Cellular Self-Structuring and Turbulent Behaviors in Atmospheric Laminar Channels
- 1Faculty of Physics, Alexandru Ioan Cuza University of Iaşi, Iaşi, Romania
- 2Department of Physics, Gheorghe Asachi Technical University of Iaşi, Iaşi, Romania
- 3Department of Geography, Faculty of Geography and Geology, Alexandru Ioan Cuza University of Iaşi, Iaşi, Romania
- 4Romanian Scientists Academy, Bucharest, Romania
Assimilating the atmosphere with multifractal entities, nonlinear behaviors in the framework of scale relativity theory regarding its hydrodynamic functionality are established at various scale resolutions. From this perspective, revealing a “hidden” symmetry of the specific multifractal force with the
Introduction
In order to better introduce and contextualize this manuscript, our past works on the subject must be first detailed and the theoretical and practical results obtained must be explained. In the first of these works, a multifractal approach of the turbulent atmosphere is generally considered, whereby implementing this theoretical framework through non-differentiable functions in the form of scale relativity theory, the fractal minimal vortex of an instance of turbulent flow is obtained (Roşu et al., 2020). This leads to equations that describe the minimal vortex itself, and the velocity fields that compose it. Once this theoretical framework has been established, certain relations are exploited in order to calculate turbulent diffusion using lidar data, and the resulting profiles are found to be in agreement with other associated published works (Roșu et al., 2020). In the second paper, the connection between atmospheric multifractal theory and lidar data is further explored by improving a turbulence cascade evolution model so that each vortex presents an increasing Hausdorff dimension (Roșu et al., 2021a). In the third paper, the improved model is found to be resulting from a gauge produced by scaling theories, which implies that it is part of a large class of possible models – most notably, however, the model is coupled to a Galerkin decomposition of the Navier-Stokes equations which produces a logistic map-type evolution equation of velocity modes (Roșu et al., 2021b). Then, this coupling makes it possible to identify quasi-laminar or fully laminar regions in atmospheric profiles, leading to “laminar channel” structures that show either ascending or descending behavior (Roșu et al., 2021b). Finally, developments were also made towards reducing the number of initial parameters that the improved turbulence cascade evolution model requires (Roșu et al., 2021b). In the current work, the particularities of these laminar channels, and laminarity in general in the context of multifractality, self-structuring of atmospheric entities and the implications of self-structuring shall be the focus of our analysis.
The Motion Operator as the Scale Covariant Derivative
Since the atmosphere both structurally and functionally can be assimilated to a multifractal object, its dynamics are characterized in the frame of scale relativity theory through the scale covariance derivative (Roșu et al., 2020; Roșu et al., 2021a; Roșu et al., 2021b):
where:
and:
-
-
-
-
-
-
-
-
-
-
In the case of atmosphere dynamics through stochastic fractalization/multifractalization (for example, Markov-type stochastic processes, non-Markov stochastic processes, etc.) we may distinguish the following patterns:
1) patterns which include atmospheric processes through homogenous behaviors characterized by a single fractal dimension, that possess the same scaling properties in any time interval (monofractal) (Jackson, 1989; Cristescu, 2008);
2) patterns which include atmospheric processes through non-homogenous behaviors characterized simultaneously by multiple fractal dimensions (multifractal). Thus, the spectrum
Then, instead of “working” with a single variable described by a strict, non-differentiable function, it is possible to “operate” only with approximations of this mathematical function, obtained by averaging them on different scale resolutions. As a consequence, any variable purposed to describe atmospheric processes will still perform as the limit of a family of mathematical functions, this being non-differentiable for null scale resolutions and differentiable otherwise (Nottale, 2011; Merches and Agop, 2015; Agop and Paun, 2017). There are many ways to define the fractal dimension: Kolmogorov, Hausdorff-Besikovitch, etc. definitions, but once one chosen to employ it in the atmosphere dynamics, it should be constant and arbitrary for the entirety of our analysis (Mandelbrot, 1982; Jackson, 1989; Cristescu, 2008).
Conservation Laws of the Multifractal Flows
Now, considering the scale covariance principle and using the operator in Eq. 1 applied to the complex velocities from Eq. 2, without any constraints, the multifractal conservation law of the specific momentum can be written in the form:
where
which reflects the fact that the motions of the atmospheric entities involve interdependent complex mechanisms, both at differential and non-differential scale resolutions.
