Evolution of E. coli Phytase Toward Improved Hydrolysis of Inositol Tetraphosphate
- 1Lehrstuhl für Biotechnologie, RWTH Aachen University, Aachen, Germany
- 2DWI-Leibniz Institut für Interaktive Materialien, Aachen, Germany
Protein engineering campaigns are driven by the demand for superior enzyme performance under non-natural process conditions, such as elevated temperature or non-neutral pH, to achieve utmost efficiency and conserve limited resources. Phytases are industrial relevant feed enzymes that contribute to the overall phosphorus (P) management by catalyzing the stepwise phosphate hydrolysis from phytate, which is the main phosphorus storage in plants. Phosphorus is referred to as a critical disappearing nutrient, emphasizing the urgent need to implement strategies for a sustainable circular use and recovery of P from renewable resources. Engineered phytases already contribute today to an efficient phosphorus mobilization in the feeding industry and might pave the way to a circular P-bioeconomy. To date, a bottleneck in its application is the drastically reduced hydrolysis on lower phosphorylated reaction intermediates (lower inositol phosphates, ≤InsP4) and their subsequent accumulation. Here, we report the first KnowVolution campaign of the E. coli phytase toward improved hydrolysis on InsP4 and InsP3. As a prerequisite prior to evolution, a suitable screening setup was established and three isomers Ins(2,4,5)P3, Ins(2,3,4,5)P4 and Ins(1,2,5,6)P4 were generated through enzymatic hydrolysis of InsP6 and subsequent purification by HPLC. Screening of epPCR libraries identified clones with improved hydrolysis on Ins(1,2,5,6)P4 carrying substitutions involved in substrate binding and orientation. Saturation of seven positions and screening of, in total, 10,000 clones generated a dataset of 46 variants on their activity on all three isomers. This dataset was used for training, testing, and inferring models for machine learning guided recombination. The PyPEF method used allowed the prediction of recombinants from the identified substitutions, which were analyzed by reverse engineering to gain molecular understanding. Six variants with improved InsP4 hydrolysis of >2.5 were identified, of which variant T23L/K24S had a 3.7-fold improved relative activity on Ins(2,3,4,5)P4 and concomitantly shows a 2.7-fold improved hydrolysis of Ins(2,4,5)P3. Reported substitutions are the first published Ec phy variants with improved hydrolysis on InsP4 and InsP3.
Introduction
Protein engineering enables to improve enzymatic performance and tailor enzymes to desired cost-effective application conditions. Directed enzyme evolution campaigns comprise iterative rounds of diversity generation and screening, which are performed until significant improvements are achieved, or the desired property is reached (Lutz, 2010). Diversity generation strategies are divided into rational, semi-rational, and random mutagenesis (Ruff et al., 2013; Tee and Wong, 2013). Rational engineering approaches are used when a localized property with an understood structure–function relationship (such as activity or selectivity) is targeted, and a crystal structure or a homology model is available (Dennig et al., 2011; Dennig et al., 2012; Feng et al., 2012; Nobili et al., 2013; Nikolaev et al., 2018). Directed evolution uses random mutagenesis and requires no prior structural knowledge, but is associated with higher screening efforts due to the enormously enlarged sequence space (Ruff et al., 2013; Tee and Wong, 2013). State of the art for the generation of high-quality mutant libraries by random mutagenesis are error-prone PCR (epPCR)-based methods. Mutant libraries designed by a semi-rational approach are generated by saturation of selected position for all 20 codons or, in case of focused mutagenesis, by substitution to one identified amino acid. Amino acid position can rationally be selected through computational analysis or result from screening of random mutagenesis libraries.
Among protein engineering strategies, the KnowVolution (knowledge gaining directed evolution) strategy (Cheng et al., 2015) is one of the most generally applicable since it is not restricted to specific properties nor to an extensive dataset. A KnowVolution campaign comprises four phases (1. Identification, 2. Determination, 3. Computational analysis, 4. Recombination), in which computational design and experimental identification are combined to minimize experimental efforts and to generate a comprehensive molecular understanding of each beneficial substitution. KnowVolution campaigns improving various properties such as activity (Islam et al., 2018), binding (Rübsam et al., 2018), pH resistance (Novoa et al., 2019), substrate specificity (Brands et al., 2021), thermal resistance (Contreras et al., 2020), processivity (Mandawe et al., 2018), regioselectivity (Ensari et al., 2020; Ji et al., 2020), and ionic liquid resistance (Wallraf et al., 2018) were reported. In essence, based on the variants already identified by the first round(s) of evolution, including screening and sequencing of variants as well as prior knowledge on the target protein, the corresponding property is deduced using structural computing methods to predict improved variants by (semi-) rational combination of the identified positions. In addition to these established approaches, machine learning algorithms have been increasingly used in recent years to augment such routines with data-driven inference of sequence- and/or structure-based patterns in collected variant fitness datasets to predict improved variants for (re-) combination (Li et al., 2019; Mazurenko et al., 2019; Yang et al., 2019; Wittmann et al., 2021).
Increasing demands for best performing enzymes under non-natural process conditions like high temperature, solvent addition, or non-neutral pH is not limited to biocatalysts in chemical synthesis. In agribusiness, phytases, which are industrial relevant feed enzymes, were deeply engineered for increased thermostability to withstand the feed pelleting process (Kim and Lei, 2008; Yao et al., 2013; Wu et al., 2014; Shivange et al., 2016; Tan et al., 2016; Herrmann et al., 2021). Furthermore, many naturally occurring phytases were engineered for broaden pH stability or improved specific activity to efficiently hydrolyze phytate under conditions present in the gastrointestinal tract of monogastric animals (Lei et al., 2013; Shivange and Schwaneberg, 2017; Herrmann et al., 2019).
Phytases catalyze the stepwise hydrolysis of phytate (InsP6). A drastically reduced hydrolytic activity is reported as the reaction proceeds, leading to the accumulation of substantial amounts of lower phosphorylated InsP species (≤InsP4) (Konietzny and Greiner, 2002). For E. coli phytase (Ec phy), a pronounced accumulation of InsP4 and InsP3 has been reported in literature (Greiner et al., 1993; Wyss et al., 1999). The bottleneck in most phytase applications is the accumulation of InsP4, even though almost all phytase applications aim for complete degradation of phytate to inositol and inorganic phosphate (PO43−). Complete hydrolysis is desired because 1) reaction intermediates still possess chelating effects on minerals and, thus, reduce their bioavailability in food and feed (Brune et al., 1992; Xu et al., 1992; Yu et al., 2012). 2) Incomplete hydrolysis leaves phosphate groups on the inositol backbone, resulting in incomplete phosphate availability in the application and incomplete resource utilization (feed and P recovery from biomass). 3) Partial phytate degradation in feed easily leads to P overfertilization and eutrophication in regions with intensive livestock breeding, as undigested InsPs are excreted in manure and then reach the farmland (Poulsen et al., 1999).
A reduced enzymatic conversion of phytases after cleavage of two to three phosphate groups from InsP6 may be due to phosphate inhibition (Kim et al., 1999; Wyss et al., 1999), a reduced activity on lower InsPs, or a combination of both (Konietzny and Greiner, 2002). Kinetic parameters on lower InsPs are not well investigated “as most of the reaction intermediates are not available in pure form and sufficient quantities” (Konietzny and Greiner, 2002). Compensating such reduced enzymatic activity requires higher dosing, longer reaction times, or toleration of incomplete hydrolysis, which engender increased costs and cause incomplete resource utilization.
A holistic view on the phosphorus management fosters closing the cycle of this disappearing nutrient. Thereby, engineered phytases contribute at different levels to a sustainable bioeconomy. Phytases as feed additives improve the P-utilization and thereby the nutrition efficiency in rod. Moreover, biotechnological phosphorus recovered from renewable resources like deoiled seeds (Herrmann et al., 2020) leads to P-depleted feed (Infanzón et al., 2022) as well as an increased bioavailability in animal feed and thereby reduced P-accumulation in the fields through the phytate poor manure. Biotransformation of phosphorus recovered from remnants of food production to valuable compounds like polyphosphates are emerging applications for a sustainable P-production, which is independent from rock mining as source (Herrmann et al., 2019).
In the past, directed evolution campaigns targeting phytases focused on the feed application and mainly aimed for improved thermostability, broadened pH optima, improved protease resistance, or enhanced specific activity on InsP6. Here, the first evolution campaign toward improved hydrolysis on InsP4 and InsP3 of the E. coli phytase enzyme is reported. Importance of complete phytate depletion and fast hydrolysis of the intermediates is to achieve increased phosphorus mobilization rates in applications. E. coli WT phytase (Ec phy) was subjected to one round of KnowVolution. Prior to evolution, cloning, cultivation, and screening conditions were established for gene expression in an episomal vector system in P. pastoris. Furthermore, InsP standards were prepared considering the relevant isomers of the enzyme, which were required as substrate for screening. Identified variants with improved InsP4 hydrolysis were characterized and recombined by rational selection and machine learning-based predictions. Finally, the molecular understanding of interacting amino acids was explored.
Materials and methods
Chemicals
All chemicals were of analytical grade or of higher quality, and purchased from AppliChem (Darmstadt, Germany), Carl Roth (Karlsruhe, Germany), Fluka (Neu-Ulm, Germany), Merck (Darmstadt, Germany), or Sigma-Aldrich Chemie (Taufkirchen, Germany). Taq and PfuS DNA polymerase were prepared in-house. Enzymes were purchased from New England Biolabs (Frankfurt, Germany) if not stated otherwise. Salt-free oligonucleotides were purchased from Eurofins MWG Operon (Ebersberg, Germany).
Plasmids and strains
The yeast expression strains Pichia pastoris (Komagataella phaffii) BSYBG11 and BSY11DKU70 as well as the plasmids pBSYAG1Zeo, pBSYA1S1Z, and BSY3S1Z were purchased from bisy e.U. (Hofstätten/Raab, Austria).
Kits
Plasmid purification (NucleoSpin® Plasmid), as well as PCR purification and gel extraction (NucleoSpin® Gel and PCR Clean-up) were performed using Macherey–Nagel kits (Düren, Germany). Protein quantification was, if not otherwise stated, carried out using the Pierce™ BCA™ Protein-Assay kit (Thermo Fisher Scientific, Darmstadt, Germany). The Bio-Rad Experion™ Automated Electrophoresis Station (Hercules, USA) was applied using the Experion™ Pro260 analysis kit to measure the protein concentration in supernatant of yeast.
Cultivation media
BMD medium: 1.34% w/v yeast nitrogen base without amino acids (Formedium, Norfolk, England), 1% w/v glucose, and 0.00004% w/v D-biotin in 200 mM potassium phosphate buffer at pH 6. Stock solutions were separately prepared in deionized H2O (dH2O). Phosphate buffer (×5) and glucose stock (×20) were sterilized by autoclaving (121°C, 20 min, 1.05 kg/cm2), while yeast nitrogen base (×10) and biotin stock (×500) were sterile filtered.
BMDM medium: BMD medium supplemented with 1% v/v or 5% v/v of methanol for BMDM-1 or BMDM-5, respectively. The medium was stored at 4°C.
LB medium: 10 g/L of NaCl, 10 g/L of tryptone, and 5 g/L of yeast extract were dissolved in dH2O and sterilized by autoclaving (121°C, 20 min, 1.05 kg/cm2). Agar plates were prepared by addition of 15 g/L of agar.
Low-salt LB medium: 5 g/L of NaCl, 10 g/L of tryptone, and 5 g/L of yeast extract were dissolved in dH2O and sterilized by autoclaving (121°C, 20 min, 1.05 kg/cm2). Agar plates were prepared with addition of 15 g/L of agar. Zeocin as selection marker was added to the plates to a final concentration of 25 μg/ml (1:4,000 dilution) after the media cooled down to ∼50°C. Agar plates were stored in the dark at 4°C and used up within 4 weeks if Zeocin was added.
YPD: 20 g of peptone from meat (Carl Roth, Karlsruhe, Germany) and 10 g of Bacto yeast extract from BD Biosciences (Miami, USA) were dissolved in 950 ml of dH2O and sterilized by autoclaving (121°C, 20 min, 1.05 kg/cm2). Before use, 50 ml of autoclaved glucose [40% (w/v)] is added. Agar plates contained 20 g/L of agar (bacteriology grade from AppliChem, Darmstadt, Germany). Zeocin was added to the plates in a final concentration of 25–100 μg/ml after the media cooled down to ∼50°C. Plates were stored in the dark at 4°C and used up within 4 weeks if Zeocin was added.
