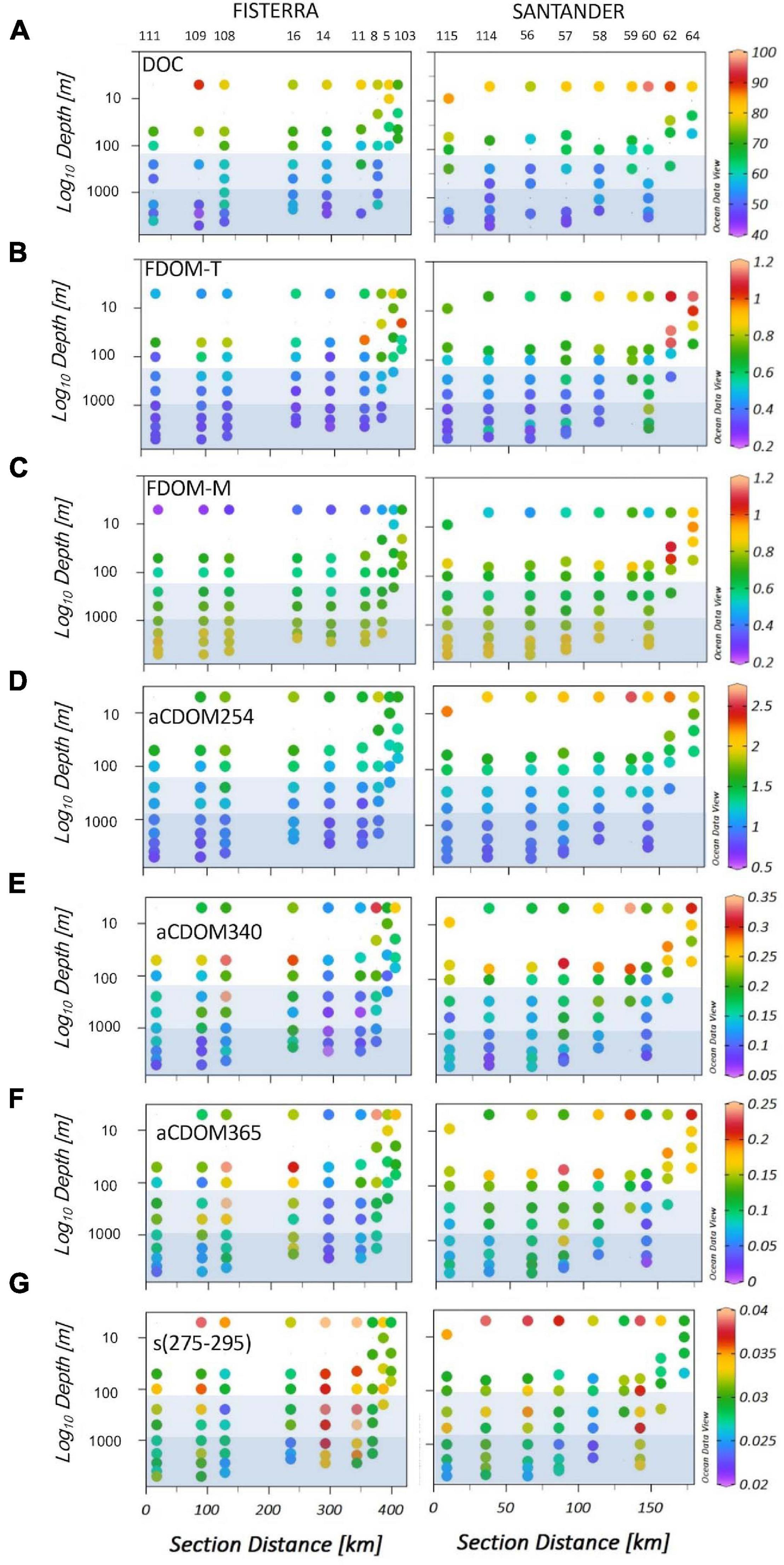
Figure 2. Spatial distribution of DOM variables along Fisterra (left) and Santander (right) sections, in the NW Iberian upwelling system and adjacent open-ocean: (A) Dissolved organic carbon concentration (DOC, μmol C L–1), (B) FDOM-T (QSU), fluorescence of labile protein-like DOM, (C) FDOM-M (QSU), fluorescence of humic-like compounds, (D) aCDOM254 (m–1), the absorbance of DOM at 254 nm; (E) aCDOM340 (m–1), the absorbance of DOM at 340 nm; (F) aCDOM365 (m–1), the absorbance of DOM at 365 nm; and (G) s(275-295), the 275-295 nm spectral slope. Light and dark blue squares indicate respectively meso- (201-1000 m) and bathypelagic (>1000 m) realms. Numbers overhead denote the sampling stations.
Abundance of the Major Prokaryotic Groups Assessed by CARD-FISH
In Fisterra (Supplementary Table 3A), the average contributions of Bacteria, Thaumarchaeota, and Euryarchaeota were not significantly different among depth realms (ANOVA, p > 0.05), accounting for ∼60, ∼13, and ∼5% of the total prokaryotic community through the water column, respectively. In Santander (Supplementary Table 3B), Bacteria represented on average 66% of total prokaryotes through the water column (ANOVA, p > 0.05), Thaumarchaeota contributed significantly less in epi- (∼9%) than in meso- (∼16%) and bathypelagic waters (∼16%) (ANOVA, p < 0.05 and post hoc Tukey’s test, p < 0.01), while Euryarchaeota showed the opposite pattern, with a significantly higher contribution in epi- (∼9%) than in meso- (∼6%) and bathypelagic (∼4%) realms (ANOVA, p < 0.05 and Tukey’s test, p < 0.01).
Prokaryotic ASV Richness and Diversity
After rarefaction, 1078698 sequences for Archaea and 254254 sequences for Bacteria were saved and classified into 855 archaeal ASVs (SAObs) and 3928 bacterial ASVs (SBObs). Averaged Good’s coverage estimates were 99.9 and 97.5% for Archaea and Bacteria, respectively, with respect to bulk prokaryotic communities (Supplementary Table 4), showing that our rarefied data successfully unveiled the diversity of the studied communities. Fisterra section harbored a higher number of unique ASVs (not found in Santander) than Santander, although most of the archaeal and bacterial ASVs (∼84% of SAObs and ∼71% of SBObs) were shared between the two sections (Supplementary Figure 7, left panel). For Archaea (Supplementary Figure 7, upper panel), ∼49% of the ASVs were shared among depth realms, although bathypelagic waters harbored the highest number of unique ASVs (96 ASVs, 11.2% of SAObs, found only at this depth realm). Compared to Archaea, only a moderate ∼22% of SBObs was shared among depth realms for bacterial communities (Supplementary Figure 7, bottom panel). The highest number of unique bacterial ASVs (930, 22.4% of SBObs) was found also in bathypelagic waters.
Spatial Patterns of Diversity and Structure of Prokaryotic Assemblages
Prokaryotic alpha-diversity showed no relationship with the distance from the coast, but it was significantly correlated with depth (Supplementary Figure 8 and Supplementary Table 5). Archaeal ASV richness (MIC = 0.527, p < 0.001) and Shannon diversity index (MIC = 0.623, p < 0.001) increased linearly with depth. For Bacteria, only the Shannon index showed a significant quadratic relationship with depth (MIC = 0.336, p < 0.001), varying at a non-constant rate through the water column. The spatial patterns derived from ASVs richness estimated by the Chao1 non-parametric estimator, as well as Simpson diversity index, were similar to those found from the observed ASV richness and Shannon diversity index, respectively (data not shown).
The ANOSIM analysis revealed absence of significant differences between Fisterra and Santander sections as well as among sampling stations within each section, considering the entire water column. For particular depth realms, only bathypelagic Archaea were significantly different between Fisterra and Santander (R = 0.336, p < 0.001). Conversely, large and significant differences in community composition were shown among sampling depth ranges (R = 0.393, p < 0.001 for Archaea; R = 0.679, p < 0.001, for Bacteria) and depth realms (R = 0.444, p < 0.001, for Archaea; R = 0.723, p < 0.001, for Bacteria). A subsequent SIMPER analysis identified that, on average, ∼12 ASVs out of a total of 855 (1.4% of SAobs) for Archaea, and ∼110 ASVs out of 3928 (2.8% of SBobs) for Bacteria, cumulatively accounted for 50% of compositional differences in prokaryotic communities among epi-, meso-, and bathypelagic realms.
Archaeal communities were composed of 32 abundant phylotypes (individually abundant ASVs, or groups of rare ASVs pooled within a given taxonomic level, accounting for >1% of the total number of reads) (see Table 2 for further details about nomenclature), while bacterial communities accounted for 38 abundant phylotypes (Table 3). Both prokaryotic communities were split in 4 clusters (Figure 3 for Archaea and Figure 4 for Bacteria): upper subsurface (cluster 1, mostly samples from 0 to <100 m depth); lower subsurface (cluster 2, including mostly samples from ∼100 to 250 m); intermediate (cluster 3, most of the samples collected between 500 and 2000 m); and deep (cluster 4, predominantly, depth ≥ 2000 m). Further details about the differences in community composition among the four clusters are available in Supplementary Figures 11, 12 for Archaea and Bacteria, respectively.
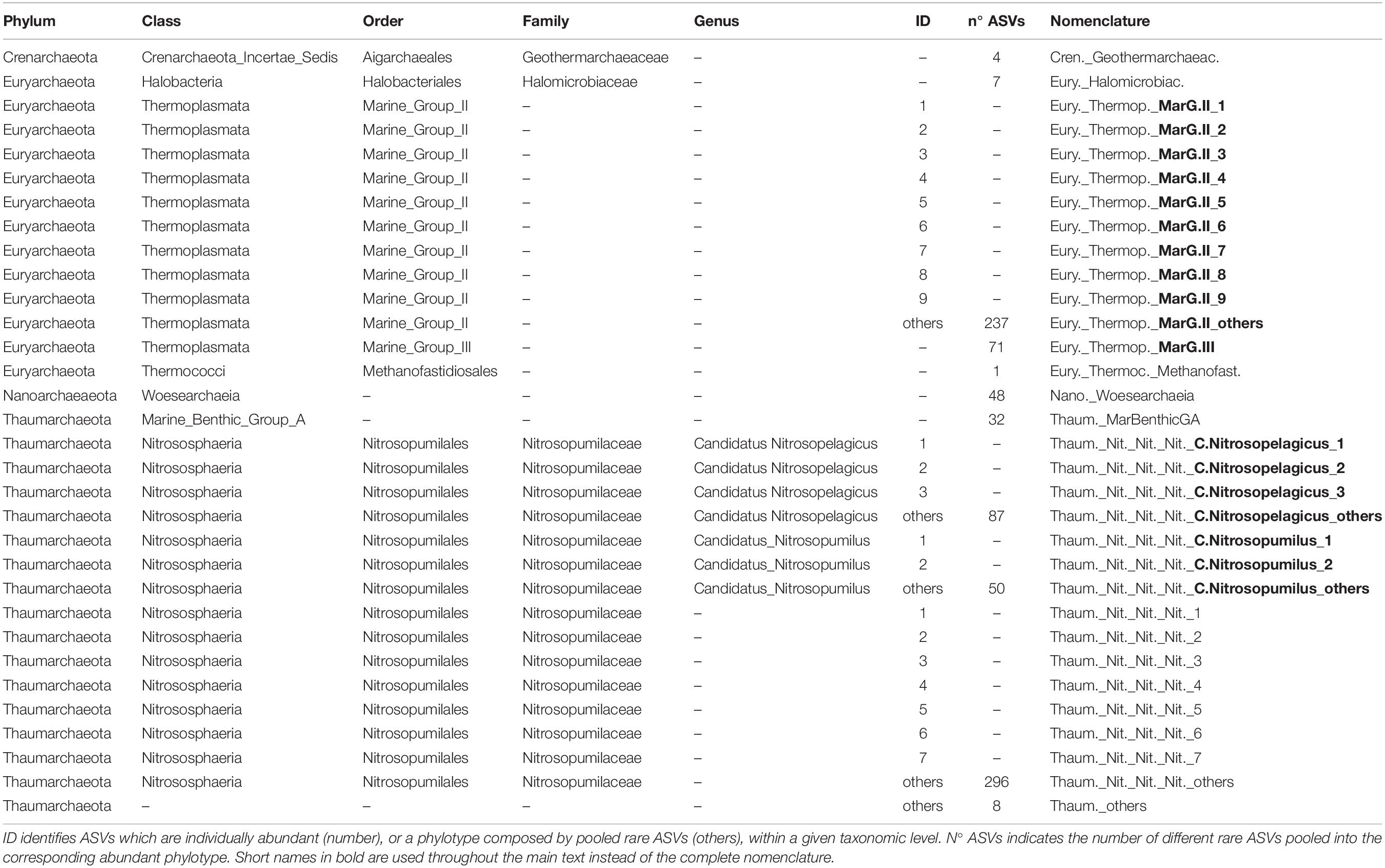
Table 2. Taxonomy and nomenclature of the Archaea abundant phylotypes (individually abundant ASVs, or groups of rare ASVs, with relative abundance > 1% of the total number of reads in the corresponding data set).
