- Department of Neurosurgery, Graduate School of Biomedical Sciences, Nagasaki University, Nagasaki, Japan
Background: Carotid artery stenosis is the cause of 15% of strokes. Neutrophil extracellular traps (NETs) and peptidyl arginine deiminase 4 (PAD4) are believed to be involved in thrombosis. This pilot study described the differential expression profile of NETs between atheromatous plaques and surrounding tissues.
Methods: Microarray datasets of carotid plaques were obtained from Gene Expression Omnibus. The normalized data were processed into comma-separated value matrix files using spreadsheet software. Analyzes of microarray data were conducted using integrated differential expression and pathway analysis.
Result: The clustering results illustrated that the classifications of plaque and control had reasonable biological validity. Pathway analysis revealed the relevance of immune response, cell signaling, and other pathways. Differentially expressed genes were detected between carotid plaques and control specimens. However, enrichment analyzes did not reveal a difference in PAD4 expression between the groups and that NET implication was only found in one cDNA microarray dataset.
Discussion: This pilot study does not necessarily dismiss the possibility of a relationship between NETs and atherothrombotic stroke. Gene expression could differ between endothelial cells and atheromas, and further studies are needed.
1 Introduction
Carotid artery stenosis is the cause of 15% of strokes (1, 2). Based on early histopathologic studies, ischemic events are associated with intraplaque hemorrhage, ulceration, calcification, lipid-rich necrosis, plaque thrombus, macrophage infiltration, and high microvessel density (3–6).
Neutrophil extracellular traps (NETs) are specialized structures released by neutrophils. NETs were initially believed to form in response to stimuli such as infection and inflammation and contribute to the elimination of pathogens such as bacteria and viruses (7). Recently, they have been suggested to participate in the regulation of inflammatory responses, blood coagulation, and pathological conditions such as autoimmune diseases and thrombosis (8–10). Elevated peptidyl arginine deiminase 4 (PAD4) levels have been detected in blood samples collected during carotid artery stenting, suggesting the involvement of NETs in the pathogenesis of atherothrombotic stroke (11).
Microarray and ribonucleic acid sequencing (RNAseq) allow comprehensive analyzes of transcriptomes. Genome-wide transcriptome analysis is often required in addition to individual gene expression analyzes. There are already re-analysis reports of existing microarray data (12, 13). However, big data analysis requires knowledge of statistics, informatics, and data science, which can pose difficulties for general biologists, physicians, and surgeons (14, 15).
In the absence of a bioinformatics expert, this study analyzed whether correlations related to NETs could be detected using historical carotid plaque-derived complementary deoxyribonucleic acid (cDNA) microarray data.
2 Materials and methods
Based on national ethical guidelines, this study did not originally fall under the category of research requiring written consent from study participants (16). This study was approved by the Institutional Review Board (number 23071016). The Gene Expression Omnibus1 database was examined using the search terms human, carotid artery, and endarterectomy. Twelve data were found as of October 2023. GSE28829 and GSE43292 datasets, which appeared to compare plaque and normal to early atheromatous vessels, were selected for the present analysis (Table 1) (17, 18). The downloaded normalized data were converted to comma-separated value (CSV) matrix files using spreadsheet software. An outline of the strategy used for the GEO original data is provided in the Supplementary Files S1–S4. Analyzes of microarray data were conducted using integrated Differential Expression and Pathway analysis (iDEP) 1.1 (19).2 The detailed methods and R session information are provided in the Supplementary File S5.
3 Results
3.1 Heatmap, principal component analysis, and differential expression analysis
The elimination q-value (false discovery rate [FDR]) was 0.10 in the iDEP computation. The clustering results indicated that the pre-specified classification of plaque and control specimens had more than moderate biological validity (Figures 1A,B). In PCA, principal component 1 (PC1) was mainly relevant to immune response, and PC2 was related to cell signaling, tissue development, neurogenesis, and other pathways (Figure 1C; Supplementary Figure S1). Differentially expressed genes (DEGs) of advanced carotid plaque were detected. Compared to microscopically normal artery, 87 upregulated and 60 downregulated DEGs were detected in advanced carotid plaque in the GSE43292 dataset (q < 0.1; Figure 2). In comparison with early plaques, 396 upregulated and 71 downregulated genes were detected in advanced carotid plaque in the GSE28829 dataset (q < 0.1; Supplementary Figure S1). See the Supplementary Files for detailed specific genes (Supplementary Files S6, S8).