Zeocin: Zeocin™ was purchased from InvivoGen (Toulouse, France) with a concentration of 100 mg/ml in solution. All liquid cultures containing a BSY plasmid were supplemented with Zeocin as selection marker. For episomal plasmids, a Zeocin concentration of 50 μg/ml was used, while cultures of chromosomally integrated plasmids were supplemented with 150 μg/ml of Zeocin.
Knowledge gaining directed evolution of Escherichia coli Appa wildtype
Generation of error-prone polymerase chain reaction libraries
The random mutagenesis library was constructed by standard epPCR methods (Cadwell and Joyce, 1992). In all PCRs, a thermal cycler (Mastercycler pro S; Eppendorf, Hamburg, Germany) and thin-wall PCR tubes (0.2 ml; Carl Roth GmbH, Karlsruhe, Germany) were used. DNA concentrations were quantified using a NanoDrop photometer (ND-1000, NanoDrop Technologies, Wilmington, DE, USA). For generation of the Ec phy library (named 0.2, 0.3, and 0.5 mM MnCl2), the plasmid pBSYA1S1Z, harboring the Ec phy WT sequence (NCBI accession no. WP_001300464.1), was subjected to an epPCR reaction with balanced dNTP concentrations. The complete gene of Ec phy was used for mutagenesis, while the α-signal peptide for secretion out of the cell was not exposed to epPCR. PCR contained: Taq DNA polymerase buffer (×1), Taq DNA polymerase (2 U), template DNA (15 ng), dNTP mix (0.2 mM), primers [0.5 µM of each, KH15 (FW primer), KH16 (RV primer), Supplementary Table S1], and MnCl2 (0.1–0.5 mM) in a total volume of 50 µl. PCR protocol is as follows: 94°C for 1 min, 1 cycle; 94°C for 30 s, 70°C for 30 s, 72°C for 40 s/kb, 30 cycles; 72°C for 10 min, 1 cycle. The obtained PCR products were digested (20 U DpnI, 16 h, 37°C) and purified using a PCR purification kit (NucleoSpin Gel or PCR Clean-up kit, Macharey–Nagel, Düren, Germany). Cloning of the epPCR products into the vector pBSYA1S1Z was achieved by homologous recombination. The strain BSY11DKU70 was used to prevent nonhomologous end joining (NHEJ) after transformation and thereby reducing empty vector background during screening. Plasmid amplification was done by PCR. PCR (50 µl) contained: 15 ng of plasmid template DNA, Q5 DNA polymerase (0.04 U/µl), 1× Q5 Reaction Buffer, 0.2 mM dNTP mix and primers KH17 and KH18 (0.5 μM each, Supplementary Table S1. PCR protocol is as follows: 98°C for 3 min, 1 cycle; 98°C for 30 s, 68°C for 30 s, 72°C for 35 s/kb, 30 cycles; 72°C for 10 min, 1 cycle. The template DNA was digested (20 U DpnI, 16 h, 37°C). After subsequent PCR clean-up, vector backbone and insert library were transformed into P. pastoris BSY11DKU70 by electroporation. This was done by adding 150 ng of vector and 190 ng of insert (molar ratio of 1–3) to competent cells. Successful cloning was confirmed by sequencing. Additionally, three epPCR libraries (named KC15, KC20, KC25) were provided by the SeSaM-Biotech GmbH, who used their own advanced epPCR protocol.
Site-saturation mutagenesis and site-directed mutagenesis
Plasmids pBSYA1S1Z or BSY3S1Z harboring the DNA sequences of Ec phy WT were used as templates for site-saturation mutagenesis (SSM) and site-directed mutagenesis (SDM). The PCR reaction was set up as whole plasmid PCR with partially overlapping primers. A modified QuikChange Mutagenesis (QCM) protocol (Hogrefe et al., 2002) was used. To introduce mutations at different sites simultaneously, this procedure was sequentially repeated. Oligonucleotide sequences can be found in Supplementary Table S1. PCR (50 µl) contained 15 ng of plasmid template DNA, Q5 DNA polymerase (0.04 U/µl), 1× Q5 reaction buffer, 0.2 mM dNTP mix, and primers KH23–KH78 (0.5 μM each, Supplementary Table S1). PCR protocol is as follows: 98°C for 60 s, 1 cycle; 98°C for 20 s, 60–65°C for 30 s, 72°C for 35 s/kb, 25 cycles; 72°C for 10 min, 1 cycle. Afterward, the template DNA was digested by the addition of 20 U DpnI (16 h, 37°C). The generated PCR products were purified using the PCR clean-up kit. Finally, 5 ng of plasmid was added to electrocompetent P. pastoris cells and transformed into the BSYBG11 strain.
Cultivation of Pichia pastoris in microtiter plate
Single colonies of P. pastoris BSYBG11 or BSY11DKU70 expressing phytase enzymes were transferred into 96-well MTPs (PS; flat bottom, Greiner Bio-One GmbH, Frickenhausen, Germany) containing YPD media (180 µl per well) supplemented with 25–150 μg/ml of Zeocin. Preculture was incubated for 72 h (30°C, 900 rpm, 90% humidity) in an MTP shaker (Minitron by Infors HT, Bottmingen, Switzerland). Main culture (180 µl YPD medium with Zeocin) was inoculated in MTP with 5 µl of preculture and cultivated for 72 h (25°C, 900 rpm, 90% humidity). The grown culture was centrifuged (4°C, 3,220 × g, 20 min) and the supernatant stored at 4°C.
Screening assay in microtiter plate
Phytase mutant libraries (epPCR and SSM, SDM) were expressed as described in section “Cultivation of P. pastoris in MTP” and the supernatant diluted accordingly to ensure a free phosphate concentration in the linear range of the AMol assay after enzymatic hydrolysis (usually dilutions of 1:40 to 1:200 in 50 mM NaOAc pH 5 were used). Enzyme dilution (50 µl) was mixed with 50 µl of ∼0.2 mM substrate solution [Ins(2,4,5)P3, Ins(2,3,4,5)P4, or Ins(1,2,5,6)P4] in an MTP and incubated (6 min, 37°C, 900 rpm, Minitron by Infors HT, Bottmingen, Switzerland). The reaction was stopped by the addition of 50 µl of trichloroacetic acid (TCA, 15% w/v), and the samples were subjected to phosphate quantification (AMol assay).
Phosphate quantification with spectrophotometric assay (ammonium molybdate assay)
Phosphate quantification was performed in MTP using the molybdenum blue reaction as described before (Shivange et al., 2012). TCA-treated samples (150 µl) were mixed with 50 µl of ammonium molybdate color developing solution (4 mM ammonium molybdate and 120 mM ascorbic acid in 3.5% v/v H2SO4) and incubated for 15 min (Eppendorf Thermomixer comfort, Hamburg, Germany; 50°C, 900 rpm). After cooling down for 4 min at room temperature, the absorbance was measured at 820 nm (Tecan Infinite M200 Pro, Männedorf, Switzerland). For quantification of free inorganic phosphate in solution, a standard curve of KH2PO4 (from 0 to 250 µM) was used.
Subcloning into expression vector
High-level protein production was achieved by chromosomal integration of the phytase genes under the control of the methanol-inducible CAT promoter. Phytase gene was subcloned from pBSYA1S1Z into the expression vector BSY3S1Z via the restriction sites NotI and XhoI. The restriction mix (1 µg template DNA, 5 µl × 0 CutSmart® buffer, 30 U of each restriction enzyme, and dH2O to 50 µl) was incubated (3 h at 37°C), and the enzymes were heat inactivated (65°C, 20 min). DNA fragments were separated by agarose gel electrophoresis and purified by gel extraction. The vector dephosphorylation and subsequent ligation reaction was performed according to the Rapid DNA Dephos and Ligation Kit (Roche Diagnostics, Mannheim, Germany) with a vector to insert ratio of 1–3. Ligation mixture was transformed into chemically competent E. coli DH5α cells, and colonies were analyzed for correctly assembled plasmids using colony PCR. Verified BSY3S1Z plasmids were isolated with NucleoSpin® Plasmid kit and digested with BamHI for linearization prior to yeast transformation. The linearization reaction (2 µg of plasmid DNA, 5 µl of 10× CutSmart® buffer, 40 U of BamHI and dH2O to 50 µl) was incubated for 3.5 h at 37°C before PCR clean-up was done. Finally, 500 ng of linearized vector was transformed into the BSYBG11 strain. Successful cloning was confirmed by sequencing.
Production of phytase variants in shake flasks
Phytase expression using the plasmid pBSYA1S1Z was performed in YPD media supplemented with 50 μg/ml of Zeocin. The main culture was inoculated to a starting OD600 of 0.4 using preculture (10 ml of YPD for 48 h, 30°C, 250 rpm), and protein production was performed for 72 h (25°C, 250 rpm). The culture supernatant was collected by centrifugation (4°C, 3,220 × g, 60 min) and stored at 4°C.
Flask expressions of phytase genes under control of the CAT promoter (BSY3S1Z plasmids) were supplemented with 150 μg/ml of Zeocin. Preculture was performed in 15 ml of YPD medium for 48 h (30°C, 250 rpm). For cultivation of the main culture, an adapted protocol was used (Hartner et al., 2008): The main culture of 185 ml of BMD was inoculated with 6.1 ml of pre-culture in a 2-L Erlenmeyer flask and grown for 48 h (30°C, 250 rpm). Induction was performed by the addition of 153 ml of BMDM-1 and followed by further incubation for 72 h. Every 24 h, additional methanol was supplemented by adding 30 ml of BMDM-5. The culture supernatant was collected by centrifugation (4°C, 3,220 × g, 60 min) and stored at 4°C.
Purification of phytase
Protein production was performed in a shake flask with chromosomal integrated plasmid (BSY3S1Z) of the respective phytase under control of the CAT promoter in P. pastoris. Supernatants of P. pastoris cells secreting the target phytase (200 ml) were buffer exchanged with equilibration buffer (50 mM NaOAc, pH 5) and concentrated to a final volume of 5 ml using Amicon® Ultra-15 centrifugal filter units (30-kDa cutoff; Merck KGaA, Darmstadt, Germany). Afterward, ion-exchange chromatography was performed with an ÄKTAprime plus (GE Healthcare, Pittsburgh, PA, USA) equipped with a 5 ml of sample loop and a HiTrap® SP HP column (5 ml, GE Healthcare, Pittsburgh, PA, USA). After equilibration of the column with five column volume (cv) equilibration buffer, the sample was loaded. The column was then washed with approximately five cv equilibration buffer and elution was conducted by a linear salt gradient (50 mM NaOAc pH 5, 0–1 M NaCl). The flow rate was set to 2 ml/min, and the total gradient length was set to 100 ml. The elution was collected in 2-ml fractions, and samples were stored at 4°C.
Size exclusion chromatography was performed with an ÄKTA pure 25 purification system equipped with a 5-ml sample loop and a HiLoad 16/600 Superdex 200 pg (GE Healthcare, Pittsburgh, PA, USA). The column was equilibrated with two cv equilibration buffer (50 mM NaOAc pH 5), and approximately 6 mg of protein (∼2.5 ml) was loaded. The flow rate was set to 1 ml/min. The run was stopped after 1 cv of the equilibration buffer has passed through the column.
