Interacting municipal-level anthropogenic and ecological disturbances drive changes in Neotropical forest carbon storage
- 1Department of Biology, Faculty of Natural Sciences, Universidad del Rosario, Bogotá, DC, Colombia
- 2Centro Interdisciplinario de Estudios sobre el Desarrollo, Universidad de Los Andes (CIDER), Bogotá, Colombia
- 3Earth Observation Science, Applications and Climate Department, European Space Agency, Frascati, Italy
- 4Science, Applications and Climate Department, European Space Agency, Frascati, Italy
- 5Department of Geography and Environmental Studies, Stellenbosch University, Stellenbosch, South Africa
- 6Pacific Southwest Research Station, USDA Forest Service, Riverside, CA, United States
Deforestation is a documented driver of biodiversity loss and ecosystem services in the tropics. However, less is known on how interacting regional and local-level anthropogenic and ecological disturbances such as land use activities, human populations, and armed conflict affect carbon storage and emissions in Neotropical forests. Therefore, we explored how local-scale, socio-ecological drivers affect carbon dynamics across space and time in a region in Colombia characterized by deforestation, land use cover (LULC) changes, and armed conflict. Specifically, using available municipal level data from a period of armed conflict (2009–2012), spatiotemporal analyses, and multivariate models, we analyzed the effects of a suite of socio-ecological drivers (e.g., armed conflict, illicit crops, human population, agriculture, etc.) on deforestation and carbon storage-emission dynamics. We found that about 0.4% of the initial forest cover area was converted to other LULC types, particularly pastures and crops. Gross C storage emissions were 4.14 Mt C, while gross carbon sequestration was 1.43 Mt C; primarily due to forest regeneration. We found that livestock ranching, illegal crop cultivation, and rural population were significant drivers of deforestation and carbon storage changes, while the influential role of armed conflict was less clear. However, temporal dynamics affected the magnitude of LULC effects and deforestation on carbon storage and emissions. The approach and findings can be used to better inform medium to long-term local and regional planning and decision-making related to forest conservation and ecosystem service policies in Neotropical forests experiencing disturbances related to global change and socio-political events like armed conflict.
1 Introduction
Anthropogenic disturbances have a profound effect on the structure, composition, and function of global forested ecosystems (Machlis and Hanson, 2008; Perring et al., 2016). In the tropics, deforestation, or the conversion of forests to non-forested land use (LU)- land cover (LC), is a primary cause of biodiversity loss, and a disruption of several provisioning, cultural, and regulating ecosystem services, including carbon dioxide sequestration and emissions due to loss of carbon storage (Sanchez-Cuervo and Aide. 2013). Regionally, such loss of tropical forests and their regulating and cultural ecosystem services also have implicit trade-offs related to local scale provisioning ecosystem services, such as timber and non-timber forest products, crop yields, and cattle production (Murad and Pearse, 2018).
Large-scale tropical deforestation is often driven by LULC conversion agents, such as industrialized agriculture and livestock production activities (Landholm et al., 2019). The effects of ecological disturbance agents such as fire, drought, pests and diseases, and extreme events related to climate change have been well studied (Achard et al., 2014), as well as the dynamic between global and national socioeconomic and political drivers and Neotropical deforestation (González-González et al., 2021). However, tropical deforestation can also be driven by other cross-scale anthropogenic disturbance drivers such as land tenure regimes, inequity and poverty, poor governability, unbalanced power structures, illicit cropping, mining, and even warfare (Hoffmann, et al., 2018). This specific role of regional and local-level anthropogenic disturbance drivers in changing ecosystem functions and services in tropical regions has been less studied (Salazar, 2016; Bautista-Cespedes et al., 2021). Indeed, regional conservation efforts and local use and management of Neotropical forests are important, as they provide multiple types of regional-continental ecosystem services such as climate regulation (e.g., carbon storage and sequestration, temperature regulation; Gibbs et al., 2007) and water regulation and provisioning services for humans settlements (Clerici et al., 2019). They can also provide local-level provisioning services such as timber, non-timber forest products, crops and forage, and cultural ecosystem services such as wildlife viewing opportunities, ecotourism as well as spiritual and educational opportunities (Carriazo et al., 2019; Ocampo-Penuela and Winton 2017). Forest carbon storage is important since deforestation of tropical forests contributes to about 15%–25% of all annual global greenhouse gas emissions (Gibbs, et al., 2007; Phillips et al., 2016). Similarly, carbon storage, sequestration, and offset programs are a key component of many local-regional Payment for Ecosystem Service and REDD+ (Reducing Emissions from Deforestation and forest Degradation in developing countries) programs and instruments.
Although anthropogenic disturbances driving deforestation and carbon storage are complex and context specific, less studied socio-ecological factors, such as: demographics, population density changes and migration, economic activities, actor groups, and factors associated with socio-economic and political factors can provide key insights into these dynamics (Leite et al., 2018; Betancur-Alarcón and Krause, 2020). For example, a less studied anthropogenic disturbance affecting tropical forests and their ecosystem services in countries such as Colombia, Democratic Republic of the Congo (DRC), Sri Lanka, Indonesia, among others, is warfare or armed conflict. Armed conflict and its associated variables such as internal displacement, violence, armed encounters, and casualties is a multi-faceted phenomenon with complex socio-political, socio-economic, and ecological dynamics and effects (Camargo et al., 2020). Armed conflict incorporates the interaction of governments military, civilians, and the environment. Warfare dynamics imply socioecological changes, because warfare requires extraction of natural resources and movement of people, which have often impacts on natural ecosystems. Depending on the relationship among ecosystems and conflict dynamics, either deforestation or “gun-point conservation” of forests will imply changes within the landscape. Although conflict has recently been used with frequency to discuss its role in national and regional tropical deforestation, it can also interact with local-regional level factors driving ecosystem services such as: resource extraction, landscape fragmentation, habitat loss, soil erosion, and socio-economic disruption (Murillo-Sandoval et al., 2020; Bautista-Cespedes et al., 2021; Liévano-Latorre et al., 2021). For a more detailed discussion about conflict and warfare ecology-related concepts, please refer to Machlis and Hanson (2008). Also refer to Schoon and Cox (2012) for an in-depth discussion of socio-ecological disturbance typologies and frameworks for distinguishing between natural and anthropogenic disturbances (i.e., drivers as used in the ecosystem services literature).
As such, forests in tropical countries provide unique opportunities to explore the effects of complex anthropogenic disturbances on the regional and local supply and demand of ecosystem services. Using such disturbances as variables in statistical models can provide context-relevant information about deforestation as well as reforestation and regeneration dynamics (Sanchez-Cuervo and Aide, 2013). In particular, tropical forests that have or are experiencing armed conflict, several factors have been found to be correlated with deforestation: rural and urban population density, agricultural activity (cattle, agro-industrial products included), infrastructure, mining (legal and illegal), and illegal cropping (crops or plants which have been deemed illegal to grow by the government, e.g., coca bush or opium poppy) (Gaveau et al., 2009; Kanninen et al., 2009; Potapov et al., 2012; Yasmi et al., 2013; Butsic et al., 2015; Camisani 2018).
However, in-situ measurements and access to such forests is complex and often not possible; thus, remote sensing techniques based on satellite imagery and geospatial analyses are regularly used to measure and monitor tropical deforestation in these contexts (Achard et al., 2014; Murad and Pearse, 2018). Free and open access to satellite imagery (e.g., from Landsat and Sentinel), geospatial datasets (Turner et al., 2015), and recently available socio-economic, commodity production, and armed conflict data can allow for the study of the spatiotemporal dynamics related to these natural and anthropogenic disturbances influencing LULC change in tropical forests, and subsequent changes to ecosystem services (Suárez et al., 2018). We refer to the interaction of natural and anthropogenic disturbances hereafter as socio-ecological drivers (Schoon and Cox. 2012).
Accordingly, this study aims to better understand how local-level socio-ecological disturbances affect deforestation and subsequent carbon storage across space and time in a socio-ecologically complex region that experienced conflict in central Colombia. The country is characterized by historic and recent high rates of deforestation due to agriculture, livestock, mining, and armed conflict (Camargo et al., 2020; Prem et al., 2020). Thus, its socio-political and socio-economic dynamics provide a unique opportunity to explore the role of socio-ecological disturbances and drivers on Neotropical forest carbon storage during 2009–2012; a period of active internal armed conflict. Our specific study objectives are three-fold:
1. Spatio-temporally estimate the area of forest conversions to other land use-land covers,
2. Spatio-temporally analyze forest carbon storage changes related to land use-land cover transitions, and
3. Explore the effects of socio-ecological drivers on deforestation and subsequent forest carbon storage-emission dynamics.
