- 1LongHua Hospital Shanghai University of Traditional Chinese Medicine, Shanghai, China
- 2Shanghai Ninth People’s Hospital Affiliated to School of Medicine, Shanghai Jiao Tong University, Shanghai, China
Siteng Fang (STF) has been shown to inhibit migration, invasion, and adhesion as well as promote apoptosis in gastric cancer (GC) cells. However, whether it can reverse the multidrug resistance (MDR) of GC to chemotherapy drugs is unknown. Thus, we aimed to elucidate the mechanism of STF in reversing the MDR of GC. The chemical composition of STF and genes related to GC were obtained from the TCMNPAS(TCM Network Pharmacology Analysis System, TCMNPAS) Database, and the targets of the active ingredients were predicted using the Swiss Target Prediction Database. The obtained data were mapped to obtain the key active ingredients and core targets of STF in treating GC. The active component-target network and protein interaction network were constructed by Cytoscape and String database, and the key genes and core active ingredients were obtained. The biological functions and related signal pathways corresponding to the key targets were analyzed and then verified via molecular docking. A total of 14 core active ingredients of STF were screened, as well as 20 corresponding targets, which were mainly enriched in cancer pathway, proteoglycan synthesis, PI3K-AKT signaling pathway, and focal adhesion. Molecular docking showed that the core active ingredients related to MDR, namely quercetin and diosgenin, could bind well to the target. In summary, STF may reverse the MDR of GC and exert synergistic effect with chemotherapeutic drugs. It mediates MDR mainly through the action of quercetin and diosgenin on the PI3K/AKT signaling pathway. These findings are the first to demonstrate the molecular mechanism of STF in reversing MDR in GC, thus providing a direction for follow-up basic research.
Introduction
Gastric cancer (GC) is one of five cancers with the highest incidence in China (1). According to the National Cancer Center, the incidence and mortality of GC ranked second among malignant tumors in China in 2015, and showed an increasing trend each year (2). Moreover, the early diagnosis rate of GC in China is low; patients with GC are often diagnosed with advanced disease. Thus, GC is a serious threat to the quality of life and health of the Chinese population. Chemotherapy is one of the important approaches to GC treatment. However, multidrug resistance (MDR) has become a common phenomenon, and it is a main reason for chemotherapy failure, making it difficult to improve the survival rate of patients with advanced GC (3). Therefore, overcoming the MDR of GC to chemotherapy and improving the efficacy of anticancer drugs are key issues in the global medical community.
Traditional Chinese medicine (TCM) has been used for the treatment of GC, and clinical studies have shown that TCM can improve the effect of western medicine, reduce the size and clinical stage of tumor, reverse MDR to chemotherapy drugs, relieve adverse reactions to chemotherapy, and improve the quality of life of patients (4). Weichang’an decoction was developed based on the clinical experience of Professor Qiu Jiaxin, a famous TCM doctor in Shanghai, China. It has been used in clinical setting as a hospital preparation for more than 30 years, and its efficacy has been confirmed by experimental studies. Weichang’an decoction can inhibit the metastasis and invasion of GC cells, induce apoptosis, regulate immunity, and regulate the expression of multiple genes (5); Siteng Fang (STF), which is composed of Radix Actinidiae Chinensis, wild grape, Sargentodoxa vine, and Chinaroot Greenbrier Rhizome Catbriar, is a small prescription of Weichang’an decoction. Its main efficacies are clearing away heat as well as detoxifying and eliminating pathogenic factors. Clinical research has demonstrated that STF improves the clinical efficacy of chemotherapy drugs and the quality of life of patients with GC (6–8). In addition, STF could inhibit the migration, invasion, and adhesion of GC cells and promote apoptosis (9). We have also previously observed a capacity of STF to reverse MDR (currently not reported). However the underlying molecular mechanism of MDR reversal remains unclear. Therefore, in this study, we aimed to clarify the molecular mechanism of STF in the treatment of GC. For this purpose, we used a combinatorial approach of network pharmacology and molecular docking technology. The findings of our study are the first to report the molecular mechanism of STF in reversing the MDR of GC, and thus will provide a direction for follow-up basic research.
