Compaction of Coarse-Textured Soils: Balance Models across Mineral and Organic Compositions
- 1Department of Soils and Agri-Food Engineering, Université Laval, Québec, QC, Canada
- 2Agriculture and Agri-Food Canada, Québec, QC, Canada
- 3Facultad de Ciencias Agrarias, Universidad de Cienfuegos, Cienfuegos, Cuba
Soil bulk density (BD), degree of compactness (DC), maximum bulk density (MBD), and critical water content (CWC) at which MBD is reached are commonly used to characterize soil compaction, and can be predicted from soil texture and organic matter content, omitting other components such as sand sub-classes and soil cementing agents and potential biases such as data redundancy and sub-compositional incoherence. Compositional data analysis is needed to account for interactions among soil components and to avoid biases. The aim of this study was to relate soil compaction indexes to the basic components of coarse-textured soils using unbiased numerical techniques. Soil samples collected in horizons A and B at 49 sites in Quebec, Canada, were analyzed for gravimetric water content, BD, particle-size distribution, MBD, CWC, organic C, total N, Si, Fe, Al, Mn, Mg, and Ca. DC was calculated as the ratio of BD to MBD. The 14 physical-chemical soil properties were expressed as isometric log-ratios balances. We conducted principal component analysis to identify the components most correlated with compaction indexes. We used regression analysis to predict MBD and CWC, and used linear mixed-effects models to predict BD and DC. The regression models accounted for up to 83% of total variation in MBD and CWC, and the linear mixed-effects models explained 58–64% of total variation in BD and DC. BD and DC were found to decrease with clay content, and increase with larger proportion of coarser particles. Organic matter content tended to reduce BD and DC, and showed little effects on MBD. Increasing evenness of sand fractions resulted in a higher MBD value. Relationships between CWC and soil texture, and between CWC and organic C were not significant. Mineral cementing agents were the major contributors to soil compaction indexes. Si, Al, Fe, and Ca oxides increased BD, DC, and CWC, but reduced MBD. The sensitivity of coarse-textured soils to compaction could be predicted to support decisions on soil resilience after ripping and on the need to implement corrective chemical, biological and physical methods such as soil amendments, structure-building crops or textural mixtures to rebalance soil compositions.
Introduction
Soil quality is defined by the chemical, physical and biological attributes of the top 15 cm (Doran and Parkin, 1996; Boiteau et al., 2014) down to rooting depth (Spoor et al., 2003). Loss of soil quality affects crop productivity and thus is a major issue in intensive production systems. Soil compaction caused by natural processes (Sanborn et al., 2011) and heavy machines (Alakukku et al., 2003) is one of the major soil degradation problems worldwide. Crop yields may drop by average rates ranging from 15% in maize across soil textural groups (Duiker and Curran, 2004; Wolkowski and Lowery, 2008) to 34% in potato grown in coarse-textured soils (Stalham et al., 2005; Wolkowski and Lowery, 2008). Potato and maize crops grown sequentially in coarse-textured soils may therefore suffer considerably from soil compaction.
Naturally compacted layers are classified as fragipan, placic horizons, duripan, petrocalcic, petrogypsic, continuous ortstein (Soil Survey Staff, 2014). Anthropic compaction results in soil air space reduced to < 10% by heavy machines and in increased cohesive forces between particles (Hamza and Anderson, 2005). An agric or plow pan may form in the arable layer; a “no-till pan” of high bulk density, low porosity, and high mechanical resistance may underlie a reduced compaction layer, and overlie a plow pan (Reichert et al., 2003; Håkansson, 2005). Water storage capacity increases with depth to pan (Frye et al., 1985). Compacted layers within 50 cm of the soil surface limit rooting depth (Grossman and Carlisle, 1969). Rootability is hampered where soil resistance exceeds 1 MPa for potato and 2–3 MPa for most other crops (Håkansson and Lipiec, 2000; Stalham et al., 2005).
Several approaches have been developed to measure soil compaction (Lipiec and Hatano, 2003). Soil bulk density (BD) is commonly used to characterize the state of soil compaction (Gupta and Allmaras, 1987). The degree of compactness (DC) (Håkansson, 1990) is defined as the ratio of (BD) to a reference (BD) obtained by uniaxial compression at a static pressure of 200 kPa. The Proctor test is a widely accepted procedure applied to disturbed soils to determine the resistance of agricultural soils to compaction (Ekwue and Stone, 1995; Thomas et al., 1996; Zhang et al., 1997) over a broad range of soil water contents under a standardized dynamic load (Hillel, 2013). Common indexes are maximum Bulk Density (MBD) under the Proctor test and the critical water content (CWC) at which MBD is reached (Zhao et al., 2007). Despite the importance of compaction indexes for guiding soil management, they are not reported in soil surveys. Direct measurement of BD requires the collection of undisturbed soil cores, a procedure considered to be labor-intensive, time-consuming, and tedious (Suuster et al., 2011). Measuring MBD and CWC to derive DC is even more difficult and time-consuming than measuring BD.
Pedotransfer regression functions have been developed to predict soil BD from soil physical and chemical properties such as texture, organic matter, total N and pH (Tranter et al., 2007; Martin et al., 2009; Jalabert et al., 2010), water content (Benites et al., 2007; Suuster et al., 2011; Brahim et al., 2012), moisture and packing density (Quiroga et al., 1999; Jones et al., 2003). However, the variation in MBD has been attributed to changes in particle-size distribution (Nhantumbo and Cambule, 2006; Zhao et al., 2008), especially clay and silt contents (Bennie and Burger, 1988). The CWC was predicted from soil texture and organic matter content (Aragón et al., 2000). However, particle-size distribution and soil organic matter can only partially explain the state of soil compaction.
Cementing agents play an important role in soil compaction and pore clogging. Cementing agents can enhance aggregate stability, leading to higher soil shear strength (Yee and Harr, 1977). Dissolved salts, organic acids, hydroxides, and oxides from secondary minerals (Duiker et al., 2003; Sanborn et al., 2011), lime, humic substances, hydroxyl-Al polymers, Al and Ca phosphates and Si3+, Fe3+, Al3+, and Ca2+ compounds act as cementing agents, whereas K promotes soil dispersion (Pagé and Berrier, 1983; Haynes and Naidu, 1998). Polyvalent cations Mn2+, Ca2+, and Mg2+ form cationic bridges with clay particles and soil organic matter (Lal and Shukla, 2004; Bronick and Lal, 2005). Hydrophilic oxy-hydroxides, Si-hydroxides, and amphiphilic humic substances interact in coarse-textured soils, whose capacity to retain water is low compared to finer textures (Tschapek, 1984). However, cementing agents are rarely considered in compaction models.
Furthermore, little attention has been paid to the nature of soil compositional data and to full compositions. Soil components are subject to methodological bias if they are not handled as compositional data (Parent et al., 2012). Compositional data are proportions of a total such as 100% (Aitchison, 1982), therefore, components are intrinsically multivariate and related to each other in a compositional space constrained between 0 and 100%: any change in one proportion must affect the other proportions. Van Den Boogaart and Tolosana-Delgado (2006) warned that statistical analyses of compositional data can be misleading or inapplicable due to systematic negative bias (one covariance is forced to be negative), sub-compositional incoherence, redundancy of information (one component can be deduced by subtracting the sum of the others from 100%), and non-normal distributions (data and their associated statistics or predicted values should not range below 0 or beyond 100%). Soil and plant compositions have been handled statistically using log ratio transformations (Parent et al., 2012; Parent L. E. et al., 2013; Parent S. E. et al., 2013). Current soil compaction research (e.g., Benites et al., 2007; Suuster et al., 2011; Brahim et al., 2012) does not avoid methodological bias due to closure and spurious correlations among soil components. Data transformation using isometric log ratios or orthonormal balances is the most appropriate to conduct multivariate analysis on compositional data (Filzmoser et al., 2009).
The objectives of this study were to: (i) express physical-chemical soil properties using unbiased compositional data analysis tools; (ii) relate soil compaction indexes (BD, DC, MBD, and CWC) to the isometric log ratio-transformed basic components of coarse-textured soils using principle components analysis and correlation analysis; (iii) predict BD, DC, MBD and, CWC from orthonormal balances using linear-mixed model and regression analysis. We hypothesized that combinations of soil texture, organic matter content, and mineral cementing agents impact differentially on soil resistance to compaction.