In the below study we analyzed a number of direct and indirect drivers of forest and land cover change to better understand the role of municipal-level socio-ecological disturbances on Neotropical Forest carbon. Such approach and information are key to better informing local and regional policies and programs such as Reduced Emission from Deforestation and Degradation (REDD+) and Payments for ecosystem services (Gibbs et al., 2007). The approach, as detailed below, can also be used to assess the sustainable provisioning of agricultural products and promoting opportunities for cultural ecosystem services (Ocampo-Peñuela and Winton, 2017; Phillips et al., 2016).
2 Materials and methods
2.1 Study area
The study area encompasses 12 different municipalities in the Departments of Meta, Guaviare and Caquetá, in central Colombia (Figure 1). The three departments have a mean annual temperature range of 25–30°C and mean annual rainfall of approximately 2,500–3,000 mm (IDEAM, 2020). The main ecosystems in the Departments include fragmented humid forests, savannas, secondary vegetation, agroecosystems and wetlands (Suarez et al., 2018). Elevation ranges from approximately to 125–4,100 m (Eastern Andean Cordillera) above sea level. Several protected areas are present in the study area (Figure 1) and include: Natural National Parks Sierra de la Macarena, Picachos, Tinigua, Serrania de Chiribiquete, and Natural National Reserve Nukak. The main socio-economic activities of the region are related to agricultural production related to coffee cultivation and livestock production, and mining to a lesser extent (DANE, 2020; Castro-Nunez et al., 2017). However, other illicit activities such as coca cultivation, illegal timber harvesting, and illicit mining operation also influence economic activities and supply chains throughout the study area (Rodríguez-de-Francisco et al., 2021). This region is home to an important biological mega-corridor between the Andes and Amazon biogeographical region: the Amazon-Andes Transition Belt (Clerici et al., 2018).
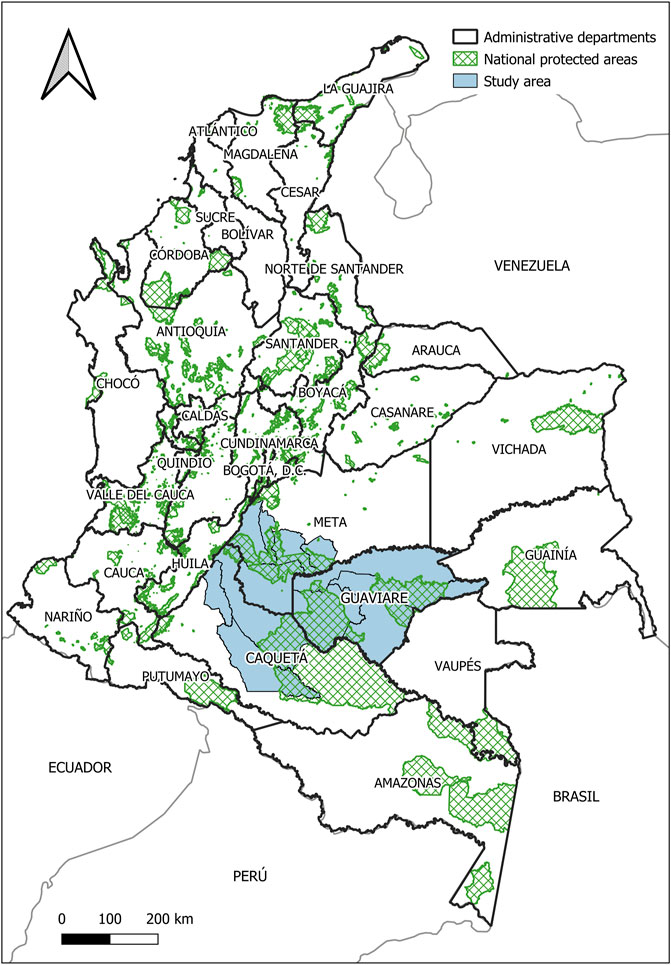
FIGURE 1. Study area (blue polygons) in the departments of Meta, Guaviare and Caquetá, Colombia. Protected areas are shown in green.
The total 2020 population in the study area’s three Departments is approximately: 411,000 in Caquetá; 86,000 in Guaviare, and more than one million people in Meta (Statista Research Department, 2021). The region has historically been characterized by armed conflict and the presence of several armed groups, including the Revolutionary Armed Forces of Colombia (FARC), National Liberation Army (ELN), paramilitaries and other groups associated with illicit crops cultivation and natural resource extraction (Rincón Ruiz et al., 2013; Bautista-Cespedes et al., 2021; Sanchez-Cuervo and Aide 2013). In this study we focus on the time interval 2009–2012, a period characterized by: 1) active and intense armed conflict (i.e., the 2009 Seguridad Democratica or Democratic Security period) that coincided with increased military spending and operations against the FARC, and 2) a transition period as of 2012 that was characterized by a lower intensity of armed conflict and a period that eventually led to a post-conflict warfare dynamics (i.e., the FARC Peace Process; Camargo et al., 2020).
2.2 Land use-land cover transitions
Specific Land use-Land cover transitions from 2009 to 2012 were estimated using the ESA Land Cover Climate Change Initiative Copernicus LC land cover products (Copernicus). The data are based on a 22 class LULC system, defined using the United Nation Food and Agriculture Organization’s (UN FAO) Land Cover Classification System (LCCS), and provides annual gridded LULC maps at 300 m resolution from 1992 to 2020. Detailed LULC class descriptions are discussed in Copernicus (2021b). The analyzed products for the years 2009 and 2012 are MERIS based global coverage at 300 m (https://earth.esa.int/eogateway/instruments/meris), generated by ESA from MERIS Full Resolution surface reflectance data. The Coordinate Reference System used for the global land cover database is a geographic coordinate system (GCS) based on the World Geodetic System 84 (WGS84) reference ellipsoid. To ensure the quality and consistency of the LC maps, the sets of annual maps were not produced independently, but they were derived from a unique baseline LC map, which was generated using the entire MERIS Full Resolution and MERIS Reduced Resolution (1,000 m) archive from 2003 to 2012, employing a machine learning spectral classification module (ESA, 2017a). The validation process was ensured through: 1) validation datasets that were not used during the production of the LC maps and 2) carried out by external parties, not involved in the production of the LC maps. The first step in a validation process was to estimate the accuracy of the latest year (2015) using an independent dataset and the process revealed that the overall accuracy of the 2015 LC map was 71.45% (ESA, 2017b). While there is no direct evaluation of the overall accuracy of the 2009–2012 products, it was reported in a second step, that there is a reported good agreement (>90%) for croplands, broadleaved evergreen forests, urban areas, bare areas, water bodies, and permanent snow and ice cover with the previous yearly products compared to the 2015 LULC map.
The 17 Copernicus LULC classes present in the study area were aggregated into six general classes (Table 1), that were subsequently used to analyzed forest to other LULC transitions during 2009–2012; (i.e., forest cover was the starting or target class; Szantoi et al., 2021).