Since, usually, multifractalization proves to be reducible to stochasticity, next we will consider the case of multifractalization by Markov-type stochastic processes, which imply the following conditions:
where
In conditions expressed by Eq. 6, Eq. 3 becomes:
in which case the separation of the atmospheric dynamics on scale resolutions implies the functionality of the following differential equations for the velocity fields:
For laminar movements of atmospheric entities, the complex velocity fields given by Eq. 2a become:
with
where
It is also possible to see from the previous equations that
By Eqs 13–15, Eq. 8 implies the multifractal hydrodynamic equations:
where with
The Eq. 16 is the multifractal conservation law of the specific momentum, while Eq. 17 is the multifractal conservation law of the states density. The potential
which quantifies the multifractality degrees of the motion trajectories of the atmospheric particles.
Coherences in Stationary Atmospheric Dynamics Through a “Hidden” Symmetry
The existence of this force will be considered as the “trigger” of the atmospheric processes that lead to turbulence. If the specific multifractal potential is constant, or if in the one-dimensional case the following condition is satisfied:
where
where
The quantities
where:
and
First, let us observe a “universal” projective parameter – the ratio of the fundamental solutions of Eq. 20 gives:
Every homographic function of this type will be a projective parameter. Among others, the function:
has the advantage to be specific to every atmospheric entity. This is not all; let another function:
which is specific to a different atmospheric entity. The fact that Eq. 26 and Eq. 27 are solutions of Eq. 23 shows that between them there is the homographic relation:
which implies the transformations group:
Equation 29 can be assimilated to a group of synchronisms between the diverse entities of the atmosphere, a process which includes synchronization between the amplitudes and phases of the atmospheric entities.
The structure of this group is typical of
where
This group admits the absolute invariant differentials:
and the 2-form (the metric):
In real terms:
and for:
the connection with Poincaré representation of the Lobachevsky plane can be obtained. Indeed, the metric is a three-dimensional Lorentz structure:
This metric reduces to that of Poincaré in case where
represents the connection form of the hyperbolic plane, the Eq. 35 then represents general Bäcklund transformation in that plane. In such a conjecture, the metric represented by Eq. 36 with the restrictions represented by Eq. 37 becomes:
It is worthwhile to mention a property connected to the integral geometry: the group in Ec. (29) is measurable (Sors and Santaló, 2004). Indeed, it is simple transitive since its structure vectors:
are identically null as it can be seen from Ec. (30), this means that is possesses an invariant function given by:
As a result, in the space of variables
where
Nonlinear Behaviors in Atmospheric Dynamics Through Harmonic Mappings
Let us suppose that the atmosphere dynamics are described by the variables
in an ambient space of metric:
In this situation, the field equations of the atmosphere dynamics are derived from a variational principle, connected to the Lagrangian:
In our case, the Eq. 42a is given by Eq. 38, the field variables being
is accepted as a starting point where
which admits the solution:
with
The previous relation can also be rewritten as:
In Figures 1–3 we shall present multiple nonlinear behaviors of atmospheric dynamics at scale resolutions in dimensionless coordinates: 1) nonlinear behaviors at a global scale resolution (Figures 1A–C); 2) non-dissipative nonlinear behaviors at a differentiable scale resolution (Figures 2A–C); 3) dissipative nonlinear behaviors at a non-differentiable scale resolution (Figures 3A–D). Let us note that, whatever the scale resolution, atmospheric dynamics prove themselves to be reducible to self-structuring cellular patterns. Furthermore, a “dephasing” between the positive and the negative parts of the imaginary part of, or the positive and negative sides of the dissipative nonlinear behaviors, can be observed (Figures 3B,C).