Materials and Methods
Screening of Active Ingredients
The network pharmacology analysis system of TCM was developed by Yang Ming, the director of Longhua Hospital Affiliated to Shanghai University of traditional Chinese Medicine (TCM Network Pharmacology Analysis System, TCMNPAS, National Computer Software Registration No. 2019SR1127090) (10). The Chinese names of Radix Actinidiae chinensis (mihoutaogen), wild grape (yeputaogen), Sargentodoxa vine (daxueteng), and Chinaroot Greenbier Rhizome Catbriar (baqia) were input into the retrieval module of chemical constituents. The Traditional Chinese Medicine Systems Pharmacology Database and Analysis Platform (TCMSP) Version 2.3, Traditional Chinese Medicine Integrated Database (TCMID) Version 2.0, and the Herbal Ingredients’ Targets (HIT) Database were screened simultaneously, with TCMNPAS linked to the databases, to determine the chemical composition of STF (11–13). The active STF ingredients were screened based on oral bioavailability (OB) ≥ 30% and drug-likeness (DL) ≥ 0.18 (14) pharmacokinetic characteristics.
Prediction of Drug Targets
To obtain the potential targets of the active ingredients of STF, the SMILES strings obtained from the TCMNPAS Database, were imported into the Swiss Target Prediction Database. Targets with 0 probability were deleted (15).
Collection of GC Related Targets
Disease ID was obtained by inputting the disease keyword “gastric cancer” to the disease gene retrieval module of TCMNPAS. The background automatically connected to GeneCard Database to obtain disease gene and then downloaded and saved the results as GC related targets (16).
Screening of Drug-Disease Key Targets
The targets of the active components of STF and GC-related targets were introduced into venny2.1.0 (https://bioinfogp.cnb.csic.es/tools/venny/index.html), and the intersection was regarded as the target protein of STF in treating GC. Cytoscape 3.7.0 (17) was used to analyze network topology parameters, and the active component-target-disease network diagram was constructed for visualization. Next, to obtain the protein interaction network diagram, the above-mentioned cross-proteins were input in String Database (https://string-db.org), the species was set as “Homo sapiens”, and the minimum interaction score was set as 0.4. Finally, the protein interaction data were imported into Cytoscape 3.7.0 to analyze the network topology parameters. The proteins above the median of “Degree” were selected as the key targets, and the corresponding chemical components were the core active components.
Enrichment Analysis of Key Targets
The target obtained from section Screening of Drug-Disease Key Targets was transferred into DAVID6.8 (https://david.ncifcrf.Gov) for Gene Ontology (GO) functional enrichment analysis and Kyoto Gene and Genome Encyclopedia (KEGG) pathway enrichment analysis. The species was set as “Homo sapiens”, and the result was set as P<0.05. GO functional enrichment analysis describes the possible molecular functions of target products, the biological processes involved, and the cellular environment. KEGG pathway enrichment analysis indicates the most significant biological process by classifying the known genome annotation information. Therefore, these methods can predict the potential active components involved in the action mechanism of STF in treating GC.
Molecular Docking Analysis
The protein IDs of the key targets were obtained from the utility module of TCMNPAS and converted into Protein Data Bank (PDB) IDs (every molecular model in the PDB has a unique accession or identification code). The 3D structure of the key proteins in PDB format were downloaded from the PDB Database (https://www.rcsb.org/). Subsequently, the SMILES strings of the active ingredient numbers, PDB IDs of the key targets, and 3D structure in PDB format obtained from 2.1 were inputted to the molecular docking module of TCMNPAS. The PSOVina algorithm was optimized based on the autodock Vina molecular docking algorithm and used PSOVina for docking (18–21). The energy range was set to 3, the accuracy was 8, and the output was 9 prediction results. The method of protein docking pocket parameters was FromLigand. Finally, the conformation and docking results of the compounds were downloaded and saved. The compound and target protein formats were converted into PDB format by Open Babel (22) and then imported into PyMOL 3.8 (https://pymol.org) to obtain 3D images of molecular docking. When the binding energy is less than -5 kJ/mol, the ligand is regarded to bind well to the receptor.
Results
Active Ingredients
In total, 112 components of STF were obtained from the TCMNPAS Database. After screening and removal of duplicate components according to the set Absorption, Distribution, Metabolism, and Excretion (ADME) parameters, 21 active components were obtained (Table 1).
Drug Targets
According to the prediction of Swiss Target Prediction Database, targets with 0 probability were removed; thus, six active components without target information were deleted. Finally, 709 potential targets of STF were obtained.
Gastric Cancer-Related Targets
A total of 515 differentially expressed targets related to GC were identified. APC, CASP10, IRF1, MUTYH, erbB2, FGFR2, PIK3CA, KLF6, KRAS, and CTNNB1 were identified as the proteins with the highest scores.