Materials and Methods
Materials
The research area located in the Province of Quebec, Canada (37°09′–36°42′ N; 38°48′–39°12′ E). We selected 49 potato farm sites where crop sequences included potato (Solanum tuberosum L.), maize (Zea mays L.), soybean [Glycine max (L.) Merr.], wheat (Triticum aestivum L.), alfalfa (Medicago sativa L.), barley (Hordeum vulgare L.), and canola (Brassica napus L.). Soils are classified as Aquents (Entisols), Orthods (Spodosols) and Udepts (Inceptisols) in the USDA soil classification system (Soil Survey Staff, 2014). In June and July 2014, 97 large (>20 kg) samples were collected from 49 horizon A and 48 horizon B to conduct Proctor tests (ASTM D1557, 2009). Sampling depth varied from site to site with the development of genetic horizons A and B. Average sampling depth for horizon A was 10.5 cm in the range of 4–18 cm. Sampling depth for horizon B was 17–48 cm, averaging 33.4 cm. Soil samples were air dried and then stored at room temperature. Smaller samples were collected in the center of each horizon using the cylinder method (Blake and Hartge, 1986).
Soil physical properties included gravimetric water content (Topp et al., 2007), BD (Blake and Hartge, 1986), particle-size distribution, MBD (ASTM D1557, 2009), CWC (ASTM D1557, 2009) expressed on an oven-dried (105°C) basis, and DC calculated as the ratio of BD to MBD. Proportions of coarse sand (0.50–2.00 mm), medium sand (0.25–0.50 mm), and fine sand (0.05–0.25 mm) were determined by dry sieving; the proportions of silt (0.002–0.05 mm) and clay (< 0.002 mm) were determined using a modified hydrometer method (Kroetsch and Wang, 2007). Soil chemical analyses were conducted using < 2.00 mm sieved samples. C and N were quantified by dry combustion (Leco-CNS). Si, Al, Fe, Mn, Mg, and Ca were extracted using the acid ammonium oxalate method (Courchesne and Turmel, 2007) and then quantified by the inductively coupled plasma (ICP) technique. Descriptive statistics of soil data are presented as raw data in Supplementary Table 1.
Log Ratio Transformation
Isometric log ratios are orthogonal projections of compositional data arranged in binary subsets of components displayed in a sequential binary partition (SBP) (Egozcue et al., 2003). As there are D-1 degrees of freedom in compositional vectors (Aitchison and Greenacre, 2002), log ratio transformation techniques consist of D-1 ILR (Egozcue et al., 2003).
For the 14 soil components, there are 13 ILR variables designed to represent the BD and DC field (F) files, on the one hand, and the MBD and CWC Proctor (P) files on the other (Figures 1, 2). Given that soil gravimetric water content relates to BD and CWC relates to MBD, F1 was set as the balance between soil water content and solid components, while P1 was the balance between CWC for the Proctor test and the solid components. The F2 and P2 balances contrasted soil organic matter with mineral components, showing the functional role of organic matter in soil aggregation. Balances were further elaborated by associating other groups of particles. The F4 and P4 balances contrasted mineral soil particles with mineral cementing agents.
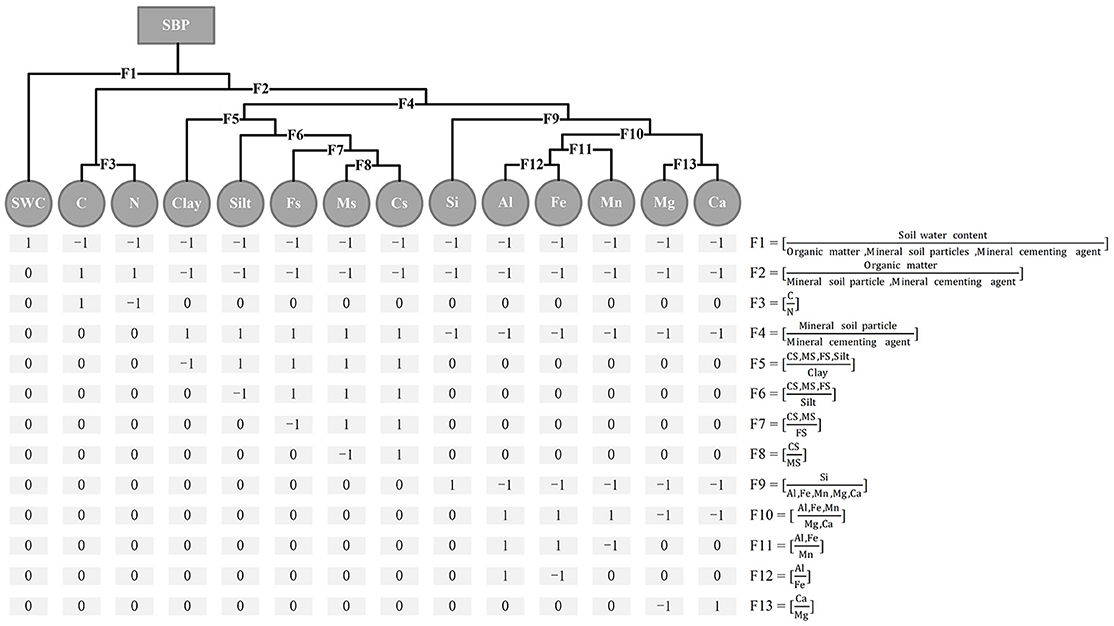
Figure 1. Sequential binary partition (SBP) of soil components to compute isometric log ratios for bulk density and degree of compactness. SWC, soil water content; Fs, fine sand; Ms, medium sand; Cs, coarse sand.
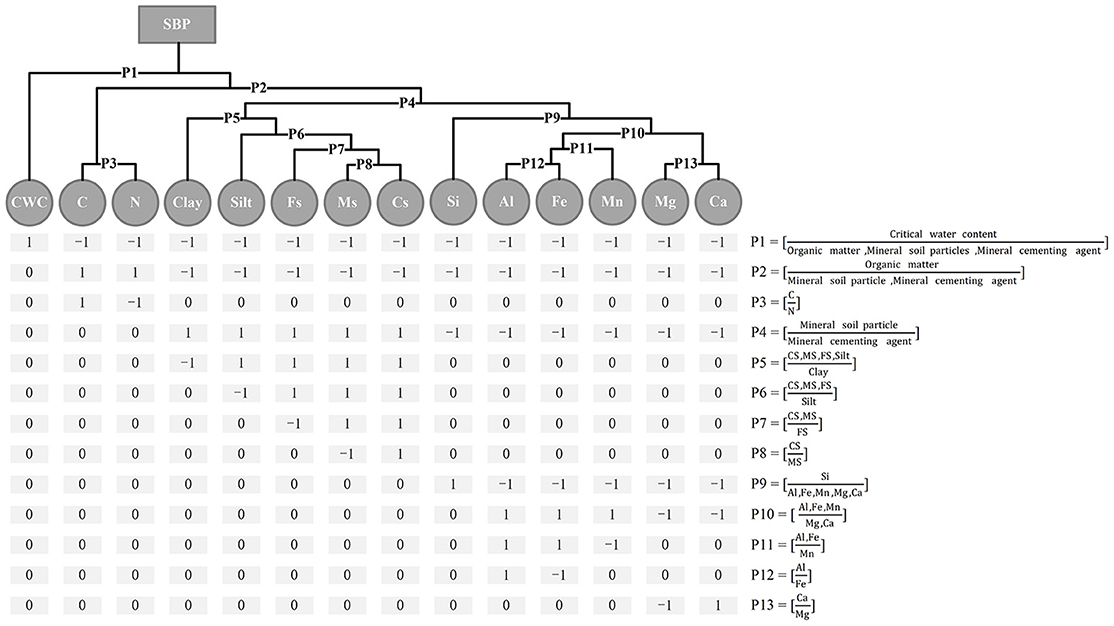
Figure 2. Sequential binary partition (SBP) of soil components to compute isometric log ratios for maximum bulk density and critical water content. CWC, critical water content; Fs, fine sand; Ms, medium sand; Cs, coarse sand.
ILR is a normalized ratio between geometric means of two subsets of functional components (tagged “+” for parts in the numerator and “−” for parts in the denominator), calculated as follows (Egozcue and Pawlowsky-Glahn, 2006):
where i = 1 to D-1, ILRi is the ith isometric log ratio in the ith row of the SBP between and , geometric means across components, and and are numbers of components labeled “+1” and “−1.”
Statistical Analysis and Model Development
Numerical analyses were performed in the R statistical computing environment using the compositions package (van den Boogaart et al., 2014) to transform compositional data into isometric log ratios, the dplyr package (Wickham and Francois, 2015) for general data manipulation, the nlme package (Filzmoser and Gschwandtner, 2015) to develop the linear mixed model, and the pls package (Revelle, 2014) for principal component analysis (PCA). We conducted PCA across (1) sampling depths and the 13 F balances to synthesize factors affecting BD and DC, and (2) the 13 P balances and 12 P balances to identify the components most closely related to MBD and CWC. Correlation analyses were conducted between the selected principal components (PCs) and compaction indexes (BD, DC, MBD, and CWC) using site scores (49 sites for the horizon A and 48 sites for the horizon B) of selected PCs.