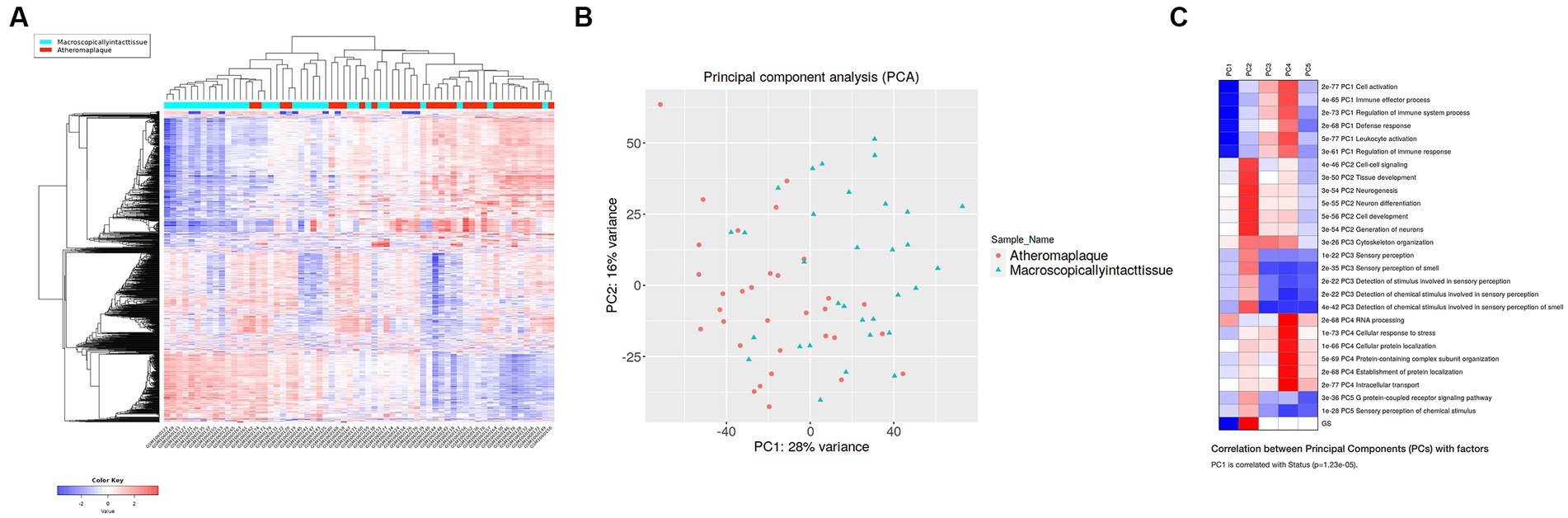
Figure 1. Hierarchical clustering heatmap (A) and principal component analysis (B) of advanced (unstable) and early (stable) atherosclerotic arteries from the GSE43292 dataset. The clusters are separated according to the pre-specified classification (plaque or normal), and the comparisons are likely to be meaningful. (C) GSE43292 pathway analysis of the PCA rotation matrix displays gene groups extracted using the results of principal component analysis. Inflammation, immune response, and other pathways were extracted.