Drug-Disease Key Targets
From the intersection of the proteins obtained in sections Drug Targets and Gastric Cancer-Related Targets, 43 proteins involved in the mechanism of STF in treating GC were obtained (Figure 1A). The active component-target-disease network diagram was obtained by using Cytoscape 3.7.0. The blue round node represents the potential target of STF in treating GC, the purple rectangle node represents the active component of STF, and the red diamond node represents the targets of GC (Figure 1B). The protein interaction was predicted by using the String Database and visualized by Cytoscape 3.7.0. The darker the node, the closer the protein interaction. The top five proteins were AKT1, ESR1, HRAS, EGFR, and STAT3 (Figure 1C). According to analysis of network topology parameters, the proteins with more than median Degree of node were selected as key targets. The network contained a total of 20 target proteins (Table 2) and 14 core active components (Table 3).
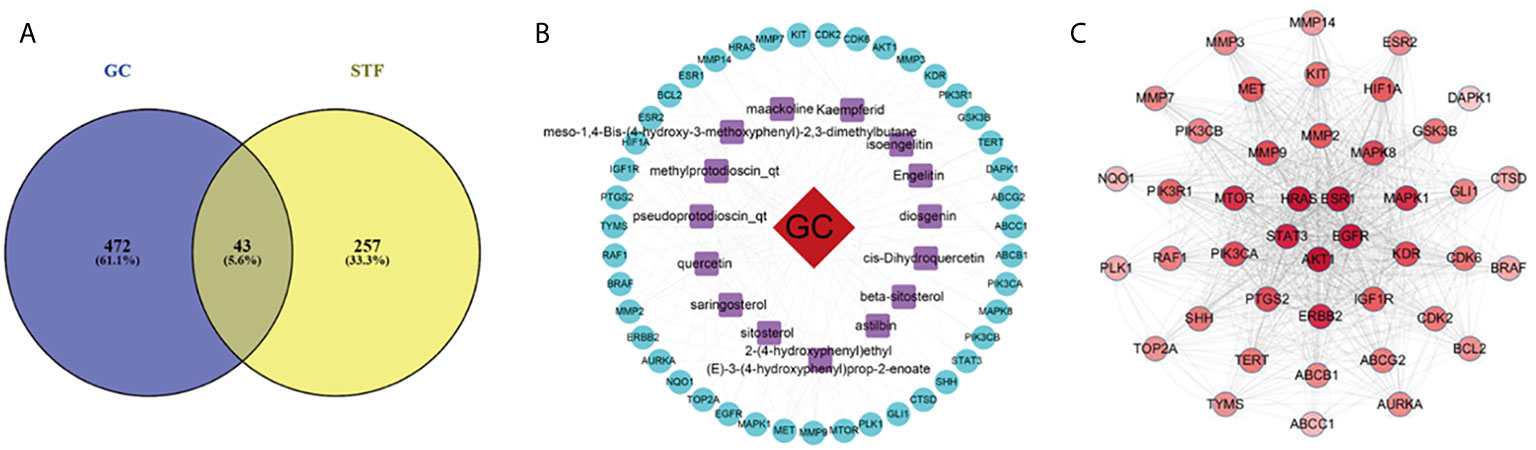
Figure 1 STF-GC gene mapping Venny map (A); Active ingredients-target-disease network (B); PPI network (C).
Enrichment Analysis of Key Targets
A total of 41 selected key targets were analyzed for GO functional and KEGG pathway enrichment analyses and screened at P<0.05. A total of 52 GO biological processes, 9 cell components, 13 molecular functions (Figure 2A), and 86 KEGG pathways (Figure 2B) were enriched. The enriched molecular functions were ATP binding, protein serine/threonine kinase activity, and metalloendopeptidase activity. The biological processes were mainly involved in the negative regulation of apoptosis, positive regulation of RNA polymerase II promoter transcription, and phosphorylation of peptide serine. The cell components were the cell membrane, nucleus, and extracellular matrix. The KEGG pathways were the pathway in cancer, proteoglycan synthesis, PI3K/AKT signaling pathway, focal adhesion, and FOXO signaling pathway.