Purification of inositol phosphate by HPLC
Inositol phosphate standards were purified by semi-preparative HPLC. For this, 400 ml of 5 mM InsP6 (in 50 mM NaOAc, pH 5) was supplemented with phytases (e.g., Ec phy) to a final concentration of 2.5 U/ml (InsP4) or 1 U/ml (InsP3), and the reaction was conducted at 37°C and 150 rpm for 30 min (InsP4 preparation) or 75 min (InsP3 preparation), respectively. The reactions were pH adjusted with HCl to pH 3 and stored on ice. InsPs were immediately pre-purified with HyperSep™ SAX cartridges (bed weight of 10 g, Thermo Fisher Scientific, Darmstadt, Germany) by gradually applying the hydrolysis reaction to the cartridge using reduced pressure of 800–850 mbar to increase the flow rate. Samples were eluted with 17.5 ml of 2 M HCl and evaporated to complete dryness using an IKA rotary evaporator (VWR International, Darmstadt, Germany; 42°C, 25 mbar, 220 rpm). InsP3 and InsP4 were dissolved in 14 ml of NaOAc (50 mM, pH 5) to reach a pH ∼2 and filtered using 0.22-µm filters. By using an HPLC system equipped with a refractive index detector RID-20A (Shimadzu Deutschland GmbH, Duisburg, Germany), loading capacity and retention time were determined on the semi-preparative column Ultrasep ES 100 RP18 (5 μm, 250 mm × 10 mm) (Dr. Maisch, Ammerbuch-Entringen, Germany). Separation and purification of InsP3 and InsP4 were performed on an HPLC system equipped with autosampler SIL-20AC HT, HPLC pump LC-20AD, column oven CTO-20AC, and fraction collector FRC-10A (Shimadzu Deutschland GmbH, Duisburg, Germany). Collection of the fractions (InsP3, InsP4) was based on the retention time determined before using the refractive index detector. Mobile phase was 50.9% v/v MeOH (gradient grade, Honeywell International, Offenbach, Germany), 47.9% v/v dH2O, 0.2% v/v formic acid, and 1.0% v/v tetrabutylammonium hydroxide (40% in water, Sigma-Aldrich Chemie 86854, Taufkirchen, Germany). The pH was adjusted to 3.7–3.8 by the addition of H2SO4 (18 M), and the mobile phase was degassed by bubbling helium for 30 min. Column temperature was set to 40°C, flow rate to 2 ml/min, and total method time was 28 min.
Collected fractions were stored at RT for 2–4 days to evaporate MeOH of the mobile phase before excess water was removed by using a rotary evaporator as described before. InsPs were resuspended in 150 ml of NaOAc (50 mM, pH 4.4) and purified on a HyperSep™ SAX cartridge (10 g) as described before. By this additional purification, the remaining tetrabutylammonium hydroxide of the mobile phase is removed. Finally, InsPs were resuspended in NaOAc (50 mM, pH 5) and stored at −20°C. In total, three different isomers were purified using this procedure: Ins(2,4,5)P3, Ins(2,3,4,5)P4, and Ins(1,2,5,6)P4. Isomer identification and determination of InsP concentrations were carried out by 1H- and 31P-NMR, performed by Dr. Sabine Willbold (Forschungszentrum Jülich, Central Institute for Engineering, Electronics and Analytics, Analytics, ZEA-3).
HPLC analysis of inositol phosphates
Separation and quantification of InsP3–InsP6 species were conducted as described previously (Herrmann et al., 2020). Method details are summarized in the Supplementary Material.
Determination of thermal stability
Thermal protein unfolding was monitored using nano differential scanning fluorimetry (nanoDSF) using the Prometheus NT.48 instrument (NanoTemper Technologies). Ec phy and phytase variants were purified, and solutions of 0.3 μg/μl were prepared (in 50 mM NaOAc pH5, total volume 100 µl). Approximately 10 μl of each sample was filled into three nanoDSF Grade Standard Capillaries (NanoTemper Technologies), respectively, and loaded into the instrument. Phytase thermal unfolding was monitored in a 1°C/min thermal ramp from 15°C to 95°C. Tm values were determined automatically by the PR.Control software.
Computational methods
Visualization of protein structure and analysis of interaction of substrate and substrate binding pocket was performed using the software packages YASARA (version 20.10.4, YASARA Biosciences GmbH, Vienna, Austria), PyMOL (version 2.5, Schrödinger, LLC, New York, NY, USA), and Chimera (version 1.14, Resource for Biocomputing, Visualization, and Informatics at the University of California, San Francisco, CA, USA) (Pettersen et al., 2004; Krieger and Vriend, 2014). As reference structure, X-ray crystal structure of phytase in complex with bound phytate was used (PDB identifier 1DKQ, 2.05 Å resolution, mercury crystal form with complexed phytate that carries inactivating substitution H17A, in contrast to the studied phytase with NCBI reference sequence WP_001300464.1, which is improbable to have affected phytate binding and is principally not affecting the phytase structure) (Lim et al., 2000).
Data-driven recombination of beneficial substitutions, including model training (parameter optimization), validation, and prediction, was performed using the data-driven protein engineering framework PyPEF (Siedhoff et al., 2021). This framework performs sequence-based model training using diverse machine learning algorithms available from the Scikit-learn Python package (Pedregosa et al., 2011). Variant sequence encoding is hereby performed using the 566 available amino acid descriptor sets from the AAindex database (Kawashima et al., 2008). Encoded sequences are either used directly as independent variables in combination with the corresponding variant fitness labels for training a model through supervised learning, or the fast Fourier transforms of the encoded sequences are used as independent model inputs. Using the PyPEF default settings, i.e., fast Fourier-transformed encodings in combination with leave-one-out cross-validation based parameter tuning of partial least squares regression models, we trained models on different data splits to minimize the impact of potential prediction biases of individual models. Model performances were computed on withheld test data using diverse metrices for quantification of model qualities (coefficient of determination (
Results and discussion
In this study, the E. coli WT phytase (Ec phy) was selected as starting variant for evolution toward improved hydrolysis on InsP4 and InsP3 and subjected to one round of KnowVolution. In this section, first the phytase expression in P. pastoris and the InsP standard preparation is reported as these are required prior to evolution. In the following four sections, the results in the four phases are detailed and summarized in Figure 1: I. Identification, II. Determination of beneficial amino acid substitutions, III. Computational analysis, IV. Recombination.
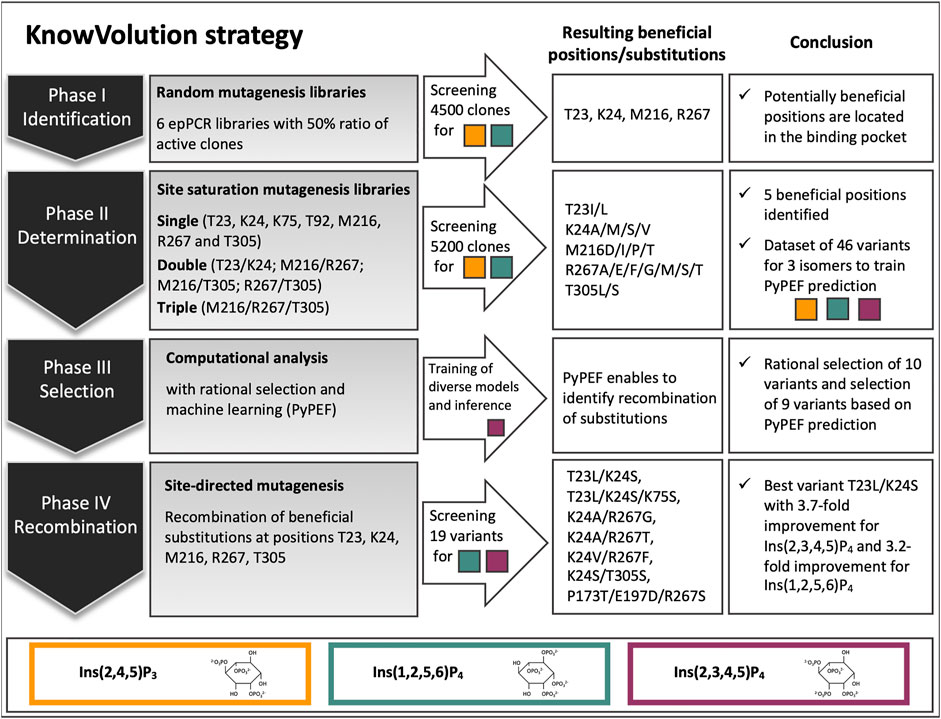
FIGURE 1. Overview of the knowledge gaining directed evolution (KnowVolution) approach of E. coli phytase (Ec phy) toward improved hydrolysis of lower inositol phosphates. In phase I: Screening of more than 4,500 clones from error-prone PCR (epPCR) libraries resulted in variants with up to 2.5-fold improved hydrolysis of Ins(1,2,5,6)P4 and the identification of potentially beneficial positions. In phase II: Determination of beneficial substitutions by SSMs was successful. A dataset of 46 variants for enzymatic activity on all three isomers [Ins(2,4,5)P3, Ins(2,3,4,5)P4, and Ins(1,2,5,6)P4] was generated. In phase III: Computational analysis was performed. Machine learning (PyPEF) enabled to predict recombinants. In phase IV: Recombination of beneficial substitutions lead to activity improved variants by up to 3.7-fold on the main E. coli isomers [Ins(2,3,4,5)P3, Ins(1,2,5,6)P4].
Purification of inositol phosphate isomers
Key requisite for a successful engineering campaign is a reliable high-throughput assay, which mimics the application conditions as well as exhibits a low standard deviation. A screening with direct detection methods for phytase substrates and products, ideally ranging from InsP6 to InsP1 or even Ins, has the advantage to provide detailed insights into the reaction progress. However, analytical methods, such as NMR (Johansson et al., 1990; Ragon et al., 2008), HPLC (Sandberg and Ahderinne, 1986), or HPIC (Phillippy and Bland, 1988; Skoglund et al., 1998), are laborious and have a low to medium throughput and, thus, are not appropriate for screening of thousands of clones in MTP for improved hydrolysis of InsP4 and InsP3. Therefore, the well-established but indirect detection method using the ammonium molybdate (AMol) assay (Murphy and Riley, 1958; Murphy and Riley, 1962; Shivange et al., 2012) was selected. After stopping the phytase reaction, the amount of free inorganic phosphate (Pi) in solution is quantified by the formation of molybdenum blue and a UV-measurement at 820 nm.
Yet, by employing an indirect detection method such as the AMol assay and using InsP6 as a substrate, it is not feasible to conduct an evolution campaign for improved hydrolysis of lower InsP: Higher levels of Pi will not necessarily indicate an enhanced hydrolysis of InsP4 or InsP3, as no information about the progress of the reaction is obtained. However, if InsP4 and InsP3 are used as substrates for the phytase reaction, there is a correlation between higher phosphate concentrations and more efficient hydrolysis of these same InsPs. Thereby, it is important to use the correct isomers for Ec phy because each enzyme has its specific hydrolysis pathway. For 6-phytase Ec phy, the main hydrolysis pathway proceeds via Ins(1,2,3,4,5)P5, Ins(2,3,4,5)P4, Ins(2,4,5)P3, Ins(2,5)P2, and leads to Ins(2)P1 as end product (Konietzny and Greiner, 2002).
In literature, the activity of Ec phy on InsP4 is estimated to be about 1/7 of that for InsP6, leading to the accumulation of the former as an intermediate during hydrolysis (Greiner et al., 1993; Wyss et al., 1999). This is clearly demonstrated in Supplementary Figure S1, in which a final concentration of 1 U/ml of Ec phy was added to 5 mM InsP6 and the reaction analyzed for InsP6–InsP3 by HPLC. After 25 min, no InsP6 or InsP5 was found, and only InsP4 as well as small amounts of InsP3 were detected. Further hydrolysis is very slow, and although the reaction is progressing, significant amounts of InsP4 are still present after >3.5 h (225 min). This highlights the need to evolve Ec phy for improved hydrolysis on InsP4 and the great potential of combining the WT enzyme with an engineered variant in applications requiring complete hydrolysis of InsP6.
In order to generate the isomers produced by Ec phy, different hydrolysis reactions of InsP6 were carried out, and the hydrolysis was stopped at certain time points. For example, to produce InsP4, 1 U/ml of Ec phy was added to 5 mM InsP6, and the reaction was terminated after 30 min, while to reach InsP3, 2.5 U/ml of Ec phy was incubated for 75 min. This ensures that the isomers produced by the WT [Ins(2,3,4,5)P4 and Ins(2,4,5)P3] (Konietzny and Greiner, 2002) were subsequently purified by semi-preparative HPLC. Successful purification was verified by injection on an analytical HPLC column to check for impurities of other InsPs. InsP3 showed a shoulder, which is caused by overloading of the analytical column. HPLC chromatogram of the purifications revealed no detectable impurities from other inositol phosphates (Figure 2). Separation and purification of Ec phy generated InsPs resulted in highly pure substrates for the evolution campaign. In the literature, it is pointed out that phytases usually have one main degradation pathway, which implies that a pathway with other isomers is also possible and, for many enzymes, already proven (Greiner et al., 1993; Greiner and Konietzny, 2010). However, literature reports describe Ins(2,3,4,5)P4 and Ins(2,4,5)P3 as main products after InsP6 hydrolysis using Ec phy, so we conclude that the prepared Ins(2,3,4,5)P4 and Ins(2,4,5)P3 mainly contained these isomers. Beside Ins(2,3,4,5)P4 and Ins(2,4,5)P3, also Ins(1,2,5,6)P4 was purified using the same procedure, but hydrolysis was performed using a commercial phytase derived from Ec phy (Zeller et al., 2015). The Ins(1,2,5,6)P4 configuration was confirmed by NMR analysis (Supplementary Figures S2 and S3). In conclusion, the two main isomers [Ins(1,2,5,6)P4 and Ins(2,3,4,5)P4] described in literature for Ec phy were used for KnowVolution strategy.