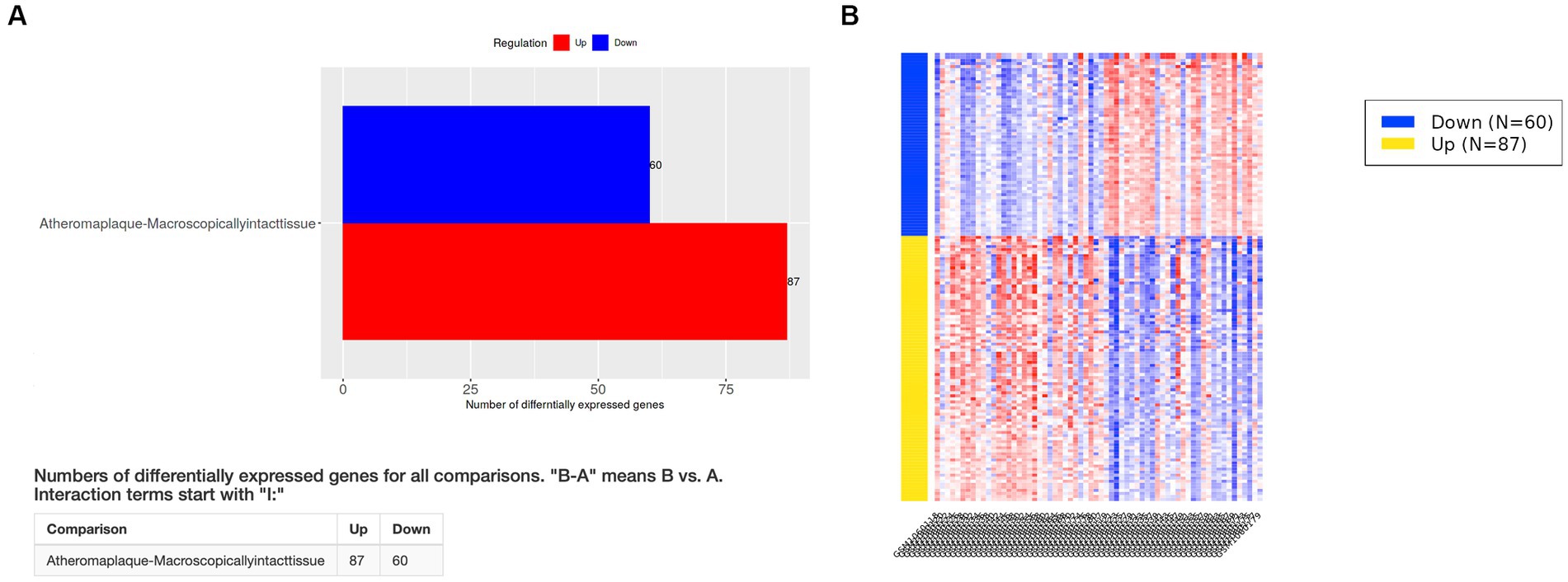
Figure 2. Differentially expressed genes (DEGs) in the GSE43292 dataset. (A) Compared to the findings for control specimens (microscopically normal artery), 87 upregulated and 60 downregulated DEGs were detected in advanced carotid plaque (q < 0.1). (B) Heatmap of DEGs in the GSE43292 dataset. See the Supplementary Files for detailed data (Supplementary Files S6, S7).
3.2 Enrichment and pathway analyzes
Pathway analysis was performed using Generally Applicable Gene-set Enrichment for Pathway Analysis (20) and Gene Ontology (21), and the selected gene sets were obtained from the Kyoto Encyclopedia of Genes and Genomes (KEGG) (22). The pathway significance cutoff (FDR) was 0.2. The main results are summarized in Table 2. The NET formation was enriched as a significant pathway only in the GSE43292 dataset, and DEGs were presented on the KEGG graph. PAD4 was not identified as a DEG in the expression analyzes. The two datasets shared the same reduced expression of histone deacetylase (HDAC), but differences were observed for histone expression (Figure 3; Supplementary Figure S1). See the Supplementary Files for detailed specific pathways (Supplementary Files S7, S9).
