Ecology of Human Medical Enterprises: From Disease Ecology of Zoonoses, Cancer Ecology Through to Medical Ecology of Human Microbiomes
- 1Computational Biology and Medical Ecology Lab, State Key Laboratory of Genetic Resources and Evolution, Kunming Institute of Zoology, Chinese Academy of Sciences, Kunming, China
- 2Center for Excellence in Animal Evolution and Genetics, Chinese Academy of Sciences, Kunming, China
- 3Molecular Evolution and Genome Diversity Lab, State Key Laboratory of Genetic Resources and Evolution, Kunming Institute of Zoology, Chinese Academy of Sciences, Kunming, China
In nature, the interaction between pathogens and their hosts is only one of a handful of interaction relationships between species, including parasitism, predation, competition, symbiosis, commensalism, and among others. From a non-anthropocentric view, parasitism has relatively fewer essential differences from the other relationships; but from an anthropocentric view, parasitism and predation against humans and their well-beings and belongings are frequently related to heinous diseases. Specifically, treating (managing) diseases of humans, crops and forests, pets, livestock, and wildlife constitute the so-termed medical enterprises (sciences and technologies) humans endeavor in biomedicine and clinical medicine, veterinary, plant protection, and wildlife conservation. In recent years, the significance of ecological science to medicines has received rising attentions, and the emergence and pandemic of COVID-19 appear accelerating the trend. The facts that diseases are simply one of the fundamental ecological relationships in nature, and the study of the relationships between species and their environment is a core mission of ecology highlight the critical importance of ecological science. Nevertheless, current studies on the ecology of medical enterprises are highly fragmented. Here, we (i) conceptually overview the fields of disease ecology of wildlife, cancer ecology and evolution, medical ecology of human microbiome-associated diseases and infectious diseases, and integrated pest management of crops and forests, across major medical enterprises. (ii) Explore the necessity and feasibility for a unified medical ecology that spans biomedicine, clinical medicine, veterinary, crop (forest and wildlife) protection, and biodiversity conservation. (iii) Suggest that a unified medical ecology of human diseases is both necessary and feasible, but laissez-faire terminologies in other human medical enterprises may be preferred. (iv) Suggest that the evo-eco paradigm for cancer research can play a similar role of evo-devo in evolutionary developmental biology. (v) Summarized 40 key ecological principles/theories in current disease-, cancer-, and medical-ecology literatures. (vi) Identified key cross-disciplinary discovery fields for medical/disease ecology in coming decade including bioinformatics and computational ecology, single cell ecology, theoretical ecology, complexity science, and the integrated studies of ecology and evolution. Finally, deep understanding of medical ecology is of obvious importance for the safety of human beings and perhaps for all living things on the planet.
Introduction
Where we came from, who we are, and where we are going have been explored since the existence of recorded history. Although most of us ignore various doomsday predictions about our planet and species, plus nature mother is often benign, it is an undisputed fact that nature can be a horrific enemy occasionally (McGuire, 2002). Humans have been fighting somewhat recurring battles against the results of its capriciousness—severe floods and storms, devastating earthquakes, cataclysmic volcanic eruptions (McGuire, 2002), and disease pandemics such as Justinian plague in year 541, which was estimated to have killed then half of world population (Morens and Fauci, 2020). The extinction of dinosaurs and 2/3 extant species at the Cretaceous period 65 million years ago reminds us that human race may exist and thrive only by geological accident and may be within a hair’s breadth of extinction, if the hypothesis of asteroid struck turns out to be true (McGuire, 2002). A recent report by Hyndman et al. (2018) suggests the protection obtained through the integration of bornaviruses into the genomes of Cretaceous-era mammals may have given them an advantage over reptiles as the predominant terrestrial vertebrates after dinosaurs went extinct. The integrated bornavirus genes, known as “endogenous bornaviral-like elements” (EBLs), which was lacking in birds and reptiles, granted mammals a level of protection against bornaviruses. As a side note, this advantage granted by EBLs is somewhat similar to the work principle of mRNA vaccine (against the COVID-19 infections). Of course, there is a fundamental difference between mRNA vaccine and EBLs. In the case of mRNA vaccine, the mRNA is not inserted into the human genome and is not inheritable. Enard and Petrov (2020), through reanalysis of the 1,000-genomes project data, detected approximately 4,500 host loci that may have preserved the footprints left by ancient viral epidemics in the past 50,000 years. Their findings suggest that RNA viruses have exerted significantly stronger selective pressures than DNA viruses across diverse human populations, also highlighting the more important zoonotic potential of RNA viruses.