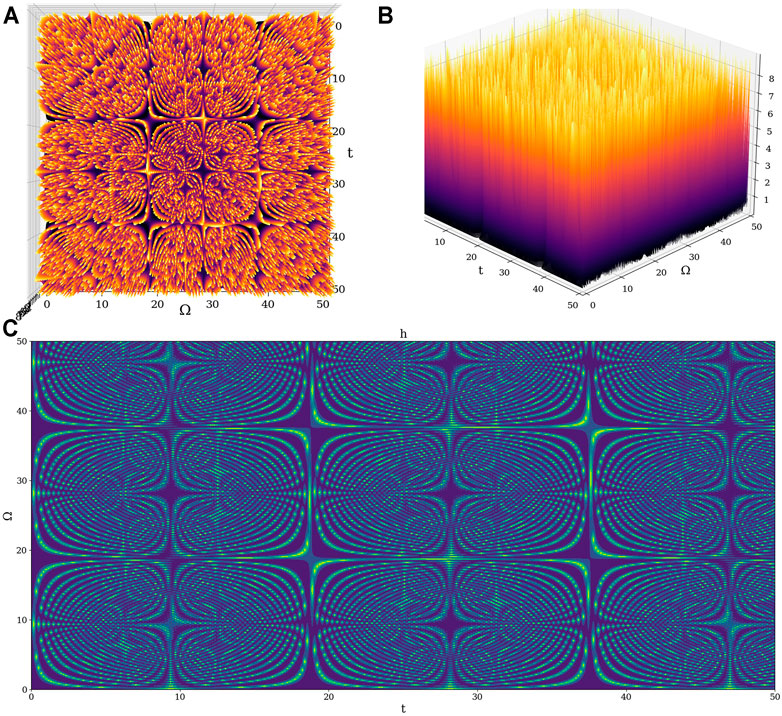
FIGURE 1. Example 3D plot of
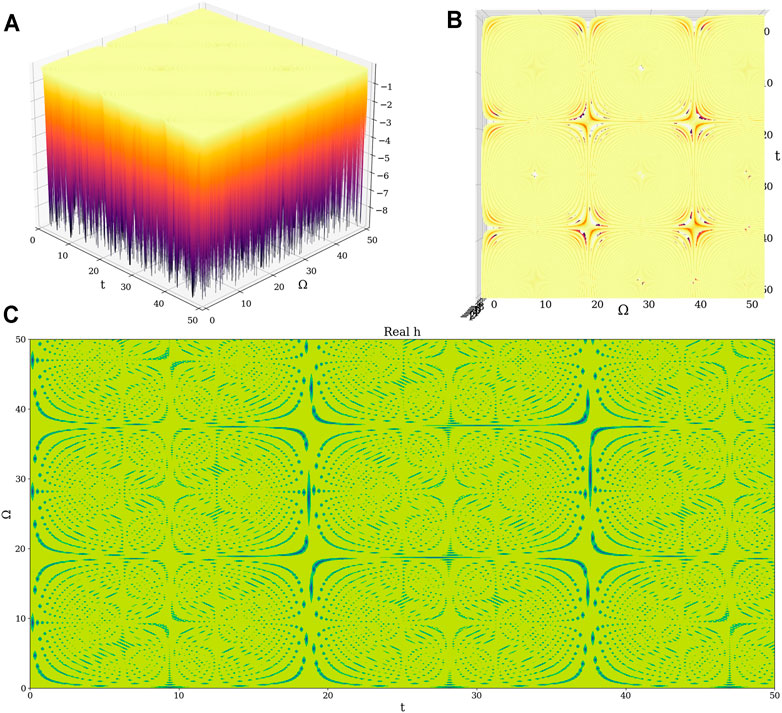
FIGURE 2. Example 3D plot of
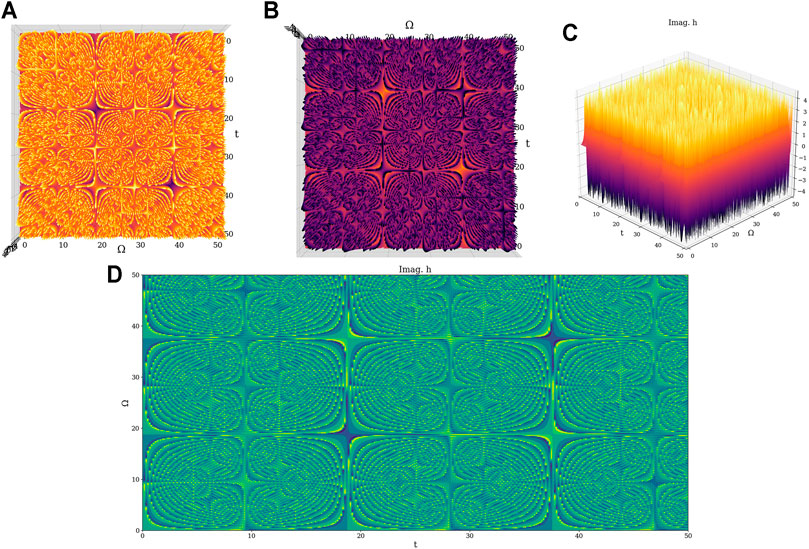
FIGURE 3. Example 3D plot of
Let us also note that the mathematical formalism of the Multifractal Theory of Motion naturally implies various operational procedures (invariance groups, harmonic mappings, groups isomorphism, embedding manifolds etc.) with quite a number of applications in complex systems dynamics (Agop and Merches, 2018; Mazilu et al., 2019). Interestingly, plotting
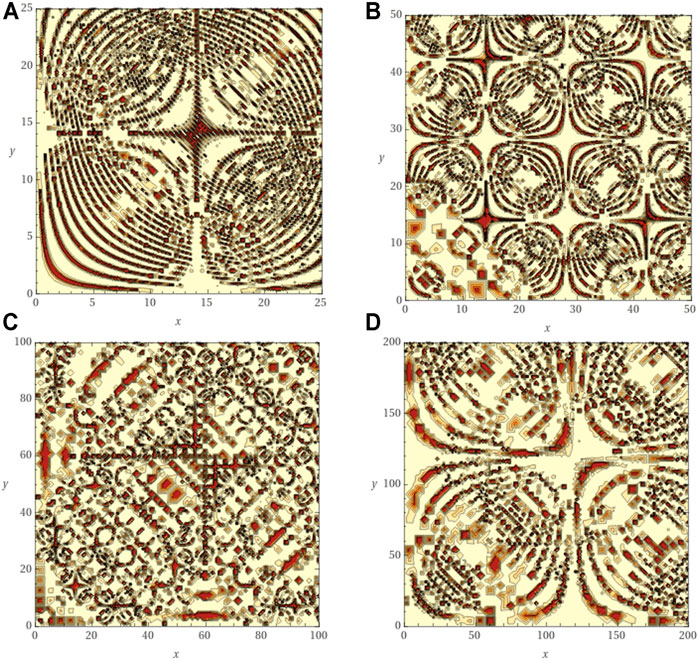
FIGURE 5. Example 2D plot of
The results presented in Figures 4, 5 also specify that, through self-structuring of atmospheric entities scale, transitions induced through the modification of fractal dimensions of movement curves that describe atmospheric dynamics can be assimilated to laminar channels. These channels are also described and observed in experimental data in our previous work (Roșu et al., 2021b).