Both horizons provided a gradient of soil properties to model BD and DC. The 13 F balances, along with sampling depth in both horizons, were used to predict BD and DC using the linear mixed-effects (LME) model as follows:
where Y is the soil compaction index (BD or DC), X is the fixed effects including 13 ILR balances, and sampling depth, β is the fixed-effects vector, Z is the random-effects matrix, b is the random-effects vector, and ε is the observational error vector. The site was considered as random effect. To model MBD and CWC, horizons A and B were separated as different entities requiring specific diagnoses for planning farm operations using regression analysis for each horizon. Prediction performance was evaluated using the Akaike information criterion (AIC) and the coefficient of determination (R2). The AIC value is used to compare and classify multiple competing models and to estimate which is the closest to the “real” process underlying the biological phenomenon under study (Burnham and Anderson, 2003; Burnham et al., 2011; Symonds and Moussalli, 2011). The coefficient of determination is the proportion of the variation that can be explained by the set of predictor variables. Mean prediction error (MPE) and root mean squared error (RMSE) were used to measure the reliability of the models, as follows:
where σi and ρi are the observed and predicted dependent variables for the ith measurement, respectively, and n is the number of observations. The MPE indicates an average underestimation (positive bias) or overestimation (negative bias) of dependent variables. For a good prediction, R2 should be as large as possible, and the AIC value, MPE, RMSE should be as small as possible (Benites et al., 2007).
Results
Variables Related to Soil BD and DC
The first four PCs explained 71.3% of the total variation in the variables included in PCA (Table 1). All PCs showed significantly negative correlations with BD, whereas PC1, PC3, and PC4 were significantly and negatively correlated with DC. However, DC is a more useful compaction index than BD because DC corrects BD for MBD, an intrinsic soil property reflecting the maximum effect of machines on the degradation of soil physical quality.
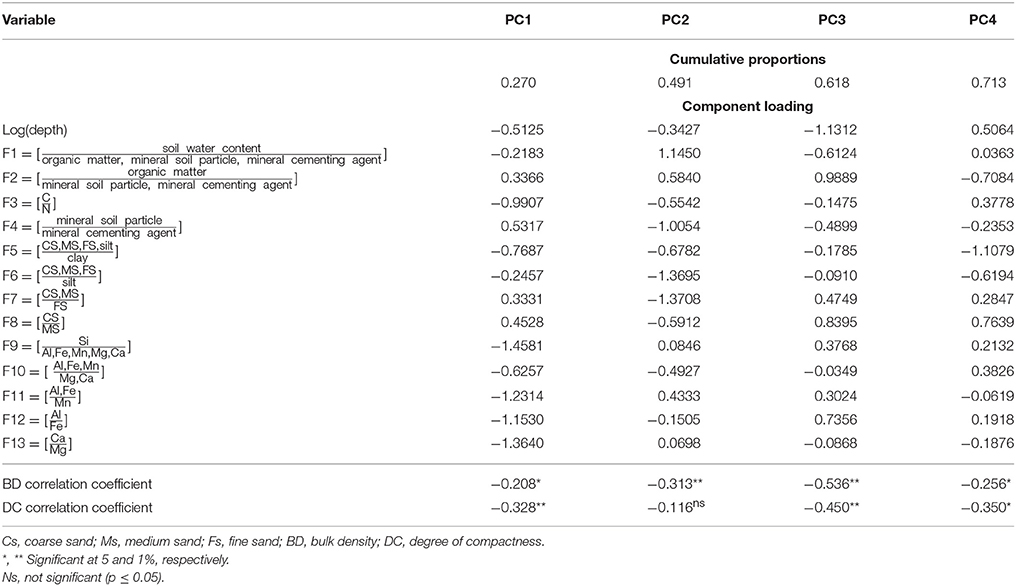
Table 1. Results of principal component analysis and loadings of component for soil bulk density and the degree of compaction.
The value and sign of the loadings and correlation coefficients are a measure of the relationships between the original variables and soil compaction indexes. The interpretation of loadings in Table 1 is straightforward. If loadings and correlation coefficients have the same sign, the relationship between ILR and compaction index is positive; otherwise, it is negative. ILRs are noted as , hence, larger values in the denominator lead to more negative numbers in the log scale, and vice versa. For example, if Al loads more on F12 = , the balance increases. If Fe loads more, the balance decreases, reversing the relationship between the balance and compaction indexes.
The effects of PC1 and PC3 on DC were highly significant (P < 0.01). The largest loadings in PC1 were F3, F9, F11, F12, and F13, indicating that DC was positively related to the mineral cementing agents, especially Si, Fe, Al, and Ca, and positively related to F3, hence DC was larger where the C/N ratio was higher. PC3 was primarily influenced by sampling depth, F2 and F8. DC was significantly higher in horizon B (Supplementary Table 1). F2, representing the balance between organic and mineral components, was negatively related to DC. F8, the ratio between coarse and medium-size sand particles, was negatively related to DC. The effects of PC4 on DC were also significant (P < 0.05), being mainly driven by F5, the [] balance, where larger particles tended to increase DC compared to clay.
In contrast with DC, BD was significantly (P < 0.01) related to PC2. PC2 was largely influenced by F1, F4, F6, and F7. F1 was negatively related to BD. F4, reflecting the balance between mineral soil particles and mineral cementing agents, was positively related to BD. Where mineral cementing agents loaded more, BD was lower. F6 and F7 represented coarser particles in the soil. The higher the proportion of coarser particles, the higher BD was. BD was influenced significantly, but to a lesser extent, by PC1 and PC4. Consistent with DC, BD was also positively related to F5, F9, F11, F12, F13, and sampling depth.
Factors Affecting Soil MBD and CWC
The first four components and their loadings for MBD are presented in Table 2. The first four PCs explained 77.5 and 72.7% of total variation in all variables in horizons A and B respectively. However, the fourth PC was not significantly related to MBD in either horizon. Mineral cementing agents loaded most on PC1. The MBD was related negatively to P9, P11, P12, and P13 balances in both horizons. In PC2, the higher proportion of soil water (P1) and soil organic matter (P2) tended to reduce MBD in horizon A, whereas the higher proportion of coarser particles (P5–P7) tended to increase MBD in both horizons. In PC3, the higher proportion of coarser particles than of medium-size particles (P8) tended to reduce MBD in horizon A, whereas the higher proportion of clay than of coarser particles (P5) tended to increase MBD in horizon B.
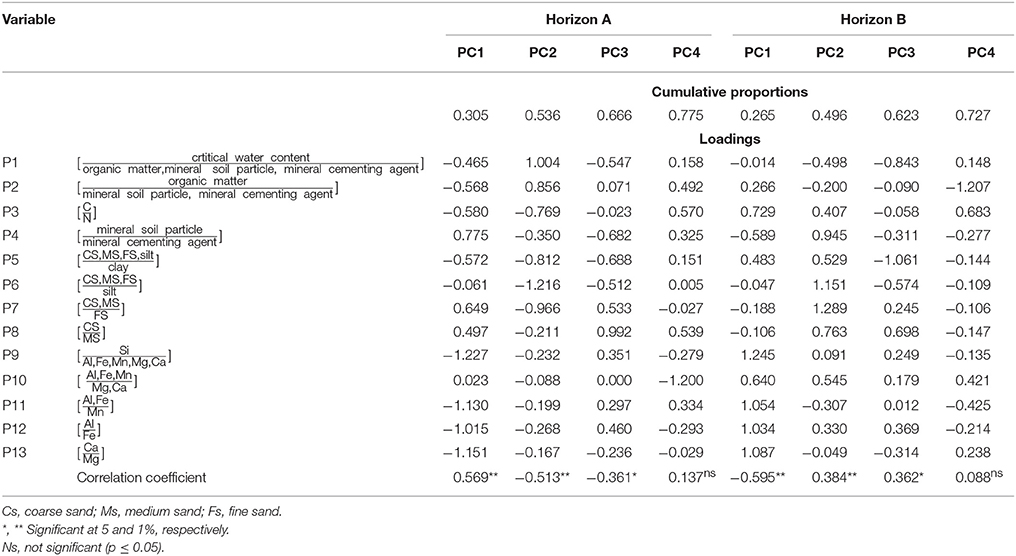
Table 2. Results of principal component analysis and loadings for soil properties related to the maximum bulk density.