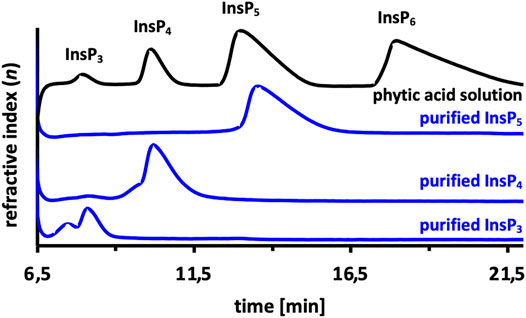
FIGURE 2. Purified inositol phosphate (InsP) standards using semi-preparative HPLC. Phytate (InsP6) was hydrolyzed by Ec phy, and the reaction stopped accordingly to purify the target inositol-phosphates (InsP3, InsP4, InsP5).
Protein production in microtiter plate and screening assay
Second prerequisite for an efficient screening assay is a reliable and homogeneous high-throughput protein production setup in MTP. In the application of P recovery from biomass, P. pastoris was selected as production host for Ec phy (Herrmann et al., 2020) due to the availability of posttranslational modifications, high cell densities, higher specific activity compared with E. coli production (Miksch et al., 2002; Huang et al., 2008; Tai et al., 2013) and its ability to efficiently secrete proteins into the culture broth. As the expression host impacts on protein production in the engineering of Ec phy, P. pastoris ΔKU70 with its episomal expression system and cloning by homologous recombination was selected for the development of the screening system in MTP. This P. pastoris BSY11DKU70 strain (bisy e.U.), in which the KU70 homolog was deleted, has the feature to avoid the less specific and error-prone nonhomologous end joining (NHEJ) (Näätsaari et al., 2012). High transformation efficiencies and the simplicity of application make this type of protein production more convenient and flexible for DNA manipulation applications compared with genomic integration (Chen et al., 2017; Gu et al., 2019). Various parameters influencing the homogenous cultivation of P. pastoris and the resulting Ec phy expression were investigated, such as amounts of vector and insert used for transformation (1–150 ng vector and 1.3–400 ng insert), influence, and duration of preculture (0–7 days), duration of main culture (1–7 days) as well as cultivation conditions (temperature: 25°C and 30°C, humidity: 70% and 90%) and Zeocin concentration in the media (40 and 100 μg/ml). Although the ΔKU70 strain shows no severe growth retardations, its doubling time is slightly higher than that of the common P. pastoris strains (Näätsaari et al., 2012), which have a log phase doubling time of ∼2 h in YPD media (Kastilan et al., 2017). Indeed, it has been shown that prolonged preculture incubation leads to lower standard deviations in cell numbers per milliliter (measured via optical density at 600 nm). A uniform inoculation of the main culture with the same number of cells is important because screening of variants does not include normalization of protein amount and assumes that protein production in each well is comparable. Optimized cultivation conditions (see Materials and methods section) yielded a uniform optical density with a standard deviation (SD) of only ∼9% for 96-well cultivation of WT Ec phy in MTP (Supplementary Figure S4A). Variations in phytase activity over all clones in one plate, however, are significantly higher with 34% (apparent) and 38% (true), although the extensive optimized cultivation conditions were used and a uniform optical density was achieved (Supplementary Figure S4B).
Standard deviations below 30% were not reproducible, even though the cells had grown uniformly. This might be due to a high copy number (multicopy presence of the episomal plasmid) within the yeast cells, which can lead to increased gene expression (Gu et al., 2019). Transformation of lower DNA amounts to reduce the probability of multiple plasmids per cell was not possible, because homologous recombination was used for cloning and higher DNA amounts are required for efficient transformation compared with fully ligated plasmids. Increased standard deviation (>15%–20%) implies an increase of the required effort for rescreening, as false positives and false negatives are more likely to occur. However, since this is consciously considered during evaluation of the results and oversampling in screening was adapted, an engineering campaign using this screening setup was conducted.
In conclusion, successful HPLC-based purification of the InsP substrates [Ins(2,4,5)P3, Ins(2,3,4,5)P4, and Ins(1,2,5,6)P4] and set up of the screening assay, including the episomal production of Ec phy in P. pastoris, enable the first evolution campaign of a phytase toward improved hydrolysis of inositol phosphates <InsP6.
Knowledge gaining directed evolution of Escherichia coli phytase toward improved hydrolysis of lower inositol phosphates
Knowledge gaining directed evolution phase I: Identification of beneficial amino acid positions
Random mutagenesis of the Ec phy gene was performed by error-prone PCR (epPCR). Three MnCl2 concentrations (0.2, 0.3, and 0.5 mM MnCl2) were tested to adjust the mutational load to ∼50% of active clones as this ratio is reported as suitable to generate mutants with 1–2 mutations per kb (Cirino et al., 2003). The ratio of active clones was determined by screening at least three 96-well MTPs with Ins(1,2,5,6)P4 as substrate and calculating the average, while epPCR libraries with 0.2 mM MnCl2 (Supplementary Figure S5A) mostly result in variants with activities comparable with the WT, 0.5 mM MnCl2 (Supplementary Figure S5B) leading to predominantly inactive variants. The ratio of active clones for epPCR libraries generated with 0.2, 0.3, and 0.5 mM MnCl2 were determined to be 62%, 39%, and 14%, respectively (Supplementary Figure S6). Based on this result, the libraries generated using 0.2 and 0.3 mM were selected for further screening. Additionally, three epPCR libraries (named KC15, KC20, and KC25), which have a ratio of active clones of 50%–60% were provided by the SeSaM-Biotech GmbH and included in the screening.
Subsequent screening was performed in MTP using Ins(1,2,5,6)P4 as substrate, and in total >4,500 clones were analyzed by the colorimetric AMol-Assay. Variants that showed an improvement were rescreened and analyzed for their ability to hydrolyze Ins(2,4,5)P3 (Figure 3). Overall, five variants (CB20 F1, CB44 D9, CB19 G12, and CB 30 H10) were identified that are significantly improved in the hydrolysis of Ins(1,2,5,6)P4 by up to 2.5-fold in case of variant CB41 E2 (Figure 3). Significant improvements in hydrolysis of Ins(2,4,5)P3 were not identified during rescreening (Figure 3C). Only variant CB32 F8 with a 1.4-fold relative improvement was identified. This supports the statement, “You get what you screen for” (Schmidt-Dannert and Arnold, 1999), as the pre-selection for rescreening were activities for Ins(1,2,5,6)P4 hydrolysis and not activity on an InsP3 isomer. In fact, this is likely to be one reason why no hit was found for InsP3. Only variant CB51 G8 showed promising activities for both isomers [Ins(2,4,5)P3 and Ins(1,2,5,6)P4] during rescreening (Figures 3A, C). In total, 11 clones were chosen for sequencing (highlighted in gray, Figure 3) and produced in a shake flask. By determining the protein concentration, expression variants were excluded, and relative improvements can be calculated.
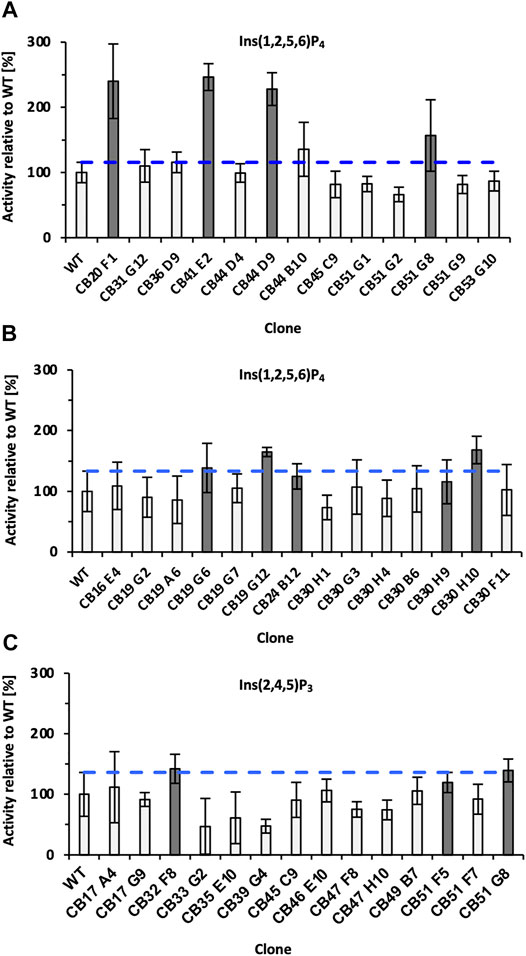
FIGURE 3. Final rescreening results of improved variants obtained from epPCR libraries. Relative improvements were determined for the substrates Ins(1,2,5,6)P4 (A,B) and Ins(2,4,5)P3 (C). Only variant CB51 G8 showed promising activities for both substrates and was consequently selected for rescreening twice (A,C). The blue dotted line represents the threshold for classifying clones as improved. Clones marked dark gray were sent for sequencing and additionally expressed in a 50 ml shake flask to determine protein adjusted improvements. Significantly improved refers to the relative activity of the variant minus SD, surpassing the relative activity of WT plus SD. WT, wild type; SD, standard deviation.
Table 1 summarizes the results of sequencing, variant origin (library), and final relative improvement factor for hydrolysis of Ins(1,2,5,6)P4 with adjusted protein concentration. The five strongest improved variants for Ins(1,2,5,6)P4 hydrolysis all carry at least one substitution in the substrate binding pocket (highlighted in bold, also see Figure 3). These mutants show a relative improvement of at least 1.9- and up to 2.5-fold compared with the WT. This strongly emphasizes the impact of substrate binding and orientation for hydrolytic activity. The fact that substitutions in the binding pocket modulate the activity on substrates proves reasonable, especially when considering the different charges of InsP6 and its intermediates. While InsP6 has a net charge of −12, InsP4 only has a net charge of −8, and the binding pocket must cope with these significant changes. Amino acids involved in capturing phytate in the binding pocket of Ec phy are predominantly positively charged or polar to attract and accurately orient the strongly negative InsP6. Variants with highest improvements for Ins(1,2,5,6)P4 hydrolysis replace positive charges by nonpolar amino acids (e.g., K24M or K24G) or even introduce negatively charged amino acids to the binding pocket (M216D), which accounts for the reduced net negative charge of InsP4 compared with InsP6.
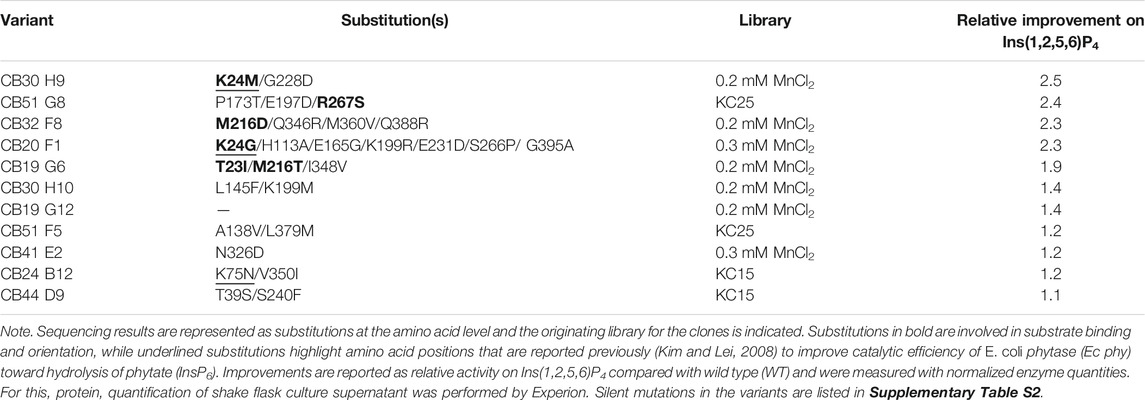
TABLE 1. Summary of the 11 improved variants obtained during screening and rescreening of error-prone PCR (epPCR) libraries.