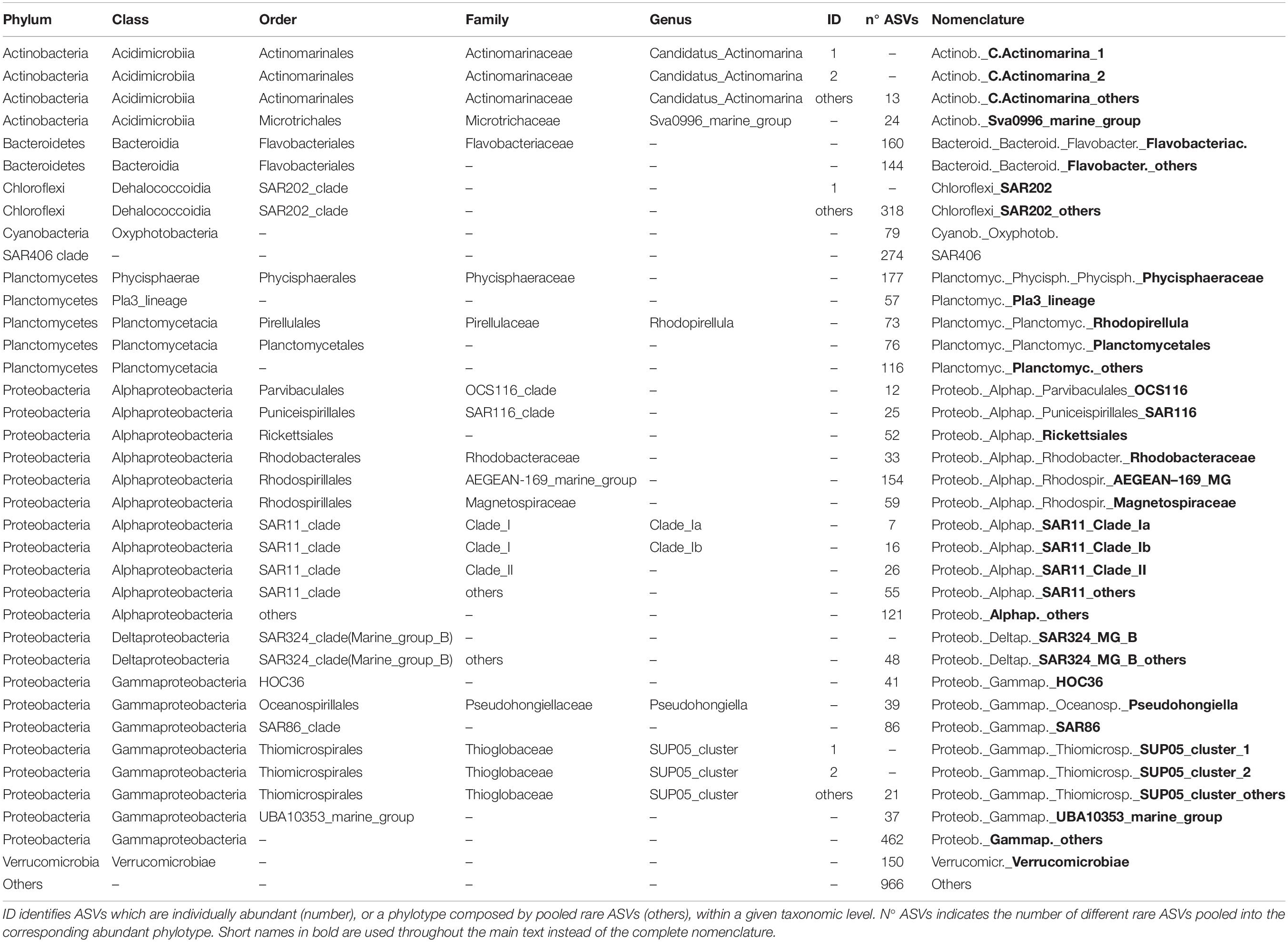
Table 3. Taxonomy and nomenclature of the Bacteria abundant phylotypes (individually abundant ASVs, or groups of rare ASVs, with relative abundance > 1% of the total number of reads in the corresponding data set).
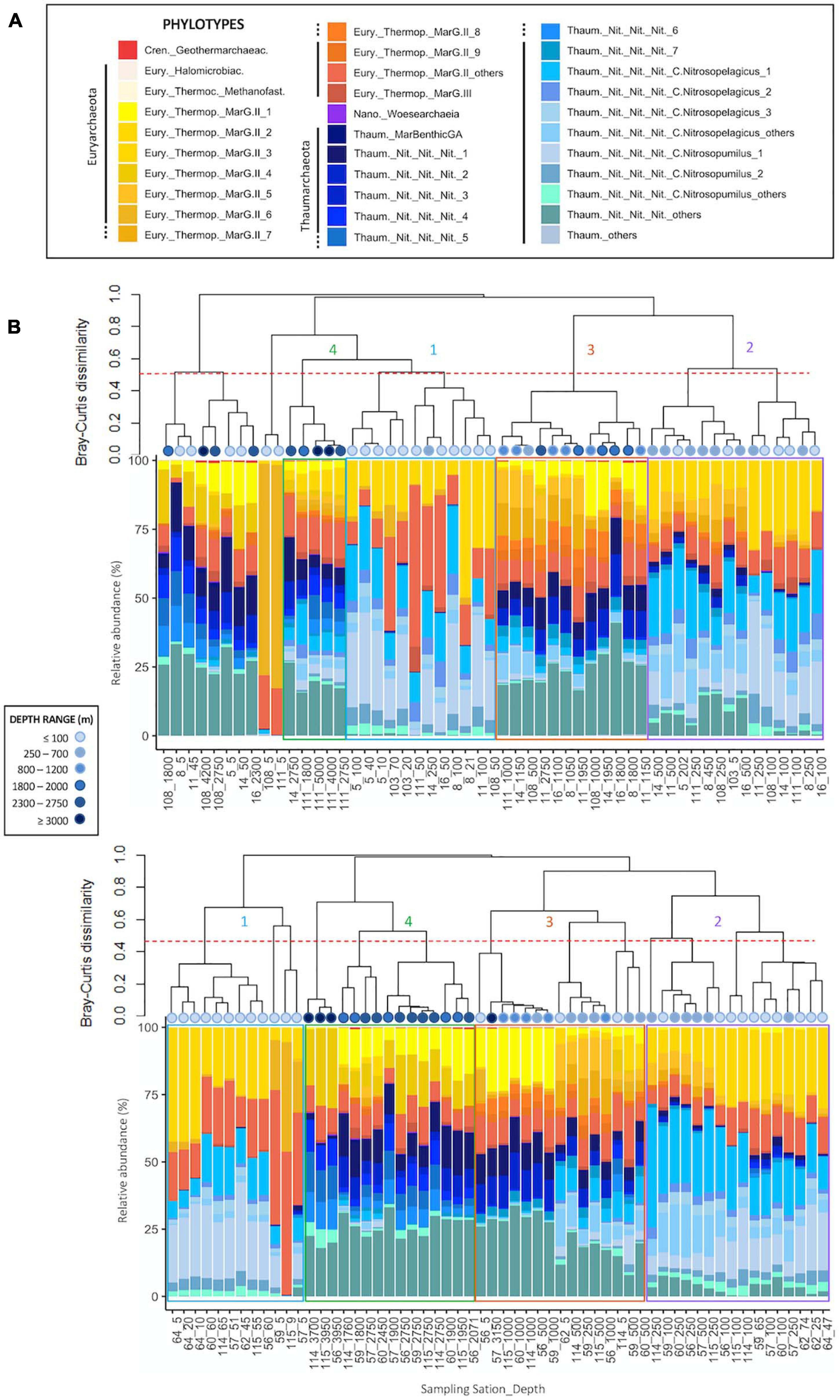
Figure 3. Cluster dendrogram based on Bray-Curtis dissimilarity (after Hellinger transformation) among samples, associated to the plot of community composition in terms of the relative abundance (%) of abundant (relative abundance higher than 1% of total number of sequences in the dataset) Archaea phylotypes per sample for panels (A) Fisterra, and (B) Santander sections. Red-dashed line shows 50% of dissimilarity. Boxes help to visualize the 4 different clusters: cluster 1 = upper subsurface (≤100 m, blue); cluster 2 = lower subsurface (100-500 m, purple); cluster 3 = intermediate (500–2000 m, orange); cluster 4 = deep communities (≥2000 m, green). See Table 2 for further details on the taxonomy of abundant archaeal phylotypes. For comparative purposes, the same analysis, but constructed under the classical equal-taxonomic-level grouping approach, is shown in Supplementary Figure 9.