SARS-CoV-2 is the gravest microbial threats to humans in the 21st century to date. The COVID-19 pandemic is obviously a stunning wakeup call that forces us to adapt, react, and reconsider the nature of our relationship with the natural world, and emerging and re-emerging infectious diseases such as COVID-19 are epiphenomena of human existence and our interactions with each other, and with natural world (Morens and Fauci, 2020). In the Anthropocene epoch, human activities have been frequently aggressive, damaging, and unbalanced interactions with nature, which creates an endless variety of opportunities for genetically unstable infectious agents (such as corona-viruses that are extremely easy to mutate) to spillover to the “unfilled” ecologic niches such as those created by biodiversity loss and climate changes (Cardinale et al., 2012; Faust et al., 2018; Johnson et al., 2020; Morens and Fauci, 2020). Without enacting essential adaptations, humans may increasingly trigger new disease emergences and remain at risk for the foreseeable future (Morens and Fauci, 2020).
Bernardo-Cravo et al. (2020) presented a pyramid model (diagram) for illustrating the manifold interactions among host, host microbiome, pathogens and the environment, which has one more component, human microbiome, than Morens and Fauci (2020) model. Figure 1 exhibited a slightly revised version of Fauci-Morens-Bernardo-Cravo model (termed FMB model hereafter) that highlights the key threads of medicine, ecology and environment. Bernardo-Cravo et al. (2020) argued that the tendency of host-disease risk or susceptibility is generally determined by his or her resistance and tolerance to pathogens, pathogen permeability of the host microbiome, pathogenicity (as determined by pathogen infectivity and virulence), as well as by environment (Figure 1). The severity of a disease may range from asymptomatic to fatality. The far-reaching influences of environmental factors, including various anthropogenic impacts such as pollution, climate change, and land use (i.e., deforestation, urbanization, and agricultural intensification) on our health and diseases have been receiving public attentions increasingly (Patz et al., 2005; Schmeller et al., 2020). We argue that most of the environmental factors, especially those caused by human activities, can lead to biodiversity loss that in turn may have significant influences on the risks of emerging diseases, particularly the spillover of zoonoses to humans, and on our susceptibility to diseases. In other words, pandemic disease emergence is likely determined