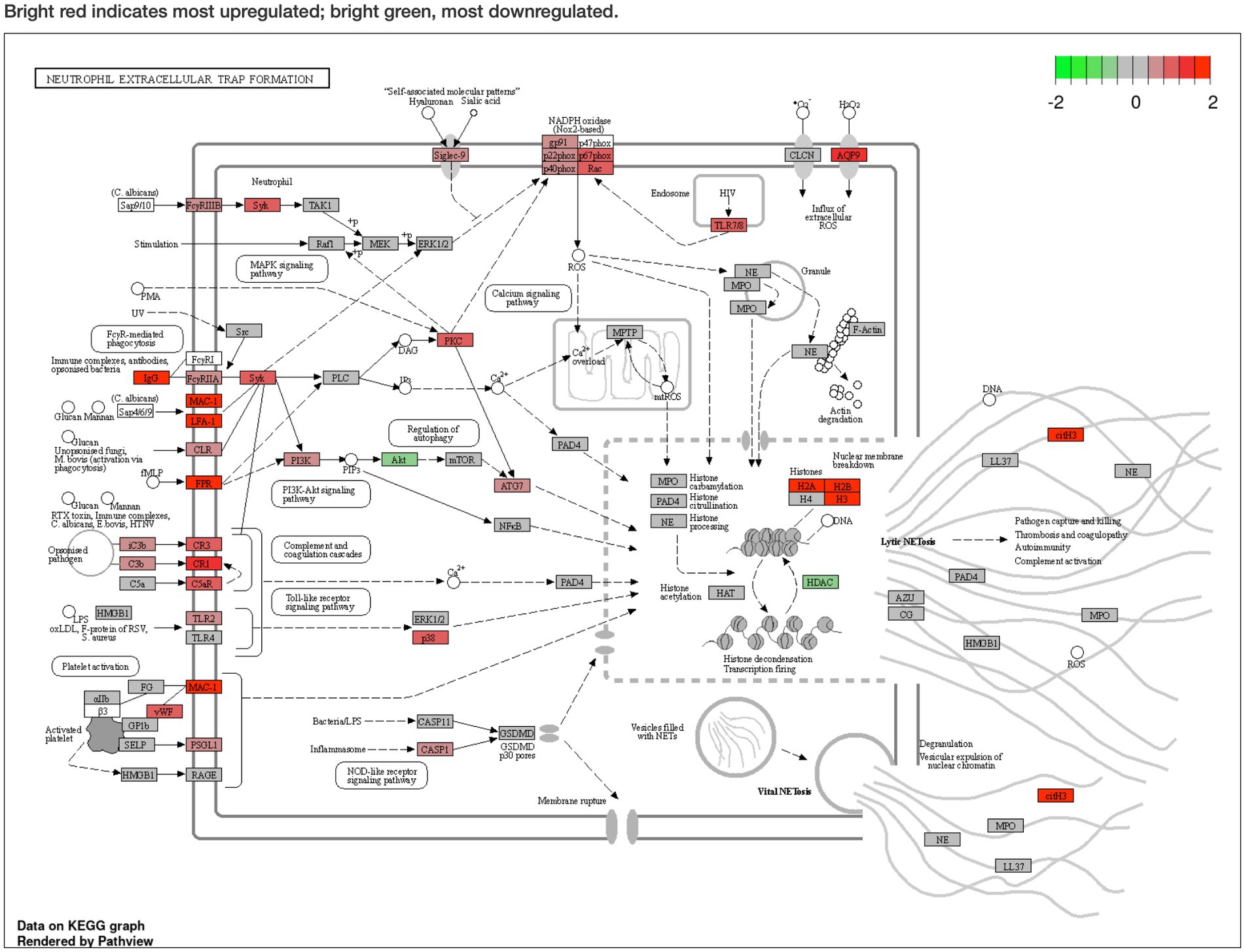
Figure 3. Pathway analysis of the GSE43292 dataset described in Kyoto Encyclopedia of Genes and Genomes graph. Neutrophil extracellular trap formation is enriched (false discovery rate < 0.2). Peptidyl arginine deiminase 4 (PAD4) expression was not significantly elevated. Histone deacetylase expression was reduced. Bright red indicates most upregulated; bright green, most downregulated. The KEGG pathway map (hsa04613 Neutrophil extracellular trap formation) is reprinted with permission from Kanehisa Laboratories.(20)
4 Discussion
Existing cDNA microarray data on carotid plaques of human origin represent a valuable source of information as they can be repeatedly analyzed to reflect the latest research, depending on the researcher’s interest. Nai et al. reported a re-analysis of the GSE43292 dataset and explored novel genes and pathways of carotid atheroma (12). Gao et al. examined immune cell infiltration between early and advanced carotid atheromatous plaque using the GSE28829 dataset (13). The cooperation of bioinformatics experts is considered essential for the former consideration. On the other hand, the latter report uses a web tool and does not necessarily require an expert, which could be another option from the present study. Our study presented a method for uploading normalized CSV matrix files to the iDEP web platform and analyzing the data (see legends in Supplementary Files S1–S4). All analyzes were performed on a graphical user interface such that the character user interface was avoided. As the analysis is performed online, a computer with standard performance was sufficient. Some typical cDNA microarray and RNAseq analysis methods are available and free of charge for scientific use (15, 19). We adopted this method in the present study because it allows visualization and display of the NETs’ DEG information on the KEGG graph.
The organization of the controls was not consistent in the present study (Table 1). Data from GSE28829 compared advanced plaque with intimal thickening and intimal xanthoma, and advanced plaque and distant macroscopically intact tissues were compared in GSE43292. One possible reason for the discrepancies between the results of the two datasets in this study could be that the former detected mainly DEGs associated with plaque progression, while the latter detected mainly DEGs associated with plaque development. The lack of control samples compared to the number of validation samples in the GSE28829 data may have also affected the results. Conversely, it remains nearly impossible to obtain human-derived normal arterial tissue as control samples from an ethical viewpoint.