Notably, the variants CB30H9 and CB51G8 with the highest relative improvements of 2.5- and 2.4-fold (Table 1) showed only moderate improvements of 1.2- and 1.6-fold, respectively, during screening and rescreening (Figure 3). CB20 F1, on the other hand, maintained the improvements from rescreening (2.4-fold) even at normalized protein concentrations (2.3-fold). For variants with increased activity after concentration adjustment, Experion analysis revealed reduced protein concentrations in the culture supernatant of P. pastoris (Supplementary Figure S7), which explains the lower activity compared with WT determined during rescreening.
In summary, screening of more than 4,500 clones from epPCR libraries (Figure 1) resulted in variants with up to 2.5-fold improved hydrolysis of Ins(1,2,5,6)P4. The identification of variants with relative improvements >2-fold demonstrates that an evolution campaign for InsP4 with the screening system established here using HPLC-purified InsP substrates is a successful strategy.
Knowledge gaining directed evolution phase II: Determination of beneficial substitutions
In phase II, site-saturation mutagenesis libraries are generated at the amino acid position identified in phase I and screened to determine beneficial substitutions. Screening of the random mutagenesis libraries in phase I revealed the amino acid positions T23, K24, M216, and R267 within the binding pocket of Ec phy as important for enhancing the hydrolytic activity on Ins(1,2,5,6)P4. The selection of positions to be targeted by site-saturation mutagenesis (SSM) was not only based on the screening results (Table 1) but also took into account the substrate binding position in the active site (Figure 4) and literature reports about improved efficiency of Ec phy (Kim and Lei, 2008). By visual examination of the interactions between InsP6 and the residues of Ec phy in the binding pocket and considering that the phosphates are cleaved in the order 6, 1, 3, 4, and 5 (Konietzny and Greiner, 2002), positions T92 and T305 were further selected. T92 is hydrogen bonded to the phosphates in position 1 and 3, which are cleaved during InsP5 and InsP4 hydrolysis, while T305 forms a hydrogen bond to R267 (Figure 4). Identified variants K24E and K43E/K75M/S187G (Kim and Lei, 2008) (corresponding to their variants K46E and K65E/K97M/S209G due to different numbering) have an improved catalytic activity on InsP6 of 56% and 152 %, respectively. Since K75 was also identified in the variant CB24 B12 in phase I, this position was also included in site-saturation mutagenesis.
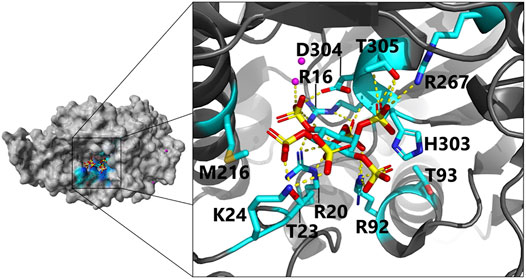
FIGURE 4. Close-up view of the active site in E. coli phytase in a complex with phytate (InsP6) as substrate (PDB code 1DKQ): Negatively charged InsP6 is predominantly bound by positively charged and polar residues via hydrogen bonds to the active site residues. Magenta dots indicate Hg2+ cations used for crystallization of the phytate-bound complex. For clarity, only direct interactions of residues with InsP6 and identified beneficial phytase engineering positions are shown (color scheme: C, cyan; O, red; N, blue; P, yellow; yellow dotted lines, hydrogen bonds). Dependent on the protonation state, H303 can also interact via hydrogen bonding with InsP6 [more details on the proposed binding mode including indirect hydrogen bonding interactions are depicted here (Lim et al., 2000)].
SSM libraries were generated for all single amino acid positions (T23, K24, K75, T92, M216, R267, and T305) and the pair T23/K24. First, the ratio of inactive and improved clones (Supplementary Figure S8) was determined. Analysis of two MTPs (180 clones, which corresponds to a 5.6-fold oversampling for single SSM libraries) revealed a ratio of 12%–22% improved clones in the SSM libraries at positions M216, R267, and T305, and thus, these three positions were selected to generate double and triple SSM libraries in all combinations (M216/R267; M216/T305; R267/T305; M216/R267/T305). The ratio of improved clones was around 5%, but almost tripled to ∼14% for library M216/R267 (Supplementary Figure S8), indicating that simultaneous substitution at these two positions can have beneficial effects on InsP4 activity of Ec phy.
Subsequently, after the initial screening of >5,200 clones was completed, more than 1,260 clones from single (T23, K24, K75, T92, M216, R267, T305), double (T23/K24, M216/R267, M216/T305, R267/T305), and triple (M216/R267/T305) SSM libraries were rescreened on all three substrates [Ins(1,2,5,6)P4, Ins(2,4,5)P3, and Ins(2,3,4,5)P4]. Twenty-eight variants with highest relative improvements were sequenced and selected for in-depth activity determination. For the latter, protein production was conducted in shake flasks, and protein concentrations in the supernatant of P. pastoris were quantified using Experion™ prior to the activity assay. The following variants were included: 1) The four best epPCR variants (CB30 H9, CB51 G8, CB32 F8, and CB20 F1), which were identified in phase I; 2) 28 variants identified during rescreening of single, double, and triple SSM libraries, which were already sequenced in phase II; 3) Five site-directed mutagenesis (SDM) variants (K24S, K24T, K75S, M216D, and R267F), which were identified as being beneficial in preliminary rescreening analysis of SSM libraries in phase II; 4) The nine double mutants resulting from recombination of the previous SDM variants (K24S/K75S, K24S/M216D, K24S/R267F, K24T/K75S, K24T/M216D, K24T/R267F, K75S/M216D, K75S/R267F, and M216D/R267F). In total, this results in 46 variants that were cultivated for activity determination on all three InsPs [Ins(1,2,5,6)P4, Ins(2,4,5)P3 and Ins(2,3,4,5)P4] with normalized protein concentrations.
The complete data set with information on sequencing results and relative hydrolysis efficiency for Ins(1,2,5,6)P4, Ins(2,4,5)P3, and Ins(2,3,4,5)P4 compared with the WT using equal protein amounts is summarized in Supplementary Table S3. The top 10 most improved variants for Ins(1,2,5,6)P4 hydrolysis are also depicted in Figure 5. Substitutions at positions M216 and R267 occur most frequently among the best variants and lead to a 2-3-fold improved hydrolysis of Ins(1,2,5,6)P4, but they do not seem to significantly change the activity on Ins(2,4,5)P3. This is fully consistent with the analysis of the ratio of improved clones for the SSM libraries (Supplementary Figures S8 and S9), since the single and simultaneous saturation of these two positions resulted in the highest ratio of improved clones. The nonpolar amino acid M216 is often replaced by other nonpolar amino acids such as Ile, Leu, or Pro, but there is a strikingly abundant discovery of M216D. Through this substitution, a negatively charged amino acid is incorporated into the active site to counterbalance the reduced net negative charge of InsP4 compared with InsP6. Substituting the positively charged R267 with nonpolar amino acids, such as Gly, Ala, or Met, reduces the positive charge of the substrate binding pocket, which could lead to a rearrangement of the bound substrate and better dissociation of the product. Further strong indication that the decreasing net negative charge of lower InsP species requires an adapted charge distribution of the active site is variant R267E. Replacing the positively charged Arg by negatively charged Glu leads to one of the highest improvements of 3.1-fold for the hydrolysis of Ins(1,2,5,6)P4 among all these mutants.
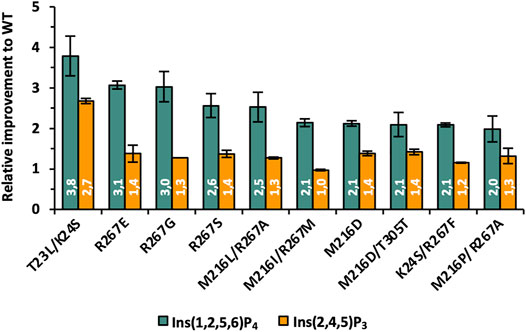
FIGURE 5. Relative improvements of best variants identified during phase II of KnowVolution. All improvements are adjusted to protein concentration.
However, the highest improvement was observed for the double mutant T23L/K24S, although only a low percentage of the single and double SSM libraries were active at these positions (3%–5%, Supplementary Figure S8). This indicates a strong synergistic effect and leads to only a few possible combinations at these positions, but these have a strong influence on the hydrolysis efficiency for lower InsPs. For T23L/K24S not only a 3.8-fold improved hydrolysis of Ins(1,2,5,6)P4 was determined, but this variant also shows a 2.7-fold increased performance for Ins(2,4,5)P3. Thus, this variant is, by far, the only one with an improved Ins(2,4,5)P3 hydrolysis of >2-fold. Analysis of the actual amino acid substitutions reveals once again the replacement of polar and positively charged residues by nonpolar (T23L) and polar (K24S) residues.
In conclusion, the determination of beneficial substitutions by SSMs targeting the positions T23, K24, K75, T92, M216, R267, and T305 of the E. coli phytase was successful. Among the 10 most improved variants for the hydrolysis of InsP4 and InsP3 (see Figure 4), substitutions are almost exclusively found at positions T23, K24, M216, and R267. These four positions were originally identified in the screening of epPCR libraries (Figure 1) that covered the entire gene of Ec phy and are part of the substrate-binding pocket. Relative improvements of up to 3.8-fold for Ins(1,2,5,6)P4 and up to 2.7-fold for Ins(2,4,5)P3 (both for the variant T23L/K24S) compared with WT represent further advances over the best variants obtained in phase I of KnowVolution using epPCR-based methods. Overall, replacing positively charged and polar residues in the binding pocket by nonpolar or even negatively charged amino acids has proven to be efficient in several variants.
Knowledge gaining directed evolution phase III: Computational analysis
The in-depth determination of beneficial substitutions in phase II resulted in a large, detailed dataset for a total of 46 variants (Supplementary Table S3), which is well suited for computer-based analysis and predictions of recombinants. Strictly rule-based recombination of substitutions to further increase enzymatic performance is a challenge and often relies on static rational factors or simple recombination of best variants. However, in particular, epistatic effects of amino acid exchanges are difficult to identify, since no spatial interaction is required for building up epistatic protein networks (Sarkisyan et al., 2016), and recombination of beneficial mutations can cause mutual extinction (Rowe et al., 2003; Bhuiya and Liu, 2010). With the growing understanding of structure–function relationships in enzymes and the increasing computing capacities, in silico methods for the prediction of beneficial substitutions are becoming steadily more significant. These methods can significantly accelerate the semi-rational engineering approach by reducing the workload involved in generating a large number of clones in the wet lab. Instead, some predicted variants are systematically generated and tested. The data set shown in Supplementary Table S4 and the fact that a property with a strong structure–function relationship is to be improved (activity) suggests the use of machine learning methods to navigate the combinatorial space of substitutions more efficiently next to rational selection to further increase the activity of Ec phy toward InsP4.
Identified substitutions, i.e., sequenced variants from KnowVolution phases I and II, were analyzed for recombination by visual inspection, structural analysis, and data-driven recombination methods. Improved variants for Ins(1,2,5,6)P4 hydrolysis harbor amino acid substitutions within the phytase substrate-binding pocket (e.g., T23I, K24M, R267S) and replaced positively charged and polar amino acids with nonpolar or negatively charged residues. Presumably, these substitutions compensate for the decreased negative net charge of InsP4 compared with InsP6 (−8 to −12), thereby, adapting the charge distribution of the binding pocket according to the less charged substrate.
Figure 6 depicts the location of T23, K24, M216, and R267—for which substitutions in phase II resulted in the highest improvements in activity—in the enzyme upon binding of InsP6. The binding pocket as central cavity of the protein is formed by a smaller α-domain (dark gray) and a more conserved α/ß domain (light gray). Beneficial substitutions were identified for both domains of the protein with T23, K24, and M216 being located in the α-domain and R267 in the α/ß domain. All of these four residues form hydrogen bonds (directly or indirectly via water molecules) to InsP6 during binding.
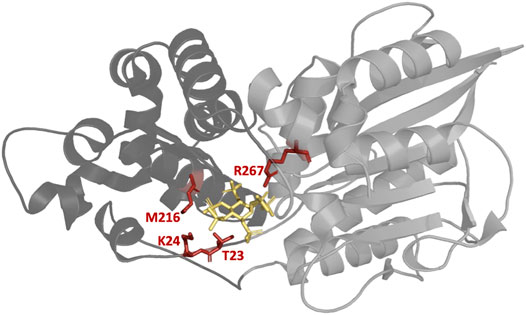
FIGURE 6. Structure of the E. coli phytase with bound InsP6 (yellow). The four highlighted residues T23, K24, M216, and R267 are all located in the binding pocket and have proven to be the most suitable sites for substitution to increase hydrolytic activity against InsP4. Smaller α-domain and the more conserved α/ß domain of Ec phy are represented in dark and light gray, respectively. PDB accession code 1DKQ. Figure created with Chimera (Pettersen et al., 2004).