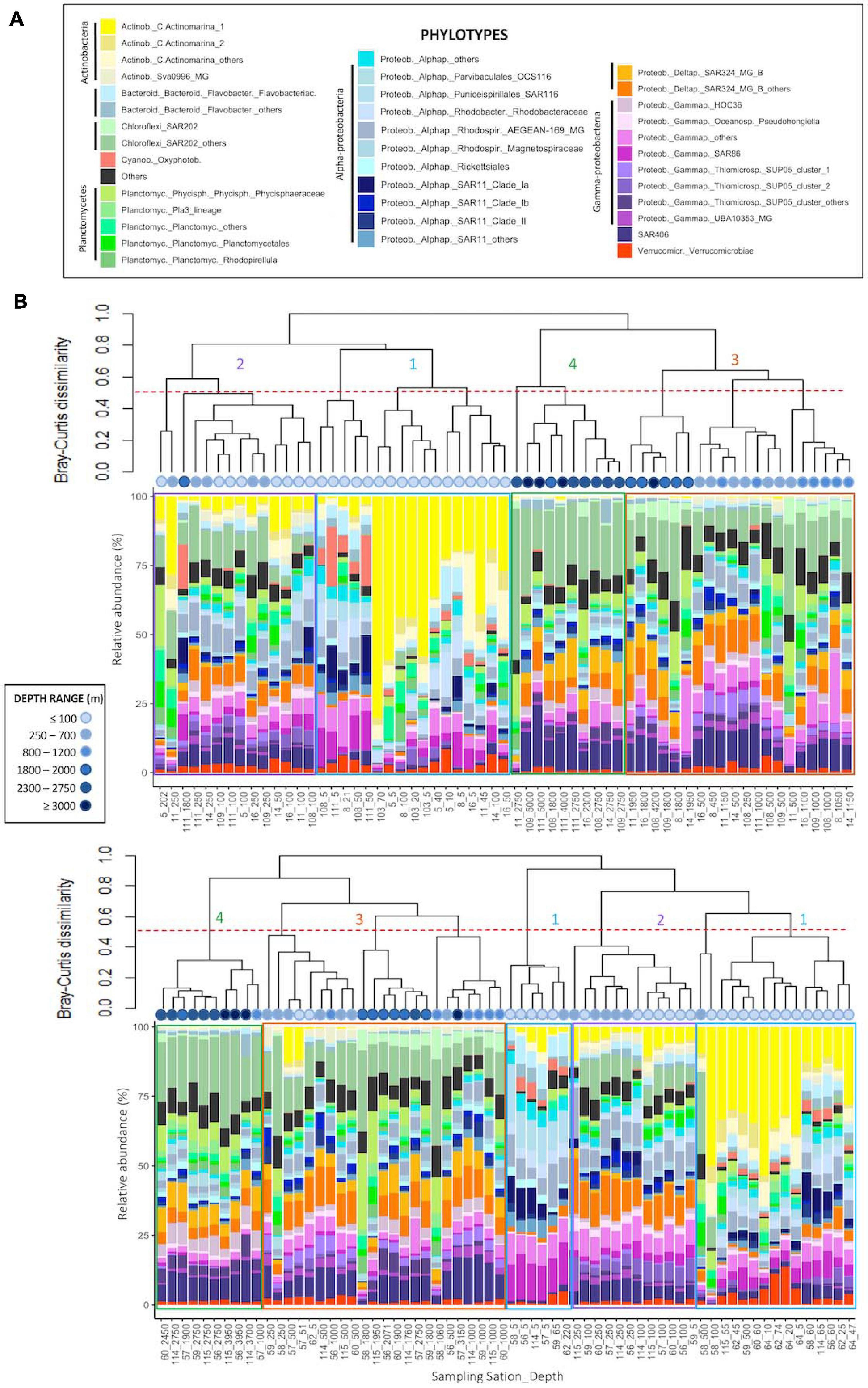
Figure 4. Cluster dendrogram based on Bray-Curtis dissimilarity (after Hellinger transformation) among samples, associated to the plot of community composition in terms of the relative abundance (%) of abundant (with relative abundance higher than 1% of total number of sequences in the dataset) Bacteria phylotypes per sample: (A) Fisterra, and (B) Santander sections. Red-dashed line shows 50% of dissimilarity. Boxes help to visualize the 4 different clusters: cluster 1 = upper subsurface (depth ≤ 100 m, blue); cluster 2 = lower subsurface (100-500 m, purple); cluster 3 = intermediate (500-2000 m, orange); cluster 4 = deep communities (≥2000 m, green). See Table 3 for further details on the taxonomy of abundant bacterial phylotypes. For comparative purposes, the same analysis, but constructed under the classical equal-taxonomic-level grouping approach, is shown in Supplementary Figure 10.
The abundant ASVs/phylotypes conforming archaeal and bacterial communities displayed 3 different vertical patterns of relative abundance throughout the water column: (i) high abundance at subsurface communities (clusters 1, 2, or both) but (almost) absence at intermediate and deep samples (clusters 3, 4, or both); (ii) the opposite trend, i.e., high abundance in clusters 3, 4, or both, but (almost) absence in clusters 1 and 2; and (iii) roughly constant relative abundance throughout the water column. These 3 distinctive patterns were found, for instance, among the 7 most abundant ASVs within the archaeal family Nitrosopumilaceae (Thaum._Nit_Nit_Nit_1 to _7; Supplementary Figures 11L-O) and among the 9 most abundant ASVs belonging to the order Marine Group II (MarG.II_1 to _9; Supplementary Figures 11B-F). For Bacteria, one ASV belonging to SAR202 was individually abundant, especially in cluster 3, while the other 318 ASVs were rare (grouped into SAR202_others) throughout the water column (Supplementary Figure 12D). These differential distributions for abundant ASVs/phylotypes were undetected with the classical grouping method at equal-taxonomic level (Supplementary Figure 9 for Archaea and Supplementary Figure 10 for Bacteria).
Prokaryotic Co-occurrence Associations
Only a few ASVs/phylotypes showed completely random relationships with their partners, i.e., their presence does not suggest the others’ presence or absence (Supplementary Figure 13), such as Flavobacteriaceae, comprising 160 ASVs, and SAR406, comprising of 274 ASVs. Conversely, most of the phylotypes have shown positive and/or negative co-occurrence relationships with up to ∼60% of the other phylotypes. The co-occurrence semi-matrix (Figure 5) was mainly composed by two groups: (i) phylotypes predominantly and positively (blue triangle) related to each other, with higher relative abundance at intermediate and deep waters (green-dashed ellipse, clusters 3 and 4; see Figures 3, 4 for Archaea and Bacteria, respectively), such as Thaum._Nit._Nit._Nit._1, _2, _3, _4 and _7, or SAR202_others; and (ii) phylotypes found throughout the water column (yellow ellipse), such as MarG.III and SAR406. The latter included a subgroup of phylotypes particularly abundant at subsurface waters (purple ellipse, clusters 1 and 2), such as SAR11-Clade_Ia, SAR116 and Thaum._Nit._Nit._Nit._5. These showed mostly negative co-occurrence relationships (red box) with the phylotypes included in the first group (green-dashed ellipse), such as between the closely related SAR11-Clade_Ia and SAR11-Clade_Ib, and Thaum._Nit._Nit._Nit._4 and _5. Additionally, both positive and negative co-occurrence relationships were found between members of the Archaea and Bacteria domains. For instance, C.Actinomarina_1 was present together with the archaeal ASVs MarG.II _2 and _6, however, negative co-occurrence was shown with respect to MarG.II_1 and _4.
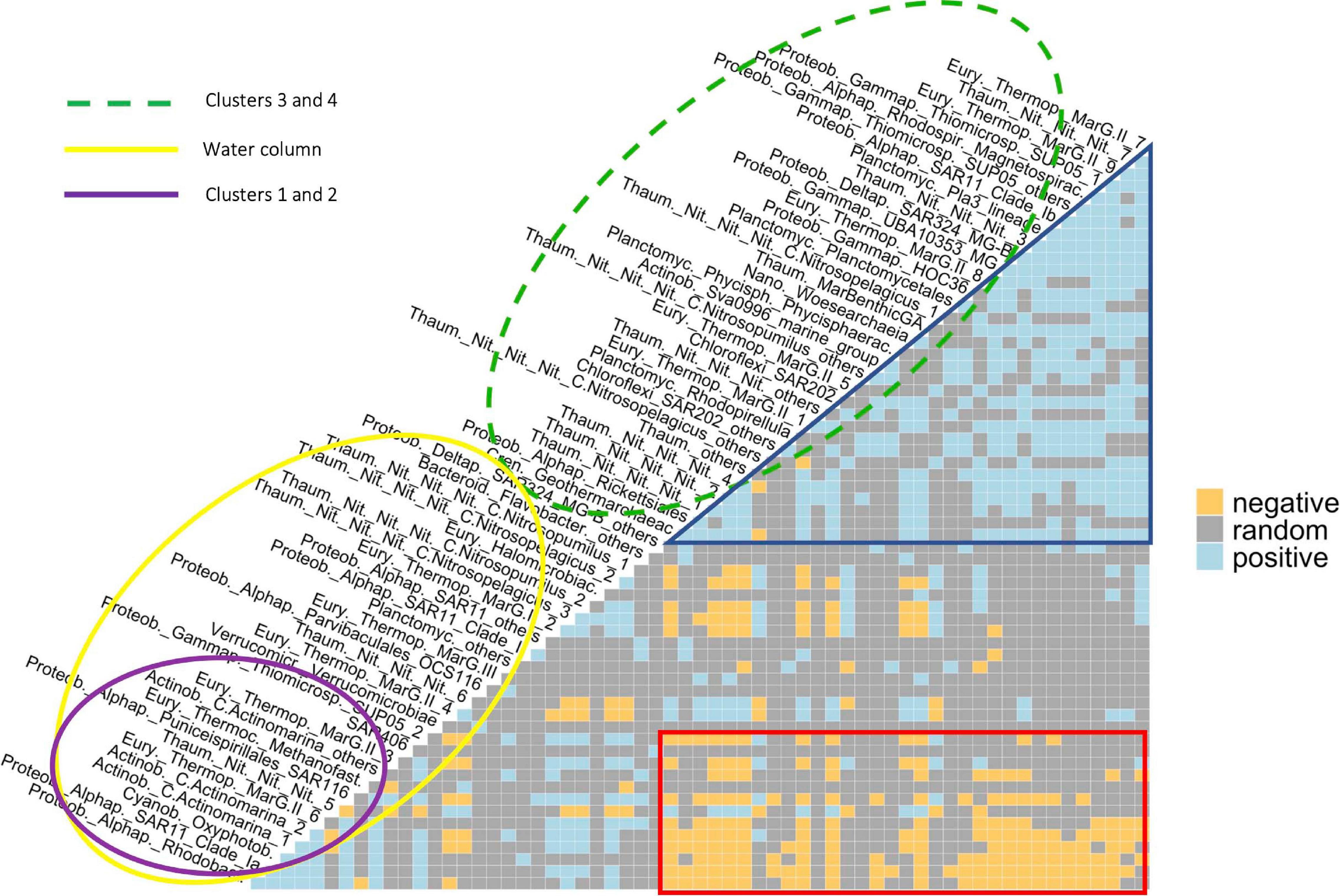
Figure 5. Co-occurrence (semi-)matrix. Blue and orange squares denote, respectively, positive and negative co-occurrence between pairwise abundant phylotypes, while gray squares mean random relationship between them. The green-dashed ellipse shows a group of ASVs/phylotypes mainly found in intermediate and deep communities (clusters 3 and 4), while the yellow ellipse includes abundant phylotypes found through the entire water column. The purple ellipse shows a sub-group of phylotypes found in higher relative abundance at upper and lower subsurface waters (clusters 1 and 2). The blue triangle and the red box highlight groups with mainly positive and negative co-occurrence relationships, respectively. See Tables 2, 3 for further details on the taxonomy of abundant archaeal and bacterial phylotypes, respectively.