High PAD4 expression was not extracted as a DEG in our re-analysis of existing microarray data. This finding is inconsistent with that reported by Simonaga et al. (11). They collected blood samples from the luminal side, which could represent a different target from our study results, in which atheromas were analyzed. In other words, it is possible that different genes could be expressed in vascular endothelial cells and atheromas even though both contribute to a series of atherosclerotic processes. Therefore, we cannot exclude the possibility that NETs are involved in the development of carotid artery plaques and their rupture. Clinicopathological studies and single-cell comprehensive gene expression analyzes could be helpful for clarifying their pathogenesis.
Several limitations to this study warrant mention. Because of the inconsistency of the controls, whether they represented normal tissue may be debatable (Table 1). Next, microarrays are not chip-compatible, making integrated analysis extremely difficult. Then, although the results of analyzes of cDNA microarray and RNAseq data can suggest certain correlations, causal relationships cannot always be proven. Furthermore, scientists should consider the final biological interpretation as the results of big data and machine learning do not necessarily have biological relevance (15, 23). Finally, this research is an analysis that is only possible within the platform created by bioinformatics researchers. The need to rely on experts will continue to be necessary when detailed fine-tuning or new analysis methods are required.
Data availability statement
The original contributions presented in the study are included in the article/Supplementary material, further inquiries can be directed to the corresponding author.
Ethics statement
The studies involving humans were approved by the Nagasaki University Hospital Institutional Review Board. The studies were conducted in accordance with the local legislation and institutional requirements. The human samples used in this study were acquired from another research group. Written informed consent for participation was not required from the participants or the participants’ legal guardians/next of kin in accordance with the national legislation and institutional requirements.
Author contributions
RT: Conceptualization, Data curation, Formal analysis, Methodology, Writing – original draft, Writing – review & editing. KU: Conceptualization, Data curation, Formal analysis, Funding acquisition, Methodology, Project administration, Resources, Software, Writing – original draft, Writing – review & editing. TI: Conceptualization, Formal analysis, Funding acquisition, Investigation, Project administration, Supervision, Validation, Visualization, Writing – original draft, Writing – review & editing. AX: Data curation, Writing – review & editing. KO: Writing – review & editing. YM: Funding acquisition, Writing – review & editing. TM: Funding acquisition, Supervision, Writing – review & editing.