For machine learning-guided recombination of identified variants in phases I and II, hydrolytic performances on the Ins(2,3,4,5)P4 isomer were considered for model construction (Supplementary Table S4). Since the number of available variants for model construction was small, the goal of predicting recombinant phytase variants from the semi-rationally identified positions was to determine which positions/substitutions were potentially favorable for recombination. Therefore, models were selected based on diverse performance metrics [coefficient of determination (
For model construction and validation, the 566 available amino acid descriptors from the AAindex database (Kawashima et al., 2008) for encoding of the variants sequences were used. Subsequently, the fast Fourier-transform representation of the encoding was used to train a supervised regression model (partial-least squares regression). In general, for this low-
Knowledge gaining directed evolution phase IV: Recombination of identified beneficial substitutions
Following machine learning-based predictions as well as rational selection of in total 19 variants in phase III of the KnowVolution campaign, genetic constructs were generated by SSM and tested for improved hydrolysis on Ins(2,3,4,5)P4 and Ins(1,2,5,6)P4. Of the 19 variants generated, 16 were expressible, with the non-expressible constructs all coming from rational selection of triple and quadruple substitutions (T23L/K24S/M216L, T23L/K24S/R267F, T23I/K24V/M216P/ R267S; Supplementary Table S8). As in phases I and II before, variants were expressed in shake flask, and protein concentrations were adjusted based on Experion™ quantification. Results are shown in Figure 7.
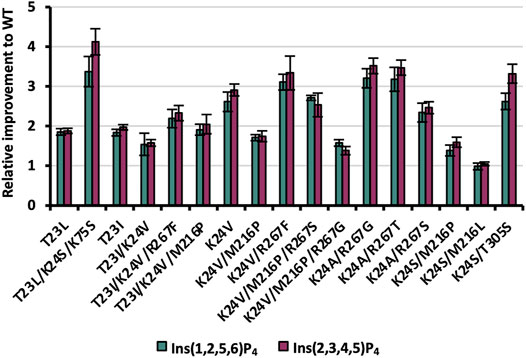
FIGURE 7. Relative improvements of expressible variants selected during KnowVolution phase III (rationally and based on machine learning predictions). Protein concentrations were adjusted for hydrolysis reactions.
Despite the low performance in accurately reproducing the fitness (e.g., low
The two rationally selected single substitutions T23L/I proved also to be beneficial with improvements ranging from 1.8- to 2.0-fold for both InsP4 isomers. These results confirm the beneficial impact of substituting the polar threonine at position 23 to no-polar leucine or isoleucine residues in variants with ≥2 substitutions from previous phase I (T23I in CB19 G6) and phase II (e.g. T23L/K24S). Three out of the five rationally selected, expressible recombinants revealed improved InsP4 hydrolysis of ≥2 (T23I/K24V/M216P, T23I/K24V/R267F, and T23L/K24S/K75S), with up to 4.1-fold improvement on Ins(2,3,4,5)P4 compared with WT for T23L/K24S/K75S. This emphasizes the benefit of complementing computational methods for recombination with rational selection. However, supervised machine learning methods benefit from the availability of data and increase their performance with the number of available variant fitness data. In particular, the training of trustworthy models for low-
Strikingly, most variants show a moderately higher improvement for Ins(2,3,4,5)P4 compared with Ins(1,2,5,6)P4 (Figure 7): This indicates that enzymatic improvement is slightly higher for the main isomer of WT Ec phy, which was the foundation of the evolution campaign.
Overall, the KnowVolution campaign revealed 23 variants with a ≥2-fold improved hydrolysis on Ins(1,2,5,6)P4 compared with the WT, with all variants carrying one, two, or three substitutions (Supplementary Tables S3 and S8). Seven recombinants were finally selected for purification and characterization: T23L/K24S, T23L/K24S/K75S, K24A/R267G, K24A/R267T, K24V/R267F, K24S/T305S, and P173T/E197D/R267S (CB51 G8). Final analysis using purified enzymes was done for the substrates Ins(1,2,5,6)P4, Ins(2,3,4,5)P4 as well as InsP6. InsP6 was considered here for the first time, as improvements in InsP4 hydrolysis may be at the expense of InsP6 activity, and most phytase applications aim for efficient hydrolysis of InsP6 to Ins. Results of the enzyme characterization are depicted in Figure 8.
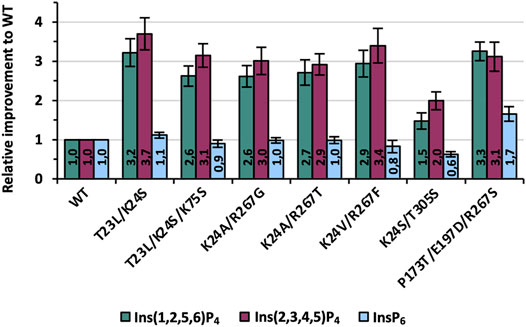
FIGURE 8. Final improvements of the seven best variants identified during one round of KnowVolution. All enzymes are purified, and activity improvements were measured in microtiter plate (MTP) and calculated from at least six independent experiments.
Determined improvements in Figure 8 for purified variants were generally comparable with the data from recombination analysis (Figure 7). Only the activity of variant K24S/T305S is lowered slightly and shows a 1.5- and 2-fold improvement for Ins(1,2,5,6)P4 and Ins(2,3,4,5)P4, respectively. All other variants, however, achieve at least a 3-fold improvement for one of the InsP4 isomers, if not for both. The best variant of this evolution campaign is T23L/K24S with improvements of 3.2- and 3.7-fold compared with the WT for Ins(1,2,5,6)P4 and Ins(2,3,4,5)P4 (Figure 1). Activity on InsP6 is almost identical to WT for most variants: Only K24S/T305S shows about 60% of the activity, whereas P173T/E197D/R267S is even improved 1.7-fold.
Furthermore, thermal unfolding experiments were performed because thermal stability is one of the most crucial and best studied phytase properties due to commercial requirements in animal feed. Thus, thermal stability is one, if not the most frequently engineered property of phytase enzymes (Shivange and Schwaneberg, 2017) and drastically decreased thermal stability of our variants could limit their industrial applicability. Measurements revealed a Tm of variants and WT of 62°C–63°C, with only P173T/E197D/R267S being slightly decreased at approximately 59°C (Supplementary Tables S9 and S10). Thus, even though our variants show improved hydrolysis on < InsP5, their activity on InsP6 as well as their thermal resistance are almost unchanged compared with the WT.
Last, specific activities were analyzed at 37°C and conditions previously reported to be used for P recovery from biomass (Herrmann et al., 2020) (Supplementary Figure S11). Based on these absolute values, it is apparent that the activity of all tested enzymes (Ec phy WT, T23L/K24S, K24V/R267F, P173T/E197D/R267S) is still highest for InsP6, indicating a very strong adaptation of Ec phy to InsP6 as the main substrate. Notably, the activity on the main isomer Ins(2,3,4,5)P4 of Ec phy WT is lower for all enzymes (even the WT) compared with Ins(1,2,5,6)P4. However, the activity levels of the variants have clearly converged: While for WT the activity on Ins(2,3,4,5)P4 corresponds to about 1/5 of the activity of InsP6 [in literature ∼1/7 was previously reported (Greiner et al., 1993)], for T23L/K24S, it is already 2/3. Remarkably, for T23L/K24S, activity on InsP4 is almost as high as the activity of WT Ec phy on InsP6 under the reaction conditions used.
With this study, we demonstrate the yet hidden potential of engineering phytases for activity on lower InsP species (≤InsP5) to unlock the full potential of enzymatic performance. Drastically changing net charges of the reaction intermediates over the course of stepwise phosphate hydrolysis from InsP6 require the use of several specialized phytases for complete and efficient hydrolysis to Ins. Here, we could identify enzyme variants with 2.7-fold improved performance on InsP3 and up to 3.7-fold improved activity on InsP4. It became apparent that consideration of the particular isomers present during the hydrolysis pathway must be accounted for, as the activities vary depending on the isomer. We anticipate the use of three specialized enzymes to cover the full hydrolysis, with each enzyme optimized for two successive InsP species: 1.) InsP6 → InsP4; 2.) InsP4 → InsP2; 3.) InsP2 → Ins. As described in literature (Wyss et al., 1999; Greiner et al., 2001; Ragon et al., 2008; Pontoppidan et al., 2012; Ariza et al., 2013), the hydrolysis of the single axial phosphate group of phytate poses a particular challenge to most enzymes, making a fourth enzyme potentially necessary only for the last hydrolysis step. However, in recent years, phytases, such as from Debaryomyces castellii (Ragon et al., 2008), were shown to be able to hydrolyze even this challenging position, which may open up the possibility of combining optimized variants from different organisms (Infanzón et al., 2022). Future research might focus on enzyme engineering for improved hydrolysis on ≤InsP2 to cover the full spectrum of InsP species during phosphorus mobilization as well as on the combination (blending) of the individually optimized enzymes in an efficient all-in-one process.
Conclusion
Phosphorus mobilization from biomass and its contribution to close the phosphorus cycle in a circular bioeconomy is mastered by phytases. Engineered phytases improved toward inositol tetraphosphate are of high relevance to decrease phytate impact in applications. Despite many protein engineering campaigns targeting properties, such as thermal stability, protease resistance, or activity for InsP6, a major bottleneck for application remains, as efficient and complete hydrolysis of phytate to inositol is required. The herein reported first successful KnowVolution campaign enabled to generate E. coli phytase (Ec phy) variants, which are tailored for improved hydrolysis on InsP4 and InsP3. Screening of more than 10,000 variants from random mutagenesis and 12 saturation libraries led to the identification of 19 beneficial substitutions. The six selected improved variants for hydrolysis on InsP4 all carry substitutions involved in substrate binding and orientation. Impressively, the variant T23L/K24S has a 3.7-fold improved relative activity on Ins(1,2,5,6)P4 and simultaneously shows a 3.2-fold improved hydrolysis of Ins(2,3,4,5)P4 and 2.7-fold for Ins(2,4,5)P3. The main lessons learned are that residues located in the active site are primarily responsible for altered activity of phytases on InsP4 and InsP3, and the variants with improved InsP4 hydrolysis are rarely optimized for InsP3. The charge changes of the substrate from InsP6 to InsP4 and further down have to be reflected in the amino acid composition of the binding pocket. While InsP6 has a negative net charge of −12, the net negative charge of InsP4 is only −8. This significant difference is also reflected in the replacement of positively charged and polar residues in the binding pocket by nonpolar or negatively charged amino acids in variants with the highest improvements. The difference in hydrolysis efficiency of the engineered variants on InsP4 isomers and InsP6 emphasize the importance of tailor-made enzymes and the fact that no universal variant, which hydrolyzes all isomers equally efficient can be predicted. The generated dataset of 46 variants for activity on all three isomers was used to train machine learning models using PyPEF to predict recombinants. This approach demonstrated a high probability of selecting improved, yet expressible variants so that in the future, the required screening capacities can be reduced by implementing machine learning in evolution campaigns. The gained insights here are also valuable information for future engineering of phytases toward improved hydrolysis of lower InsPs. In conclusion, enzyme preparations for application may be a blend of different enzyme variants, which are specifically engineered for InsP6 and the lower inositol phosphates (≤InsP4), respectively. In the future, all phytase applications will benefit from complete hydrolysis, regardless of whether they are in human nutrition, animal feeding, or P recovery. More efficient P utilization will 1) enable feed that carefully satisfy animal needs with respect to nutrient and mineral contents, 2) reduce the demand of P from rock mining, 3) minimize P leaching into the environment, and 4) improve P recovery technologies from biomass. We believe that tailor-made phytases are a key element for a more efficient resource utilization of P from phytate, which brings us closer to a responsible and sustainable use of this scarce resource in a circular bioeconomy.
Data Availability Statement
The original contributions presented in the study are included in the article/Supplementary Material, further inquiries can be directed to the corresponding authors.