Other nonlinear behaviors can be obtained by expanding the states density, the specific multifractal potential, and the multifractal non-differentiable velocity field. To this end, let us rewrite Eq. 22 based on Eq. 47, which produces:
In Figures 6A–C Eq. 48 is represented in dimensionless coordinates for a spontaneous symmetry break obtained through a Wick-type rotation in the hyperbolic plane (Ovchinnikov, 2016; Ovchinnikov et al., 2016; Hamilton, 2017). In such an operational procedure in dimensionless coordinates,
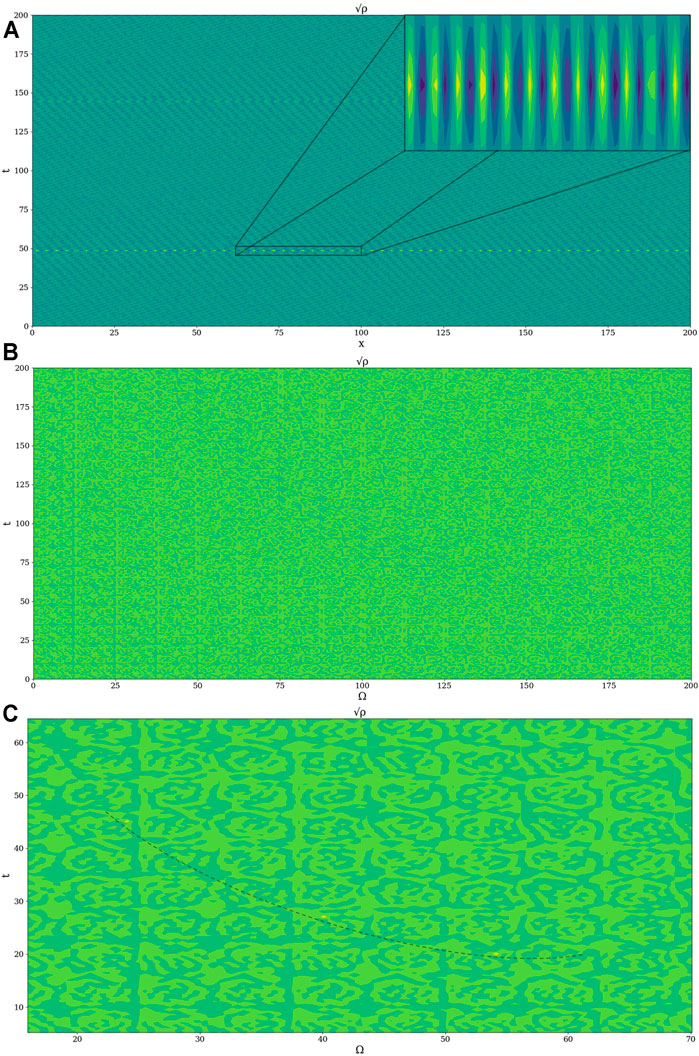
FIGURE 6. Example 2D plot of
Moving on to plots of other parameters of interest, the
De aici, one can now calculate
The difference between the standard fluctuations of the plotted parameter fields and the spontaneous symmetry break peaks is too high in order to correctly show the orders of magnitude of each at the same time (Figures 7, 8). It must also be specified that these spontaneous symmetry break peaks are not calculus artefacts produced by point-wise derivation through Python code; the plots are drawn from analytically obtained functions.