by dynamic equilibriums of complex globally distributed ecosystems consisting of animals, pathogens, humans, and the environment (Cardinale et al., 2012; Newbold et al., 2015, 2018; Plowright et al., 2017; Rohr et al., 2020). Biodiversity conservation, which can be defined as “preserving functioning ecosystems with predominantly native species” (Rohr et al., 2020), is therefore of critical importance for us to fight against the emerging pandemics such as ongoing COVID-19.
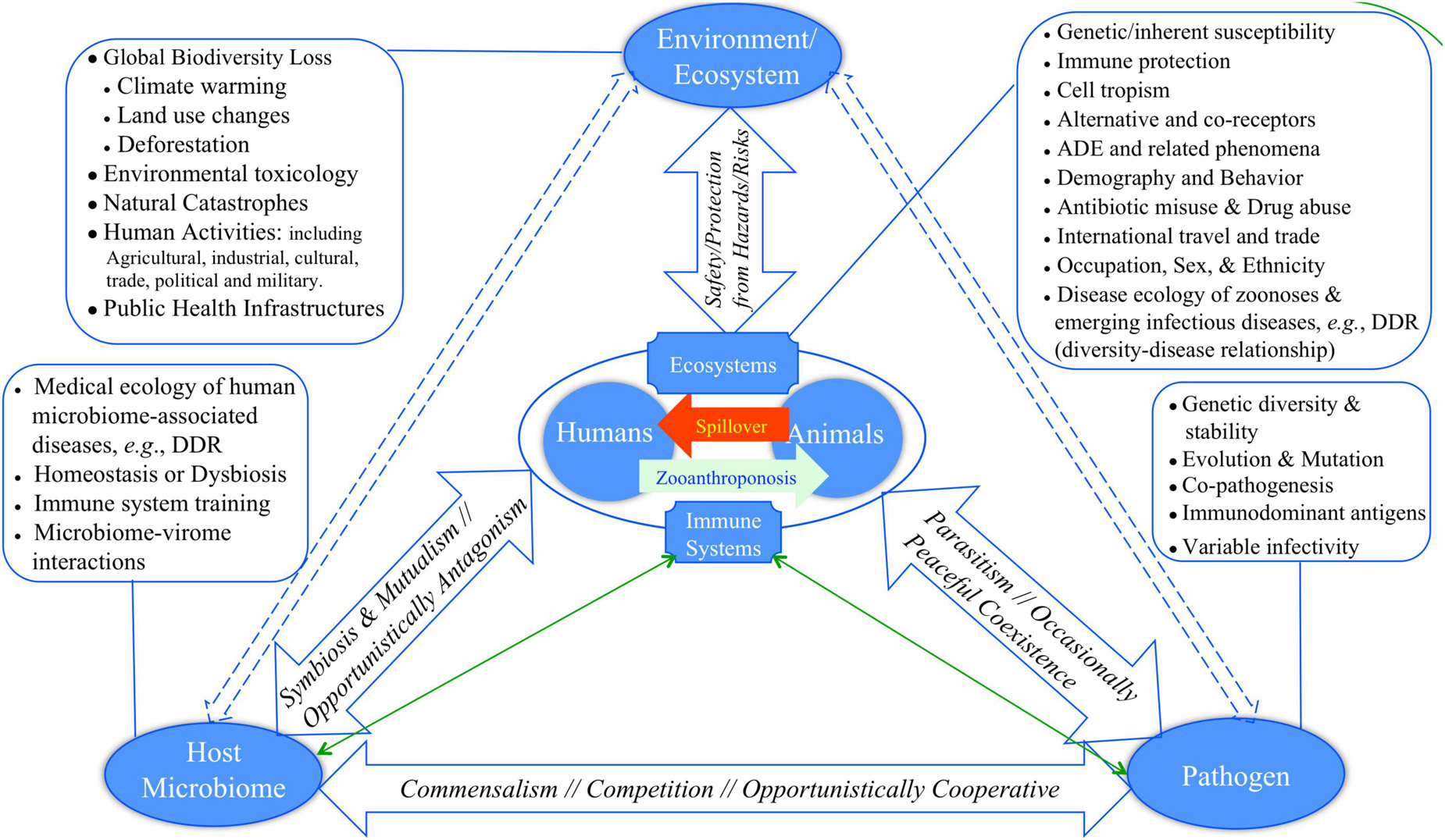
Figure 1. Revised FMB (Fauci-Morens-Bernardo-Cravo) pyramid model of human diseases for describing the four-way interactions between host, host microbiome, pathogen, and environment (Fauci and Morens, 2012; Bernardo-Cravo et al., 2020; Morens and Fauci, 2020): (i) The relationship between host and his or her microbiome may not be monotonically competitive; for example, host microbiome can be refuge camps for opportunistic pathogens, and the microbiome may lose suppressive effects to opportunistic pathogen when itself enters alternative dysbiosis states. (ii) Similarly, the relationship between host and host microbiome can be complicated, could even be frenemy relationship; for example, diseases such as BV (bacterial vaginosis) may be caused by the dysbiosis of vaginal microbiome, and there may not be a formally identified BV pathogen. On the other hand, host physiology and immunology can significant impact the ecology and physiology of microbiomes, and therefore their relationship are bidirectional. In fact, the triangle relationship in the floor of the pyramid should by cyclic, and perhaps the cyclic nature is a norm in all facets of the pyramid.
Broadly speaking, medical enterprises humans involve are not limited to the diseases of humans and animals that are briefly touched previously. One obvious missing block is the diseases of plants, particularly of crops and forests, from which humans get food and major ecosystem services such as timbers, habitats for wildlife, conservation of soil erosion, prevention of desertification and watershed preservation and stable rainfall and climate. Figure 2 summarizes the major components of medical enterprises we discuss in this article, from disease and/or insect pests of plants (crops and forests), animals (livestock and wildlife), and humans. Obviously, the medical enterprises span many facets of science, technology, socioeconomics, and humanity (e.g., Dobson et al., 2020). In the present article, our focus is exclusively on ecological facet, the justifications of which are briefly explained below.