Molecular Docking Analysis
Two of the screened active components, quercetin and diosgenin, were potentially associated with MDR, as discussed below, and the PI3K/AKT pathway was screened as one of the top 10 KEGG pathways related to MDR. These active components were subjected to molecular docking to the receptor proteins of the PI3K/AKT pathway. The results showed that the binding energies of quercetin and diosgenin with the target receptor protein were less than -5 kJ/mol, indicating that they could bind well to the target receptor (Table 4). The molecular docking diagram is shown in Figure 3. The results confirmed that quercetin and diosgenin could interact with PI3K and AKT. Quercetin and PI3K formed a hydrogen bond through ASP1017. Quercetin and AKT formed three hydrogen bonds through VAL182, TYR306, and ARG308. Diosgenin and PI3K formed a hydrogen bond through ASP1017. Diosgenin and AKT formed a hydrogen bond through GLY775. Quercetin and AKT formed the highest number of hydrogen bonds, and all the binding sites of quercetin and diosgenin with PI3K were on the ASP1017 residue, indicating that quercetin and diosgenin were the two core active components of STF and that the effect of STF in treating GC and reversing MDR might be mediated by the PI3K/AKT signaling pathway.
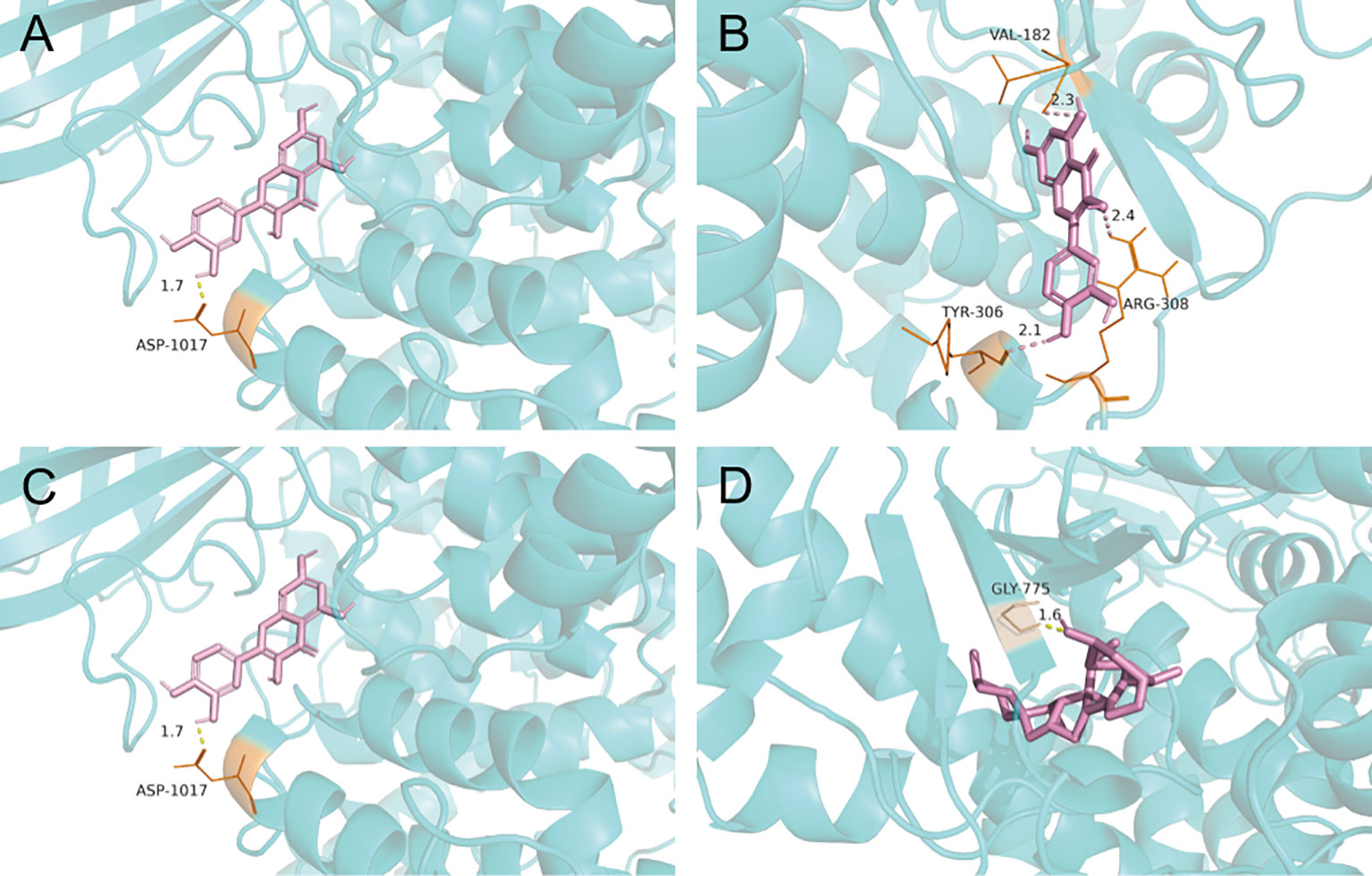
Figure 3 Molecular docking pattern map of quercetin-PI3K (A); Molecular docking pattern map of quercetin-AKT (B); Molecular docking pattern map of diosgenin-PI3K (C); Molecular docking pattern map of diosgenin-AKT (D).