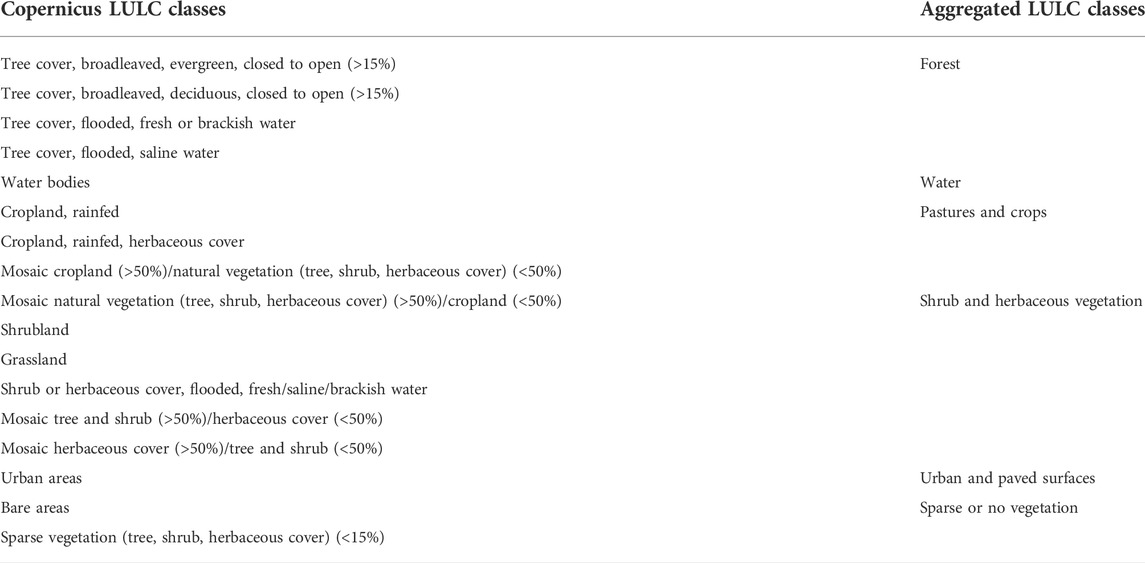
TABLE 1. ESA LC CCI land use and land cover classes (LULC; left column) and aggregated LULC classes in this work (right column).
2.3 Forest carbon storage changes
Mean aboveground gross carbon (C) storage densities (Mg C/ha) were compiled for the various LULC classes based on context-relevant information from the region (Table 2). These carbon storage densities were used to estimate the carbon storage per LULC class per area for the analysis period and carbon storage changes from the transitions from 2009 to 2012. These changes in gross carbon storage were converted to a per year basis, or annualized, during the 3-year period and are reported as carbon sequestration in the case of annual carbon storage increases, or conversely carbon emissions in the case of annual carbon storage losses.
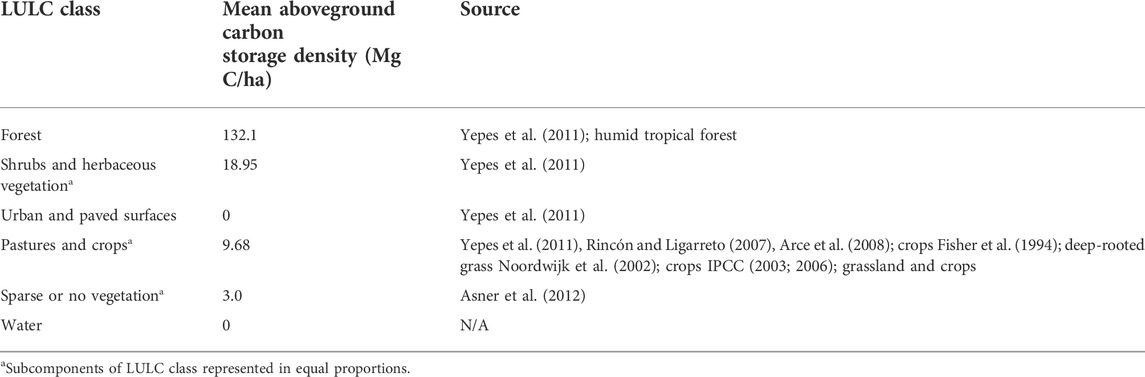
TABLE 2. Land use-Land Cover (LULC) classes and reported mean aboveground carbon storage densities used in this study, with bibliographic source.
2.4 Socio-ecological drivers of forest carbon emissions
We analyzed available and relevant socio-ecological drivers associated with deforestation across 12 municipalities (Supplementary Table S1) from Table 3. The data were at the municipal-level to better understand their role as regional-local scale drivers of forest loss and subsequent carbon storage changes (i.e., emissions) in the study area during the study period. We first used an exploratory analysis to identify spatiotemporal dynamics and patterns in the data, as well as data quality issues (Behrens, 1997). Accordingly, we used Pearson correlation analyses and Stepwise Regression to reduce errors associated with redundant variables, identify statistically significant variables, and to address multi-collinearity issues (Harrell, 2013).
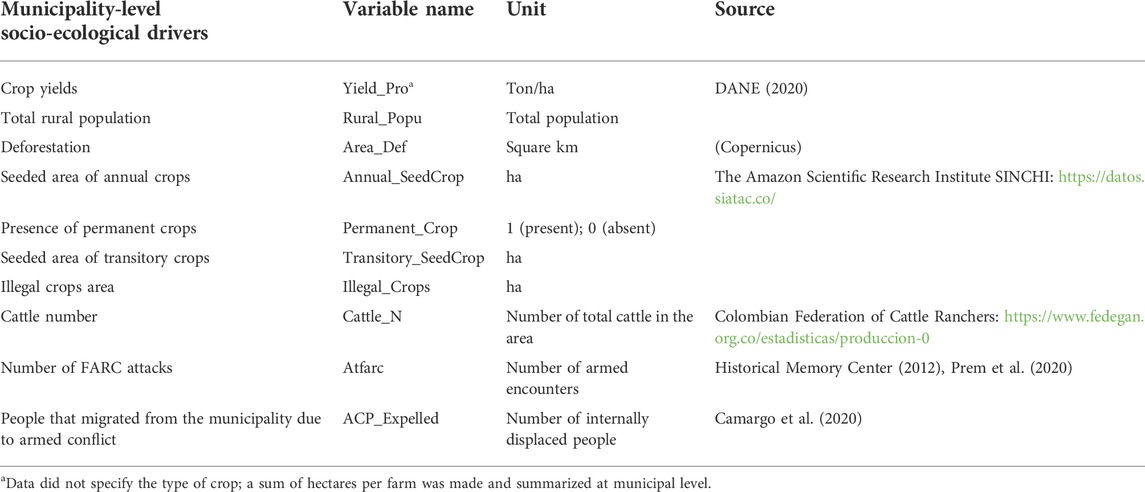
TABLE 3. Municipal-level socio-ecological drivers (i.e., anthropogenic and ecological disturbances) affecting deforestation and carbon storage change in Neotropical forests in central Colombia.
The selected variables were also tested for spatial autocorrelation using Moran’s I index (Celemín, 2009; Durán and Monsalves, 2020) and a spatial weighted matrix with a first-order queen contiguity matrix to evaluate variable pattern distribution in each municipality (Carracedo and Debón, 2017). To identify spatial changes in our variables we also used a Spatial Delay Analysis with a weighted average of random variables in neighboring locations (Pérez Pineda, 2006). These tests found no spatial auto-correlation issues.
Accordingly, these drivers in Table 3 were then used to statistically analyze the effect of municipal-level socio-ecological drivers on forest loss and its relationship with carbon storage during 2009–2012. First, we developed a mean comparison or variance model (Model 1, hereafter) to account for continuous and categorical data and their independence (Villa et al., 2012). Here we used a factorial design that considers a single factor, i.e., the dependent variable (deforestation) and the levels reported in Table 3 (Palmer Po, 2019). We tested for two different temporal groups, “A” for 2009 and “B” for 2012, respectively, and used the test levels a and b (a, b ≥ 2), with a factorial arrangement or design of a x b treatments, or:
where
To better understand the role of year (i.e., time) and a municipality’s characteristics on deforestation, we also used a second analysis (Model 2, hereafter) of the form:
where Are_def is the deforested area and x varies for each of the 12 municipalities (Appendix A). Model 2 was based on a factorial multivariate analysis of variance (MANOVA) model to account for the temporal nature of deforestation across different years (Warne, 2014). We evaluated a null hypothesis in which the value of the mean in the dependent variable can be statistically dependent on each group of independent variables. We used four conventional statistics to test for variance means in the multivariate model, specifically: Pillai’s Trace, Wilk’s Lambda, Hotelling’s Trace, and Roy’s Largest Root (Chen, 2011). All significance tests were obtained with a fixed value of p = 0.05. All statistical analyses were performed using R v. 3.6 (R Core Team, 2017).
A resuming flow-chart of all processing steps is reported in Supplementary Figure S1.