Hydrography and DOM Driving Prokaryotic Assembly
Archaeal and bacterial alpha-diversity metrics were not correlated with the environmental variables [salinity, nitrite concentration, FDOM-T, FDOM-M, aCDOM254, and s(275-295)]. The exception was a moderate inverse monotonic relationship between archaeal alpha-diversity (SAObs and ShannonA) and both FDOM-T and aCDOM254 (Supplementary Table 6).
The RDA analysis revealed the best set of hydrographic and DOM quality parameters explaining the vertical structure of prokaryotic communities (Figure 6). Prokaryotic assemblages inhabiting deep waters (particularly MarG.II _4 and Thaum._Nit._Nit._Nit._1, _2, _4, _5 and _6 in the Archaea domain, and Pla3_lineage, SUP05_cluster_others, SAR202, SAR324_MG_B, and Others in Bacteria) were strongly linked to FDOM-M. Interestingly, some upper bathypelagic (average depth = 1700 m) archaeal samples from Fisterra (black circle) were included in the intermediate cluster, dominated by Thaum._Nit._Nit._Nit._3 and _7, and MarG.II_7, _8 and _9 and influenced by FDOM-M and salinity. Conversely, bacteria in the intermediate cluster were mainly related to FDOM-M but not influenced by salinity. Upper subsurface Archaea assemblages (particularly MarG.II_2, _6 and _others) were strongly linked to aCDOM254 and FDOM-T, and in a lesser extent to nitrite concentration, whereas lower subsurface Archaea assemblages were mainly associated to salinity (linked to C.Nitrosopelagicus_others and _1, and MarG.II_3) and the slope s(275-295) (linked to C.Nitrosopumilus_1 and _2, and to C.Nitrosopelagicus_1 and _2). However, both upper and lower subsurface bacterial assemblages were related to aCDOM254, nitrite concentration, FDOM-T, salinity and s(275-295). Those variables showed, however, different influence over specific phylotypes. While aCDOM254 and the slope s(275-295) were mainly related to Rhodobacteraceae, Cyanob._Oxyphotob., and Flavobacteriac., FDOM-T was linked to SUP05_cluster_2 and C.Actinomarina_others. Likewise, salinity was mainly associated to Planctomycetales and C.Actinomarina_2, whereas nitrite concentration mainly influenced the distribution of Rhodopirellula, Planctomyc._others, and Phycisphaeraceae (Planctomycetes).
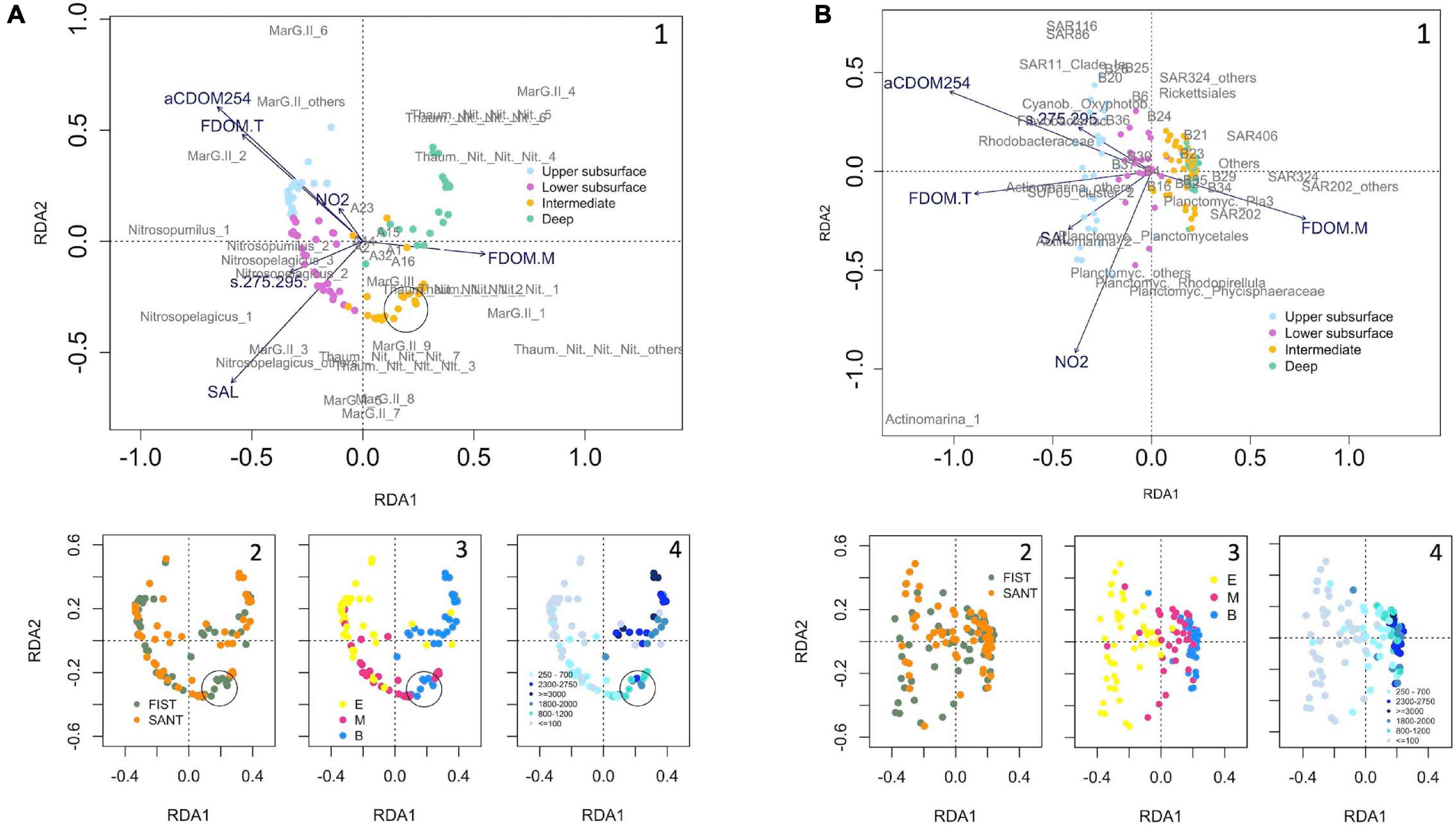
Figure 6. Redundancy analysis (RDA) derived from Hellinger-standardized relative abundance for the abundant phylotypes of panels (A) Archaea and (B) Bacteria, and abiotic (environmental and DOM-related) variables, after removing collinear variables. The arrow length represents the strength of the correlation between the environmental variables and the samples. The longer the arrow length, the stronger the correlation. The perpendicular distance between the samples or the abundant phylotypes and the environmental variables reflects their correlations. The smaller the distance, the stronger the correlation. Samples are color-coded as a function of: (1) cluster, based on community dissimilarity (see Figure 4 for Archaea and Figure 5 for Bacteria); (2) transect (FIST = Fisterra, green, and SANT = Santander, orange); (3) depth realm [E = Epipelagic (<200 m), M = Mesopelagic (200–1000 m), and B = Bathypelagic (>1000 m)]; and (4) sampling depth range (m). See Tables 2, 3 for further details on the taxonomy of abundant archaeal and bacterial phylotypes, respectively. Abbreviations in this figure: A1: Cren._Geothermarchaeac; A2: Eury._Halomicrobiac.; A14: Eury._Thermoc._Methanofast.; A15: Nano._Woesearchaeia; A16: Thaum._MarBenthicGA; A23: C.Nitrosopumilus_others; A32: Thaum._others; B4: Actinob._Sva0996_marine_group; B6: Flavobacter._others; B16: OCS116; B20: AEGEAN-169_marine_group; B21: Magnetospiraceae; B23: SAR11_Clade_Ib; B24: SAR11_Clade_II; B25: SAR11_others; B26: Proteob._Alphap._others; B29: HOC36; B30: Pseudohongiella; B32: SUP05_cluster_1; B34: SUP05_cluster_others; B35: UBA10353_marine_group; B36: Proteob._Gammap._others; B37: Verrucomicrobiae.
Discussion
The study of the spatial dynamics of prokaryotic communities in relation to biotic interactions, hydrographic conditions, and particularly to the quantity and quality of DOM, is essential to better understand the driving forces and the functional traits of individual populations in marine ecosystems. Here, we studied how biotic and abiotic factors drive prokaryotic spatial patterns, niche partitioning, and the differentiation of ecotypes for closely related ASVs/phylotypes, from coastal to offshore and from surface to deep ecosystems, in an upwelling-influenced area.
Vertical Niche Partitioning of Prokaryotic Communities
Our data displayed that most of ASVs were shared between sections. Furthermore, alpha-diversity metrics were not related to the distance to the coast. The horizontal homogeneity in prokaryotic alpha-diversity, previously shown by Hernando-Morales et al. (2018) by comparing bacterial OTU richness from two sites (separated ∼200 km) within the Galician upwelling system, is now extended to ASV-level Archaea and Bacteria diversity and a larger spatial scale (up to ∼600 km), including the Bay of Biscay and offshore waters along coastal-oceanic gradients in the Atlantic Ocean off the Iberian Peninsula. Upwelling filaments off Iberia were previously estimated to export ∼35-58% of the net community production produced in the coastal upwelling systems to the offshore system (Álvarez-Salgado et al., 2007). Furthermore, advection from the French shelf has been shown to reach the Santander hydrographical section (Puillat et al., 2004; Reverdin et al., 2013). Thereby, the associated horizontal transport of subsurface waters may have resulted in the connectivity of prokaryotic communities at the horizontal scale through passive dispersal in the area receiving exported waters. However, the DOM pool is degraded both by photobleaching in surface and by prokaryotic activity (Nieto-Cid et al., 2006) and, in turn, it is modified during this horizontal transport, likely explaining the changes in DOM optical properties found from coastal to offshore stations. On the other side, the substantial number of ASVs shared among epi-, meso- and bathypelagic realms points towards a significant vertical connectivity among prokaryotic communities (Frank et al., 2016; Milici et al., 2016; Mestre et al., 2018). However, the highest number of unique ASVs at bathypelagic waters, as found previously globally (Salazar et al., 2016), along with depth-increasing prokaryotic alpha-diversity and relative abundance of the bacterial group Others (including ∼1000 ASVs in very low relative abundance), highlight the relevance of the “rare biosphere” at the deep dark ocean as a reservoir of genomic diversity (Sogin et al., 2006).