The first four components and their loadings for CWC are presented in Table 3. The first four PCs explained 78.0 and 76.4% of total variation in all variables in horizons A and B respectively. The four PCs were significantly related to CWC in horizon A, and only the third PC was significantly related in horizon B. The CWC was related positively to P9, P11, P12, and P13 balances involving mineral cementing agents that loaded most on PC1 of horizon A. PC2 in horizon A revealed that increasing the proportion of organic matter compared to mineral solids (P2) increased CWC, whereas coarser particles (P6 and P7) tended to reduce CWC. PC3 in horizon A displayed a relatively higher content of coarse sand than of medium sand (P8), leading to a larger CWC, whereas a higher proportion of clay than of coarser particles (P5) tended to increase CWC in horizon B. Since P10 contributed most to PC4 of horizon A, a higher proportion of sesquioxides (Al, Fe, Mn) than of divalent cations (Ca, Mg) was positively related to CWC in horizon A.
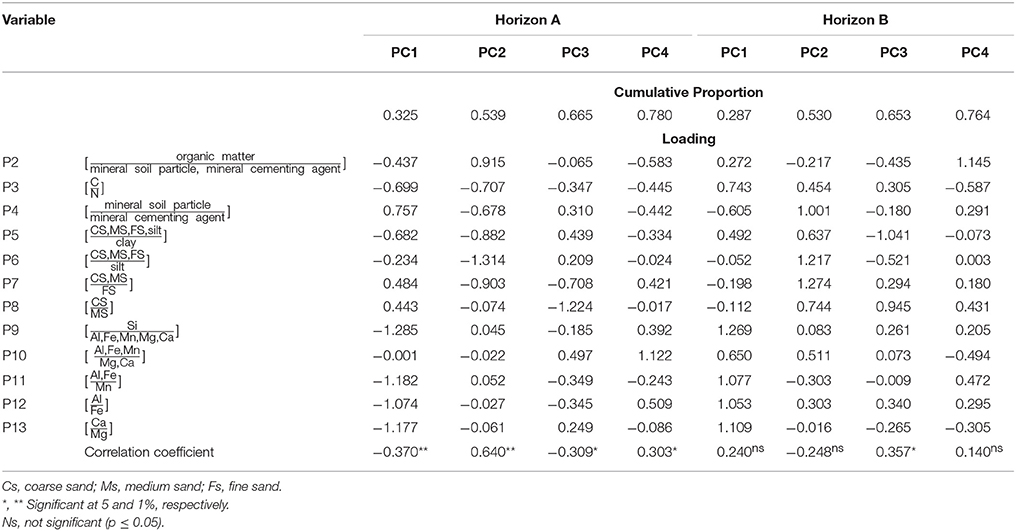
Table 3. Principal component analysis and loadings for soil properties related to the critical water content.
Predictive Models for Compaction Indexes
BD and DC
Model coefficients and their significance are presented in Table 4. The F2, F4, F6, F7, F9, and F12 balances, meaning organic matter content, particle-size distribution and mineral cementing agents, significantly influenced BD prediction. The F1, F4, F5, F9, F10, and F12 balances, meaning soil gravimetric water content, particle-size distribution and mineral cementing agents, significantly influenced DC prediction. The MPE was 0.008 and 0.002 for BD and DC, respectively, indicating some underestimation of both BD and DC (Figure 3).
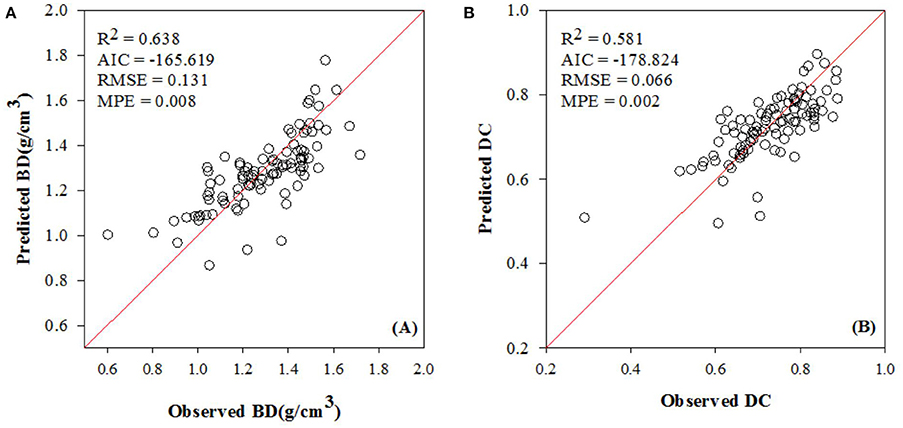
Figure 3. Model prediction of (A) BD and (B) DC. BD, bulk density; DC, degree of compactness; AIC, akaike information criterion; MPE, mean prediction error; RMSE, root mean squared error.
MBD and CWC
Results of the regression model for predicting MBD are as follow:
Horizon A,
Horizon B,
Regression models for predicting CWC resulted in the following equations:
Horizon A,
Horizon B,
The R2 values were larger for MBD and CWC in horizon A and were much lower in the horizon B. The MBD was attained at specific CWC values depending on soil properties. The MPE was small. Asterisk (*) in the equations indicates the significant variables at P < 0.05. The results suggested a small number of balances significantly (P < 0.05) affected the prediction of MBD and CWC. The MBD prediction primarily involved the P4 and P9 balances, which are related to particle-size distribution and mineral cementing agents. The CWC prediction primarily involved the P4, P5, and P9 balances. In both cases, mineral cementing agents proved to be significant components.
The MBD and CWC were closely related to each other in horizon A only (Figure 4). Therefore, MBD varied widely in horizon A but did not change significantly with CWC in horizon B. For a median coarse-textured soil in our dataset, the estimated MBD was 1.59 g cm−3 for the horizon A and 1.54 g cm−3 for the horizon B, and the estimated CWC was 205 g kg−1 for the horizon A and 144 g kg−1 for the horizon B, due to major differences in composition.
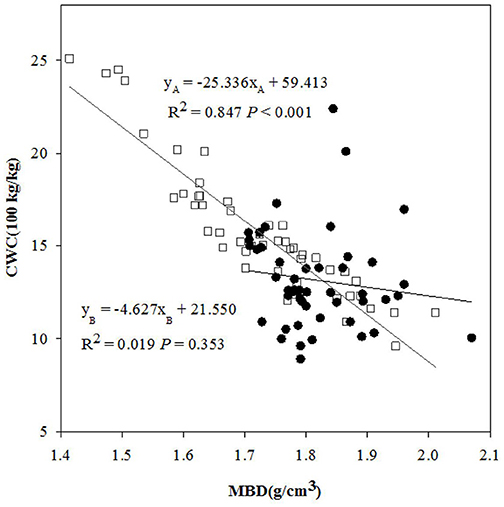
Figure 4. Relationship between maximum bulk density (MBD, g cm−3) and critical water content (CWC, 100 kg kg−1). Open and closed symbols refer to horizon A and horizon B, respectively.
Discussion
Effects of Sampling Depth and Soil Components on BD and DC
As shown by significant differences in BD and DC between horizons A and B, the sub-layers were more compacted than the arable layer for the pressure generated by loading the soil increases BD values deeper in the soil (Tranter et al., 2007). Studies have concluded that a small part of the variability in BD can be ascribed to sampling depth (Calhoun et al., 2001; De Vos et al., 2005; Heuscher et al., 2005). In the present study, sampling depth was not an independent variable as it is in most BD and DC prediction models (Reichert et al., 2009), because sampling depth is confounded with soil compositions.
Previous research reported that the degree of compaction mainly depends on soil moisture, texture and organic matter content (Jones et al., 2003; Hamza and Anderson, 2005; Dexter et al., 2008). BD has been found to decrease with higher clay and clay-plus-silt contents which is in agreement with our result (Kaur et al., 2002; Benites et al., 2007; Reichert et al., 2009), whereas the effect of medium and coarse sand on BD varied among soils, regions and horizons (Calhoun et al., 2001; Kaur et al., 2002; De Vos et al., 2005). Medium sand appeared to increase BD compared to coarse sand in our coarse-textured soils, in agreement with previous studies (Suuster et al., 2011). The effect of clay on DC was found to be negatively related to clay content but must also depend on organic matter content (da Silva et al., 1997).
It has been frequently reported that the BD of high-C soils can be explained by the variation in C content, whereas soil texture has a major effect on the BD of low-C soils (Manrique and Jones, 1991; Kaur et al., 2002). Total N showed a negative relationship with BD and DC (Benites et al., 2007). In the present study, organic matter content also tended to decrease the BD and DC of coarse-textured soils, whereas the [] balance was related positively to BD and DC. The [] balance decreases from lighter particulate organic matter to heavier fractions associated with soil mineral particles in agricultural soils (Yang et al., 2012). Total C thus provided partial information on the real contribution of organic matter to soil resistance to compaction.