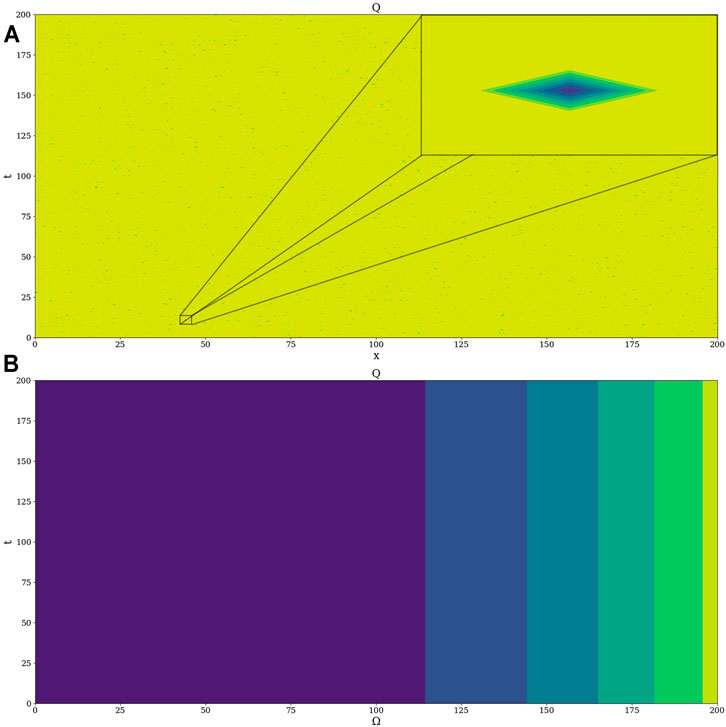
FIGURE 7. Example 2D plot of
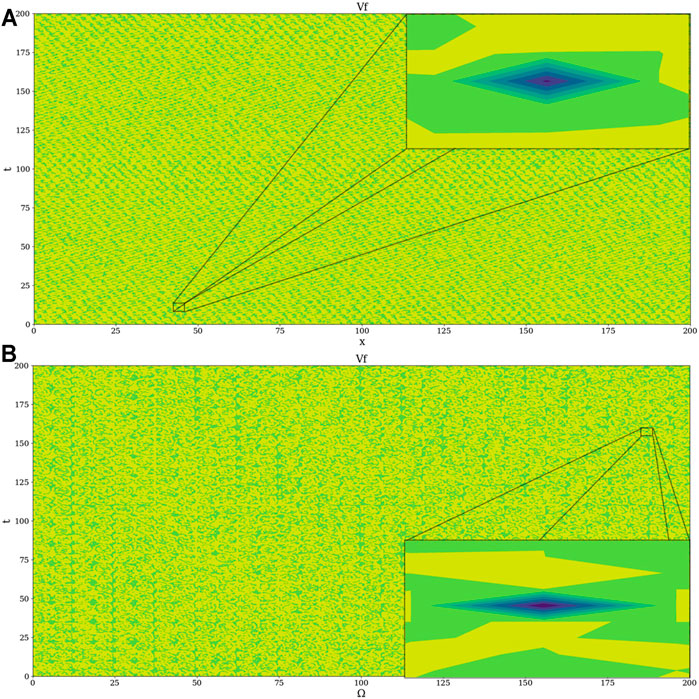
FIGURE 8. Example 2D plot of
The occurrence of anomalous, intense positive and negative peaks in the topology of our plots shows local spontaneous symmetry break for our equations corresponding to the
The objective now is to determine how and when these symmetry breaks occur, and this shall be done by checking the general variability of the potential at a varying
However, given our relatively limited spatial conditions, it shall suffice to consider the analysis of
This parameter can then be iterated across
Thus, there is a very clear and ordered intermittency between homogeneity, inhomogeneity, and “mixture” states, and it is this behavior that gives rise to the gradual process of turbulent energy cascades. All throughout this analysis, it is important to remember that the bifurcation maps show the “potentiality of turbulence” of a given flow at a given point as
In our previous works, we have made use of a modified
Given the fact that
In our previous study, a similar conclusion regarding the
In order to better illustrate the potential origin of this quasi-periodic and intermittent character of the inhomogeneity of the multifractal potential, it is possible to rewrite