3 Results
3.1 Land use land cover change
Land use and land cover transitions (2009–2012) derived from the Copernicus dataset (Copernicus, 2021a) are shown in Figure 2. The area extent of the LULC transitions involving forests in the study area are shown in Table 4. The main LULC transition is represented by the conversion of Forests to Pastures and Crops (23,227 ha), while the second largest transition is represented by Forest conversion to Shrubs and Herbaceous Vegetation (11,413 ha); the latter indicating a process of deforestation followed by regeneration, and/or forest degradation. A total of 11,918 ha represents areas where forest regeneration has occurred as shown by the areas of pastures, crops, shrubs, and herbaceous LULCs converting to secondary forests (Table 4).
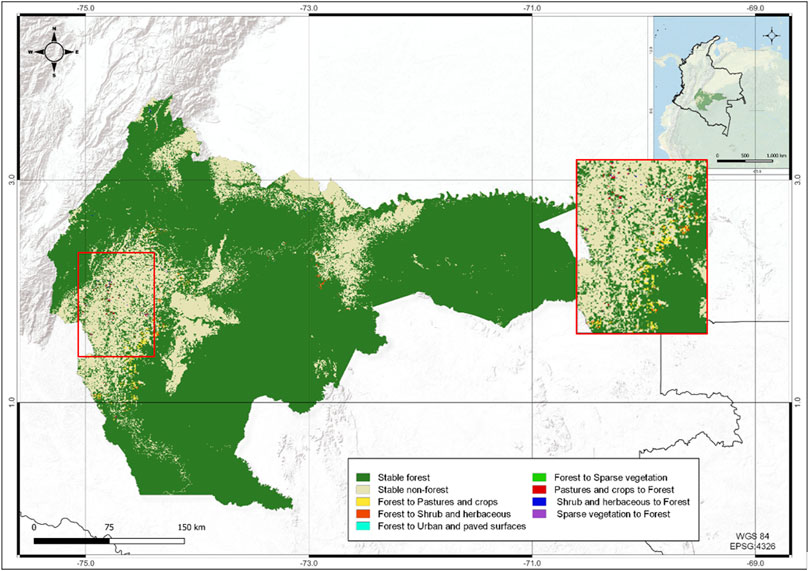
FIGURE 2. Land use land cover transitions in the study area in 2009–2012. Red frame: a zoom western of Sabanas del Yarí region in central Colombia.
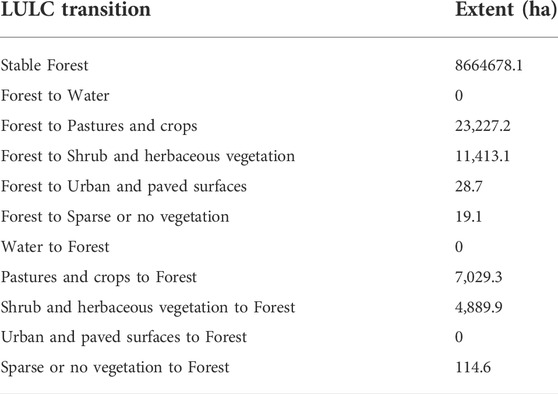
TABLE 4. Land use and land cover (LULC) transitions type and extent (ha) in the central Colombian study area during 2009–2012.
3.2 Forest carbon storage and emission dynamics
Overall, gross carbon emissions in the study period were 4.14 Mt C (average annual C emissions were 1.38 Mt C yr−1), and gross carbon sequestration 1.43 Mt C (average annual C sequestration was 0.48 Mt C yr−1). The non-annualized difference between gross carbon storage and carbon emission was estimated at 2.71 Mt C in the study area during 2009–2012. Figures 3, 4 show municipal-level forest C storage changes (i.e., emissions) for targeted LULC transitions, respectively. Forest carbon emissions and gross carbon storage estimations between 2009 and 2012 are shown in Table 5 and were calculated using the carbon density values presented in Table 2. Table 5 shows that carbon storage increases are overall, lower than carbon emissions. Municipalities with the areas of greatest carbon storage occurred in the municipalities of San Vicente del Caguán, San José del Guaviare, La Macarena, and Cartagena del Chairá (Figure 4. We note that these estimates were not analyzed as compositional data). The highest carbon emissions occurred in Forests that were converted to pasture and crop areas in the municipalities of San Vicente del Caguán, Cartagena de Chairá and La Macarena (Figure 3). The municipalities of Mesetas and Vistahermosa had the lowest values of carbon emissions and storage, respectively.
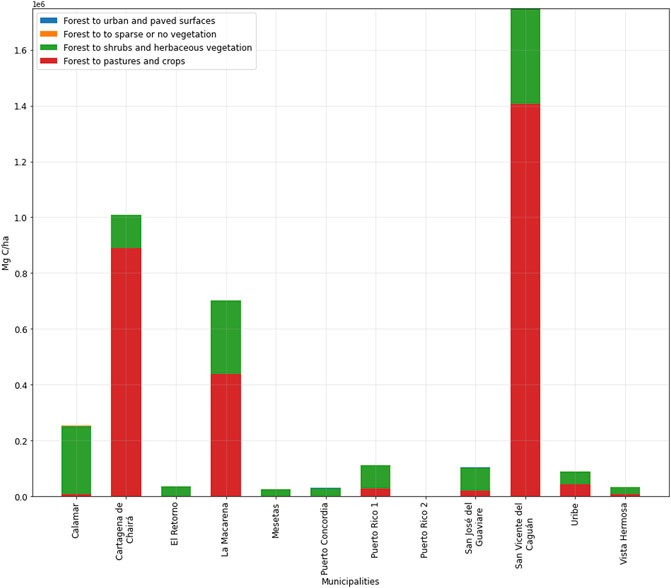
FIGURE 3. Forest carbon emissions (Mg C * 106) by municipality and land use land cover transitions in the study area during 2009–2012.
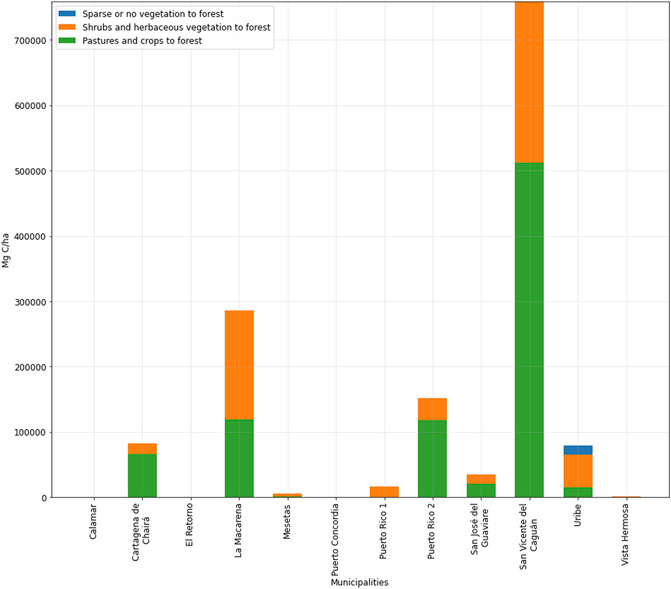
FIGURE 4. Carbon storage due to forest regeneration and/or degradation (Mg C) by municipality and land use and land cover transition in the study area during 2009–2012.
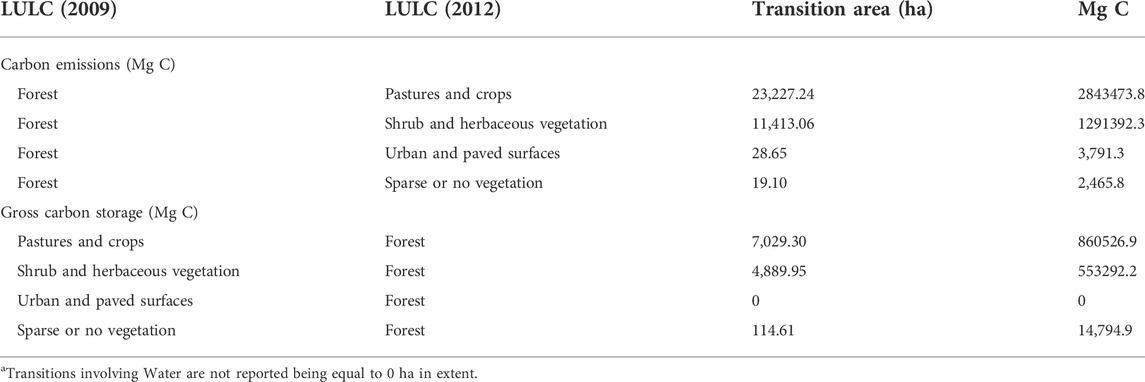
TABLE 5. Forest gross carbon emissions and gross carbon storage between 2009 and 2012 in the study area.