Discussion
The aim of this study was to elucidate the molecular mechanism of STF in treating GC. A total of 14 core active components of STF were screened out, including kaempferol, quercetin, isoengelitin, methylprotodioscin, and diosgenin. The key signaling pathways included the PI3K/AKT signaling pathway, FOXO signal pathway, and ERBB signaling pathway. Basic studies have confirmed that quercetin, diosgenin, and the PI3K/AKT signaling pathway can regulate MDR to chemotherapeutic drugs. Quercetin is one of the active components of Radix Actinidiae Chinensis, and studies had shown that in addition to anti-inflammatory, antioxidant, apoptosis-inducing, and antiangiogenesis effects, quercetin has chemosensitizing effect to enhance the sensitivity of drug-resistant cells to drugs. The effects of quercetin on GC cells have been studied by Sylwia Borska et al. (23) using EPG85-257P cells and daunorubicin-resistant EPG85-257RDB cells. The results showed that quercetin inhibited the growth of the sensitive EPG85-257P cells and had a synergistic effect with daunorubicin. In the drug-resistant EPG85-257RDB cells, quercetin acted as a chemosensitizer, and the drug resistance mechanism of these cells might be related to a decrease in p-glycoprotein (p-gp) expression, obstruction of drug transport, and downregulation of ABCB1 gene expression. The study suggested that quercetin may be effective in reversing the classical drug resistance of GC cells. Zhaolin Chen et al. (24) confirmed that quercetin can increase the accumulation of rhodamine 123 and adriamycin, increase the sensitivity of BEL/5-FU cells to chemotherapeutic drugs, and downregulate the expression of ABCB1, ABCC1, and ABCC2, and that its effect was dependent on FZD7 through the Wnt/β-catenin pathway; quercetin can, at least partially, reverse chemotherapy resistance by inhibiting FZD7. Thus, this compound can be developed into an effective natural sensitizer to reverse the drug resistance of human liver cancer. Diosgenin is one of the active components of Chinaroot Greenbier Rhizome Catbriar, which has pharmacological effects, including anti-inflammatory, anticancer, antiviral, and hypotensive effects. Bu Tong Sun et al. (25) screened candidate MDR inhibitors among more than 300 natural compounds and revealed that diosgenin exerted inhibitory effect on MDR1 promoter activity. Experiments showed that diosgenin decreased the MDR of HepG2/adriamycin cells, significantly inhibited the expression of P-gp, and increased the accumulation of adriamycin in HepG2/adriamycin cells, indicating that diosgenin is an effective MDR reversal agent and a potential adjuvant drug for tumor chemotherapy. Another study suggested that diosgenin can reverse MDR to adriamycin by inhibiting the nuclear factor kappa B (NF-κB) signaling pathway and downregulating MDR1 expression (26). The PI3K/AKT signaling pathway is the main driving force of various cell functions. Excessive activation of this pathway plays a key role in cancer progression; it can promote tumorigenesis by regulating nutrient metabolism, cell proliferation, cell migration, and angiogenesis. Moreover, abnormal activation of PI3K/AKT is key to the regulation of MDR, mainly through the expression of death-related protein, ABC transporter, and glycogen synthase kinase-3β (GSK-3β), as well as synergistic effect with NF-κB and mammalian target rapamycin (mTOR). Some studies have suggested that P-gp and BCRP can be downregulated by PI3K110α and -110β to restore the drug sensitivity of drug-resistant human epidermoid carcinoma and non-small cell lung cancer, and that ABC family proteins and AKT may play an independent role in enhancing MDR (27–29).