Funding
The author(s) declare financial support was received for the research, authorship, and/or publication of this article. This study was supported by Grants-in-Aid for Scientific Research from JSPS KAKENHI (C) 20 K09351 (to YM), (C) 21 K09154 (to KU), (C) 21 K09180 (to TI), and (C) 21 K09129 (to TM) and from Fostering Joint International Research 20KK0254 (to YM).
Acknowledgments
The authors would like to pay tribute to the original plaque research teams (17, 18), the developers of bioinformatics tools (19–22, 24), and the sample donors to GEO. In this study, the authors referred to the Online Mendelian Inheritance in Man and Ensembl database (25, 26). The R logo is (C) 2016 The R Foundation, released under the terms of the Creative Commons Attribution-ShareAlike 4.0 International License (CC-BY-SA 4.0; https://www.r-project.org) (24). The copyright holder of the KEGG pathway map (hsa04613 Neutrophil extracellular trap formation) is Kanehisa Laboratories (22). We would also like to thank Enago for the English language review.
Conflict of interest
The authors declare that the research was conducted in the absence of any commercial or financial relationships that could be construed as a potential conflict of interest.
The reviewer TF declared a shared affiliation with the authors to the handling editor at the time of review.
Publisher’s note
All claims expressed in this article are solely those of the authors and do not necessarily represent those of their affiliated organizations, or those of the publisher, the editors and the reviewers. Any product that may be evaluated in this article, or claim that may be made by its manufacturer, is not guaranteed or endorsed by the publisher.
Supplementary material
The Supplementary material for this article can be found online at: https://www.frontiersin.org/articles/10.3389/fneur.2023.1267136/full#supplementary-material
Supplementary Datasheet 1-4 | Data for integrated Differential Expression and Pathway analysis. Matrix data were processed using spreadsheet software and saved as CSV files (Suppl1GSE28829_series_matrix, and Suppl3GSE43292_series_matrix). Attribute data were additionally attached (Suppl2GSE28829_series_attribute, and Suppl4GSE43292_series_attribute). When you process original Gene Expression Omnibus data, (1) the 1 × 1 cell must be blank data. (2) Paste the gene name or ID column from the 2 × 1 cell. Select the column, and specify the display format as a character string. (3) Provide the names of the specimens from the 1 × 2 cell. (4) Paste the expression data and complete the matrix data. (5) Remove the unnecessary description of statistics and other data. Unnecessary cells, columns, and rows should be removed. (6) Save the matrix as a CSV file. (15) See the Suppl1GSE28829_series_matrix and Suppl3GSE43292_series_matrix files for examples.
Supplementary Datasheet 5 | GSE43292 differentially expressed genes.
Supplementary Datasheet 6 | GSE43292 enriched phenomena.
Supplementary File 7 | GSE28829 differentially expressed genes.
Supplementary File 8 | GSE28829 enriched phenomena.