3.3 Socio-ecological drivers of forest carbon storage changes
Our factorial design for Model 1 was used to account for the relationships or interactions among the anthropogenic and ecological disturbances in the study area during the analysis period. Accordingly, these socio-ecological drivers comprising the model (Table 3), were analyzed to determine the significance of temporal changes among them (Oehlert, 2010). Again, Model 2 in turn was used to understand the temporal influence of these socio-ecological drivers on deforestation and carbon storage changes. We note that Model 2 is a multivariate model with two dependent variables that avoids the use of “year” as a dependent variable; as used in Model 1 (See Section 3.3.1 below).
3.3.1 Statistical analysis
Model 1 was significant (0.019, <p = 0.05), and can explain some of the variation in deforestation observed in the study area. We found that some variables such as illegal crops during both 2009 and 2012 groups were significant. Accordingly, we used both these groups/years as a fixed factor in the model and adopted an H0 hypothesis where the means are the same. All the variables related to this hypothesis were tested (Table 3) and all were found not be not significant; therefore, the null hypothesis was rejected. We found that permanent crops (variable Permanent_C) was redundant within the model and thus not explanatory. Overall, the model factors explained 86.5% of the variance in deforestation (Area_Def). The limits and significance of the estimators in the variables that have Year as a factor are shown in Table 6. Results show that “Year” when used as a factor was not significant. However illicit crops and number of cattle were found to be significant (p = 0.05) and rural population was somewhat significant (p = 0.07). The results showing the effects of the model with the factor (Year) and the interaction and intersection for each factor are shown in Table 7.
Using results from Tables 6, 7, an equation was developed to better understand the interactions among the different socio-ecological drivers of deforestation (Area_Def); the variables are explained in Table 8.
Although results (Tables 5, 6) showed a significant relationship between deforestation and year, cattle numbers (p < 0.05) and illegal crops (p < 0.05) as well as moderately significant with rural population (p < 0.07); interestingly year 2009 showed no statistical significance. To better understand how these interacting variables over time (i.e., year) influenced deforestation, we used multivariate model 2 with municipalities as the fixed effect and removing the variable Permanent C. This corrected model resulted in an acceptable level of significance (adjusted model of 95%) and explained the two dependent variables, deforestation and year, and their spatiotemporal relationship with several drivers. We found that Year had a negative adjusted R2 (−1.09; R2 = 0.714) while Deforestation had a positive Adjusted R2 (0.954; R2 = 0.994); indicating that deforestation was not a significant driver with respect to time. However, deforestation was significantly related to other variables such as illegal crops and specific municipal-level dynamics (Table 7).
4 Discussion
This study used available geospatial and socio-ecological data to analyze deforestation, LULC changes, and drivers influencing forest C storage dynamics in a region that experienced armed conflict in central Colombia. We found that forest cover loss (i.e., deforestation) was primarily a function of forest conversion to pastures-crops in our study area. This result was likely influenced by the governmental policies and programs related to the promotion of the agricultural production and livestock ranching between 2009-2010 (Suarez et al., 2018; Bautista-Cespedes et al., 2021). Our findings also indicate that some of the socio-ecological drivers behind deforestation and carbon storage changes were related to the amount of rural population, livestock ranching activity, and illicit crop cultivation, however the presence of armed groups such as the FARC was less clear (Camargo et al., 2020; Bautista-Cespedes et al., 2021).
More specifically, our LULC change analyses shows an increase in agriculture and pastures and conversely a decrease in forest cover. We found that almost 347 km2 of forests, about 0.4% of the initial forest cover extension, were converted to other LULC types between 2009 and 2012 (Table 4). Deforestation in the study region is known to be linked to both “land grabbing” for illegal ranching and land speculation for expected future increases in land value (Rodríguez-de-Francisco et al., 2021). The use of this area by the armed groups is also well known, as these groups strategically used forested areas during the period, for both illicit cultivation of coca and military-related operation operations (Camargo et al., 2020; Castro-Nunez et al., 2017). Previous studies have found that the effect of armed conflict on deforestation in Colombia is complex and varies across regions and time, but in general most literature agrees that deforestation might increase after the cessation of intense armed conflict (Murillo-Sandoval et al., 2021; Reuveny et al., 2010; Clerici et al., 2020; Baker et al., 2003). During 2012, initial discussions that led to the peace agreement with FARC have been reported as another potential factor that could have influenced deforestation and conversion to extensive uncontrolled farming and mining operations. as well as the movements of armed groups (Yu. et al., 2014; Bautista-Cespedes et al., 2021; Salazar, 2016). However, as we will later discuss, there is limited literature regarding how armed conflict directly and indirectly affects ecosystem function and services, particularly carbon storage and climate regulation.
Studies suggest that regions similar to our study area have experienced lower deforestation rates during conflict as compared to post-conflict periods (Castro-Nunez et al., 2017). After the cease-fire period in 2015 many of these forests experienced high rates of deforestation and conversion to land uses related to cattle farming, mining, and licit/illicit cropping (Bautista-Cespedes et al., 2021). Increase in deforestation during post-conflict was facilitated by easier access to pasture areas for cattle and crop farming due to the exit of FARC, and the return of internally displaced peoples (Negret, et al., 2019; Landholm et al., 2019). Nevertheless, studies like Bautista-Cespedes et al. (2021) and Salas-Salazar (2016) document that deforestation dynamics within each municipality can be different due to power-influence dynamics or warfare dynamics as well as the specific history of the rural areas during and after the armed conflict. Castro-Nunez et al. (2017) reports that during Colombia’s period of actively armed conflict, there were increases in certain LU activities that resulted in agricultural colonization practices that promote forest loss and thus increased carbon emissions. With respect to protected areas, Liévano-Latorre et al. (2021) found that only areas administered by Colombian natural parks were effective in avoiding deforestation (2000–2017), but that the presence of FARC increased deforestation at the regional level (Liévano-Latorre et al., 2021).
We found that there were specific socio-ecological drivers in addition to armed conflict driving C storage changes. The municipalities in the study area experienced several complex socio-political processes during our analysis period related to unregulated cattle ranching and both legal and illegal mining (UNODC and Ministerio de Justicia y del Derecho, 2015). Such activities have also been associated with deforestation activities and also contribute to explain the increase in carbon emissions (Table 5). Coca production has frequently been linked to deforestation in humid tropical forested ecosystems in Colombia (Rincón-Ruiz and Kallis et al., 2013), however, most of the carbon emissions rates in San Vicente del Caguán can also be related to agroforestry practices such as rubber and cocoa production, infrastructure development (González et al., 2018). In municipalities such as San José del Guaviare and La Macarena, agricultural practices could involve extensive illicit livestock and crop operations. But in other municipalities such as Puerto Rico (Meta), Vistahermosa, Uribe and Mesetas, few changes were observed in regard to forest carbon storage losses (Figure 3). These trends are likely a result of historic ranching and coca crop cultivation activities in recent history and thus no prominent changes in carbon storage losses were found in our study for these municipalities (UNODC and Ministerio de Justicia y del Derecho, 2015).
Our modelling approach accounted for the different and interacting municipal-level socio-ecological drivers affecting deforestation across space and time in the study area. By accounting for these temporal disturbances in Model 2 we also obtained a much higher fit relative to Model 1 (Table 7). The drivers identified by both our statistical models show that many of them are likely interacting (Table 7). Such interacting activities that affect deforestation can include illicit crop cultivation, ranching, internally displaced people, and armed conflict (Sanchez-Cuervo and Aide. 2013; Rincón Ruiz et al., 2013). Such extractive natural resource activities are primarily found in deforested areas in less mountainous and more accessible areas (Sanchez-Cuervo and Aide. 2013; Bautista-Cespedes et al., 2021). Accordingly, our results are in-line with other studies (e.g., González-González et al., 2021; Ganzenmüller et al., 2022) in that different variables drive deforestation differently across space and time. In terms of ecosystem service provision, Castro-Nunez et al. (2017) investigated the spatial associations between carbon in woody biomass and conflict-related variables in Colombia’s Amazon region and found an inverse relation between carbon and both armed actions and conflict victims in the Amazon region.