In addition, preliminary research on the mechanism of STF in treating GC showed that STF can inhibit the growth of GC MGC-803 cells by regulating the Smac/Survivin signaling pathway in vitro, reduce the adhesion and invasion ability of SGC-7901 cells, inhibit cell migration, and induce apoptosis. Moreover, it can inhibit the growth of SGC-7901 GC cell xenograft tumor in nude mice in vivo; this effect may be related to the promotion of apoptosis, upregulation of the expression of apoptosis-related proteins caspase-8 and caspase-9, promotion of PARP editing, and downregulation of Livin protein expression (30–34). STF was also shown to inhibit the invasion, migration, and adhesion of GC cells and promote apoptosis, as confirmed by previous research. Furthermore, at the molecular level, molecular docking analysis showed that quercetin and diosgenin bound well with the active sites of PI3K and AKT, the key targets of the PI3K/AKT pathway, which verified the accuracy of this study to some extent.
In conclusion, this study preliminarily explored the potential molecular mechanism of STF in reversing the MDR of GC to chemotherapy through network pharmacology and molecular docking technology. The present study revealed quercetin and diosgenin as the core active components of STF as well as the PI3K/AKT signaling pathway as an important pathway involved in the effect STF in reversing the MDR of GC. Taken together, our results suggested that STF has the effect of reversing the MDR of GC and that it can play a synergistic effect with chemotherapy drugs. STF mainly reversed MDR through the action of quercetin and diosgenin on the PI3K/AKT signaling pathway. These findings provide a direction for future studies to further explore the mechanism of STF in treating GC. However, these conclusions were made based on theoretical simulations and thus still need to be verified by experiments. The authors’ team will conduct experiments as a follow-up to the present work.
Data Availability Statement
All data generated or analyzed during this study are included in this article.
Author Contributions
LG conceptualized and designed the study. LG acquired, analyzed and interpreted the data. LG and HS drafted/revised the work for intellectual content and context. LZ gave the final approval and overall responsibility for the published work. All authors contributed to the article and approved the submitted version.
Funding
The research was funded by Shanghai Science and Technology Commission Development Foundation, award number is 16ZR1437500; Shanghai Health and Family Planning Commission of Medical Science and Technology Innovation Project, award number is ZYKC201701009.
Conflict of Interest
The authors declare that the research was conducted in the absence of any commercial or financial relationships that could be construed as a potential conflict of interest.
The reviewer QX declared a shared affiliation, with no collaboration, with one of the authors HS to the handling editor at the time of the review.
Acknowledgments
The authors would like to thank the participating centers and members. This research did not receive any specific grant from funding agencies in the public, commercial, or not-for-profit sectors.
References
1. Chen H, Zheng R, Wang L, Lv Z, Du L, Wei W, et al. Progress in Cancer Epidemiology Research in China in 2019. Chin J Dis Con (2020) 24:373–9. doi: 10.16462/j.cnki.zhjbkz.2020.04.001
2. Sun K, Zheng R, Zhang S, Zeng H, Zou X, Chen R, et al. Report of Cancer Incidence and Mortality in Different Areas of China, 2015. Chin Onc (2019) 28:1–11. doi: cnki:sun:zhlu.0.2019-01-001
3. Huang WJ, Ruan S, Wen F, Lu X, Gu S, Chen X, et al. Multidrug Resistance of Gastric Cancer: The Mechanisms and Chinese Medicine Reversal Agents. Cancer Manag Res (2020) 12:12385–94. doi: 10.2147/CMAR.S274599