This can then be considered as:
with:
which results in:
This function has been plotted in Figure 10. In itself, the simple trigonometric function
In terms of the theory presented here, our results clearly suggest that the transition from laminar to chaotic might come from spontaneous sources; the opposite is also true, given the correspondence between the logistic map and the Navier-Stokes equation and the sudden intervals of stability found in the logistic map throughout the Pomeau-Manneville area. This then suggests that there exist laminar channels throughout the atmosphere – not only that, but the cellular linear entities found in
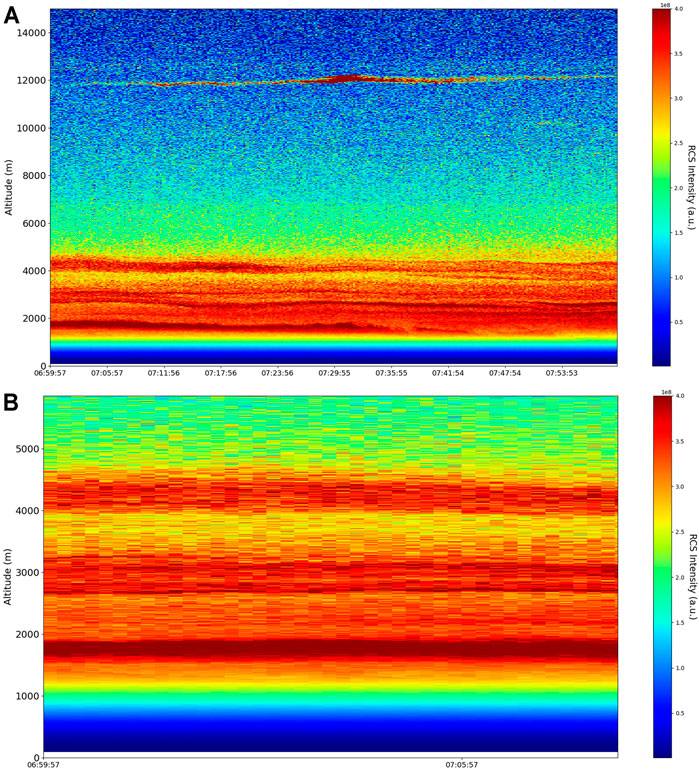
FIGURE 11. (A) RCS timeseries, Bucharest, Romania, 13/06/2019, (B) zoomed RCS timeseries, Bucharest, Romania, 13/06/2019; area of interest for subsequent laminar channel study.
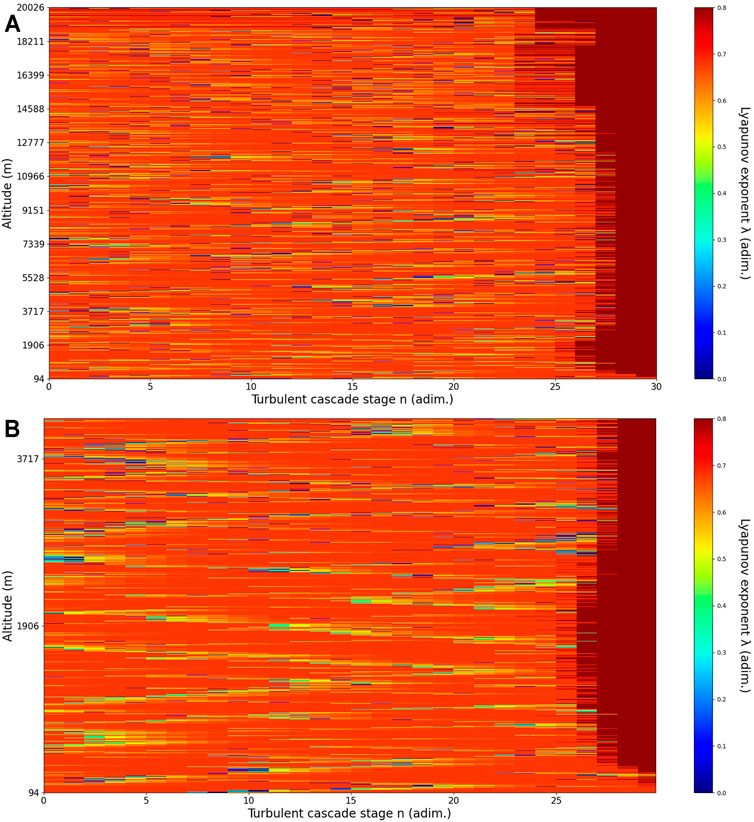
FIGURE 12. (A) Lyapunov exponent per turbulent cascade stage colormap altitude plot, Bucharest, Romania, 13/06/2019, 07:00:00, (B) zoomed-in Lyapunov exponent per turbulent cascade stage colormap altitude plot, Bucharest, Romania, 13/06/2019, 07:00:00.