However, we do note some limitations in our study, First, we only used available geospatial data, with a spatial resolution of 300 m × 300 m, without field verification. Second, our armed conflict variable was limited to presence or absence (binary data) of FARC-related armed groups in each municipality. The use of continuous data for armed conflict might have yielded new results and insights. Future research should account for additional anthropogenic and ecological disturbances to better analyse deforestation, such as mining, wood extraction (forest degradation), expansion of infrastructure, and other conflict-related drivers such as type of armed groups, and casualties.
This study’s findings shows that using socio-ecological data as proxies for disturbance agents—and their influence on deforestation and carbon storage—can be a viable approach for parsing out socio-political and economically relevant drivers, such as illicit crops, FARC attacks, and local-level land use activities affecting ecosystem service provision. Such dynamics can also depend on issues not addressed in this study, such as those related to governance and associated policies, laws, and events such as the fumigation of illicit crops or even the recent peace processes. Our findings and those from the literature demonstrate in general the need for improved governability as well as governance in remote rural locations in places such as this region in Colombia.
In conclusion, the approach and findings of this study can be used to better inform local-level decision-making related to forest conservation and ecosystem service provision policies and programs in Neotropical forests. Policies and legislation incentivizing the use of conservation instruments such as Payment for Ecosystem Services, for example, are well established in places such as Colombia. Thus, use of available, municipal-level data as used in this study can also be used to monitor and evaluate how socio-economic, political, and ecological indicators can drive not only the sustainable provisioning and regulating ecosystem services, but also opportunities for enhancing cultural ecosystem services in areas that lack data and access to on-site measurements.
Data availability statement
The datasets used in this study can be found in public online repositories; the source of these can be found in the article reference section. Access to the data produced in this research work will be made available upon request to the corresponding author.
Author contributions
FE, NC, and ZS developed the original research proposal; GT, MO, AG-G, and NC performed the calculations. GT and MO wrote the original draft; all authors contributed to the final version of the manuscript and agreed to its submission.
Funding
This research was founded by Universidad del Rosario, Small Grant 2017 “Trayectorias socioeconómicas, ecológicas y políticas de la deforestación en bosques tropicales en transición de postconflicto: lecciones para Colombia”.
Acknowledgments
We acknowledge the Dirección de Investigación e Innovación of Universidad del Rosario for granting the funds and for administrative support.
Conflict of interest
The authors declare that the research was conducted in the absence of any commercial or financial relationships that could be construed as a potential conflict of interest.
Publisher’s note
All claims expressed in this article are solely those of the authors and do not necessarily represent those of their affiliated organizations, or those of the publisher, the editors and the reviewers. Any product that may be evaluated in this article, or claim that may be made by its manufacturer, is not guaranteed or endorsed by the publisher.
Supplementary material
The Supplementary Material for this article can be found online at: https://www.frontiersin.org/articles/10.3389/fenvs.2022.937147/full#supplementary-material
References
Achard, F., Beuchle, R., Mayaux, P., Stibig, H., Bodart, C., Brink, A., et al. (2014). Determination of tropical deforestation rates and related carbon losses from 1990 to 2010. Glob. Change Biol. 20 (8), 2540–2554. doi:10.1111/gcb.12605
Arce, N., Ortiz, E., Villalobos, M., and Cordero, S. (2008). Existencias de carbono en charrales y sistemas agroforestales de cacao y banano de fincas indígenas bribri y cabécar de Talamanca. Costa Rica. Agroforestería las Américas 46, 30–33.
Asner, G. P., Clark, J. K., Mascaro, J., Galindo García, G. A., Chadwick, K. D., Navarrete Encinales, D. A., et al. (2012). High-resolution mapping of forest carbon stocks in the Colombian Amazon. Biogeosciences 9 (7), 2683–2696. doi:10.5194/bg-9-2683-2012
Baker, M., Clausen, R., Kanaan, R., N’Goma, M., Roule, T., and Thomson, J. (2003). Conflict timber: Dimensions of the problem in Asia and Africa, Volume III, African cases. Final report submitted to the United States agency for international development (Burlington, VT, United States: ARD)
Bautista-Cespedes, O. V., Willemen, L., Castro-Nunez, A., and Groen, T. A. (2021). The effects of armed conflict on forest cover changes across temporal and spatial scales in the Colombian Amazon. Reg. Environ. Change 22 (3), 70. doi:10.1007/s10113-021-01770-6
Behrens, J. T. (1997). Principles and procedures of exploratory data analysis. Psychol. Methods 2 (2), 131–160. doi:10.1037/1082-989x.2.2.131
Betancur-Alarcón, L., and Krause, T. (2020). Reaching for the mountains at the end of a rebelocracy: Changes in land and water access in Colombia’s highlands during the post-peace agreement phase. Front. Environ. Sci. 8 (2), 29. doi:10.3389/fenvs.2020.546821
Butsic, V., Baumann, M., Shortland, A., Walker, S., and Kuemmerle, T. (2015). Conservation and conflict in the Democratic Republic of Congo: The impacts of warfare, mining, and protected areas on deforestation. Biol. Conserv. 191 (191), 266–273. doi:10.1016/j.biocon.2015.06.037
Camargo, G., Sampayo, A. M., Peña Galindo, A., Escobedo, F. J., Carriazo, F., and Feged-Rivadeneira, A. (2020). “Exploring the dynamics of migration, armed conflict, urbanization, and anthropogenic change in Colombia,”. Editor B. Xue, 15, e0242266. doi:10.1371/journal.pone.0242266PLOS ONE11
Camisani, P. B. (2018). Sri Lanka: A political ecology of socio-environmental conflicts and development projects. Sustain. Sci. 13 (3), 693–707. doi:10.1007/s11625-018-0544-7
Carracedo, P., and Debon, A. (2017). Seleccion de modelos espacio-temporales con datos de panel en Matlab y R. Rect@. 18 (1), 93–118. doi:10.24309/recta.2017.18.2.01
Carriazo, F., Labarta, R., and Escobedo, F. J. (2019). Incentivizing sustainable rangeland practices and policies in Colombia’s Orinoco region. Land use policy 95, 104203. doi:10.3390/land10020108
Castro-Nunez, A., Mertz, O., and Sosa, C. C. (2017). Geographic overlaps between priority areas for forest carbon-storage efforts and those for delivering peacebuilding programs: Implications for policy design. Environ. Res. Lett. 12 (5), 054014. doi:10.1088/1748-9326/aa6f20
Celemín, J. P. (2009). Autocorrelación espacial e indicadores locales de asociación espacial Importancia. estructura y aplicación [Internet]. Available at: https://www.redalyc.org/pdf/3832/383239099001.pdf.
Chen, C-Y. (2011). Statistical and dynamical analyses of propagation mechanisms of solitary internal waves in a two-layer stratification. J. Mar. Sci. Technol. 16 (1), 100–114. doi:10.1007/s00773-010-0112-z
Clerici, N., Armenteras, D., Kareiva, P., Botero, R., Ramírez-Delgado, J. P., Forero-Medina, G., et al. (2020). Deforestation in Colombian protected areas increased during post-conflict periods. Sci. Rep. 10 (1), 4971. doi:10.1038/s41598-020-61861-y
Clerici, N., Cote-Navarro, F., Escobedo, F. J., Rubiano, K., and Villegas, J. C. (2019). Spatio-temporal and cumulative effects of land use-land cover and climate change on two ecosystem services in the Colombian Andes. Sci. Total Environ. 685 (12594), 1181–1192. doi:10.1016/j.scitotenv.2019.06.275
Clerici, N., Salazar, C., Pardo-Díaz, C., Jiggins, C. D., Richardson, J. E., and Linares, M. (2018). Peace in Colombia is a critical moment for Neotropical connectivity and conservation: Save the northern Andes-Amazon biodiversity bridge. Conserv. Lett. 12, e12594. doi:10.1111/conl.12594
Copernicus Copernicus climate change service tutorial CDR land cover (brokered from CCI land cover) [internet]. 2021b. Available at: https://datastore.copernicus-climate.eu/documents/satellite-land-cover/D4.3.3-Tutorial_CDR_LC-CCI_v2.0.7cds_PRODUCTS_v1.0.1.pdf.