Prokaryotic beta-diversity was similar in the two transects at the different depths, except for the significant differences in archaeal communities in the upper bathypelagic (∼1700 m average depth). One explanation to the latter could be the more notorious signal of the high-salinity and low-oxygen oligotrophic Mediterranean Water (MW) along with a high degree of water mass mixing in Fisterra (Ruíz-Villarreal et al., 2006), evidencing that these upper bathypelagic samples were similar to the intermediate cluster (linked to the salinity signature, attributed to the influence of oligotrophic MW, Figure 6). On the contrary, a significant vertical niche partitioning of prokaryotic communities was found to be consistent for Archaea and Bacteria. Previous studies in the North Atlantic Ocean suggested differences in bacterial community structure among different water masses (Agogué et al., 2011; Frank et al., 2016) and depth realms (Guerrero-Feijóo et al., 2017). In the present study, however, Archaea and Bacteria assemblages were split into four vertical clusters, with the main differences in prokaryotic community composition found between subsurface and deeper communities (Figures 3, 4), according to previous findings at global scale (Sunagawa et al., 2015). We suggest that the division into upper and lower subsurface clusters was likely due to a physical constraint. Upper subsurface communities might be retained within the layer of higher PAR irradiance and stronger stratification (the first 100 m of the water column; Supplementary Figure 4), which limits the vertical mixing of surface waters (Cheng et al., 2020). This result is in accordance with the significant differences in prokaryotic community composition between superficial and deep chlorophyll maximum samples previously found at global scale (Sunagawa et al., 2015). Moreover, there were no differences in community composition within mesopelagic nor bathypelagic depth realms, in accordance with previous investigations (Salazar et al., 2016; Hernando-Morales et al., 2018; Li et al., 2018). Yet, how these vertical patterns might vary with stratification seasonality and mesoscale events in our study area requires further investigation.
Most archaeal and bacterial groups were uneven in terms of abundance. For instance, only 9 out to 246 ASVs belonging to the order MarG.II were abundant (relative abundance > 1% of the total number of reads) individually. In addition, abundant phylotypes were not equally distributed spatially. The three vertical patterns of relative abundance found among prokaryotic members might likely be interpreted as a differentiation between generalists (present throughout the water column) versus specialists (abundant/absent under particular environmental conditions). Among the generalists in their vertical distribution, SAR406 and SAR324 were also found to be generalists in their global-scale horizontal distribution, i.e., they are cosmopolitan (Salazar et al., 2016). On the other side, our approach has allowed to distinguish a variety of spatial distributions for MarG.II relatives, previously undetected in this study area (Guerrero-Feijóo et al., 2017). For instance, some ASVs displayed higher relative abundances at deep than at superficial waters (e.g., MarG.II_1 and _4), according to Rinke et al. (2019). Thus, MarG.II might likely have disparate impacts on carbon remineralization in the marine pelagic environment through their metabolic diversity (Zhang et al., 2015; Tully, 2019), and/or its microdiversity could increase the stability of microbial communities dealing with environmental fluctuation (García-García et al., 2019). Similarly, members of the family Nitrosopumilaceae (Thaumarchaeota) were distributed among the four vertical clusters, differentially related to particular hydrographic parameters and DOM quality indicators, in accordance with the recently demonstrated existence of ecotypes within this archaeal phylum (Reji et al., 2019). Importantly, the existence of depth-ecotypes displayed in this study would be undetectable by using the classical ASVs pooling methodology at equal taxonomic level (Supplementary Figures 9, 10 for Archaea and Bacteria, respectively). A similar result was recently found by Mena and collaborators, who found that seasonal niche partitioning of superficial communities was only unveiled when analyzed at oligotype level, however unseen at order level (Mena et al., 2020).
Co-occurrence Associations Among Prokaryotic Phylotypes
Our results showed a differentiation of the co-occurrence semimatrix into two main groups, suggesting non-randomly assembled communities. Most importantly, both negative and positive co-occurrence relationships were found among taxonomically close relatives. The production of similar suites of DOM has been demonstrated for closely related phytoplankton species (Becker et al., 2014). Likewise, it could be expected that closely related prokaryotic ASVs were responsible for the uptake and remineralization of similar suites of DOM, hence competing for similar resources. Then, environmental forcing may select for ASVs which competitively exclude the others (negative co-occurrence) [e.g., between MarG.II_6 and _7, related to nitrite, and the high-salinity signal of the oligotrophic MW, respectively (Figure 6)]. Alternatively, it may generate niche partitioning, allowing positive co-occurrence among competing ASVs/phylotypes [e.g., among Thaum._Nit._Nit._Nit._1, _2, _3, _4 and _7, all abundant at intermediate/deep communities (Supplementary Figure 11), and related to FDOM-M (Figure 6)]. Likewise, positive co-occurrence was found among ASVs implicated in the sulfur cycle in marine environments, such as Rhodobacteraceae (Pujalte et al., 2014), SAR116 (Choi et al., 2015), SAR11 (Howard et al., 2006), and Cyanob._Oxyphotob. (e.g., McParland and Levine, 2019). These positive co-occurrences might potentially suggest partial functional redundancy (Galand et al., 2018) for ASVs/phylotypes sharing an ecological niche. However, small differences in ecological requirements could allow the coexistence of organisms that share some specific function in the ecosystem (Bryson et al., 2017). Alternatively, they could perform different roles in the marine biogeochemical cycles or experiment potential reciprocal interactions via dissolved organic matter production/transformations (Bayer et al., 2019). For instance, SAR11 requires the thiamine precursor compound 4-amino-5- hydroxymethyl-2-methylpyrimidine (HMP), however they lack the enzyme ThiC that catalyzes the reaction to produce HMP in bacteria (Giovannoni, 2017). According to the Black-Queen Hypothesis [“the beneficiary can stop performing a costly function that is provided by the leaky helper”; (Morris et al., 2012)], HMP production by B1 phototrophic marine cyanobacteria (Carini et al., 2014) would explain the positive co-occurrence found between SAR11 and Cyanob._Oxyphotob. in our studied area.
Hydrography and DOM Quality Indicators Driving Niche Partitioning
Our investigation has found significant links between specific ASVs/phylotypes and different DOM quantity and quality indicators. Prokaryotic assemblages in deep waters, likely depleted in bio-available organic matter, were related to FDOM-M, the fluorescence of resistant humic-like substances generated in situ as a sub-product of microbial respiration (Nieto-Cid et al., 2006), as previously shown in Fisterra (Guerrero-Feijóo et al., 2017), and now extended to the Bay of Biscay. The capacity of some phylotypes to chemolithotrophy, such as Thaumarchaeota (ASVs Thaum._Nit._Nit._Nit._1, _2, _4, _5 and _6), that are ammonia-oxidizing Archaea (Varela et al., 2011; Guerrero-Feijóo et al., 2018; Santoro et al., 2019), and SUP_05 that are nitrate-reducing, sulfur-oxidizing γ-proteobacteria (Bourbonnais et al., 2012) might play a substantial role in sustaining the dark ocean’s respiratory activity. On the other side, the predicted role in high-molecular-weight organic matter degradation of MarG.II turns it into a key-taxon for understanding remineralization in the global ocean (Tully, 2019), particularly the ASVs MarG.II_1 and _4 in deep waters in our study area. Likewise, SAR202, dominant in deep communities, according to Varela et al. (2008), has been found to poses a large number of oxidative enzymes which might catalyze the final steps in the biological oxidation of relatively recalcitrant organic compounds (Landry et al., 2017). Some deep ecotypes could be opportunistic taxa within the bathypelagic assemblages, conferring them a broader capability of substrate utilization (Sebastián et al., 2021). Alternatively, mixotrophy., i.e., carrying out chemolithoautotrophic inorganic carbon fixation and heterotrophy (Sorokin, 2003; Alonso-Sáez et al., 2010) has been recently suggested to represent an ecologically relevant trait for prokaryotes to survive across the global dark ocean (Acinas et al., 2021).
The observed differences on the link between intermediate and subsurface assemblages with hydrography and DOM quality indicators suggest an important disparity between Archaea and Bacteria domains. This disconnection was also found by Pereira et al. (2021), who found that Archaea completely disappeared from superficial waters in the NW Mediterranean Sea during low-productive summer, contrarily to Bacteria that were present all year round. In our study area, archaeal upper subsurface communities were dominated by MarG.II, particularly MarG.II_2 and MarG.II_others. They likely belong to the ecotype MarG.IIA, possessing proteorhodopsins (Santoro et al., 2019), which can generate a light-driven chemiosmotic potential (Frigaard et al., 2006; Iverson et al., 2012). Conversely, archaeal lower subsurface communities (cluster 2) were dominated by MarG.II_3, which was, however, found in very low abundance in cluster 1. We suggest that MarG.II_3 might be likely more sensitive to light intensity or, alternatively, this ASV could exploit organic resources others than those available at upper subsurface waters. Our results also evidenced several ASVs of genera Candidatus Nitrosopumilus and Candidatus Nitrosopelagicus, described as ammonia-oxidizing Archaea (AOA) (Santoro et al., 2019), highly positively related to s(275-295), i.e., to changes in DOM molecular weight and/or aromaticity (Helms et al., 2008). Candidatus Nitrosopumilus has been demonstrated to release hydrophobic amino acids which could be crucial for auxotrophic microbes for these compounds, such as members of SAR11 clade (Bayer et al., 2019). This findings agree with the positive co-occurrence between SAR11_others and C.Nitrosopumilus_2 displayed in this work. The group MarG.III, although present throughout the water column, appeared to be closely related to intermediate communities (cluster 3, influenced by FDOM-M and the MW salinity signature), providing new knowledge on its little-known distribution (Santoro et al., 2019).
Unlike for Archaea, both upper and lower subsurface bacterial communities (clusters 1 and 2) were related to several environmental drivers [aCDOM254, nitrite concentration, FDOM-T, MW salinity signature and s(275-295)], suggesting a wider spectra of niches/metabolisms per cluster. SAR11 was found in relatively lower relative abundance than expected, especially in the stations closer to the coast. Actinobacteria was dominant in the upper subsurface assemblage, in agreement to its globally reported high abundance in the photic zone (Ghai et al., 2013), where they derive energy from the phytoplankton-produced dimethylsulfopropinate (DMSP) (Mizuno et al., 2015). However, C.Actinomarina_others were particularly related to FDOM-T (the fluorescence of labile protein-like molecules), C.Actinomarina_2 to salinity, and C.Actinomarina_1 to nitrite concentration. In the photic layer, where phytoplanktonic production of organic carbon takes place, Flavobacteriac., Cyanob._Oxyphotob., and Rhodobacteraceae were positively related to the aCDOM254, a proxy for the concentration (quantity) of DOC (Lønborg and Álvarez-Salgado, 2014), and in a lesser extent to the spectral slope s(275-295) (aromatic quality of DOM). The higher values of this slope at subsurface waters suggest associations among these phylotypes and the low molecular weight DOM, likely derived from photoreactions reducing DOM molecular weight (Miller and Moran, 1997; Catalá et al., 2018). Several Planctomycetes, which were shown to be involved in different processes regarding N cycling in marine environments, such as N2 fixation (Delmont et al., 2018), anaerobic ammonium-oxidization (Van Teeseling et al., 2015), and dissimilatory nitrate (or nitrite) reduction to ammonia (Pajares and Ramos, 2019), were positively related to nitrite concentration in our study area. This different dependency on environmental forcing for Archaea and Bacteria could allow some niche spaces to be shared by members of these two domains of life, which resulted in positive co-occurrence relationships between Archaea and Bacteria such as found in our study.