Author Contributions
KH designed the study, performed the experiments, analyzed the data, supervised the work, and wrote the chapter. AJR contributed to the overall design and implementation of the research, and in the writing of the Result section. CB supported the culture and screening conditions for P. pastoris in MTP and was involved in the generation and in screening of the epPCR libraries. Within the framework of the Value-PP project, SeSaM-Biotech provided three epPCR libraries. JE and IH were involved in the generation and screening of SSM and SDM libraries. NS performed the computational analysis and MD predictions and wrote the corresponding chapter. MD contributed to the revision of the manuscript. US was involved in the project design, manuscript revision, and funding acquisition.
Funding
This work was funded by Deutsche Bundesstiftung Umwelt (DBU project Value-PP, AZ 33006/01-32; DBU project PHANG, AZ 34976/01-36). PyPEF was developed within the MERLIN project funded by Bundesministerium für Bildung und Forschung (BMBF; FKZ 01DJ20014). The authors gratefully acknowledge Sabine Willbold (Central Institute for Engineering, Electronics and Analytics, Analytics (ZEA-3), Jülich, Germany) for performing the NMR analysis.
Conflict of Interest
The authors declare that the research was conducted in the absence of any commercial or financial relationships that could be construed as a potential conflict of interest.
Publisher’s Note
All claims expressed in this article are solely those of the authors and do not necessarily represent those of their affiliated organizations, or those of the publisher, the editors, and the reviewers. Any product that may be evaluated in this article, or claim that may be made by its manufacturer, is not guaranteed nor endorsed by the publisher.
Supplementary Material
The Supplementary Material for this article can be found online at: https://www.frontiersin.org/articles/10.3389/fceng.2022.838056/full#supplementary-material
Abbreviations
Ec phy, E. coli phytase; InsP, inositol phosphate; MTP, microtiter plate; P, phosphorus.
References
Ariza, A., Moroz, O. V., Blagova, E. V., Turkenburg, J. P., Waterman, J., Roberts, S. M., et al. (2013). Degradation of Phytate by the 6-phytase from Hafnia Alvei: A Combined Structural and Solution Study. PLoS One 8, e65062. doi:10.1371/journal.pone.0065062
Bhuiya, M.-W., and Liu, C.-J. (2010). Engineering Monolignol 4-O-Methyltransferases to Modulate Lignin Biosynthesis. J. Biol. Chem. 285, 277–285. doi:10.1074/jbc.M109.036673
Brands, S., Brass, H. U. C., Klein, A. S., Sikkens, J. G., Davari, M. D., Pietruszka, J., et al. (2021). KnowVolution of Prodigiosin Ligase PigC towards Condensation of Short-Chain Prodiginines. Catal. Sci. Technol. 11, 2805–2815. doi:10.1039/D0CY02297G
Brune, M., Rossander-Hultén, L., Hallberg, L., Gleerup, A., and Sandberg, A.-S. (1992). Iron Absorption from Bread in Humans: Inhibiting Effects of Cereal Fiber, Phytate and Inositol Phosphates with Different Numbers of Phosphate Groups. J. Nutr. 122, 442–449. doi:10.1093/jn/122.3.442
Cadwell, R. C., and Joyce, G. F. (1992). Randomization of Genes by PCR Mutagenesis. Genome Res. 2, 28–33. doi:10.1101/gr.2.1.28
Chen, H., Cao, C., Kulinich, A., Liu, L., Jung, Y.-S., and Voglmeir, J. (2017). Engineering of an Episomal Plasmid Suitable for High-Throughput Expression in Pichia pastoris. Cchts 20, 726–733. doi:10.2174/1386207320666170925145531
Cheng, F., Zhu, L., and Schwaneberg, U. (2015). Directed Evolution 2.0: Improving and Deciphering Enzyme Properties. Chem. Commun. 51, 9760–9772. doi:10.1039/c5cc01594d
Cirino, P. C., Mayer, K. M., and Umeno, D. (2003). “Generating Mutant Libraries Using Error-Prone PCR,” in Directed Evolution Library Creation - Methods and Protocols. Editors F. H. Arnold, and G. Georgiou (Berlin: Springer), 3–9.
Contreras, F., Thiele, M. J., Pramanik, S., Rozhkova, A. M., Dotsenko, A. S., Zorov, I. N., et al. (2020). KnowVolution of a GH5 Cellulase from Penicillium verruculosum to Improve thermal Stability for Biomass Degradation. ACS Sustain. Chem. Eng. 8, 12388–12399. doi:10.1021/acssuschemeng.0c02465
Dennig, A., Marienhagen, J., Ruff, A. J., Guddat, L., and Schwaneberg, U. (2012). Directed Evolution of P 450 BM 3 into a P-Xylene Hydroxylase. ChemCatChem 4, 771–773. doi:10.1002/cctc.201200092
Dennig, A., Shivange, A. V., Marienhagen, J., and Schwaneberg, U. (2011). OmniChange: The Sequence Independent Method for Simultaneous Site-Saturation of Five Codons. PLoS One 6, e26222. doi:10.1371/journal.pone.0026222
Ensari, Y., de Almeida Santos, G., Ruff, A. J., and Schwaneberg, U. (2020). Engineered P450 BM3 and cpADH5 Coupled cascade Reaction for β-oxo Fatty Acid Methyl Ester Production in Whole Cells. Enzyme Microb. Techn. 138, 109555. doi:10.1016/j.enzmictec.2020.109555
Feng, X., Sanchis, J., Reetz, M. T., and Rabitz, H. (2012). Enhancing the Efficiency of Directed Evolution in Focused Enzyme Libraries by the Adaptive Substituent Reordering Algorithm. Chem. Eur. J. 18, 5646–5654. doi:10.1002/chem.201103811
Greiner, R., Alminger, M. L., and Carlsson, N.-G. (2001). Stereospecificity of Myo-Inositol Hexakisphosphate Dephosphorylation by a Phytate-Degrading Enzyme of Baker's Yeast. J. Agric. Food Chem. 49, 2228–2233. doi:10.1111/j.1745-4514.2001.tb00736.x
Greiner, R., Konietzny, U., and Jany, K. D. (1993). Purification and Characterization of Two Phytases from Escherichia coli. Arch. Biochem. Biophys. 303, 107–113. doi:10.1006/abbi.1993.1261
Greiner, R., and Konietzny, U. (2010). “Phytases: Biochemistry, Enzymology and Characteristics Relevant to Animal Feed Use,” in Enzymes in Farm Animal Nutrition. Editors M. R. Bedford, and G. Partridge (Oxfordshire: CABI), 96–128. doi:10.1079/9781845936747.0096
Gu, Y., Gao, J., Cao, M., Dong, C., Lian, J., Huang, L., et al. (2019). Construction of a Series of Episomal Plasmids and Their Application in the Development of an Efficient CRISPR/Cas9 System in Pichia pastoris. World J. Microbiol. Biotechnol. 35, 1–10. doi:10.1007/s11274-019-2654-5
Hartner, F. S., Ruth, C., Langenegger, D., Johnson, S. N., Hyka, P., Lin-Cereghino, G. P., et al. (2008). Promoter Library Designed for fine-tuned Gene Expression in Pichia pastoris. Nucleic Acids Res. 36, e76. doi:10.1093/nar/gkn369
Herrmann, K. R., Hofmann, I., Jungherz, D., Wittwer, M., Infanzón, B., Hamer, S. N., et al. (2021). Generation of Phytase Chimeras with Low Sequence Identities and Improved thermal Stability. J. Biotechnol. 339, 14–21. doi:10.1016/J.JBIOTEC.2021.07.005
Herrmann, K. R., Ruff, A. J., Infanzón, B., and Schwaneberg, U. (2019). Engineered Phytases for Emerging Biotechnological Applications beyond Animal Feeding. Appl. Microbiol. Biotechnol. 103, 6435–6448. doi:10.1007/s00253-019-09962-1
Herrmann, K. R., Ruff, A. J., and Schwaneberg, U. (2020). Phytase-based Phosphorus Recovery Process for 20 Distinct Press Cakes. ACS Sustain. Chem. Eng. 8, 3913–3921. doi:10.1021/acssuschemeng.9b07433
Hogrefe, H. H., Cline, J., Youngblood, G. L., and Allen, R. M. (2002). Creating Randomized Amino Acid Libraries with the QuikChange Multi Site-Directed Mutagenesis Kit. Biotechniques 33, 1158–1165. doi:10.2144/02335pf01
Huang, H., Luo, H., Wang, Y., Fu, D., Shao, N., Wang, G., et al. (2008). A Novel Phytase from Yersinia Rohdei with High Phytate Hydrolysis Activity under Low pH and strong Pepsin Conditions. Appl. Microbiol. Biotechnol. 80, 417–426. doi:10.1007/s00253-008-1556-5
Infanzón, B., Herrmann, K. R., Hofmann, I., Willbold, S., Ruff, A. J., and Schwaneberg, U. (2022). Phytase Blends for Enhanced Phosphorous Mobilization of Deoiled Seeds. Enzyme Microb. Techn. 153, 109953. doi:10.1016/j.enzmictec.2021.109953
Islam, S., Laaf, D., Infanzón, B., Pelantová, H., Davari, M. D., Jakob, F., et al. (2018). KnowVolution Campaign of an Aryl Sulfotransferase Increases Activity toward Cellobiose. Chem. Eur. J. 24, 17117–17124. doi:10.1002/CHEM.201803729
Ji, Y., Islam, S., Cui, H., Dhoke, G. V., Davari, M. D., Mertens, A. M., et al. (2020). Loop Engineering of Aryl Sulfotransferase B for Improving Catalytic Performance in Regioselective Sulfation. Catal. Sci. Technol. 10, 2369–2377. doi:10.1039/D0CY00063A
Jia, Z., Lim, D., Golovan, S., and Forsberg, C. W. (2000). Crystal Structures of Escherichia coli Phytase and its Complex with Phytate. Nat. Struct. Biol. 7, 108–113. doi:10.1038/72371
Johansson, C., Kördel, J., and Drakenberg, T. (1990). Analysis of Myo-Inositol Phosphates by 2D 1H-n.m.R. Spectroscopy. Carbohydr. Res. 207, 177–183. doi:10.1016/0008-6215(90)84047-X
Kastilan, R., Boes, A., Spiegel, H., Voepel, N., Chudobová, I., Hellwig, S., et al. (2017). Improvement of a Fermentation Process for the Production of Two PfAMA1-DiCo-Based Malaria Vaccine Candidates in Pichia pastoris. Sci. Rep. 7, 1–13. doi:10.1038/s41598-017-11819-4
Kawashima, S., Pokarowski, P., Pokarowska, M., Kolinski, A., Katayama, T., and Kanehisa, M. (2008). AAindex: Amino Acid index Database, Progress Report 2008. Nucleic Acids Res. 36, D202–D205. doi:10.1093/NAR/GKM998
Kim, D.-H., Oh, B.-C., Choi, W.-C., Lee, J.-K., and Oh, T.-K. (1999). Enzymatic Evaluation of Bacillus Amyloliquefaciens Phytase as a Feed Additive. Biotechnol. Lett. 21, 925–927. doi:10.1023/A:1005602717835
Kim, M.-S., and Lei, X. G. (2008). Enhancing Thermostability of Escherichia coli Phytase AppA2 by Error-Prone PCR. Appl. Microbiol. Biotechnol. 79, 69–75. doi:10.1007/s00253-008-1412-7
Konietzny, U., and Greiner, R. (2002). Molecular and Catalytic Properties of Phytate-Degrading Enzymes (Phytases). Int. J. Food Sci. Tech. 37, 791–812. doi:10.1046/j.1365-2621.2002.00617.x
Krieger, E., and Vriend, G. (2014). YASARA View-Molecular Graphics for All Devices-From Smartphones to Workstations. Bioinformatics 30, 2981–2982. doi:10.1093/BIOINFORMATICS/BTU426
Lei, X. G., Weaver, J. D., Mullaney, E., Ullah, A. H., and Azain, M. J. (2013). Phytase, a New Life for an “Old” Enzyme. Annu. Rev. Anim. Biosci. 1, 283–309. doi:10.1146/annurev-animal-031412-103717
Li, G., Dong, Y., and Reetz, M. T. (2019). Can Machine Learning Revolutionize Directed Evolution of Selective Enzymes. Adv. Synth. Catal. 361, 2377–2386. doi:10.1002/ADSC.201900149