Abbreviations
cDNA, complementary deoxyribonucleic acid; CSV, comma-separated value; DEGs, differentially expressed genes; FDR, false discovery rate; GEO, Gene Expression Omnibus; HDAC, histone deacetylase; iDEP, integrated Differential Expression and Pathway analysis; KEGG, Kyoto Encyclopedia of Genes and Genomes; NETs, neutrophil extracellular traps; PAD4, peptidyl arginine deiminase 4; PC, principal component; PCA, principal component analysis; RNAseq, ribonucleic acid sequencing
Footnotes
References
1. Rothwell, PM, Eliasziw, M, Gutnikov, SA, Warlow, CP, and Barnett, HJ, Carotid Endarterectomy Trialists Collaboration. Endarterectomy for symptomatic carotid stenosis in relation to clinical subgroups and timing of surgery. Lancet. (2004) 363:915–24. doi: 10.1016/S0140-6736(04)15785-1
2. Chaturvedi, S, Bruno, A, Feasby, T, Holloway, R, Benavente, O, Cohen, SN, et al. Carotid endarterectomy--an evidence-based review: report of the Therapeutics and Technology Assessment Subcommittee of the American Academy of Neurology. Neurology. (2005) 65:794–801. doi: 10.1212/01.wnl.0000176036.07558.82
3. Imparato, AM, Riles, TS, and Gorstein, F. The carotid bifurcation plaque: pathologic findings associated with cerebral ischemia. Stroke. (1979) 10:238–45. doi: 10.1161/01.STR.10.3.238
4. Fryer, JA, Myers, PC, and Appleberg, M. Carotid intraplaque hemorrhage: the significance of neovascularity. J Vasc Surg. (1987) 6:341–9. doi: 10.1016/0741-5214(87)90004-8
5. Virmani, R, Kolodgie, FD, Burke, AP, Farb, A, and Schwartz, SM. Lessons from sudden coronary death: a comprehensive morphological classification scheme for atherosclerotic lesions. Arterioscler Thromb Vasc Biol. (2000) 20:1262–75. doi: 10.1161/01.ATV.20.5.1262
6. Howard, DP, Van Lammeren, GW, Rothwell, PM, Redgrave, JN, Moll, FL, De Vries, JP, et al. Symptomatic carotid atherosclerotic disease: correlations between plaque composition and ipsilateral stroke risk. Stroke. (2015) 46:182–9. doi: 10.1161/STROKEAHA.114.007221
7. Brinkmann, V, Reichard, U, Goosmann, C, Fauler, B, Uhlemann, Y, Weiss, DS, et al. Neutrophil extracellular traps kill bacteria. Science. (2004) 303:1532–5. doi: 10.1126/science.1092385
8. Sorensen, OE, and Borregaard, N. Neutrophil extracellular traps - the dark side of neutrophils. J Clin Invest. (2016) 126:1612–20. doi: 10.1172/JCI84538
9. Papayannopoulos, V. Neutrophil extracellular traps in immunity and disease. Nat Rev Immunol. (2018) 18:134–47. doi: 10.1038/nri.2017.105
10. Franck, G, Mawson, TL, Folco, EJ, Molinaro, R, Ruvkun, V, Engelbertsen, D, et al. Roles of PAD4 and NETosis in experimental atherosclerosis and arterial injury: implications for superficial Erosion. Circ Res. (2018) 123:33–42. doi: 10.1161/CIRCRESAHA.117.312494
11. Shimonaga, K, Matsushige, T, Takahashi, H, Hashimoto, Y, Yoshiyama, M, Ono, C, et al. Peptidylarginine deiminase 4 as a possible biomarker of plaque instability in carotid artery stenosis. J Stroke Cerebrovasc Dis. (2021) 30:105816. doi: 10.1016/j.jstrokecerebrovasdis.2021.105816
12. Nai, W, Threapleton, D, Lu, J, Zhang, K, Wu, H, Fu, Y, et al. Identification of novel genes and pathways in carotid atheroma using integrated bioinformatic methods. Sci Rep. (2016) 6:18764. doi: 10.1038/srep18764
13. Gao, J, Shi, L, Gu, J, Zhang, D, Wang, W, Zhu, X, et al. Difference of immune cell infiltration between stable and unstable carotid artery atherosclerosis. J Cell Mol Med. (2021) 25:10973–9. doi: 10.1111/jcmm.17018