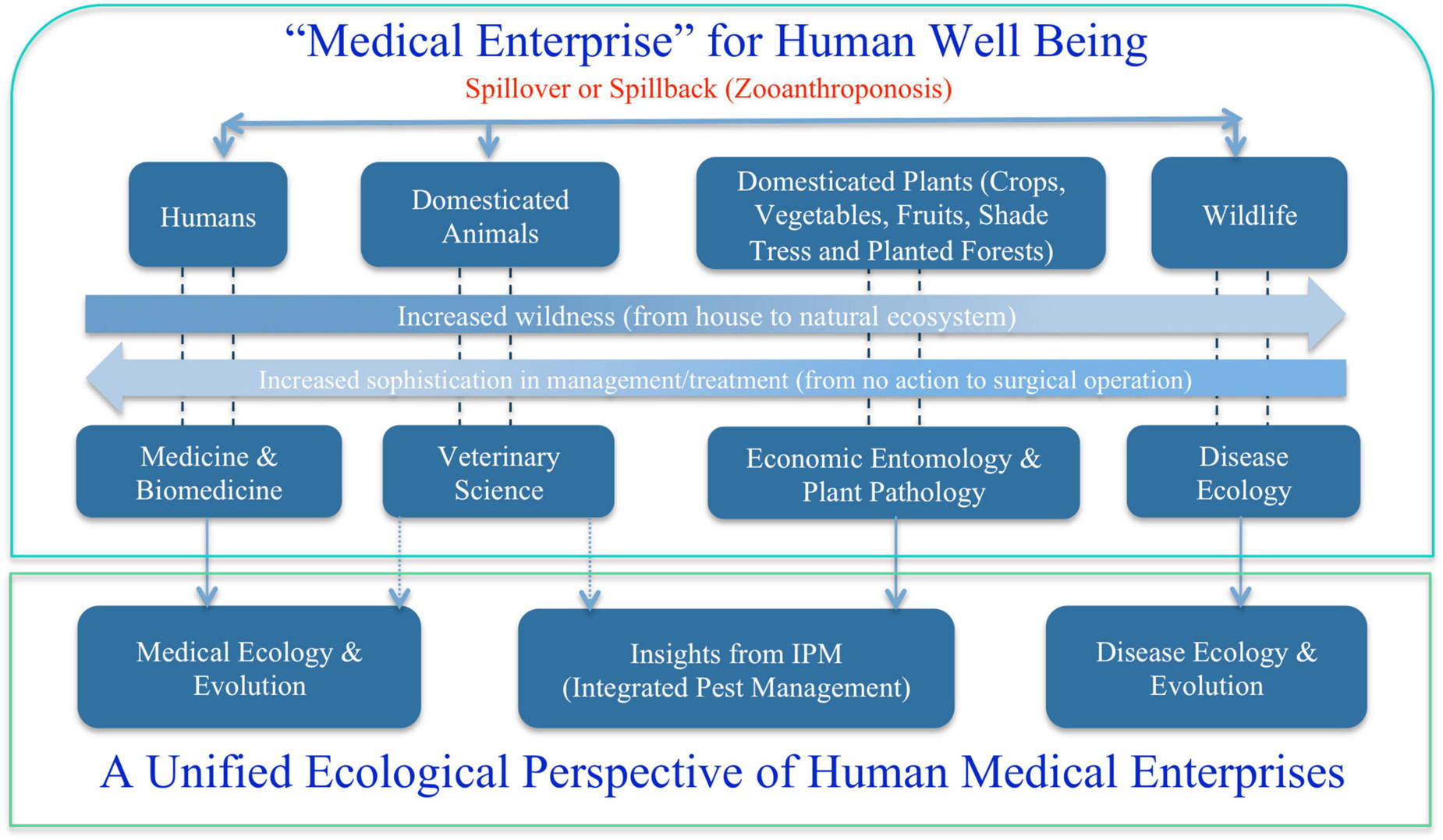
Figure 2. A unified ecological perspective of the human medical enterprises (sciences and technologies): three fields or subjects—medical ecology for human diseases, integrated pest management (IPM) for diseases and insect pests of crops and forests, and disease ecology of wildlife.
The ecological perspective of “One Health” is a strategy for tackling diseases, which takes into accounts all components and factors that may cause or raise risk of disease, and in which properties of human, environmental, and animal health are assessed in a unified manner to detect, understand, and solve public health problems (Ellwanger et al., 2020). Those components/factors may include environmental and ecological/wildlife, as well as domestic animal and human. The human factors also cover behavioral and medical issues, such as cultural, political and other socio-economic drivers that can influence disease spread and epidemics (Lloyd-Smith et al., 2005, 2009; Luis et al., 2015, 2018; Cunningham et al., 2017; Ellwanger et al., 2020). The devastating impacts of zoonoses on human health and wellbeing have been vividly demonstrating by the current COVID-19 pandemic, which is currently classified as emerging infectious disease (EID) with probable animal origin. Identifying the possible zoonotic emergence and the exact mechanisms responsible for its initial transmission can play a critical role in designing and implementing suitable preventive barriers against the further transmission of SARS-CoV-2. In consideration of the strong interrelatedness among animals, humans, and environment, prioritized research focus on one-health approach is likely to identify critical intervention steps in the transmission of zoonotic viruses (Gibb et al., 2020a,b; Latinne et al., 2020; Rahman et al., 2020; Tiwari et al., 2020).