The RCS data used in this study has been obtained from the RALI Multiwavelength Raman Lidar Platform, which is a part of the National Institute for Research and Development in Optoelectronics INOE 2000 in Bucharest, Romania, 93
Regarding the obtained RCS data, many features of the atmosphere can be directly observed in what appears to be a calm, typical atmospheric scenario, including but not limited to stratospheric cirrus clouds, pollutant plumes and the PBL – it possible to confidently exclude the presence of clouds in the lower atmosphere, given the fact that Meteomanz.com displays clear conditions for the “Bucuresti Filaret” station, which happens to be closest to the lidar platform, and also given the fact that ACTRIS lidar data collecting procedure demands that data collecting start only during clear conditions (Figure 11). In relation to the laminar analysis, certain “V-shaped structures” can be observed; these are the laminar channels, and their presence is given by the fact that, at a certain altitude, the inequality necessary for laminar behavior is satisfied, and then satisfied again at a different altitude at a different or similar scale (Figure 12). We thus identify “ascending” or “descending” laminar channels, and these structures can help explain various atmospheric transport phenomena regarding aerosols and cloud formations, but also regarding PBL stability (Figure 12). The classification difference between “ascending” and “descending” is justified by the fact that the turbulent cascade progresses from larger to smaller scales; thus, it is necessary to assume that a continuation of the laminar channel at higher altitude towards smaller scales indicates, for example, that the channel is ascendent.
Conclusion
In this study, we have found that representing laminar atmospheric flows in a multifractal framework reveals unexpected results that might explain emergent turbulent behavior. Even by approaching these equations from an irrotational perspective, it is shown that relations between them and the
Data Availability Statement
The raw data supporting the conclusions of this article will be made available by the authors, without undue reservation.
Author Contributions
Conceptualization, I-AR, MC, D-CN, and MA; methodology, I-AR and MA; software, I-AR; validation, I-AR, MC, D-CN, and MA; formal analysis, I-AR, MC, D-CN, and MA; investigation, I-AR, MC, D-CN, and MA; resources, I-AR, D-CN, and MC; data curation, I-AR, MC, and MA; writing—original draft preparation, I-AR, MC, D-CN, and MA; writing—review and editing, I-AR, MC, D-CN, and MA; visualization, I-AR, MC, D-CN, and MA; supervision, MA and D-CN; project administration, I-AR, MC, D-CN, and MA; funding acquisition, I-A.R, D-CN, and MC. All authors have read and agreed to the published version of the manuscript.
Funding
This work was supported by a grant from the Romanian Ministry of Education and Research, CNCS-UEFISCDI, project number PN-III-P1-1.1-TE-2019-1921, within PNCDI III.
Conflict of Interest
The authors declare that the research was conducted in the absence of any commercial or financial relationships that could be construed as a potential conflict of interest.
Publisher’s Note
All claims expressed in this article are solely those of the authors and do not necessarily represent those of their affiliated organizations, or those of the publisher, the editors and the reviewers. Any product that may be evaluated in this article, orclaim that may be made by its manufacturer, is not guaranteed or endorsed by the publisher.
Acknowledgments
The authors acknowledge the RADO (Romanian Atmospheric 3D research Observatory) and ACTRIS – RO (Aerosol, Clouds and Trace gases Research InfraStructure - Romania) for providing the lidar data used in this study. They also acknowledge the Faculty of Geography and Geology of the “Alexandru Ioan Cuza” University of Iaşi for the financial support offered for the publication of this study.
References
Adam, M., Nicolae, D., Stachlewska, I. S., Papayannis, A., and Balis, D. (2020). Biomass burning events measured by lidars in EARLINET - Part 1: Data analysis methodology. Atmos. Chem. Phys., 20, 13905–13927. doi:10.5194/acp-20-13905-2020
Agop, M., and Mazilu, N. (2011). Skyrmions: A Great Finishing Touch to Classical Newtonian Philosophy. New York, NY: Nova Science Publisher. Available at: https://www.researchgate.net/publication/287262893_Skyrmions_A_great_finishing_touch_to_classical_newtonian_philosophy.
Agop, M., and Merches, I. (2018). Operational Procedures Describing Physical Systems. CRC Press, Boca Raton, Florida.
Agop, M., and Paun, P. V. (2017). On the New Perspective of Fractal Theory. Fundaments and Applications; Editura Academiei Romane, București, Romania.
Alfonsi, G. (2009). Reynolds-averaged Navier–Stokes Equations for Turbulence Modeling, Appl. Mech. Rev., 62, 040802 (20 pages). doi:10.1115/1.3124648
Belegante, L., Bravo-Aranda, J. A., Freudenthaler, V., Nicolae, D., Nemuc, A., and Ene, D.(2018). Experimental Techniques for the Calibration of Lidar Depolarization Channels in EARLINET. Atmos. Meas. Tech., 11(2), 1119–1141. doi:10.5194/amt-11-1119-2018
Cristescu, C. P. (2008). Nonlinear Dynamics and Chaos. Theoretical Fundaments and Applications. Bucharest: Romanian Academy Publishing House, Bucharest, Romania.