Manrique and Jones (1991) suggested that texture and soil properties other than organic C play a more significant role in controlling BD deeper in the soil profile. A large contribution of water content to subsoil compaction is expected primarily on coarse- and medium-textured soils and heavy-textured soils with high shrink-swell potential (Suuster et al., 2011). The [] balance increased DC, facilitating particle rearrangement, whereas soil gravimetric water content reduced MBD as the soil liquid phase is of low density and is incompressible.
Conversely, although it represents just a small part of total C, dissolved organic carbon (DOC) may contribute to the formation of naturally compacted layers (Sanborn et al., 2011). The main sources of organic C in subsoils are DOC, plant roots and root exudates, and organic particulates transported from soil surface (Rumpel and Kögel-Knabner, 2011). Organic C is a complex key soil quality index characterized by two major biochemical pools (Andrén and Kätterer, 1997) and several size fractions (Six et al., 2002; Stewart et al., 2008; Tong et al., 2014). Hence, total C should be further split into several C fractions (i.e., light fraction organic carbon, dissolved organic carbon and particulate organic carbon) to determine their respective significance in BD and DC models.
Effects of Soil Components on MBD and CWC
Felton and Ali (1992) found that the addition of organic matter increased soil porosity and water retention and reduced MBD as determined by the Proctor test. The effectiveness of organic matter depended on soil texture and organic matter quality (Zhang et al., 1997). Organic matter may increase in significance in cases where living and dead roots provide a more filamentous network across the soil profile (Soane, 1990). In the present study, the balance that included organic matter content showed negligible effect, probably because the range of soil organic matter contents was relatively narrow.
Clay content may lead to lower MBD (Smith et al., 1997) or show little effect (Aragón et al., 2000; Ball et al., 2000). The relationship between MBD and clay-plus-silt is quadratic for specific ranges of soil texture (Nhantumbo and Cambule, 2006; Mujtaba et al., 2014). Moolman and Weber (1978) reported that increasing evenness of particle-size distribution resulted in a higher MBD value, indicating the need to include particle-size balances such as P5 and P6 in MBD models. In contrast, Van Der Watt (1969) analyzed soils where MBD could be well-predicted from coarse sand (0.5–2.0 mm) only. Well-graded sands have a higher MBD or lower CWC compared to poorly graded sands (Guerrero, 2004; Mujtaba et al., 2014).
There is usually a close relationship between CWC and soil texture or organic C (Wagner et al., 1994; Aragón et al., 2000), but no such relationship was observed in the present study, apparently due to the narrow range of organic C contents. However, clay content was found to be linearly and positively related to CWC. The CWC was poorly predictable in horizon B. Mujtaba et al. (2014) and Guerrero (2004) found that MBD and CWC were poorly related in sandy soils. When water is added to dry soil, particles absorb a film of water. A certain amount of added water thickens the water film, allowing the soil particles to slide over each other in a process known as lubrication, which is triggered by fine-grain particles (Ishibashi and Hazarika, 2010). Bruand et al. (2005) concluded that particle-size distribution and the mineralogy of silt and clay particles associated with sand can lead to variations in physical properties, e.g., water retention, resistance to penetration and permeability of sandy soil. Zhao et al. (2008) reported that CWC was closely related to the liquid and plastic limits that integrate several soil properties such as particle-size distribution, organic matter content, and clay mineralogy. Hence, CWC could be predicted more accurately using liquid and plastic limits (Soane et al., 1972).
Effects of Mineral Cementing Agents on Compaction Indexes
Mineral binding agents must have positive effect on maintaining soil structure and resisting to soil compaction (Lal and Shukla, 2004; Bronick and Lal, 2005). Where the average content of Al, Fe, Mn, Mg and Ca loaded more, BD and DC values were less. The positive relationship between F11, F12 with BD and DC appeared to reflect the effect of Al, Fe, and Mn on soil mass rather than on soil strength, as soil particle density increased with content of soil oxides, agreeing with McKeague and Sprout (1975). BD and DC were positively related to F13 ([]). Increasing soil aggregation and structural stability can be observed in soil with high Ca compared to Mg attributed to the thinner hydration radius of Ca and its effect on clay flocculation (Favaretto et al., 2006).
In general, only soil texture and organic C are used to predict MBD (Aragón et al., 2000; Nhantumbo and Cambule, 2006). We showed the importance of mineral cementing agents in predicting MBD of agricultural soils, as found by Zhao et al. (2008) for forest soils in British Columbia, Canada. The Si, Al, Fe and Ca oxides increased CWC but reduced MBD. Zhao et al. (2008) found that Al and Fe oxides were positively related to CWC, but were negatively related to MBD. Concentrations of Si, Mn, Al, Fe, Ca and Mg oxides are positively related to CWC as such compounds are hydrophilic (Tschapek, 1984). The negative relationship between MBD and mineral cementing agents in our study reflected the enhanced soil strength due to the presence of soil oxides.
Remediation Techniques Supported by the Predictive Models
Mechanical, biological and chemical means can be implemented alone or in combination to enhance soil resilience to compaction. The moisture content of coarse-textured soils should be < 50 g kg−1, well below the median CWC of 205 g kg−1 in horizon A and 144 g kg−1 in horizon B, to achieve fracturing and shattering of the subsoil while avoiding damage to the soil due to compaction and problems with excessive draft or dust (Bannan and Wrigley, 2013). It has been reported (Goldsmith et al., 2001) that plant growth is not hampered during beneficial slope stabilization for engineering works where the degree of compaction is 0.80–0.85. For the median MBD values of 1.59 g cm−3 and 1.54 g cm−3 obtained through our prediction model for the horizon A and horizon B, it means BD of 1.27–1.35 and 1.23–1.31 for the horizon A and horizon B for the median conditions of our coarse-textured soil.
Rippers can be designed to suit specific soil conditions (Godwin, 2007) and combined with cropping systems and tillage practices to avoid coalescence (Lampurlanés and Cantero-Martinez, 2003; Reintam et al., 2008). Cover crops such as ryegrass can enhance soil quality through root channeling and organic matter additions (Darby et al., 2014; McNally et al., 2015). The LME models can assess the requirement for amendments to rebalance soil compositions to increase soil resilience to compaction. Soil amendments are commonly applied in the form of animal manure, gypsum (SO4.2H2O), lime, and municipal waste from waste water treatment plants providing Al and Fe.
Gypsum can reduce (1) the harmful effect of Mg on the soil structure of fine-textured soils resulting from the higher ion hydration radius of Mg compared to Ca (Favaretto et al., 2006) and (2) the subsoil Al toxicity in acid coarse-textured soils resulting from Al neutralization by the sulfate ion (Noble et al., 1988; Sumner, 1993). Gypsum increased maize yield by 29–50% on sandy loams (Toma et al., 1999), not only modifying the soil [] balance but also improving plant rootability. The beneficial effects of gypsum combined with ripping may last up to 16 years (Toma et al., 1999).
There are also ways to alter the texture of surface soils, such as partially mixing upper and lower layers if other soil quality attributes, such as organic matter content, are not unduly affected. Soil texture can also be modified to increase the water storage capacity of coarse-textured soils by applying to deficient soils the residual fine soil materials adhering to potato tubers, collected in the fall before tuber storage.
Conclusions
Soil resistance to compaction and resilience can be diagnosed from soil components such as particle-size distribution, organic C fractions, cementing agents, and water content. However, current diagnostic guidelines do not integrate cementing agents that may influence coalescence, hence the frequency of corrective measures. In this paper, compaction indexes for coarse-textured agricultural soils were predicted from soil gravimetric water content, soil organic matter, mineral soil particles and mineral cementing agents. BD and DC were found to decrease with higher clay content, and to increase with higher proportions of coarser particles. Organic matter content tended to decrease the BD and DC of the coarse-textured soils. The balance involving organic matter showed little effects on MBD, whereas increasing evenness of sands fractions resulted in a higher MBD value. The relationships between CWC and soil texture and organic C were not close in the present study. Mineral cementing agents were major contributors to soil compaction indexes. The Si, Al and Fe oxides and Ca increased BD, DC and CWC but reduced MBD. The effect of soil organic matter depended on soil texture, and both organic matter and mineral cementing agents showed similar effects in soil compaction. Balances among components accounted for interactions among soil components.
The LME models explained 58–64% of total variation in BD and DC, and the regression models accounted for up to 83% of total variation in MBD and CWC. The most reliable models related soil composition to MBD and CWC. For a median coarse-textured soil of our soil samples, the estimated CWC obtained from our regression models was 205 g kg−1 for horizon A and 144 g kg−1 for horizon B. To minimize subsoil compaction in coarse-textured soils, soil gravimetric water content should be well below the CWC level at MBD estimated from the models.
Furthermore, the compositional balances discussed in this paper raise the question of how to rebuild soil structure by rebalancing the components. The diagnosis of compaction in coarse-textured soils could support decisions on implementing not only mechanical corrective means but also chemical, biological and physical methods to rebalance soil compositions using mineral and organic amendments, structure-building crops, and textural mixtures. The predictive models could be expanded to include soil C fractions and biological soil quality indexes to fully address the balance systems controlling soil resilience to compaction.