Copernicus Copernicus climate data store | Copernicus climate data store [internet]. cds.climate.copernicus.eu 2021a. Available at: https://cds.climate.copernicus.eu/cdsapp#!/dataset/satellite-land-cover?tab=overview.
DANE - Departamento Administrativo Nacional de Estadística (2020). Geoportal DANE - marco geoestadístico nacional (MGN). Accessed at: https://www.dane.gov.co/index.php/estadisticas-por-tema/cuentas-nacionales/cuentas-nacionales-departamentales.
DANE - Departamento Administrativo Nacional de Estadística (2017). Geoportal DANE ANDA. Access to anonymous metadata and microdata. Accessed at: https://sitios.dane.gov.co/anda-index/.
Durán, D., and Monsalves, M. J. (2020). Spatial autocorrelation of breast cancer mortality in the metropolitan region, Chile: An ecological study. Medwave 20 (01), e7766. doi:10.5867/medwave.2020.01.7766
IPCC- Intergovernmental Panel on Climate Change (2006). in IPCC guidelines for national greenhouse gas inventories. Prepared by the national greenhouse gas inventories programme. Published by. Editors H. S. Eggleston, L. Buendia, K. Miwa, and T. y. Tanabe K. Ngara (Hayama (Japan): IGES). Available at: https://www.ipcc-nggip.iges.or.jp/public/gpglulucf/gpglulucf_files/GPG_LULUCF_FULL.pdf
ESA (2017a). Land cover CCI algorithm theoretical basis document. Part III: Classification. Available at: https://www.esa-landcover-cci.org/?q=webfm_send/139.
ESA (2017b). Land cover CCI product user guide. Available at: https://www.esa-landcover-cci.org/?q=webfm_send/112.
Fisher, M. J., Rao, I. M., Ayarza, M. A., Lascano, C. E., Sanz, J. I., Thomas, R. J., et al. (1994). Carbon storage by introduced deep-rooted grasses in the South American savannas. Nature 371, 236. Available at: https://www.nature.com/articles/371236a0.
Ganzenmüller, R., Sylvester, J. M., and Castro-Nunez, A. (2022). What peace means for deforestation: An analysis of local deforestation dynamics in times of conflict and peace in Colombia. Front. Environ. Sci. 10 (1), 21. doi:10.3389/fenvs.2022.803368
Gaveau, D. L. A., Wich, S., Epting, J., Juhn, D., Kanninen, M., and Leader-Williams, N. (2009). The future of forests and orangutans (Pongo abelii) in sumatra: Predicting impacts of oil palm plantations, road construction, and mechanisms for reducing carbon emissions from deforestation. Environ. Res. Lett. 4 (3), 034013. doi:10.1088/1748-9326/4/3/034013
Gibbs, H. K., Brown, S., Niles, J. O., and Foley, J. A. (2007). Monitoring and estimating tropical forest carbon stocks: Making REDD a reality. Environ. Res. Lett. 2 (4), 045023. doi:10.1088/1748-9326/2/4/045023
González, J., Cubillos, A., Chadid, M., Cubillos, A., Arias, M., Zúñiga, E., et al. (2018). Caracterización de las principales causas y agentes de la deforestación a nivel nacional período 2005-2015. Bogotá: Instituto de Hidrología. Meteorología y Estudios Ambientales – IDEAM-. Ministerio de Ambiente y Desarrollo Sostenible. Programa ONU-REDD Colombia.
González-González, A., Villegas, J. C., Clerici, N., and Salazar, J. F. (2021). Spatial-temporal dynamics of deforestation and its drivers indicate need for locally-adapted environmental governance in Colombia. Ecol. Indic. 126, 107695. doi:10.1016/j.ecolind.2021.107695
Harrell, F. E. (2013). Regression modeling strategies: With applications to linear models, logistic regression, and survival analysis. New York: Springer-Verlag.
Hoffmann, C., García Márquez, J. R., and Krueger, T. (2018). A local perspective on drivers and measures to slow deforestation in the Andean-Amazonian foothills of Colombia. Land Use Policy 77, 379. Available at: https://www.sciencedirect.com/science/article/pii/S0264837717304179.
IDEAM - Instituto de Hidrología Meteorología y Estudios Ambientales (2020). Atlas climatológico. Available at: http://atlas.ideam.gov.co/visorAtlasClimatologico.html (Accessed May 27, 2021).
IDEAM-Instituto de Hidrología Meteorología y estudios ambientales instituto alexander von Humboldt. (I.Humboldt). Instituto Geográfico Agustín Codazzi (IGAC). Instituto de Investigaciones Marinas y Costeras “José Benito Vives de Andréis” (Invemar) y Ministerio de Ambiente y Desarrollo Sostenible. (2017). Mapa de Ecosistemas Continentales. Costeros y Marinos de Colombia (MEC) [map]. Versión 2.1. escala 1:100.000. URL:Available at: http://www.ideam.gov.co/web/ecosistemas/mapa-ecosistemas-continentales-costeros-marinos.
IPCC-Intergovernmental Panel on Climate Change (2003). Good practice guidance for land use, land-use change and forestry. IPCC National Greenhouse Gas Inventories Programme, Institute for Global Environmental Strategies IGES, 610.
Kanninen, M., Juhn, D., Leader-Williams, N., Epting, J., Gaveau, D. L. A., Wich, S., et al. (2009). The future of forests and orangutans (Pongo abelii) in sumatra: Predicting impacts of oil palm plantations. Road construction. And mechanisms for reducing carbon emissions from deforestation. Environ. Res. Lett. 4 (3), 034013. doi:10.1088/1748-9326/4/3/034013
Landholm, D. M., Pradhan, P., and Kropp, J. P. (2019). Diverging forest land use dynamics induced by armed conflict across the tropics. Glob. Environ. Change 56, 86–94. doi:10.1016/j.gloenvcha.2019.03.006
Leal Martínez, D. L,, E(13)ique Caballero, N. C., and Herrera Acosta, R. (2014). El derecho de las víctimas del conflicto armado frente a la restitución de tierras en Colombia. criterio juridic. garantista 5 (10), 3. doi:10.26564/21453381.433
Leite, A., Cáceres, A., Melo, M., Mills, M. S. L., and Monteiro, A. T. (2018). Reducing emissions from Deforestation and forest Degradation in Angola: Insights from the scarp forest conservation “hotspot. Land Degrad. Dev. 29 (12), 4291–4300. doi:10.1002/ldr.3178
Liévano-Latorre, L. F., Brum, F. T., and Loyola, R. (2021). How effective have been guerrilla occupation and protected areas in avoiding deforestation in Colombia? Biol. Conserv. 253, 108916. doi:10.1016/j.biocon.2020.108916
Machlis, G. E., and Hanson, T. (2008). Warfare ecology. BioScience 58 (8), 729–736. doi:10.1641/b580809
Memory Center, Historical (2012). Bases de datos - ¡Basta ya! Colombia: Memorias de guerra y dignidad. [Internet]. Bases de datos - ¡Basta ya! Colombia: Memorias de guerra y dignidad. Available at: http://www.centrodememoriahistorica.gov.co/micrositios/informeGeneral/basesDatos.html.
Murad, C. A., and Pearse, J. (2018). Landsat study of deforestation in the Amazon region of Colombia: Departments of Caquetá and putumayo. Remote sensing applications. Soc. Environ. 11, 16. doi:10.26564/21453381.433
Murillo-Sandoval, P. J., Van Dexter, K., Van Den Hoek, J., Wrathall, D., and Kennedy, R. (2020). The end of gunpoint conservation: Forest disturbance after the Colombian peace agreement. Environ. Res. Lett. 15 (3), 034033. doi:10.1088/1748-9326/ab6ae3
Negret, P. J., Sonter, L., Watson, J. E. M., Possingham, H. P., Jones, K. R., Suarez, C., et al. (2019). Emerging evidence that armed conflict and coca cultivation influence deforestation patterns. Biol. Conserv. 239, 108176. doi:10.1016/j.biocon.2019.07.021
Noordwijk, M., Rahayu, S., Hairiah, K., Wulan, Y. C., Farida, A., and Verbist, B. (2002). Carbon stock assessment for a forest-to-coffee conversion landscape in sumber-jaya (lampung. Indonesia): From allometric equations to land use change analysis. Sci. China Ser. C Life Sci. 45, 75–86.