Conclusion
Our study displayed horizontally homogeneous prokaryotic communities along two coastal-oceanic gradients in the Atlantic Ocean off the Iberian Peninsula, except for the difference in upper bathypelagic Archaea (attributed to the most notorious influence of the MW in Fisterra). Conversely, a significant vertical niche partitioning was revealed for Archaea and Bacteria assemblages, related to the vertical variability in environmental conditions (DOM quality and quantity and hydrography). Deep prokaryotic assemblages were related to refractory humic-like organic matter (FDOM-M), while archaeal and bacterial intermediate and subsurface clusters were differentially linked to DOM quality and hydrography. This different dependence on environmental forcing suggests a differentiation between Archaea and Bacteria in their responses to environmental changing conditions, potentially having a major impact under the predicted climate change scenario. Most importantly, we unveiled the existence of depth-ecotypes for closely related ASVs/phylotypes, previously undetected in the studied area. Overall, our study sheds light on the ecological processes and drivers shaping archaeal and bacterial community structure and ultimately, their biogeography, with implications for the organic matter cycling in marine ecosystems.
Data Availability Statement
The datasets presented in this study can be found in online repositories. The names of the repository/repositories and accession number(s) can be found below: https://www.ncbi.nlm.nih.gov/, PRJNA639438.
Author Contributions
MV conceived and planned the work and was the MODUPLAN cruise leader. MV, MN-C, and EG-F carried out the onboard work. TR-R and AA performed the bioinformatics. MN-C carried out DOM analyses. TR-R performed the analysis and interpretation of data and wrote the manuscript. All authors contributed to the manuscript and approved the submitted version.
Funding
Funding for sampling and analysis was provided by the projects “Fuentes de materia orgánica y diversidad funcional del microplancton en las aguas profundas del Atlántico Norte” (MODUPLAN, Ref. CTM2011-24008-MAR, 2012-2015, Plan Nacional I+D+i), and “Deep Standard Oceanographic Sections monitoring program RADIALES-PRODUNDOS,” funded by the Instituto Español de Oceanografía (IEO). This work was partly funded by European Union Atlantic Area Interreg project iFADO (EAPA/165_2016) and from the Axencia Galega de Innovación (GAIN, Xunta de Galicia, Spain). TR-R was supported by the MICINN program “Personal Técnico de Apoyo a la Investigación” (PTA, Ref. PTA2015-10948-I) and the project iFADO. MN-C was funded by the projects HOTMIX and FERMIO (MINECO, CTM2011–30010–C02 MAR and CTM2014-57334-JIN, respectively), both co-financed with FEDER funds. AA was funded by grant REMEI (CTM2015-70340-R) from the Spanish Ministry of Economy, Industry and Competitiveness, and Grant 2017SGR/1568 from the CIRIT-Generalitat de Catalunya to Consolidated Research Groups.
Conflict of Interest
The authors declare that the research was conducted in the absence of any commercial or financial relationships that could be construed as a potential conflict of interest.
Publisher’s Note
All claims expressed in this article are solely those of the authors and do not necessarily represent those of their affiliated organizations, or those of the publisher, the editors and the reviewers. Any product that may be evaluated in this article, or claim that may be made by its manufacturer, is not guaranteed or endorsed by the publisher.
Acknowledgments
We thank the captain and the crew of R/V Sarmiento de Gamboa for their support during MODUPLAN cruise, V. Vieitez, D. Roque, and M. J. Pazó for their assistance with DOM analysis, Carlota Rodríguez for assistance with DNA extractions and PCRs, M. Ruíz-Villarreal for providing the satellite images and critically commenting hydrography data, and Federico Baltar for his helpful comments on the manuscript. High-performance computing analyses were run by means of the remote supercomputing resources of the Centro de Supercomputación de Galicia (CESGA).
Supplementary Material
The Supplementary Material for this article can be found online at: https://www.frontiersin.org/articles/10.3389/fmars.2021.673171/full#supplementary-material
Footnotes
References
Acinas, S. G., Sánchez, P., Salazar, G., Cornejo-Castillo, F. M., Sebastián, M., Logares, R., et al. (2021). Deep ocean metagenomes provide insight into the metabolic architecture of bathypelagic microbial communities. Commun. Biol. 4, 1–15. doi: 10.1038/s42003-021-02112-2
Agogué, H., Lamy, D., Neal, P. R., Sogin, M. L., and Herndl, G. J. (2011). Water mass-specificity of bacterial communities in the North Atlantic revealed by massively parallel sequencing. Mol. Ecol. 20, 258–274. doi: 10.1111/j.1365-294X.2010.04932.x
Alonso-Sáez, L., Galand, P. E., Casamayor, E. O., Pedrós-Alió, C., and Bertilsson, S. (2010). High bicarbonate assimilation in the dark by Arctic bacteria. ISME J. 412, 1581–1590. doi: 10.1038/ismej.2010.69
Álvarez-Salgado, X. A., and Miller, A. E. (1998). Simultaneous determination of dissolved organic carbon and total dissolved nitrogen in seawater by high temperature catalytic oxidation: conditions for precise shipboard measurements. Mar. Chem. 62, 325–333. doi: 10.1016/S0304-4203(98)00037-1
Álvarez-Salgado, X. A., Arístegui, J., Barton, E. D., and Hansell, D. A. (2007). Contribution of upwelling filaments to offshore carbon export in the subtropical Northeast Atlantic Ocean. Limnol. Oceanogr. 52, 1287–1292. doi: 10.4319/lo.2007.52.3.1287
Álvarez-Salgado, X. A., Labarta, U., Fernández-Reiriz, M. J., Figueiras, F. G., Rosón, G., Piedracoba, S., et al. (2008). Renewal time and the impact of harmful algal blooms on the extensive mussel raft culture of the Iberian coastal upwelling system (SW Europe). Harmful Algae 7, 849–855. doi: 10.1016/J.HAL.2008.04.007
Amaral, V., Graeber, D., Calliari, D., and Alonso, C. (2016). Strong linkages between DOM optical properties and main clades of aquatic bacteria. Limnol. Oceanogr. 61, 906–918. doi: 10.1002/lno.10258
Auladell, A., Sánchez, P., Sánchez, O., Gasol, J. M., and Ferrera, I. (2019). Long-term seasonal and interannual variability of marine aerobic anoxygenic photoheterotrophic bacteria. ISME J. 13, 1975–1987. doi: 10.1038/s41396-019-0401-4
Azam, F., Fenchel, T., Field, J., Gray, J., Meyer-Reil, L., and Thingstad, F. (1983). The ecological role of water-column microbes in the sea. Mar. Ecol. Prog. Ser. 10, 257–263. doi: 10.3354/meps010257
Barberán, A., Bates, S. T., Casamayor, E. O., and Fierer, N. (2012). Using network analysis to explore co-occurrence patterns in soil microbial communities. ISME J. 6, 343–351. doi: 10.1038/ismej.2011.119
Bayer, B., Hansman, R. L., Bittner, M. J., Noriega-Ortega, B. E., Niggemann, J., Dittmar, T., et al. (2019). Ammonia-oxidizing archaea release a suite of organic compounds potentially fueling prokaryotic heterotrophy in the ocean. Environ. Microbiol. 21, 4062–4075. doi: 10.1111/1462-2920.14755
Becker, J. W., Berube, P. M., Follett, C. L., Waterbury, J. B., Chisholm, S. W., DeLong, E. F., et al. (2014). Closely related phytoplankton species produce similar suites of dissolved organic matter. Front. Microbiol. 5:111. doi: 10.3389/fmicb.2014.00111
Bergauer, K., Fernandez-Guerra, A., Garcia, J. A. L., Sprenger, R. R., Stepanauskas, R., Pachiadaki, M. G., et al. (2018). Organic matter processing by microbial communities throughout the Atlantic water column as revealed by metaproteomics. Proc. Natl. Acad. Sci. U.S.A. 115, E400–E408. doi: 10.1073/pnas.1708779115
Bode, A., Álvarez, M., Ruíz-Villarreal, M., and Varela, M. M. (2019). Changes in phytoplankton production and upwelling intensity off A Coruña (NW Spain) for the last 28 years. Ocean Dyn. 69, 861–873. doi: 10.1007/s10236-019-01278-y
Bode, A., Varela, M., Barquero, S., Ossorio-Alvarez, M. T., and Gonzalez, N. (1998). Preliminary studies on the export of organic matter during phytoplankton blooms off la coruña (Northwestern Spain). J. Mar. Biol. Assoc. 78, 1–15. doi: 10.1017/s0025315400039928
Bourbonnais, A., Juniper, S. K., Butterfield, D. A., Devol, A. H., Kuypers, M. M. M., Lavik, G., et al. (2012). Activity and abundance of denitrifying bacteria in the subsurface biosphere of diffuse hydrothermal vents of the Juan de Fuca Ridge. Biogeosciences 9, 4661–4678. doi: 10.5194/bg-9-4661-2012
Bryson, S., Li, Z., Chavez, F., Weber, P. K., Pett-Ridge, J., Hettich, R. L., et al. (2017). Phylogenetically conserved resource partitioning in the coastal microbial loop. ISME J. 11, 2781–2792. doi: 10.1038/ismej.2017.128
Burnham, K. P., and Anderson, D. R. (2002). Model Selection and Multi-Model Inference: A Practical Information-Theoretic Approach, 2nd Edn. New York: Springer.
Callahan, B. J., McMurdie, P. J., and Holmes, S. P. (2017). Exact sequence variants should replace operational taxonomic units in marker-gene data analysis. ISME J. 11, 2639–2643. doi: 10.1038/ismej.2017.119
Callahan, B. J., McMurdie, P. J., Rosen, M. J., Han, A. W., Johnson, A. J. A., and Holmes, S. P. (2016). DADA2: High-resolution sample inference from Illumina amplicon data. Nat. Methods 13, 581–583. doi: 10.1038/nmeth.3869
Carini, P., Campbell, E. O., Morré, J., Sañudo-Wilhelmy, S. A., Cameron Thrash, J., Bennett, S. E., et al. (2014). Discovery of a SAR11 growth requirement for thiamin’s pyrimidine precursor and its distribution in the Sargasso Sea. ISME J. 8, 1727–1738. doi: 10.1038/ismej.2014.61
Casas, B., Varela, M., Canle, M., González, N., and Bode, A. (1997). Seasonal variations of nutrients, seston and phytoplankton, and upwelling intensity off La Coruna (NW Spain). Estuar. Coast. Shelf Sci. 44, 767–778. doi: 10.1006/ecss.1996.0155
Catalá, T. S., Martínez-Pérez, A. M., Nieto-Cid, M., Álvarez, M., Otero, J., Emelianov, M., et al. (2018). Dissolved organic matter (DOM) in the open Mediterranean Sea. I. Basin–wide distribution and drivers of chromophoric DOM. Prog. Oceanogr. 165, 35–51. doi: 10.1016/j.pocean.2018.05.002
Chao, A. (1984). Board of the foundation of the scandinavian journal of statistics nonparametric estimation of the number of classes in a population nonparametric estimation of the number of classes in a population. source scand. J. Stat. 11, 265–270.