The “One Health” strategy has been playing a critical role in investigating and dealing with emerging and reemerging infectious diseases, and it is undoubtedly one of the most successful ecological frameworks for dealing with emerging and reemerging infectious diseases. Since there are already many excellent reviews on “One Health” strategy, we are not going to further discuss it in this article. Different from the One Health strategy, in this article, we further broaden the scope of our discussion to virtually all major medical enterprises aimed to protect human well-beings from diseases of plants, livestock, wildlife, and ourselves. Furthermore, we focus on theoretical ecology foundations for diseases and supporting fields such as genomics, metagenomics, bioinformatics, and computational biology.
Ecology is not only relevant but also critical to virtually all major aspects of medical enterprises (sciences and technologies) illustrated in Figure 2. This is because pathogen, the causing agent of disease, is not an isolated entity; instead, pathogen interacts with its host and constitutes an ecosystem, not to mention that both pathogen and host are influenced by their environment. Ecosystem and environment are the very entities that ecology investigates. In fact, pathogen and host, and possible vector organism that introduces pathogen to host, frequently constitute the core of the host-pathogen ecosystem. As shown in Figure 2, at least three ecological subjects: disease ecology, IPM and medical ecology, are associated with medical enterprises of human well beings. The remainder of this reviewer is organized as four sections: (i) disease ecology of plants; (ii) disease ecology of animals; (iii) cancer ecology; (iv) ecology of human microbiome associated diseases; and (v) proposal toward a unified medical ecology of human diseases.
Disease Ecology of Plants—The Integrated Pest Management
Before discussing the emerging/reemerging infectious diseases of animals and humans, we first discuss the diseases of plants. A simplified view of diseases is the diseased states of hosts caused by pathogens, which are usually microbes (bacteria, fungal, viruses, etc.) but insect pests, nematodes, mites as “pathogen” for plants (crops and forests) are obviously the largest exception. Here, we first discuss the disease ecology of plants, i.e., the integrated pest management (IPM).
The disease ecology can be defined as “the ecological study of host-pathogen interactions within the context of their environment and evolution” (Kilpatrick and Altizer, 2010). Broadly speaking, the origin of disease ecology can be traced back to the 1930s when entomologists studied the insect-parasitoid dynamics, known as Nicholson–Bailey model, which can be considered as difference equation version of the classic Lotka–Volterra differential equations for modeling species interactions (predator-prey, competition, etc.). The latter was proposed by mathematician and physicist Alfred Lotka and Vito Volterra during 1910s–1920s and their work set the foundation for theoretical (mathematical) ecology (Kingsland, 1995). Therefore, it can be seen that from the very beginning, theoretical (mathematical) ecology has been closely associated with the study of disease pathogen, although initially it was about the disease of animals and plants. The field took off in the 1950s and 1960s when population ecologists, entomologists, and plant pathologists were engaged in hot debates on the mechanisms of population regulation in nature. Theoretically, the debates helped to expand the breadth of theoretical ecology, and somewhat “ironically” shifted the focus of ecology away from population ecology, because the debates pushed ecologists and mathematicians to recognize that the mechanisms of population regulation and closely related themes such as population (ecosystem) stability, species extinctions could hardly be understood without looking into beyond species interactions. Those debates culminated at annual Cold Spring Harbor symposiums on population ecology in the 1960s but, as many ecologists believe, generated little consensus until today, other than shifting the focus of ecology from population ecology to community ecology, and directly catalyzed the emergence of ecosystem ecology from 1960s to 1980s. Although population ecology has never regained its glory since then, the methodology of using mathematical models pioneered by population ecologists has been firmly established as a tradition in whole ecological sciences, including landscape ecology and global change ecology (or planetary ecology). Practically, those debates had far reaching impacts on the strategies for humans to fight against diseases and insect pests of crop and forest plants.