4. Liu H. Research Progress on Chinese Materia Medica for Reversing Tumor Multidrug Resistance. Chin Herb Med (2015) 46:1096–102. doi: cnki:sun:zcyo.0.2015-07-028
5. Chen W, Bin C, Zhu Y, Zhao A. Research Progress of Professor Qiu’s Weichangan in Gastric Cancer. World Trad Chin Med (2017) 12:2864–8. doi: cnki:sun:sjza.0.2017-11-074
6. Ma J. Influence of Chemotherapy Combined With Traditional Chinese Medicine Siteng Recipe on the Curative Effect and Survival Quality in Patients With Gastric Cancer After Operation. Chin Med Mod Dis Edu CN (2020) 18:129–31. doi: cnki:sun:zzyy.0.2020-15-055
7. Chen Q. Analysis of the Effect of Chemotherapy Combined With Traditional Chinese Medicine Siteng Fang on the Quality of Life of Postoperative Gastric Cancer Patients. Cardiovasc D Elec J Int Trad Chin West Med (2020) 8(02):177–8. doi: 10.16282/j.cnki.cn11-9336/r.2020.02.142
8. Mao ZJ, Shen KP, Zhu LM, Yao Q, Zheng J. Effect of Chemotherapy Combined With Traditional Chinese Medicine Si Teng Fang on the Quality of Life of Patients After Gastric Cancer Surgery. J Colorectal Anal Surg (2016) 22(S2):156–7. doi: cnki:sun:dcgm.0.2016-S2-108
9. Zhu L, Shen K, Zhou H, Pan C, Yao Q. Effect of Wei Chang An and Si Teng Fang on Stem Cells CD133~+ of Human Colon Cancer Strains. J Shanghai Jiaotong Univ (Med E) (2016) 36:161–165+171. doi: cnki:sun:shey.0.2016-02-006
10. Yang M, Chen JL, Xu LW, Ji G. Navigating Traditional Chinese Medicine Network Pharmacology and Computational Tools. Evid Based Complement Alternat Med (2013) 2013:731969. doi: 10.1155/2013/731969
11. Hao Y, Li Y, Kang H, Zhang D, Lin T, Tang K, et al. HIT: Linking Herbal Active Ingredients to Targets. Nucleic Acids Res (2011) 39:D1055–9. doi: 10.1093/nar/gkq1165
12. Ru J, Li P, Wang J, Zhou W, Li B, Huang C, et al. TCMSP: A Database of Systems Pharmacology for Drug Discovery From Herbal Medicines. J Cheminform (2014) 6:13. doi: 10.1186/1758-2946-6-13
13. Huang L, Xie D, Yu Y, Liu H, Shi Y, Shi T, et al. TCMID 2.0: A Comprehensive Resource for TCM. Nucleic Acids Res (2018) 46:D1117–20. doi: 10.1093/nar/gkx1028
14. Yang M, Chen J, Shi X, Xu L, Xi Z, You L, et al. Development of in Silico Models for Predicting P-Glycoprotein Inhibitors Based on a Two-Step Approach for Feature Selection and Its Application to Chinese Herbal Medicine Screening. Mol Pharm (2015) 12:3691–713. doi: 10.1021/acs.molpharmaceut.5b00465
15. Gfeller D, Michielin O, Zoete V. Shaping the Interaction Landscape of Bioactive Molecules. Bioinformatics (2013) 29:3073–9. doi: 10.1093/bioinformatics/btt540
16. Rappaport N, Twik M, Plaschkes I, Nudel R, Stein T, Levitt J, et al. MalaCards: An Amalgamated Human Disease Compendium With Diverse Clinical and Genetic Annotation and Structured Search. Nucleic Acids Res (2017) 45:D877–87. doi: 10.1093/nar/gkw1012
17. Shannon P, Markiel A, Ozier O, Baliga N, Wang J, Ramage D, et al. Cytoscape: A Software Environment for Integrated Models of Biomolecular Interaction Networks. Genome Res (2003) 13:2498–504. doi: 10.1101/gr.1239303
18. Trott O, Olson AJ. AutoDock Vina: Improving the Speed and Accuracy of Docking With a New Scoring Function, Efficient Optimization, and Multithreading. J Comput Chem (2010) 31:455–61. doi: 10.1002/jcc.21334
19. Ng MC, Fong S, Siu SW. PSOVina: The Hybrid Particle Swarm Optimization Algorithm for Protein-Ligand Docking. J Bioinform Comput Biol (2015) 13:1541007. doi: 10.1142/S0219720015410073
20. Tai HK, Jusoh SA, Siu S. Chaos-Embedded Particle Swarm Optimization Approach for Protein-Ligand Docking and Virtual Screening. J Cheminform (2018) 10:62. doi: 10.1186/s13321-018-0320-9
21. Burley SK, Berman HM, Bhikadiya C, Bi C, Chen L, Costanzo LD, et al. RCSB Protein Data Bank: Biological Macromolecular Structures Enabling Research and Education in Fundamental Biology, Biomedicine, Biotechnology and Energy. Nucleic Acids Res (2019) 47:D464–74. doi: 10.1093/nar/gky1004