Chen, H., and Boutros, P. C. (2011). VennDiagram: a package for the generation of highly-customizable venn and euler diagrams in R. BMC Bioinform. 12:35. doi: 10.1186/1471-2105-12-35
Cheng, W.-H., Lu, H.-P., Chen, C.-C., Jan, S., and Hsieh, C. (2020). Vertical beta-diversity of bacterial communities depending on water stratification. Front. Microbiol. 11:449. doi: 10.3389/fmicb.2020.00449
Choi, D. H., Park, K. T., An, S. M., Lee, K., Cho, J. C., Lee, J. H., et al. (2015). Pyrosequencing revealed sar116 clade as dominant dddp-containing bacteria in oligotrophic NW Pacific Ocean. PLoS One 10:e0116271. doi: 10.1371/journal.pone.0116271
Coutinho, F. H., Meirelles, P. M., Moreira, A. P. B., Paranhos, R. P., Dutilh, B. E., and Thompson, F. L. (2015). Niche distribution and influence of environmental parameters in marine microbial communities: a systematic review. PeerJ 2015:e1008. doi: 10.7717/peerj.1008
Delmont, T. O., Quince, C., Shaiber, A., Esen, ÖC., Lee, S. T., Rappé, M. S., et al. (2018). Nitrogen-fixing populations of Planctomycetes and Proteobacteria are abundant in surface ocean metagenomes. Nat. Microbiol. 3, 804–813. doi: 10.1038/s41564-018-0176-9
Dobal-Amador, V., Nieto-Cid, M., Guerrero-Feijoo, E., Hernando-Morales, V., Teira, E., and Varela, M. M. (2016). Vertical stratification of bacterial communities driven by multiple environmental factors in the waters (0-5000 m) off the Galician coast (NW Iberian margin). Deep. Res. Part I Oceanogr. Res. Pap. 114, 1–11. doi: 10.1016/j.dsr.2016.04.009
Frank, A. H., Garcia, J. A. L., Herndl, G. J., and Reinthaler, T. (2016). Connectivity between surface and deep waters determines prokaryotic diversity in the North Atlantic Deep Water. Environ. Microbiol. 18, 2052–2063. doi: 10.1111/1462-2920.13237
Frigaard, N. U., Martinez, A., Mincer, T. J., and DeLong, E. F. (2006). Proteorhodopsin lateral gene transfer between marine planktonic Bacteria and Archaea. Nature 439, 847–850. doi: 10.1038/nature04435
Galand, P. E., Pereira, O., Hochart, C., Auguet, J. C., and Debroas, D. (2018). A strong link between marine microbial community composition and function challenges the idea of functional redundancy. ISME J. 12, 2470–2478. doi: 10.1038/s41396-018-0158-1
García-García, N., Tamames, J., Linz, A. M., Pedrós-Alió, C., and Puente-Sánchez, F. (2019). Microdiversity ensures the maintenance of functional microbial communities under changing environmental conditions. ISME J. 13, 2969–2983. doi: 10.1038/s41396-019-0487-8
Ghai, R., Mizuno, C. M., Picazo, A., Camacho, A., and Rodriguez-Valera, F. (2013). Metagenomics uncovers a new group of low GC and ultra-small marine Actinobacteria. Sci. Rep. 3:2471. doi: 10.1038/srep02471
Giovannoni, S. J. (2017). SAR11 bacteria: the most abundant plankton in the oceans. Ann. Rev. Mar. Sci. 9, 231–255. doi: 10.1146/annurev-marine-010814-015934
Good, I. J. (1953). The population frequencies of species and the estimation of population parameters. Biometrika 40, 237–264. doi: 10.1093/biomet/40.3-4.237
Green, S. A., and Blough, N. V. (1994). Optical absorption and fluorescence properties of chromophoric dissolved organic matter in natural waters. Limnol. Oceanogr. 39, 1903–1916. doi: 10.4319/lo.1994.39.8.1903
Griffith, D. M., Veech, J. A., and Marsh, C. J. (2016). Cooccur: probabilistic species co-occurrence analysis in R. J. Stat. Softw. 69, 1–17. doi: 10.18637/jss.v069.c02
Guerrero-Feijóo, E., Nieto-Cid, M., Sintes, E., Dobal-Amador, V., Hernando-Morales, V., Álvarez, M., et al. (2017). Optical properties of dissolved organic matter relate to different depth-specific patterns of archaeal and bacterial community structure in the North Atlantic Ocean. FEMS Microbiol. Ecol. 93:fiw224. doi: 10.1093/femsec/fiw224
Guerrero-Feijóo, E., Sintes, E., Herndl, G. J., and Varela, M. M. (2018). High dark inorganic carbon fixation rates by specific microbial groups in the Atlantic off the Galician coast (NW Iberian margin). Environ. Microbiol. 20, 602–611. doi: 10.1111/1462-2920.13984
Gutiérrez-Barral, A., Teira, E., Hernández-Ruiz, M., and Fernández, E. (2021). Response of prokaryote community composition to riverine and atmospheric nutrients in a coastal embayment: role of organic matter on Vibrionales. Estuar. Coast. Shelf Sci. 151:107196. doi: 10.1016/j.ecss.2021.107196
Hansen, H. P., and Koroleff, F. (2007). “Determination of nutrients,” in Methods of Seawater Analysis: Third, Completely Revised and Extended Edition, eds K. Grasshoff, K. Kremling, and M. Ehrhardt (Weinheim: Wiley-VCH Verlag GmbH), 159–228. doi: 10.1002/9783527613984.ch10
Helms, J. R., Stubbins, A., Ritchie, J. D., Minor, E. C., Kieber, D. J., and Mopper, K. (2008). Absorption spectral slopes and slope ratios as indicators of molecular weight, source, and photobleaching of chromophoric dissolved organic matter. Limnol. Oceanogr. 53, 955–969. doi: 10.4319/lo.2008.53.3.0955
Herlemann, D. P. R., Labrenz, M., Jürgens, K., Bertilsson, S., Waniek, J. J., and Andersson, A. F. (2011). Transitions in bacterial communities along the 2000 km salinity gradient of the Baltic Sea. ISME J. 5, 1571–1579. doi: 10.1038/ismej.2011.41
Hernando-Morales, V., Varela, M. M., Needham, D. M., Cram, J., Fuhrman, J. A., and Teira, E. (2018). Vertical and seasonal patterns control bacterioplankton communities at two horizontally coherent coastal upwelling sites off galicia (NW Spain). Microb. Ecol. 76, 866–884. doi: 10.1007/s00248-018-1179-z
Howard, E. C., Henriksen, J. R., Buchan, A., Reisch, C. R., Bürgmann, H., Welsh, R., et al. (2006). Bacterial taxa that limit sulfur flux from the ocean. Science 314, 649–652. doi: 10.1126/science.1130657
Huse, S. M., Welch, D. M., Morrison, H. G., and Sogin, M. L. (2010). Ironing out the wrinkles in the rare biosphere through improved OTU clustering. Environ. Microbiol. 12, 1889–1898. doi: 10.1111/j.1462-2920.2010.02193.x
Iverson, V., Morris, R. M., Frazar, C. D., Berthiaume, C. T., Morales, R. L., and Armbrust, E. V. (2012). Untangling genomes from metagenomes: revealing an uncultured class of marine euryarchaeota. Science 335, 587–590. doi: 10.1126/science.1212665
Klindworth, A., Pruesse, E., Schweer, T., Peplies, J., Quast, C., Horn, M., et al. (2013). Evaluation of general 16S ribosomal RNA gene PCR primers for classical and next-generation sequencing-based diversity studies. Nucleic Acids Res. 41:e1. doi: 10.1093/nar/gks808
Koeppel, A., Perry, E. B., Sikorski, J., Krizanc, D., Warner, A., Ward, D. M., et al. (2008). Identifying the fundamental units of bacterial diversity: a paradigm shift to incorporate ecology into bacterial systematics. Proc. Natl. Acad. Sci. U.S.A. 105, 2504–2509. doi: 10.1073/pnas.0712205105
Landry, Z., Swa, B. K., Herndl, G. J., Stepanauskas, R., and Giovannoni, S. J. (2017). SAR202 genomes from the dark ocean predict pathways for the oxidation of recalcitrant dissolved organic matter. MBio 8, e413–e417. doi: 10.1128/mBio.00413-17
Langdon, C. (2010). “Determination of dissolved oxygen in seawater by winkler titration using the amperometric technique,” in GO-SHIP Repeat Hydrography Manual: A collection of Expert Reports and Guidelines, eds E. M. Hood, C. L. Sabine, and B. M. Sloyan 1–18.
Lavin, A., Valdes, L., Sánchez, F., Abaunza, P., Forest, A., Boucher, J., et al. (2006). “The bay of biscay: the encountering of the ocean and the shelf,” in The Sea, Volume 14B: The Global Coastal Ocean. Interdisciplinary Regional Studies and Syntheses, eds A. Robinson and K. Brink (Harvard University Press), 933–1001.
Legendre, P., and Gallagher, E. D. (2001). Ecologically meaningful transformations for ordination of species data. Oecologia 129, 271–280. doi: 10.1007/s004420100716
Li, Y., Sun, L. L., Sun, M. L., Su, H. N., Zhang, X. Y., Xie, B., et al. (2018). Vertical and horizontal biogeographic patterns and major factors affecting bacterial communities in the open South China Sea. Sci. Rep. 8:8800. doi: 10.1038/s41598-018-27191-w
Lima-Mendez, G., Faust, K., Henry, N., Decelle, J., Colin, S., Carcillo, F., et al. (2015). Determinants of community structure in the global plankton interactome. Science 348:1262073. doi: 10.1126/science.1262073
Lindh, M. V., and Pinhassi, J. (2018). Sensitivity of bacterioplankton to environmental disturbance: a review of baltic sea field studies and experiments. Front. Mar. Sci. 5:361. doi: 10.3389/fmars.2018.00361
Lønborg, C., and Álvarez-Salgado, X. A. (2014). Tracing dissolved organic matter cycling in the eastern boundary of the temperate North Atlantic using absorption and fluorescence spectroscopy. Deep Sea Res. Part I Oceanogr. Res. Pap. 85, 35–46. doi: 10.1016/J.DSR.2013.11.002
Martin, M. (2011). Cutadapt removes adapter sequences from high-throughput sequencing reads. EMBNET.J. 17:10. doi: 10.14806/ej.17.1.200
Massana, R., Murray, A. E., Preston, C. M., DeLong, E. F., Veth, C., Pernthaler, A., et al. (1997). Vertical distribution and phylogenetic characterization of marine planktonic Archaea in the Santa Barbara Channel. Appl. Environ. Microbiol. 63, 50–56. doi: 10.1128/aem.71.5.2303-2309.2005
McParland, E. L., and Levine, N. M. (2019). The role of differential DMSP production and community composition in predicting variability of global surface DMSP concentrations. Limnol. Oceanogr. 64, 757–773. doi: 10.1002/lno.11076
Mena, C., Reglero, P., Balbín, R., Martín, M., Santiago, R., and Sintes, E. (2020). Seasonal niche partitioning of surface temperate open ocean prokaryotic communities. Front. Microbiol. 11:1749. doi: 10.3389/fmicb.2020.01749
Mestre, M., Ruiz-González, C., Logares, R., Duarte, C. M., Gasol, J. M., and Sala, M. M. (2018). Sinking particles promote vertical connectivity in the ocean microbiome. Proc. Natl. Acad. Sci. U.S.A. 115, E6799–E6807. doi: 10.1073/pnas.1802470115
Milici, M., Deng, Z. L., Tomasch, J., Decelle, J., Wos-Oxley, M. L., Wang, H., et al. (2016). Co-occurrence analysis of microbial taxa in the Atlantic ocean reveals high connectivity in the free-living bacterioplankton. Front. Microbiol. 7:649. doi: 10.3389/fmicb.2016.00649
Miller, W. L., and Moran, M. A. (1997). Interaction of photochemical and microbial processes in the degradation of refractory dissolved organic matter from a coastal marine environment. Limnol. Oceanogr. 42, 1317–1324. doi: 10.4319/lo.1997.42.6.1317
Mizuno, C. M., Rodriguez-Valera, F., and Ghai, R. (2015). Genomes of planktonic acidimicrobiales: widening horizons for marine actinobacteria by metagenomics. MBio 6:e2083-14. doi: 10.1128/mBio.02083-14
Morris, J. J., Lenski, R. E., and Zinser, E. R. (2012). The black queen hypothesis: evolution of dependencies through adaptive gene loss. MBio 3:e36-12. doi: 10.1128/mBio.00036-12
Needham, D. M., Sachdeva, R., and Fuhrman, J. A. (2017). Ecological dynamics and co-occurrence among marine phytoplankton, bacteria and myoviruses shows microdiversity matters. ISME J. 11, 1614–1629. doi: 10.1038/ismej.2017.29
Nieto-Cid, M., Álvarez-Salgado, X. A., and Pérez, F. F. (2006). Microbial and photochemical reactivity of fluorescent dissolved organic matter in a coastal upwelling system. Limnol. Oceanogr. 51, 1391–1400. doi: 10.4319/lo.2006.51.3.1391
Oksanen, J., Blanchet, F. G., Kindt, R., Legendre, P., Minchin, P. R., O’Hara, R. B., et al. (2012). Vegan: Community Ecology Package. R Package Version 2.01-1.