By the 1960s, a consensus has been written into the textbook, and the consensus was that it is generally neither wise nor feasible to eradicate insect pests and/or plant diseases (Kogan and Jepson, 2007). The so-termed IPM is both the strategy and philosophy for humans to deal with the pest (insect pest and plant diseases) problem (Figure 3). Philosophically, we human usually have to coexist with pests, and indeed, we should tolerate their damages as long as the damages are below the so-termed economic tolerance level (ETL) (Kogan and Jepson, 2007). The reason we have to tolerate pests is certainly not because we do not wish to eradicate them, simply because we usually cannot destroy them or the eradication is too costly to bear. In fact, during the approximately two decades after World War II, the wide availability and usage of the pesticide DDT, once convinced people that the insect pest problem was solved forever. DDT was first developed in 1939 and was first used during World War II to clear South Pacific islands of malaria-causing insects for United States troops while being used as an effective delousing powder in Europe. It was believed to be the most powerful pesticide human had ever invented given it could virtually kill all kinds of insects tested from lice, mosquitoes, to caterpillars, and the inventor was awarded Nobel chemical prize.
When DDT was first introduced for civilian use in 1945, few expressed doubts. One was nature writer Edwin Way Teale (The Pulitzer Prize Winner of 1966), who warned, “A spray as indiscriminate as DDT can upset the economy of nature as much as a revolution upsets social economy. Ninety percent of all insects are good, and if they are killed, things go out of kilter right away.” Another was Rachel Carlson, the author of “Silent Spring” (1962) (NRDC, 2015), which spawned the environment movement since 1960s and the establishment of EPA (Environmental Protection Agency) in the United States. It was already known that in the 1960s, DDT was found in the ocean’s deepest and most inaccessible reaches such as fishes, mollusks and seabirds, and in penguin of the South Arctic. When the pesticides such as DDT was banned from usages due its potential healthy implications, entomologists and plant pathologists began to adopt a more ecological and environmental friendly approach, that is, the IPM (e.g., Kogan and Jepson, 2007). With the IPM philosophy, besides backing off from the eradication to tolerating (co-existence) strategy, and an integrated approach (rather than relying on a single tactic in particular pesticide, which should be minimized or avoided as much as possible) with multiple tactics such as quarantine, biological control with natural enemies, crop rotation, mixed plantation, biodiversity augmentation, etc. should be adopted. With the IPM, complex system analysis and mathematical modeling of pest-crop (forest) ecosystem should be used to quantify the ETL and economic threshold (ET), to predict the pest population dynamics, and to devise decision-making rules. By the 1990s, decision support systems (DSSs) using expert system and AI technologies had already been advocated to implement and guide the practice of IPM (Ma et al., 1992; Kogan and Jepson, 2007). Of course, constrained by then state-of-the-art in AI, the application of AI in IPM was rather primitive in today’s standards. Nevertheless, the ideas to use mathematical modeling and AI technology still certainly make sense not only in today’s IPM, but also in biomedicine of humans, especially as future trends (He et al., 2019; Zeng et al., 2021).
In retrospect of the history of IPM and in perspective of bio- and clinical medicines, we may draw the following four analogical principles:
(i) First, the tolerance vs. eradication philosophy: with IPM (Figure 3), the tolerance or coexistence is the predominant strategy, although the so-termed TPM (total pest management) for a handful of insect pests were attempted with mixed results, similarly attempted in veterinary medicine of livestock. In bio-/clinical- medicine of most human diseases, humans are forced to coexist with pathogens from population perspective, although a handful of human pathogens appear to have disappeared (e.g., SARS) or have been eradicated by humans (e.g., Smallpox). We argue that even though the eradication of human pathogens seems infeasible as in the IPM, the determination and efforts are often the top priority because the tolerance thresholds for human diseases are much smaller and frequently approach to zero.