Lutz, S. (2010). Beyond Directed Evolution-Semi-Rational Protein Engineering and Design. Curr. Opin. Biotechnol. 21, 734–743. doi:10.1016/j.copbio.2010.08.011
Mandawe, J., Infanzon, B., Eisele, A., Zaun, H., Kuballa, J., Davari, M. D., et al. (2018). Directed Evolution of Hyaluronic Acid Synthase fromPasteurella Multocidatowards High-Molecular-Weight Hyaluronic Acid. ChemBioChem 19, 1414–1423. doi:10.1002/CBIC.201800093
Mazurenko, S., Prokop, Z., and Damborsky, J. (2019). Machine Learning in Enzyme Engineering. ACS Catal. 10, 1210–1223. doi:10.1021/acscatal.9b04321
Miksch, G., Kleist, S., Friehs, K., and Flaschel, E. (2002). Overexpression of the Phytase from Escherichia coli and its Extracellular Production in Bioreactors. Appl. Microbiol. Biotechnol. 59, 685–694. doi:10.1007/s00253-002-1071-z
Murphy, J., and Riley, J. P. (1962). A Modified Single Solution Method for the Determination of Phosphate in Natural Waters. Analytica Chim. Acta 27, 31–36. doi:10.1016/S0003-2670(00)88444-5
Murphy, J., and Riley, J. P. (1958). A Single-Solution Method for the Determination of Soluble Phosphate in Sea Water. J. Mar. Biol. Ass. 37, 9–14. doi:10.1017/S0025315400014776
Näätsaari, L., Mistlberger, B., Ruth, C., Hajek, T., Hartner, F. S., and Glieder, A. (2012). Deletion of the Pichia pastoris KU70 Homologue Facilitates Platform Strain Generation for Gene Expression and Synthetic Biology. PLoS One 7, e39720. doi:10.1371/journal.pone.0039720
Nikolaev, D. M., Shtyrov, A. A., Panov, M. S., Jamal, A., Chakchir, O. B., Kochemirovsky, V. A., et al. (2018). A Comparative Study of Modern Homology Modeling Algorithms for Rhodopsin Structure Prediction. ACS Omega 3, 7555–7566. doi:10.1021/acsomega.8b00721
Nobili, A., Gall, M. G., Pavlidis, I. V., Thompson, M. L., Schmidt, M., and Bornscheuer, U. T. (2013). Use of 'small but Smart' Libraries to Enhance the Enantioselectivity of an Esterase fromBacillus Stearothermophilustowards Tetrahydrofuran-3-Yl Acetate. FEBS J. 280, 3084–3093. doi:10.1111/febs.12137
Novoa, C., Dhoke, G. V., Mate, D. M., Martínez, R., Haarmann, T., Schreiter, M., et al. (2019). KnowVolution of a Fungal Laccase toward Alkaline pH. ChemBioChem 20, 1458–1466. doi:10.1002/CBIC.201800807
Pedregosa, F., Varoquaux, G., Gramfort, A., Michel, V., Thirion, B., Grisel, O., et al. (2011). Scikit-learn: Machine Learning in Python. J. Mach. Learn. Res. 12, 2825–2830. doi:10.5555/1953048
Pettersen, E. F., Goddard, T. D., Huang, C. C., Couch, G. S., Greenblatt, D. M., Meng, E. C., et al. (2004). UCSF Chimera?A Visualization System for Exploratory Research and Analysis. J. Comput. Chem. 25, 1605–1612. doi:10.1002/jcc.20084
Phillippy, B. Q., and Bland, J. M. (1988). Gradient Ion Chromatography of Inositol Phosphates. Anal. Biochem. 175, 162–166. doi:10.1016/0003-2697(88)90374-0
Pontoppidan, K., Glitsoe, V., Guggenbuhl, P., Quintana, A. P., Nunes, C. S., Pettersson, D., et al. (2012). In Vitroandin Vivodegradation Ofmyo-Inositol Hexakisphosphate by a Phytase fromCitrobacter Braakii. Arch. Anim. Nutr. 66, 431–444. doi:10.1080/1745039X.2012.735082
Poulsen, H. D., Jongbloed, A. W., Latimier, P., and Fernández, J. A. (1999). Phosphorus Consumption, Utilisation and Losses in Pig Production in France, The Netherlands and Denmark. Livestock Prod. Sci. 58, 251–259. doi:10.1016/S0301-6226(99)00013-5
Ragon, M., Aumelas, A., Chemardin, P., Galvez, S., Moulin, G., and Boze, H. (2008). Complete Hydrolysis of Myo-Inositol Hexakisphosphate by a Novel Phytase from Debaryomyces Castellii CBS 2923. Appl. Microbiol. Biotechnol. 78, 47–53. doi:10.1007/s00253-007-1275-3
Rowe, L. A., Geddie, M. L., Alexander, O. B., and Matsumura, I. (2003). A Comparison of Directed Evolution Approaches Using the β-Glucuronidase Model System. J. Mol. Biol. 332, 851–860. doi:10.1016/S0022-2836(03)00972-0
Rübsam, K., Davari, M., Jakob, F., and Schwaneberg, U. (2018). KnowVolution of the Polymer-Binding Peptide LCI for Improved Polypropylene Binding. Polymers 10, 423. doi:10.3390/POLYM10040423
Ruff, A. J., Dennig, A., and Schwaneberg, U. (2013). To Get what We Aim for - Progress in Diversity Generation Methods. FEBS J. 280, 2961–2978. doi:10.1111/febs.12325
Sandberg, A.-S., and Ahderinne, R. (1986). HPLC Method for Determination of Inositol Tri-, Tetra-, Penta-, and Hexaphosphates in Foods and Intestinal Contents. J. Food Sci. 51, 547–550. doi:10.1111/j.1365-2621.1986.tb13875.x
Sarkisyan, K. S., Bolotin, D. A., Meer, M. V., Usmanova, D. R., Mishin, A. S., Sharonov, G. V., et al. (2016). Local Fitness Landscape of the green Fluorescent Protein. Nature 533, 397–401. doi:10.1038/nature17995
Schmidt-Dannert, C., and Arnold, F. H. (1999). Directed Evolution of Industrial Enzymes. Trends Biotechnol. 17, 135–136. doi:10.1016/S0167-7799(98)01283-9
Shivange, A. V., Roccatano, D., and Schwaneberg, U. (2016). Iterative Key-Residues Interrogation of a Phytase with Thermostability Increasing Substitutions Identified in Directed Evolution. Appl. Microbiol. Biotechnol. 100, 227–242. doi:10.1007/s00253-015-6959-5
Shivange, A. V., and Schwaneberg, U. (2017). “Recent Advances in Directed Phytase Evolution and Rational Phytase Engineering,” in Directed Enzyme Evolution: Advances and Applications. Editor M. Alcalde (BerlinCham: Springer), 145–172. doi:10.1007/978-3-319-50413-1_6
Shivange, A. V., Serwe, A., Dennig, A., Roccatano, D., Haefner, S., and Schwaneberg, U. (2012). Directed Evolution of a Highly Active Yersinia Mollaretii Phytase. Appl. Microbiol. Biotechnol. 95, 405–418. doi:10.1007/s00253-011-3756-7
Siedhoff, N. E., Illig, A.-M., Schwaneberg, U., and Davari, M. D. (2021). PyPEF-An Integrated Framework for Data-Driven Protein Engineering. J. Chem. Inf. Model. 61, 3463–3476. doi:10.1021/acs.jcim.1c00099
Skoglund, E., Carlsson, N.-G., and Sandberg, A.-S. (1998). High-Performance Chromatographic Separation of Inositol Phosphate Isomers on Strong Anion Exchange Columns. J. Agric. Food Chem. 46, 1877–1882. doi:10.1021/jf9709257
Tai, H.-M., Yin, L.-J., Chen, W.-C., and Jiang, S.-T. (2013). Overexpression of Escherichia coli Phytase in Pichia pastoris and its Biochemical Properties. J. Agric. Food Chem. 61, 6007–6015. doi:10.1021/jf401853b
Tan, H., Miao, R., Liu, T., Cao, X., Wu, X., Xie, L., et al. (2016). Enhancing the thermal Resistance of a Novel Acidobacteria-Derived Phytase by Engineering of Disulfide Bridges. J. Microbiol. Biotechnol. 26, 1717–1722. doi:10.4014/jmb.1604.04051
Tee, K. L., and Wong, T. S. (2013). Polishing the Craft of Genetic Diversity Creation in Directed Evolution. Biotechnol. Adv. 31, 1707–1721. doi:10.1016/j.biotechadv.2013.08.021
Wallraf, A.-M., Liu, H., Zhu, L., Khalfallah, G., Simons, C., Alibiglou, H., et al. (2018). A Loop Engineering Strategy Improves Laccase Lcc2 Activity in Ionic Liquid and Aqueous Solution. Green. Chem. 20, 2801–2812. doi:10.1039/C7GC03776G
Wittmann, B. J., Johnston, K. E., Wu, Z., and Arnold, F. H. (2021). Advances in Machine Learning for Directed Evolution. Curr. Opin. Struct. Biol. 69, 11–18. doi:10.1016/j.sbi.2021.01.008
Wu, T.-H., Chen, C.-C., Cheng, Y.-S., Ko, T.-P., Lin, C.-Y., Lai, H.-L., et al. (2014). Improving Specific Activity and Thermostability of Escherichia coli Phytase by Structure-Based Rational Design. J. Biotechnol. 175, 1–6. doi:10.1016/j.jbiotec.2014.01.034
Wyss, M., Brugger, R., Kronenberger, A., Rémy, R., Fimbel, R., Oesterhelt, G., et al. (1999). Biochemical Characterization of Fungal Phytases (Myo -Inositol Hexakisphosphate Phosphohydrolases): Catalytic Properties. Appl. Environ. Microbiol. 65, 367–373. doi:10.1128/aem.65.2.367-373.1999
Xu, P., Price, J., Wise, A., and Aggett, P. J. (1992). Interaction of Inositol Phosphates with Calcium, Zinc, and Histidine. J. Inorg. Biochem. 47, 119–130. doi:10.1016/0162-0134(92)84048-R
Yang, K. K., Wu, Z., and Arnold, F. H. (2019). Machine-learning-guided Directed Evolution for Protein Engineering. Nat. Methods 16, 687–694. doi:10.1038/s41592-019-0496-6
Yao, M.-Z., Wang, X., Wang, W., Fu, Y.-J., and Liang, A.-H. (2013). Improving the Thermostability of Escherichia coli Phytase, appA, by Enhancement of Glycosylation. Biotechnol. Lett. 35, 1669–1676. doi:10.1007/s10529-013-1255-x
Yu, S., Cowieson, A., Gilbert, C., Plumstead, P., and Dalsgaard, S. (2012). Interactions of Phytate and Myo-Inositol Phosphate Esters (IP1-5) Including IP5 Isomers with Dietary Protein and Iron and Inhibition of Pepsin1. J. Anim. Sci. 90, 1824–1832. doi:10.2527/jas.2011-3866
Keywords: appA, protein engineering, directed evolution, phytate hydrolysis, P, phosphorus, InsP4, machine learning
Citation: Herrmann KR, Brethauer C, Siedhoff NE, Hofmann I, Eyll J, Davari MD, Schwaneberg U and Ruff AJ (2022) Evolution of E. coli Phytase Toward Improved Hydrolysis of Inositol Tetraphosphate. Front. Chem. Eng. 4:838056. doi: 10.3389/fceng.2022.838056
Received: 17 December 2021; Accepted: 20 January 2022;
Published: 09 March 2022.
Edited by:
Sabino De Gisi, Politecnico di Bari, ItalyCopyright © 2022 Herrmann, Brethauer, Siedhoff, Hofmann, Eyll, Davari, Schwaneberg and Ruff. This is an open-access article distributed under the terms of the Creative Commons Attribution License (CC BY). The use, distribution or reproduction in other forums is permitted, provided the original author(s) and the copyright owner(s) are credited and that the original publication in this journal is cited, in accordance with accepted academic practice. No use, distribution or reproduction is permitted which does not comply with these terms.
*Correspondence: Ulrich Schwaneberg, u.schwaneberg@biotec.rwth-aachen.de; Anna Joëlle Ruff, aj.ruff@biotec.rwth-aachen.de
†ORCID: Ulrich Schwaneberg, 0000-0003-4026-701X; Anna Joelle Ruff, 0000-0001-9318-052X; Kevin R. Herrmann, 0000-0002-5224-0239; Niklas E. Siedhoff, 0000-0002-1131-4924; Mehdi D. Davari, 0000-0003-0089-7156; Christin Brethauer, 0000-0003-4794-0203
‡Present Address: Mehdi D. Davari, Department of Bioorganic Chemistry, Leibniz Institute of Plant Biochemistry, Halle, Germany
§These authors share first authorship