Author Contributions
YX and LP designed the study. NZ co-supervised the project. YX, ML, and MJ collected and analyzed soil samples. YX and SP analyzed the data. YX and LP wrote the manuscript. MJ, SP, ML, NZ reviewed the manuscript.
Funding
This project was supported financially by the Natural Sciences and Engineering Council of Canada (DG-2254, CRDPJ 385199–09, CRDPJ 469358–14), the International Council for Canadian Studies (MAJ fellowship), Centre Sève on plant productivity (FRQNT), and the following Canadian potato growers: Cultures Dolbec Inc., St-Ubalde, Quebec, Canada; Groupe Gosselin FG Inc., Pont Rouge, Quebec, Canada; Agriparmentier Inc. and Prochamps Inc., Notre-Dame-du-Bon-Conseil, Quebec, Canada; Ferme Daniel Bolduc et Fils Inc., Péribonka, Quebec, Canada.
Conflict of Interest Statement
The authors declare that this study received funding from Cultures Dolbec Inc.; Groupe Gosselin FG Inc.; Agriparmentier Inc.; Prochamps Inc.; Ferme Daniel Bolduc et Fils Inc. The funders were not involved in the study design or collection, analysis, or interpretation of the data.
Supplementary Material
The Supplementary Material for this article can be found online at: https://www.frontiersin.org/article/10.3389/fevo.2017.00083/full#supplementary-material
References
Aitchison, J. (1982). The statistical analysis of compositional data. J. R. Stat. Soc. Ser. B Methodol. 44, 139–177.
Aitchison, J., and Greenacre, M. (2002). Biplots of compositional data. J. R. Stat. Soc. Ser. C Appl. Stat. 51, 375–392. doi: 10.1111/1467-9876.00275
Alakukku, L., Weisskopf, P., Chamen, W. C. T., Tijink, F. G. J., Van Der Linden, J. P., Pires, S., et al. (2003). Prevention strategies for field traffic-induced subsoil compaction: a review: Part 1. Machine/soil interactions. Soil Tillage Res. 73, 145–160. doi: 10.1016/S0167-1987(03)00107-7
Andrén, O., and Kätterer, T. (1997). ICBM: the introductory carbon balance model for exploration of soil carbon balances. Ecol. Appl. 7, 1226–1236. doi: 10.1890/1051-0761(1997)007[1226:ITICBM]2.0.CO;2
Aragón, A., Garcıa, M. G., Filgueira, R. R., and Pachepsky, Y. A. (2000). Maximum compactibility of Argentine soils from the Proctor test: the relationship with organic carbon and water content. Soil Tillage Res. 56, 197–204. doi: 10.1016/S.0167-1987(00)00144-6
ASTM D1557 (2009). Standard Test Methods for Laboratory Compaction Characteristics of Soil Using Modified Effort. West Conshohocken, PA: ASTM.
Ball, B. C., Campbell, D. J., and Hunter, E. A. (2000). Soil compactibility in relation to physical and organic properties at 156 sites in UK. Soil Tillage Res. 57, 83–91. doi: 10.1016/S0167-1987(00)00145-8
Bannan, C., and Wrigley, R. (2013). Soil Amelioration in Acidic, Yellow Earths of the Western Australian Wheat belt with Sub-Surface Compaction and Acidity. Available online at: http://www.liebegroup.org.au/wp-content/uploads/2013/10/Christian-Bannan-Website.pdf
Benites, V. M., Machado, P. L., Fidalgo, E. C., Coelho, M. R., and Madari, B. E. (2007). Pedotransfer functions for estimating soil bulk density from existing soil survey reports in Brazil. Geoderma 139, 90–97. doi: 10.1016/j.geoderma.2007.01.005
Bennie, A. T. P., and Burger, R. T. (1988). Penetration resistance of fine sandy apedal soils as affected by relative bulk density, water content and texture. South Afr. J. Plant Soil 5, 5–10. doi: 10.1080/02571862.1988.10634239
Blake, G. R., and Hartge, K. H. (1986). “Bulk density,” in Methods of Soil Analysis. Part 1. Physical and Mineralogical Methods, ed A. Klute (Madison, WI: American Society of Agronomy, Inc.), 363–375.
Boiteau, G., Goyer, C., Rees, H. W., and Zebarth, B. J. (2014). Differentiation of potato ecosystems on the basis of relationships among physical, chemical and biological soil parameters. Can. J. Soil Sci. 94, 463–476. doi: 10.4141/cjss2013-095
Brahim, N., Bernoux, M., and Gallali, T. (2012). Pedotransfer functions to estimate soil bulk density for Northern Africa: Tunisia case. J. Arid. Environ. 81, 77–83. doi: 10.1016/j.jaridenv.2012.01.012
Bronick, C. J., and Lal, R. (2005). Soil structure and management: a review. Geoderma 124, 3–22. doi: 10.1016/j.geoderma.2004.03.005
Bruand, A., Hartmann, C., and Lesturgez, G. (2005). “Physical properties of tropical sandy soils: a large range of behaviours,” in Management of Tropical Sandy Soils for Sustainable Agriculture. A Holistic Approach for Sustainable Development of Problem Soils in the Tropics (Khon Kaen). Available online at: https://hal-insu.archivesouvertes.fr/file/index/docid/79666/filename/Bruand_keynote_paper.pdf
Burnham, K. P., and Anderson, D. R. (2003). Model Selection and Multimodel Inference: a Practical Information-Theoretic Approach. New York, NY: Springer Science & Business Media.
Burnham, K. P., Anderson, D. R., and Huyvaert, K. P. (2011). AIC model selection and multimodel inference in behavioral ecology: some background, observations, and comparisons. Behav. Ecol. Sociobiol. 65, 23–35. doi: 10.1007/s00265-010-1029-6
Calhoun, F. G., Smeck, N. E., Slater, B. L., Bigham, J. M., and Hall, G. F. (2001). Predicting bulk density of Ohio soils from morphology, genetic principles, and laboratory characterization data. Soil Sci. Soc. Am. J. 65, 811–819. doi: 10.2136/sssaj2001.653811x
Courchesne, F., and Turmel, M.-C. (2007). “Extractable Al, Fe, Mn, and Si,” in Soil Sampling and Methods of Analysis, 2nd Edn., eds M. R. Carter and E. G. Gregorich (Boca Raton, FL: CRC Press), 307–315.
Darby, H., Harwood, H., and Monahan, S. (2014). Corn Interseeding Trial. Available online at: http://www.uvm.edu/extension/cropsoil/wp-content/uploads/2013-Corn-Interseeding-Trial.pdf
da Silva, A. P., Kay, B. D., and Perfect, E. (1997). Management versus inherent soil properties effects on bulk density and relative compaction. Soil Tillage Res. 44, 81–93. doi: 10.1016/S0167-1987(97)00044-5
De Vos, B., Van Meirvenne, M., Quataert, P., Deckers, J., and Muys, B. (2005). Predictive quality of pedotransfer functions for estimating bulk density of forest soils. Soil Sci. Soc. Am. J. 69, 500–510. doi: 10.2136/sssaj2005.0500
Dexter, A. R., Richard, G., Arrouays, D., Czyż, E. A., Jolivet, C., and Duval, O. (2008). Complexed organic matter controls soil physical properties. Geoderma 144, 620–627. doi: 10.1016/j.geoderma.2008.01.022
Doran, J. W., and Parkin, T. B. (1996). Quantitative indicators of soil quality: a minimum data set. SSSA Spec. Publ. 49, 25–38. doi: 10.2136/sssaspecpub49.c2
Duiker, S. W., and Curran, W. S. (2004). “Rye cover crop management in corn,” in Tillage Conference for Sustainable Agriculture (Raleigh), 208.