(ii) Second, integrated applications of multiple control tactics are appropriate in both IPM and biomedicine. Quarantines to prevent the spread of invasion insect pests and plant diseases are standard practices in the international trade and a basic law enforcement function of customs on global scale. In fact, quarantines are often the only effective measures to control invasion pests. Similarly, to control COVID-19 like pathogens, apparently, quarantines are equally important, if not more. Lockdown can be considered as “local- or population level-quarantines,” and social distancing and masking may be considered as quarantine measures at the scale of individuals. Even if we do not believe integrated treatments or measures are necessary or desirable for treating human diseases, the alarm from superbugs (antibiotic resistant bacteria) is a proof for the severe side effects of chemical drugs. Although it is obvious that chemical drugs are and will still be predominant treatment measures for human diseases, the importance of alternative medicines has received increasing recognition. We argue that the recognition of human microbiome in human health and diseases can be considered as the counterpart of recognizing the importance of natural enemies in IPM—the biological control. In fact, biological control or bio-control is well recognized as the most desirable control tactics thanks to its ecological safety because it usually only kills pest and produces no harms to humans and environment. Without biological control, the only way to produce truly organic food may be to supply the humans with the “leftover” of insect pests or plant diseases, which may also partially explain the high cost (low yield) of organic foods, not to mention the aesthetic devaluation of the vegetables and fruits bitten by bugs. Therefore, we believe that the importance of human microbiome (especially human virome) in prevention, containment and treatment of human diseases cannot be overly emphasized because there must be natural opponents of pathogens among thousands (if not millions) species of fellow microbes and because competition or struggling for living is part of life as predicted by Darwin’s evolutionary theory.
(iii) In the era of IPM, mathematical modeling (including system analysis and primitive AI in the 1990s) plays significant role for predicting pest dynamics and decision-making (when and what integrated management measures should be taken promptly). In bio-/clinical medicine, besides traditional mathematical modeling and renovated AI technologies, bioinformatics and computational biology become indispensible. Without them, we cannot even “see” the existence of “natural opponents” of pathogens in human microbiomes. One example of showing the emergence of bioinformatics in biomedicine is the transformation of the previously mentioned Cold Spring Harbor, which is famous for its bioinformatics today, while it was well known for its symposiums on population ecology, as mentioned previously.
(iv) Where are the first principles that motivated the IPM philosophy and also the underlying mechanisms that support the IPM strategy and most of its tactics? The answer is ecological science (Figure 3). In fact, virtually the whole insect ecology and significant part of crop (forest) ecology are devoted to the IPM. Similarly, microbiome research is first an ecological problem because understanding species interactions (among microbes and between microbes and their hosts) is a typical topic of ecological science. Somewhat unique to microbiome research is that the ecological studies of human microbiome depend on bioinformatics and computational biology. In fact, the subject of molecular ecology also depends on bioinformatics. This is because in the IPM era, human naked eyes augmented by optical microscopes (occasionally electronic microscopes) are sufficient for the identifications and counting of pathogens/pests; however, for microbiome research, DNA sequencing technology and consequent bioinformatics analyses are indispensable for the very first step of microbiome research—identify microbial species and estimating their abundances. For this reason, medical ecology of human microbiome can be defined as cross-disciplinary studies of human microbiomes for the objectives to understand their implications to human health and diseases from the ecological perspective, which are supported by bioinformatics and computational biology, theoretical ecology, clinical medicine and medical microbiology.
Disease Ecology of Animals With a Focus on Zoonoses
The disease ecology, of course, is not limited to the ecology for plant diseases as briefly introduced previously, where the IPM has been established as the philosophy and strategy for managing insect pests and diseases of plants (crop, vegetables, fruits, and forests), and the biological control (bio-control with natural enemies) is well regarded as the most appropriate measure because of its ecological and environmental friendly nature. In addition, biodiversity augmentation such as mixed plantation of tree plants (mixed forests) has been demonstrated to be effective in managing forest diseases and insect pests. Nevertheless, the role of biodiversity augmentation in control plant diseases and pests is limited, perhaps because manipulating plant diversity is often economically unworthy or infeasible. Of course, increasing natural enemies may also be considered as increasing biodiversity, but it is usually categorized as bio-control. As it is briefly discussed below, in the disease ecology of wildlife, biodiversity or the so-termed diversity–disease relationship (DDR) has been a focus from early days of zoonotic research. Indeed, the importance of DDR of wildlife zoonoses cannot be overly emphasized because it is highly relevant to the spillover risk of zoonoses to humans!