Observatorio de Drogas en Colombia.| Centro de Conocimiento [internet] . Available at: http://www.odc.gov.co
Ocampo-Peñuela, N., and Winton, R. S. (2017). Economic and conservation potential of bird-watching tourism in postconflict Colombia. Trop. Conservation Sci. 10, 194008291773386. doi:10.1177/1940082917733862
Oehlert, G. A. (2010). First course in design and analysis of experiments. Available at: http://users.stat.umn.edu/∼gary/book/fcdae.pdf.
Palmer Pol, A. L. (2019). Estadística Aplicada con R 11. Análisis de Diseños Factoriales de Bloqueo con el Package Pblock. Palma (Illes Balears), España: Edicions U.
Pérez Pineda., J. A. (2006). Spatial econometrics and regional science. Investig. Económica. 65 (258), 129–160. doi:10.1111/j.1435-5957.2008.00208.x
Perring, M. P., De Frenne, P., Baeten, L., Maes, S. L., Depauw, L., Blondeel, H., et al. (2016). Global environmental change effects on ecosystems: The importance of land-use legacies. Glob. Chang. Biol. 22 (4), 1361–1371. doi:10.1111/gcb.13146
Phillips, J., Duque, Á., Scott, C., Wayson, C., Galindo, G., Cabrera, E., et al. (2016). Live aboveground carbon stocks in natural forests of Colombia. For. Ecol. Manage. 374, 119–128. doi:10.1016/j.foreco.2016.05.009
Potapov, P. V., Turubanova, S. A., Hansen, M. C., Adusei, B., Broich, M., Altstatt, A., et al. (2012). Quantifying forest cover loss in Democratic Republic of the Congo, 2000–2010, with Landsat ETM+ data. Remote Sens. Environ. 122, 106–116. doi:10.1016/j.rse.2011.08.027
Prem, M., Saavedra, S., and Vargas, J. F. (2020). End-of-conflict deforestation: Evidence from Colombia’s peace agreement. World Dev. 129 (129), 104852. doi:10.1016/j.worlddev.2019.104852
R Core Team (2017). R: A language and environment for statistical computing. Available at: https://www.R-project.org/.
Reuveny, R., Mihalache-O’Keef, A. S., and Quan, Li (2010). The effect of warfare on the environment. J. Peace Res. 47 (6), 749–761. doi:10.1177/0022343310382069
Rincón, A., and Ligarreto, G. (2007). Productividad de la asociación maíz-astos en suelos ácidos del Piedemonte Llanero colombiano. Revista Corpoica-Ciencia y Tecnología Agropecuaria 9 (1), 73–80. doi:10.21930/rcta.vol9_num1_art:107
Rincón, Á., and Ligarreto, G. (2008). Productividad de la asociación maíz–pastos en suelos ácidos del Piedemonte Llanero colombiano. Ciencia Tecnología Agropecuaria 9 (1), 73–80. doi:10.21930/rcta.vol9_num1_art:107
Rincón-Ruiz, A., and Kallis, G. (2013). Caught in the middle, Colombia’s war on drugs and its effects on forest and people. Geoforum 46, 60. Available at: https://www.sciencedirect.com/science/article/abs/pii/S0016718512002837.
Rincón-Ruiz, A., Pascual, U., and Flantua, S. (2013). Examining spatially varying relationships between coca crops and associated factors in Colombia, using geographically weight regression. Appl. Geogr. 37, 23–33. doi:10.1016/j.apgeog.2012.10.009
Rodríguez-de-Francisco, J. C., del Cairo, C., Ortiz-Gallego, D., Velez-Triana, J. S., Vergara-Gutiérrez, T., and Hein, J. (2021). Post-conflict transition and REDD+ in Colombia: Challenges to reducing deforestation in the Amazon. For. Policy Econ. 127, 102450doi:10.1016/j.forpol.2021.102450
Salazar, G. S. (2016). El conflicto armado y su incidencia en la configuración territorial: Reflexiones desde la ciencia geográfica para la consolidación de un periodo de pos negociación del conflicto armado en Colombia. Bitácora Urbano Territorial 26 (2), 45. doi:10.15446/bitacora.v26n2.57605
Sanchez-Cuervo, A. M., and Aide, T. M. (2013). Identifying hotspots of deforestation and reforestation in Colombia (2001–2010): Implications for protected areas. Ecosphere 4 (11), art143. doi:10.1890/es13-00207.1
Schoon, M. L., and Cox, M. E. (2012). Understanding disturbances and responses in social-ecological systems. Soc. Nat. Resour. 25 (2), 141–155. doi:10.1080/08941920.2010.549933
Statista Research Department (2021). Statista research department. Available at: https://es.statista.com/estadisticas/1191612/numero-de-personas-en-colombia-por-departamento (Accessed January 6, 2022).
Suarez, A., Árias-Arévalo, P. A., and Martínez-Mera, E. (2018). Environmental sustainability in post-conflict countries: Insights for rural Colombia. Environ. Dev. Sustain. 20 (3), 997–1015. doi:10.1007/s10668-017-9925-9
Szantoi, Z., Jaffrain, G., Gallaun, H., Bielski, C., Ruf, K., Lupi, A., et al. (2021). Quality assurance and assessment framework for land cover maps validation in the Copernicus Hot Spot Monitoring activity. Eur. J. Remote Sens. 54 (1), 538–557. doi:10.1080/22797254.2021.1978001
Turner, W., Rondinini, C., Pettorelli, N., Mora, B., Leidner, A. K., Szantoi, Z., et al. (2015). Free and open-access satellite data are key to biodiversity conservation. Biol. Conserv. 182, 173. Available at: https://www.sciencedirect.com/science/article/abs/pii/S000632071400473X.
UNODC y Ministerio de Justicia y del Derecho (2015). Caracterización Regional de la problemática asociada a las drogas ilícitas en el departamento del Meta. Guaviare y Caqueta. Available at http://www.odc.gov.co/TERRITORIOS/Documentos/Caracterizaciones.
Villa, Romero. A. R., Moreno Altamirano, L., and García de la Torre, G. S. (2012). Epidemiología y estadística en salud pública. McGraw-Hill Medical.
Warne, R. (2014). A primer on multivariate analysis of variance (MANOVA) for behavioral scientists. Pract. Assess. Res. Eval. 1917. doi:10.7275/sm63-7h70
Yasmi, Y., Kelley, L. C., and Enters, T. (2013). Community–outsider conflicts over forests: Perspectives from southeast asia. For. Policy Econ. 33, 21–27. doi:10.1016/j.forpol.2012.05.001
Yepes, A., Navarrete, D. A., Phillips, J. F., Duque, A. J., Cabrera, E., and Galindoet, al. (2011). Estimación de las emisiones de dióxido de carbono generadas por deforestación durante el periodo 2005-2010. Bogotá (Colombia): Instituto de Hidrología. Available at: http://www.ideam.gov.co/documents/13257/13548/Emisiones.pdf/a86b9bd1-0050-4bb9-a54f-c3ad6b3cda26.
Appendix A: Factors used in Model 2 to understand the role of a specific year (i.e., time) and a municipality’s characteristics on deforestation in Neotropical forests of central Colombia
Keywords: Colombia, socio-ecological systems, ecosystem services, armed conflict, deforestation, spatiotemporal analyses
Citation: Toro G, Otero MP, Clerici N, Szantoi Z, González-González A and Escobedo FJ (2022) Interacting municipal-level anthropogenic and ecological disturbances drive changes in Neotropical forest carbon storage. Front. Environ. Sci. 10:937147. doi: 10.3389/fenvs.2022.937147
Received: 05 May 2022; Accepted: 22 August 2022;
Published: 13 September 2022.
Edited by:
Irene Petrosillo, University of Salento, ItalyReviewed by:
Swapan Talukdar, Jamia Millia Islamia, IndiaSolhanlle Bonilla-Duarte, Santo Domingo Institute of Technology, Dominican Republic
Copyright © 2022 Toro, Otero, Clerici, Szantoi, González-González and Escobedo. This is an open-access article distributed under the terms of the Creative Commons Attribution License (CC BY). The use, distribution or reproduction in other forums is permitted, provided the original author(s) and the copyright owner(s) are credited and that the original publication in this journal is cited, in accordance with accepted academic practice. No use, distribution or reproduction is permitted which does not comply with these terms.
*Correspondence: Nicola Clerici, nicola.clerici@urosario.edu.co