Duiker, S. W., Rhoton, F. E., Torrent, J., Smeck, N. E., and Lal, R. (2003). Iron (hydr) oxide crystallinity effects on soil aggregation. Soil Sci. Soc. Am. J. 67, 606–611. doi: 10.2136/sssaj2003.6060
Egozcue, J. J., and Pawlowsky-Glahn, V. (2006). Simplicial geometry for compositional data. Geol. Soc. Lond. Spec. Publ. 264, 145–159. doi: 10.1144/GSL.SP.2006.264.01.11
Egozcue, J. J., Pawlowsky-Glahn, V., Mateu-Figueras, G., and Barcelo-Vidal, C. (2003). Isometric logratio transformations for compositional data analysis. Math. Geol. 35, 279–300. doi: 10.1023/A:1023818214614
Ekwue, E. I., and Stone, R. J. (1995). Organic matter effects on the strength properties of compacted agricultural soils. Trans. ASAE 38, 357–365. doi: 10.13031/2013.27804
Favaretto, N., Norton, L. D., Joern, B. C., and Brouder, S. M. (2006). Gypsum amendment and exchangeable calcium and magnesium affecting phosphorus and nitrogen in runoff. Soil Sci. Soc. Am. J. 70, 1788–1796. doi: 10.2136/sssaj2005.0228
Felton, G. K., and Ali, M. (1992). Hydraulic parameter response to incorporated organic matter in the B-horizon. Trans. ASAE 35, 1153–1160. doi: 10.13031/2013.28713
Filzmoser, P., and Gschwandtner, M. (2015). “mvoutlier”: Multivariate Outlier Detection Based on Robust Methods. Available online at: https://cran.r-project.org/web/packages/mvoutlier/index.html
Filzmoser, P., Hron, K., and Reimann, C. (2009). Univariate statistical analysis of environmental (compositional) data: problems and possibilities. Sci. Total Environ. 407, 6100–6108. doi: 10.1016/j.scitotenv.2009.08.008
Frye, W. W., Smith, W. G., and Williams, R. J. (1985). Economics of winter cover crops as a source of nitrogen for no-till corn. J. Soil Water Conserv. 40, 246–248.
Godwin, R. J. (2007). A review of the effect of implement geometry on soil failure and implement forces. Soil Tillage Res. 97, 331–340. doi: 10.1016/j.still.2006.06.010
Goldsmith, W., Silva, M., and Fischenich, C. (2001). Determining Optimal Degree of Soil Compaction for Balancing Mechanical Stability and Plant Growth Capacity. DTIC Document.
Grossman, R. B., and Carlisle, F. J. (1969). Fragipan soils of the eastern United States. Adv. Agron. 21, 237–279. doi: 10.1016/S0065-2113(08)60099-1
Guerrero, A. M. A. (2004). Effects of the Soil Properties on the Maximum Dry Density Obtained from the Standard Proctor Test. thesis, Orlando, FL: University of Central Florida.
Gupta, S. C., and Allmaras, R. R. (1987). “Models to assess the susceptibility of soils to excessive compaction,” in Advances in Soil Science, ed N. C. Brady (New York, NY: Springer), 65–100.
Håkansson, I. (1990). A method for characterizing the state of compactness of the plough layer. Soil Tillage Res. 16, 105–120. doi: 10.1016/0167-1987(90)90024-8
Håkansson, I. (2005). Machinery-Induced Compaction of Arable Soils. Incidence—Consequences—Countermeasures. Swedish University of Agricultural Sciences, Department of Soil Sciences.
Håkansson, I., and Lipiec, J. (2000). A review of the usefulness of relative bulk density values in studies of soil structure and compaction. Soil Tillage Res. 53, 71–85. doi: 10.1016/S0167-1987(99)00095-1
Hamza, M. A., and Anderson, W. K. (2005). Soil compaction in cropping systems: a review of the nature, causes and possible solutions. Soil Tillage Res. 82, 121–145. doi: 10.1016/j.still.2004.08.009
Haynes, R. J., and Naidu, R. (1998). Influence of lime, fertilizer and manure applications on soil organic matter content and soil physical conditions: a review. Nutr. Cycl. Agroecosyst. 51, 123–137. doi: 10.1023/A:1009738307837
Heuscher, S. A., Brandt, C. C., and Jardine, P. M. (2005). Using soil physical and chemical properties to estimate bulk density. Soil Sci. Soc. Am. J. 69, 51–56. doi: 10.2136/sssaj2005.0051
Jalabert, S. S. M., Martin, M. P., Renaud, J.-P., Boulonne, L., Jolivet, C., Montanarella, L., et al. (2010). Estimating forest soil bulk density using boosted regression modelling. Soil Use Manag. 26, 516–528. doi: 10.1111/j.1475-2743.2010.00305.x
Jones, R. J., Spoor, G., and Thomasson, A. J. (2003). Vulnerability of subsoils in Europe to compaction: a preliminary analysis. Soil Tillage Res. 73, 131–143. doi: 10.1016/S0167-1987(03)00106-5
Kaur, R., Kumar, S., and Gurung, H. P. (2002). A pedo-transfer function (PTF) for estimating soil bulk density from basic soil data and its comparison with existing PTFs. Soil Res. 40, 847–858. doi: 10.1071/SR01023
Kroetsch, D., and Wang, C. (2007). “Particle Size distribution,” in Soil Sampling and Methods of Analysis, 2nd Edn., eds M. R. Carter and E. G. Gregorich (Boca Raton, FL: CRC Press).
Lampurlanés, J., and Cantero-Martinez, C. (2003). Soil bulk density and penetration resistance under different tillage and crop management systems and their relationship with barley root growth. Agron. J. 95, 526–536. doi: 10.2134/agronj2003.0526
Lipiec, J., and Hatano, R. (2003). Quantification of compaction effects on soil physical properties and crop growth. Geoderma 116, 107–136. doi: 10.1016/S0016-7061(03)00097-1
Manrique, L. A., and Jones, C. A. (1991). Bulk density of soils in relation to soil physical and chemical properties. Soil Sci. Soc. Am. J. 55, 476–481. doi: 10.2136/sssaj1991.03615995005500020030x
Martin, M. P., Lo Seen, D., Boulonne, L., Jolivet, C., Nair, K. M., Bourgeon, G., et al. (2009). Optimizing pedotransfer functions for estimating soil bulk density using boosted regression trees. Soil Sci. Soc. Am. J. 73, 485–493. doi: 10.2136/sssaj2007.0241
McKeague, J. A., and Sprout, P. N. (1975). Cemented subsoils (duric horizons) in some soils of British Columbia. Can. J. Soil Sci. 55, 189–203. doi: 10.4141/cjss75-027
McNally, S. R., Laughlin, D. C., Rutledge, S., Dodd, M. B., Six, J., and Schipper, L. A. (2015). Root carbon inputs under moderately diverse sward and conventional ryegrass-clover pasture: implications for soil carbon sequestration. Plant Soil 392, 289–299. doi: 10.1007/s11104-015-2463-z
Moolman, J. H., and Weber, H. W. (1978). 'n Ondersoek na die bydrae van die fynsandfraksie tot die verdigbaarheid van fynsandgronde in Suid-Kaapland. Agrochemophysica 10, 39–46.
Mujtaba, H., Farooq, K., and Rashid, I. (2014). Experimental Investigation on compaction properties of sandy soils. Pak. J. Engg. Appl. Sci. 14, 115–125.
Nhantumbo, A. B., and Cambule, A. H. (2006). Bulk density by proctor test as a function of texture for agricultural soils in Maputo province of Mozambique. Soil Tillage Res. 87, 231–239. doi: 10.1016/j.still.2005.04.001
Noble, A. D., Sumner, M. E., and Alva, A. K. (1988). The pH dependency of aluminum phytotoxicity alleviation by calcium sulfate. Soil Sci. Soc. Am. J. 52, 1398–1402. doi: 10.2136/sssaj1988.03615995005200050036x
Pagé, F., and Berrier, J. (1983). Composition du matériel liant dans des horizons à ortstein, duriques, fragiques et cimentés intergrades du Québec. Can. J. Soil Sci. 63, 435–453. doi: 10.4141/cjss83-045
Parent, L. E., de Almeida, C. X., Hernandes, A., Egozcue, J. J., Gülser, C., Bolinder, M. A., et al. (2012). Compositional analysis for an unbiased measure of soil aggregation. Geoderma 179–180, 123–131. doi: 10.1016/j.geoderma.2012.02.022
Parent, L. E., Parent, S.-É., Hébert-Gentile, V., Naess, K., and Lapointe, L. (2013). Mineral balance plasticity of cloudberry (Rubus chamaemorus) in Quebec-Labrador bogs. Am. J. Plant Sci. 4, 1508–1520. doi: 10.4236/ajps.2013.47183
Parent, S.-É., Parent, L. E., Egozcue, J. J., Rozane, D.-E., Hernandes, A., Lapointe, L., et al. (2013). The plant ionome revisited by the nutrient balance concept. Front. Plant Sci. 4:39. doi: 10.3389/fpls.2013.00039
Quiroga, A. R., Buschiazzo, D. E., and Peinemann, N. (1999). Soil compaction is related to management practices in the semi-arid Argentine pampas. Soil Tillage Res. 52, 21–28. doi: 10.1016/S0167-1987(99)00049-5
Reichert, J. M., Reinert, D. J., and Braida, J. A. (2003). Qualidade dos solos e sustentabilidade de sistemas agrícolas. Ci. Amb. 27, 29–48.