Hamilton, M. J. (2017). Mathematical Gauge Theory. Springer International Publishing AG, New York US.
Jaynes, E. T. (1973). The Well-Posed Problem. Foundations Phys., 3(4), 477–493. doi:10.1007/bf00709116
Mandelbrot, B. B. (1982). The Fractal Geometry of Nature; W. H. Freeman: San Fracisco, CA, USA, pp. 80–103.
Mazilu, N., Agop, M., and Mercheş, I. (2019). The Mathematical Principles of Scale Relativity Physics: The Concept of Interpretation. CRC Press, Boca Raton, Florida.
McDonough, J. M., and Huang, M. T. (2004). A ‘poor Man's Navier–Stokes Equation’: Derivation and Numerical Experiments—The 2‐D Case. Int. J. Numer. Methods Fluids, 44(5), 545–578. doi:10.1002/fld.657
Merches, I., and Agop, M. (2015). Differentiability and Fractality in Dynamics of Physical Systems. World Scientific, Singapore.
Mihaileanu, N. (1972). Geometrie analitica, proiectiva si diferentiala. Complemente, Ed. Didactica si Pedagogica, Bucharest, 3.
Nicolae, D., Vasilescu, J., Talianu, C., Binietoglou, I., Nicolae, V., Andrei, S., et al. (2018). A Neural Network Aerosol-Typing Algorithm Based on Lidar Data. Atmos. Chem. Phys., 18(19), 14511–14537. doi:10.5194/acp-18-14511-2018
Nottale, L. (2011). Scale Relativity and Fractal Space-Time: A New Approach to Unifying Relativity and Quantum Mechanics. Imperial College Press: London, UK, pp. 403–425.
Ovchinnikov, I. V. (2016). Introduction to Supersymmetric Theory of Stochastics. Entropy, 18(4), 108.doi:10.3390/e18040108
Ovchinnikov, I. V., Schwartz, R. N., and Wang, K. L. (2016). Topological Supersymmetry Breaking: The Definition and Stochastic Generalization of Chaos and the Limit of Applicability of Statistics. Mod. Phys. Lett. B, 30(08), 1650086.doi:10.1142/s021798491650086x
Roşu, I. A., Cazacu, M. M., Ghenadi, A. S., Bibire, L., and Agop, M. (2020). On a Multifractal Approach of Turbulent Atmosphere Dynamics. Front. Earth Sci., 8, 216. doi:10.3389/feart.2020.00216
Roşu, I. A., Cazacu, M. M., and Agop, M. (2021a). Multifractal Model of Atmospheric Turbulence Applied to Elastic Lidar Data. Atmosphere, 12(2), 226. doi:10.3390/atmos12020226
Roşu, I. A., Nica, D. C., Cazacu, M. M., and Agop, M. (2021b). Towards Possible Laminar Channels through Turbulent Atmospheres in a Multifractal Paradigm. Atmosphere, 12(8), 1038. doi:10.3390/atmos12081038
Sors, L. A. S., and Santaló, L. A. (2004). Integral Geometry and Geometric Probability. Cambridge University Press, Cambridge, England.
Tatarski, V. I. (2016). Wave Propagation in a Turbulent Medium. Courier Dover Publications, Mineola, New York, US.
Keywords: self-structuring, turbulent (channel) flow, laminar channel flow, atmosphere, multifractal, LIDAR - remote sensing
Citation: Roșu I-A, Nica D-C, Cazacu MM and Agop M (2022) Cellular Self-Structuring and Turbulent Behaviors in Atmospheric Laminar Channels. Front. Earth Sci. 9:801020. doi: 10.3389/feart.2021.801020
Received: 24 October 2021; Accepted: 14 December 2021;
Published: 21 January 2022.
Edited by:
Iuliana Oprea, Colorado State University, United StatesReviewed by:
Eugen Radu, University of Aveiro, PortugalLeila Marek Crnjac, Technical School Centre Maribor, Slovenia
Copyright © 2022 Roșu, Nica, Cazacu and Agop. This is an open-access article distributed under the terms of the Creative Commons Attribution License (CC BY). The use, distribution or reproduction in other forums is permitted, provided the original author(s) and the copyright owner(s) are credited and that the original publication in this journal is cited, in accordance with accepted academic practice. No use, distribution or reproduction is permitted which does not comply with these terms.
*Correspondence: Dragos-Constantin Nica, dragos.nica@uaic.ro; Marius Mihai Cazacu, cazacumarius@gmail.com