Omori, Y., Saeki, A., Wada, S., Inagaki, Y., and Hama, T. (2020). Experimental analysis of diurnal variations in humic-like fluorescent dissolved organic matter in surface seawater. Front. Mar. Sci. 7:589064. doi: 10.3389/fmars.2020.589064
Pajares, S., and Ramos, R. (2019). Processes and microorganisms involved in the marine nitrogen cycle: knowledge and gaps. Front. Mar. Sci. 6:739. doi: 10.3389/fmars.2019.00739
Paliy, O., and Shankar, V. (2016). Application of multivariate statistical techniques in microbial ecology. Mol. Ecol. 25, 1032–1057. doi: 10.1111/mec.13536
Pedrós-Alió, C., Acinas, S. G., Logares, R., and Massana, R. (2018). “Marine microbial diversity as seen by high throughput sequencing,” in Microbial Ecology of the Oceans, eds J. M. Gasol and D. Kichman (New Jersey: Wiley-Blackwell), 592.
Pereira, O., Hochart, C., Boeuf, D., Auguet, J. C., Debroas, D., and Galand, P. E. (2021). Seasonality of archaeal proteorhodopsin and associated Marine Group IIb ecotypes (Ca. Poseidoniales) in the North Western Mediterranean Sea. ISME J. 15, 1302–1316. doi: 10.1038/s41396-020-00851-4
Prieto, E., González-Pola, C., Lavín, A., Sánchez, R. F., and Ruiz-Villarreal, M. (2013). Seasonality of intermediate waters hydrography west of the iberian peninsula from an 8 yr semiannual time series of an oceanographic section. Ocean Sci. 9, 411–429. doi: 10.5194/os-9-411-2013
Puillat, I., Lazure, P., Jégou, A. M., Lampert, L., and Miller, P. I. (2004). Hydrographical variability on the French continental shelf in the Bay of Biscay, during the 1990s. Cont. Shelf Res. 24, 1143–1163. doi: 10.1016/j.csr.2004.02.008
Pujalte, M. J., Lucena, T., Ruvira, M. A., Arahal, D. R., and Macián, M. C. (2014). “The family rhodobacteraceae,” in The Prokaryotes, eds E. Rosenberg, E. F. DeLong, S. Lory, E. Stackebrandt, and F. L. Thompson (Berlin: Springer), 439–512. doi: 10.1007/978-3-642-30197-1_377
Quast, C., Pruesse, E., Yilmaz, P., Gerken, J., Schweer, T., Yarza, P., et al. (2013). The SILVA ribosomal RNA gene database project: improved data processing and web-based tools. Nucleic Acids Res. 41, D590–D596. doi: 10.1093/nar/gks1219
Ramette, A. (2007). Multivariate analyses in microbial ecology. FEMS Microbiol. Ecol. 62, 142–160. doi: 10.1111/j.1574-6941.2007.00375.x
Reji, L., Tolar, B. B., Smith, J. M., Chavez, F. P., and Francis, C. A. (2019). Differential co-occurrence relationships shaping ecotype diversification within Thaumarchaeota populations in the coastal ocean water column. ISME J. 13, 1144–1158. doi: 10.1038/s41396-018-0311-x
Reshef, D. N., Reshef, Y. A., Finucane, H. K., Grossman, S. R., McVean, G., Turnbaugh, P. J., et al. (2011). Detecting novel associations in large data sets. Science 334, 1518–1524.
Reverdin, G., Marié, L., Lazure, P., d’Ovidio, F., Boutin, J., Testor, P., et al. (2013). Freshwater from the Bay of Biscay shelves in 2009. J. Mar. Syst. 109–110, S134–S143. doi: 10.1016/j.jmarsys.2011.09.017
Rinke, C., Rubino, F., Messer, L. F., Youssef, N., Parks, D. H., Chuvochina, M., et al. (2019). A phylogenomic and ecological analysis of the globally abundant marine group II archaea (Ca. Poseidoniales ord. nov.). ISME J. 13, 663–675. doi: 10.1038/s41396-018-0282-y
Ruíz-Villarreal, M., González-Pola, C., Sánchez, R., Plaza, F., Díaz del Río, G., Cabanas, J. M., et al. (2006). “Recent observations of mediterranean water in the bay of biscay,” in Proceeding of the International Conference on Oceanography of the Bay of Biscay, 277–280.
Salazar, G., Cornejo-Castillo, F. M., Benítez-Barrios, V., Fraile-Nuez, E., Álvarez-Salgado, X. A., Duarte, C. M., et al. (2016). Global diversity and biogeography of deep-sea pelagic prokaryotes. ISME J. 10, 596–608. doi: 10.1038/ismej.2015.137
Santoro, A. E., Richter, R. A., and Dupont, C. L. (2019). Planktonic marine archaea. Ann. Rev. Mar. Sci. 11, 131–158. doi: 10.1146/annurev-marine-121916-063141
Sebastián, M., Forn, I., Auladell, A., Gómez-Letona, M., Sala, M. M., Gasol, J. M., et al. (2021). Differential recruitment of opportunistic taxa leads to contrasting abilities in carbon processing by bathypelagic and surface microbial communities. Environ. Microbiol. 23, 190–206. doi: 10.1111/1462-2920.15292
Sogin, M. L., Morrison, H. G., Huber, J. A., Welch, D. M., Huse, S. M., Neal, P. R., et al. (2006). Microbial diversity in the deep sea and the underexplored “rare biosphere”. Proc. Natl. Acad. Sci. U.S.A. 103, 12115–12120. doi: 10.1073/pnas.0605127103
Sorokin, D. Y. (2003). Oxidation of inorganic sulfur compounds by obligately organotrophic bacteria. Microbiol. 726, 641–653. doi: 10.1023/B:MICI.0000008363.24128.E5
Stedmon, C. A., and Nelson, N. B. (2015). “The optical properties of DOM in the ocean,” in Biogeochemistry of Marine Dissolved Organic Matter, eds D. A. Hansell and C. A. Carlson (Academic Press), 481–508. doi: 10.1016/B978-0-12-405940-5.00010-8
Sunagawa, S., Coelho, L. P., Chaffron, S., Kultima, J. R., Labadie, K., Salazar, G., et al. (2015). Structure and function of the global ocean microbiome. Science 348:1261359. doi: 10.1126/science.1261359
R Core Team (2017). R: A Language and Environment for Statistical Computing. Vienna: R Foundation for Statistical Computing.
Tully, B. J. (2019). Metabolic diversity within the globally abundant marine group II euryarchaea offers insight into ecological patterns. Nat. Commun. 10, 1–12. doi: 10.1038/s41467-018-07840-4
Van Teeseling, M. C. F., Mesman, R. J., Kuru, E., Espaillat, A., Cava, F., Brun, Y. V., et al. (2015). Anammox planctomycetes have a peptidoglycan cell wall. Nat. Commun. 6, 1–6. doi: 10.1038/ncomms7878
Varela, M. M., Rodríguez-Ramos, T., Guerrero-Feijóo, E., and Nieto-Cid, M. (2020). Changes in activity and community composition shape bacterial responses to size-fractionated marine DOM. Front. Microbiol. 11:2635. doi: 10.3389/fmicb.2020.586148
Varela, M. M., Van Aken, H. M., and Herndl, G. J. (2008). Abundance and activity of Chloroflexi-type SAR202 bacterioplankton in the meso- and bathypelagic waters of the (sub)tropical Atlantic. Environ. Microbiol. 10, 1903–1911. doi: 10.1111/j.1462-2920.2008.01627.x
Varela, M. M., van Aken, H. M., Sintes, E., Reinthaler, T., and Herndl, G. J. (2011). Contribution of crenarchaeota and bacteria to autotrophy in the North Atlantic interior. Environ. Microbiol. 13, 1524–1533. doi: 10.1111/j.1462-2920.2011.02457.x
Veech, J. A. (2013). A probabilistic model for analysing species co-occurrence. Glob. Ecol. Biogeogr. 22, 252–260. doi: 10.1111/j.1466-8238.2012.00789.x
Zhang, C. L., Xie, W., Martin-Cuadrado, A. B., and Rodriguez-Valera, F. (2015). Marine group II Archaea, potentially important players in the global ocean carbon cycle. Front. Microbiol. 6:1108. doi: 10.3389/fmicb.2015.01108
Zobell, C. E., and Anderson, D. Q. (1936). Observations on the multiplication of bacteria in different volumes of stored sea water and the influence of oxygen tension and solid surfaces. Biol. Bull. 71, 324–342. doi: 10.2307/1537438
Keywords: Archaea, bacteria, diversity, co-occurrence, dissolved organic matter, DOM optical properties, ecotypes, niche partitioning
Citation: Rodríguez-Ramos T, Nieto-Cid M, Auladell A, Guerrero-Feijóo E and Varela MM (2021) Vertical Niche Partitioning of Archaea and Bacteria Linked to Shifts in Dissolved Organic Matter Quality and Hydrography in North Atlantic Waters. Front. Mar. Sci. 8:673171. doi: 10.3389/fmars.2021.673171
Received: 26 February 2021; Accepted: 19 July 2021;
Published: 12 August 2021.
Edited by:
Tony Gutierrez, Heriot-Watt University, United KingdomReviewed by:
Kai Ziervogel, University of New Hampshire, United StatesJiwen Liu, Ocean University of China, China
Copyright © 2021 Rodríguez-Ramos, Nieto-Cid, Auladell, Guerrero-Feijóo and Varela. This is an open-access article distributed under the terms of the Creative Commons Attribution License (CC BY). The use, distribution or reproduction in other forums is permitted, provided the original author(s) and the copyright owner(s) are credited and that the original publication in this journal is cited, in accordance with accepted academic practice. No use, distribution or reproduction is permitted which does not comply with these terms.
*Correspondence: Tamara Rodríguez-Ramos, tamara.rodriguez@ieo.es