Reichert, J. M., Suzuki, L. E. A. S., Reinert, D. J., Horn, R., and Håkansson, I. (2009). Reference bulk density and critical degree-of-compactness for no-till crop production in subtropical highly weathered soils. Soil Tillage Res. 102, 242–254. doi: 10.1016/j.still.2008.07.002
Reintam, E., Trükmann, K., and Kuht, J. (2008). Effect of Cirsium arvense L. on soil physical properties and crop growth. Agric. Food Sci. 17, 153–164. doi: 10.2137/145960608785328206
Revelle, W. (2014). “psych”: Procedures for Psychological, Psychometric, and Personality Research. Evanston, IL: Northwestern University.
Rumpel, C., and Kögel-Knabner, I. (2011). Deep soil organic matter—a key but poorly understood component of terrestrial C cycle. Plant Soil 338, 143–158. doi: 10.1007/s11104-010-0391-5
Soil Survey Staff (2014). Keys to Soil Taxonomy, 12th Edn. Washington, DC: USDA, National Resources Conservation Service.
Sanborn, P., Lamontagne, L., and Hendershot, W. (2011). Podzolic soils of Canada: Genesis, distribution, and classification. Can. J. Soil Sci. 91, 843–880. doi: 10.4141/cjss10024
Six, J., Callewaert, P., Lenders, S., De Gryze, S., Morris, S. J., Gregorich, E. G., et al. (2002). Measuring and understanding carbon storage in afforested soils by physical fractionation. Soil Sci. Soc. Am. J. 66, 1981–1987. doi: 10.2136/sssaj2002.1981
Smith, C. W., Johnston, M. A., and Lorentz, S. (1997). Assessing the compaction susceptibility of South African forestry soils. II. Soil properties affecting compactibility and compressibility. Soil Tillage Res. 43, 335–354. doi: 10.1016/S0167-1987(97)00023-8
Soane, B. D. (1990). The role of organic matter in soil compactibility: a review of some practical aspects. Soil Tillage Res. 16, 179–201. doi: 10.1016/0167-1987(90)90029-D
Soane, B. D., Campbell, D. J., and Herkes, S. M. (1972). The characterization of some Scottish arable topsoils by agricultural and engineering methods. J. Soil Sci. 23, 93–104. doi: 10.1111/j.1365-2389.1972.tb01645.x
Spoor, G., Tijink, F. G. J., and Weisskopf, P. (2003). Subsoil compaction: risk, avoidance, identification and alleviation. Soil Tillage Res. 73, 175–182. doi: 10.1016/S0167-1987(03)00109-0
Stalham, M. A., Allen, E. J., and Herry, F. X. (2005). Effects of Soil Compaction on Potato Growth and its Removal by Cultivation. Oxford: Research review R260 British Potato Council. Available online at: http://www.potato.org.uk/sites/default/files/publication_upload/R260%20ADAS%20Soil%20Compaction%20Review.pdf
Stewart, C. E., Plante, A. F., Paustian, K., Conant, R. T., and Six, J. (2008). Soil carbon saturation: linking concept and measurable carbon pools. Soil Sci. Soc. Am. J. 72, 379–392. doi: 10.2136/sssaj2007.0104
Sumner, M. E. (1993). Gypsum and acid soils: the world scene. Adv. Agron. U.S.A. 51, 1–32. doi: 10.1016/s0065-2113(08)60589-1
Suuster, E., Ritz, C., Roostalu, H., Reintam, E., Kõlli, R., and Astover, A. (2011). Soil bulk density pedotransfer functions of the humus horizon in arable soils. Geoderma 163, 74–82. doi: 10.1016/j.geoderma.2011.04.005
Symonds, M. R., and Moussalli, A. (2011). A brief guide to model selection, multimodel inference and model averaging in behavioural ecology using Akaike's information criterion. Behav. Ecol. Sociobiol. 65, 13–21. doi: 10.1007/s00265-010-1037-6
Thomas, G. W., Haszler, G. R., and Blevins, R. L. (1996). The effects of organic matter and tillage on maximum compactability of soils using the proctor test. Soil Sci. 161, 502–508. doi: 10.1097/00010694-199608000-00005
Toma, M., Sumner, M. E., Weeks, G., and Saigusa, M. (1999). Long-term effects of gypsum on crop yield and subsoil chemical properties. Soil Sci. Soc. Am. J. 63, 891–895. doi: 10.2136/sssaj1999.634891x
Tong, X., Xu, M., Wang, X., Bhattacharyya, R., Zhang, W., and Cong, R. (2014). Long-term fertilization effects on organic carbon fractions in a red soil of China. Catena 113, 251–259. doi: 10.1016/j.catena.2013.08.005
Topp, G. C., Parkin, G. W., and Ferre, T. P. A. (2007). “Soil water content,” in Soil Sampling and Methods of Analysis, 2nd Edn., eds M. R. Carter and E. G. Gregorich (Boca Raton, FL: CRC Press).
Tranter, G., Minasny, B., McBratney, A. B., Murphy, B., McKenzie, N. J., Grundy, M., et al. (2007). Building and testing conceptual and empirical models for predicting soil bulk density. Soil Use Manag. 23, 437–443. doi: 10.1111/j.1475-2743.2007.00092.x
Tschapek, M. (1984). Criteria for Determining the hydrophilicity-hydrophobicity of Soils. Z. Für Pflanzenernähr. Bodenkd. 147, 137–149. doi: 10.1002/jpln.19841470202
Van Den Boogaart, K. G., and Tolosana-Delgado, R. (2006). Compositional data analysis with Rand the package compositions. Geol. Soc. Lond. Spec. Publ. 264, 119–127. doi: 10.1144/GSL.SP.2006.264.01.09
van den Boogaart, K. G., Tolosana-Delgado, R., and Bren, M. (2014). “Compositions”: Compositional Data Analysis in R Package. Available online at: https://cran.r-project.org/web/packages/compositions/index.html
Van Der Watt, H. V. H. (1969). Influence of particle size distribution on soil compactibility. Agrochemophysica 1, 79–86.
Wagner, L. E., Ambe, N. M., and Ding, D. (1994). Estimating a Proctor density curve from intrinsic soil properties. Trans. ASAE 37, 1121–1125. doi: 10.13031/2013.28185
Wickham, H., and Francois, R. (2015). “dplyr”: A Grammar of Data Manipulation. Available online at: https://cran.r-project.org/web/packages/dplyr/index.html
Wolkowski, R., and Lowery, B. (2008). Soil Compaction: Causes, Concerns and Cures. Division of Cooperative Extension of the University of Wisconsin Extension A3367. Available online at: http://www.soils.wisc.edu/extension/pubs/A3367.pdf
Yang, X. M., Xie, H. T., Drury, C. F., Reynolds, W. D., Yang, J. Y., and Zhang, X. D. (2012). Determination of organic carbon and nitrogen in particulate organic matter and particle size fractions of Brookston clay loam soil using infrared spectroscopy. Eur. J. Soil Sci. 63, 177–188. doi: 10.1111/j.1365-2389.2011.01421.x
Yee, C. S., and Harr, R. D. (1977). Influence of soil aggregation on slope stability in the Oregon Coast Ranges. Environ. Geol. 1, 367–377. doi: 10.1007/BF02380505
Zhang, H., Hartge, K. H., and Ringe, H. (1997). Effectiveness of organic matter incorporation in reducing soil compactibility. Soil Sci. Soc. Am. J. 61, 239–245. doi: 10.2136/sssaj1997.03615995006100010033x
Zhao, Y., Krzic, M., Bulmer, C. E., and Schmidt, M. G. (2008). Maximum bulk density of British Columbia forest soils from the proctor test: relationships with selected physical and chemical properties. Soil Sci. Soc. Am. J. 72, 442–452. doi: 10.2136/sssaj2007.0075
Keywords: cementing agents, compositional data analysis, soil compaction, soil texture, prediction models, Proctor test
Citation: Xu Y, Jimenez MA, Parent S-É, Leblanc M, Ziadi N and Parent LE (2017) Compaction of Coarse-Textured Soils: Balance Models across Mineral and Organic Compositions. Front. Ecol. Evol. 5:83. doi: 10.3389/fevo.2017.00083
Received: 13 April 2017; Accepted: 11 July 2017;
Published: 28 July 2017.
Edited by:
Urs Feller, University of Bern, SwitzerlandCopyright © 2017 Xu, Jimenez, Parent, Leblanc, Ziadi and Parent. This is an open-access article distributed under the terms of the Creative Commons Attribution License (CC BY). The use, distribution or reproduction in other forums is permitted, provided the original author(s) or licensor are credited and that the original publication in this journal is cited, in accordance with accepted academic practice. No use, distribution or reproduction is permitted which does not comply with these terms.
*Correspondence: Léon E. Parent, leon-etienne.parent@fsaa.ulaval.ca