14. Ujifuku, K, Fujimoto, T, Sato, K, Morofuji, Y, Muto, H, Masumoto, H, et al. Exploration of Pericyte-derived factors implicated in lung Cancer brain metastasis protection: a pilot messenger RNA sequencing using the blood-brain barrier in vitro model. Cell Mol Neurobiol. (2022) 42:997–1004. doi: 10.1007/s10571-020-00988-y
15. Ujifuku, K, Morofuji, Y, and Masumoto, H. RNA sequencing data analysis on the maser platform and the tag-count comparison graphical userInterface. Methods Mol Biol. (2022) 2535:157–70. doi: 10.1007/978-1-0716-2513-2_13
16. Eba, J, and Nakamura, K. Overview of the ethical guidelines for medical and biological research involving human subjects in Japan. Jpn J Clin Oncol. (2022) 52:539–44. doi: 10.1093/jjco/hyac034
17. Ayari, H, and Bricca, G. Identification of two genes potentially associated in iron-heme homeostasis in human carotid plaque using microarray analysis. J Biosci. (2013) 38:311–5. doi: 10.1007/s12038-013-9310-2
18. Doring, Y, Manthey, HD, Drechsler, M, Lievens, D, Megens, RT, Soehnlein, O, et al. Auto-antigenic protein-DNA complexes stimulate plasmacytoid dendritic cells to promote atherosclerosis. Circulation. (2012) 125:1673–83. doi: 10.1161/CIRCULATIONAHA.111.046755
19. Ge, SX, Son, EW, and Yao, R. iDEP: an integrated web application for differential expression and pathway analysis of RNA-Seq data. BMC Bioinformatics. (2018) 19:534. doi: 10.1186/s12859-018-2486-6
20. Luo, W, Friedman, MS, Shedden, K, Hankenson, KD, and Woolf, PJ. GAGE: generally applicable gene set enrichment for pathway analysis. BMC Bioinformatics. (2009) 10:161. doi: 10.1186/1471-2105-10-161
21. Gene Ontology, C, Aleksander, SA, Balhoff, J, Carbon, S, Cherry, JM, Drabkin, HJ, et al. The Gene Ontology knowledgebase in 2023. Genetics. (2023) 224:31. doi: 10.1093/genetics/iyad031
22. Kanehisa, M, and Goto, S. KEGG: Kyoto encyclopedia of genes and genomes. Nucleic Acids Res. (2000) 28:27–30. doi: 10.1093/nar/28.1.27
23. Ching, T, Himmelstein, DS, Beaulieu-Jones, BK, Kalinin, AA, Do, BT, Way, GP, et al. Opportunities and obstacles for deep learning in biology and medicine. J R Soc Interface. (2018) 15:20170387. doi: 10.1098/rsif.2017.0387
24. R Core Team. R: A language and environment for statistical computing. Vienna, Austria: R Core Team (2019).
25. Amberger, J, Bocchini, C, and Hamosh, A. A new face and new challenges for online Mendelian inheritance in man (OMIM(R)). Hum Mutat. 32:564–7. doi: 10.1002/humu.21466
Keywords: atheromatous plaque, carotid endarterectomy, cDNA microarray, neutrophil extracellular traps, peptidyl arginine deiminase 4
Citation: Takahira R, Ujifuku K, Izumo T, Xie A, Okamura K, Morofuji Y and Matsuo T (2023) Do neutrophil extracellular traps implicate in atheromatous plaques from carotid endarterectomy? Re-analyzes of cDNA microarray data by surgeons. Front. Neurol. 14:1267136. doi: 10.3389/fneur.2023.1267136
Edited by:
Yanlin Zhang, Second Affiliated Hospital of Soochow University, ChinaReviewed by:
Manoj Kumar Tembhre, All India Institute of Medical Sciences, IndiaTakashi Fujimoto, Nagasaki University, Japan
Manovriti Thakur, University of Bern, Switzerland
Copyright © 2023 Takahira, Ujifuku, Izumo, Xie, Okamura, Morofuji and Matsuo. This is an open-access article distributed under the terms of the Creative Commons Attribution License (CC BY). The use, distribution or reproduction in other forums is permitted, provided the original author(s) and the copyright owner(s) are credited and that the original publication in this journal is cited, in accordance with accepted academic practice. No use, distribution or reproduction is permitted which does not comply with these terms.
*Correspondence: Tsuyoshi Izumo, go-izumo@hotmail.co.jp
†These authors have contributed equally to this work