22. O’Boyle NM, Banck M, James CA, Morley C, Vandermeersch T, Hutchison GR, et al. Open Babel: An Open Chemical Toolbox. J Cheminform (2011) 3:33. doi: 10.1186/1758-2946-3-33
23. Borska S, Chmielewska M, Wysocka T, Zalesinska MD, Zabel M, Dziegiel P, et al. In Vitro Effect of Quercetin on Human Gastric Carcinoma: Targeting Cancer Cells Death and MDR. Food Chem Toxicol (2012) 50:3375–83. doi: 10.1016/j.fct.2012.06.035
24. Chen Z, Huang C, Ma T, Jiang L, Tang L, Shi T, et al. Reversal Effect of Quercetin on Multidrug Resistance Via FZD7/β-Catenin Pathway in Hepatocellular Carcinoma Cells. Phytomedicine (2018) 43:37–45. doi: 10.1016/j.phymed.2018.03.040
25. Sun BT, Zheng LH, Bao YL, Yu CL, Wu Y, Meng XY, et al. Reversal Effect of Dioscin on Multidrug Resistance in Human Hepatoma HepG2/adriamycin Cells. Eur J Pharmacol (2011) 654:129–34. doi: 10.1016/j.ejphar.2010.12.018
26. Wang L, Meng Q, Wang C, Liu Q, Peng J, Huo X, et al. Dioscin Restores the Activity of the Anticancer Agent Adriamycin in Multidrug-Resistant Human Leukemia K562/adriamycin Cells by Down-Regulating MDR1 Via a Mechanism Involving NF-κB Signaling Inhibition. J Nat Prod (2013) 76:909–14. doi: 10.1021/np400071c
27. Faes S, Dormond O. PI3K and AKT: Unfaithful Partners in Cancer. Int J Mol Sci (2015) 16:21138–52. doi: 10.3390/ijms160921138
28. Liu R, Chen Y, Liu G, Li C, Song Y, Cao Z, et al. PI3K/AKT Pathway as a Key Link Modulates the Multidrug Resistance of Cancers. Cell Death Dis (2020) 11:797. doi: 10.1038/s41419-020-02998-6
29. Zhang L, Li Y, Wang Q, Chen Z, Li X, Wu Z, et al. The PI3K Subunits, P110α and P110β are Potential Targets for Overcoming P-gp and BCRP-mediated MDR in Cancer. Mol Cancer (2020) 19:10. doi: 10.1186/s12943-019-1112-1
30. Shen K, Liu W, Hu B, Pan C. Impacts of Si Teng Formula on Anoikis Resistance of Human Gastric Cancer SGC-7901 Cell. Shijie Huaren Xiaohua Zazhi (2010) 5:1025–8. doi: cnki:sun:xxhb.0.2010-34-004
31. Shen K, Liu W, Hu B, Pan C. Treatment With Sitengfang Decreases Cell Adhesion, Invasion and Migration in Human Gastric Carcinoma Cell Line SGC-7901. World J Int Med (2010) 18:3616–20. doi: 10.11569/wcjd.v18.i34.3616
32. He P, Shen K, Hu B, Liang Y. Effects of Siteng Fang on Tumor Growth and Apoptosis in Subcutaneously Transplanted Human Gastric Carcinoma in Nude Mice. CN J Trad Chin Med Phar (2013) 28:3632–5. doi: cnki:sun:bxyy.0.2013-12-050
33. He P, Shen K, Hu B, Lu Y. Effects of Siteng Formula on Expression of Caspase-3, Cleaved PARP and Livin in SGC-7901 Gastric Carcinoma. Chin A TCM (2014) 32:247–9. doi: 10.13193/j.issn.1673-7717.2014.02.006
Keywords: Siteng Fang, gastric cancer, network pharmacology, molecular docking, multidrug resistance
Citation: Guo L, Shi H and Zhu L (2021) Siteng Fang Reverses Multidrug Resistance in Gastric Cancer: A Network Pharmacology and Molecular Docking Study. Front. Oncol. 11:671382. doi: 10.3389/fonc.2021.671382
Received: 23 February 2021; Accepted: 06 April 2021;
Published: 07 May 2021.
Edited by:
Dong-Hua Yang, St. John’s University, United StatesCopyright © 2021 Guo, Shi and Zhu. This is an open-access article distributed under the terms of the Creative Commons Attribution License (CC BY). The use, distribution or reproduction in other forums is permitted, provided the original author(s) and the copyright owner(s) are credited and that the original publication in this journal is cited, in accordance with accepted academic practice. No use, distribution or reproduction is permitted which does not comply with these terms.
*Correspondence: Limin Zhu, zhulimin2000@126.com
†These authors have contributed equally to this work and share first authorship