Strictly speaking, the previous discussed IPM is traditionally not investigated in the context of disease ecology; instead it belongs to the domain of applied entomology (agricultural entomology, forest entomology, and horticulture entomology) and plant pathology. The reason we put the IPM into the context of disease ecology is to draw the common and essential principles underlying the diseases of plants, animals, and humans as shown later. The strict usage of disease ecology is usually limited to the diseases of the wildlife. Obviously, pathogens of wildlife are not only a common and integral part of natural ecosystems, but they are also linked to dynamics of wildlife populations. Their actions may drive their hosts to the extinctions occasionally; consequently pose deadly challenges to conservation efforts of endangered species. In the long run, they are also drivers of evolution and play pervasive ecological and evolutionary roles in the ecology and evolution of wildlife. At the foundational level, the mission of disease ecology includes the efforts to deepen our understanding on the pathogen transmission and spreading over space and time as well as their impact on host populations. These efforts also set foundation for epidemiology, which aims to identify risk factors for infectious and non-infectious diseases. Box 1 summarized some key concepts and aspects of disease ecology.
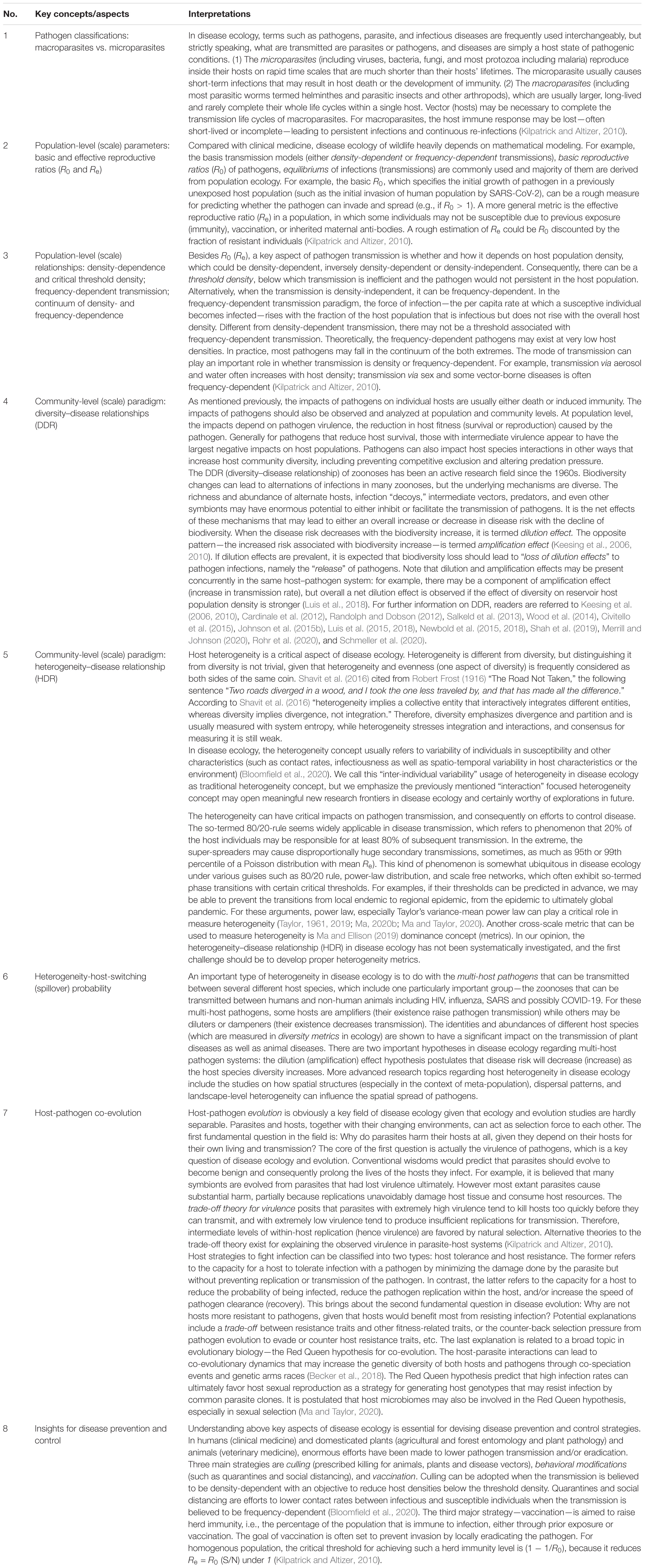
Box 1. Eight selected key concepts and topics in the disease ecology of zoonoses, mainly summarized from Kilpatrick and Altizer (2010) and others.
In our opinion, the field of zoonoses and EID are severely fragmented, possibly due to its highly cross-disciplinary nature. The fragmentation is highly undesirable because an inadvertent knowledge gap may leave the door open for an unwelcome black swan to enter. Therefore, sufficient coverage from cross-disciplinary perspectives is crucial for the healthy development of the field. The field traditionally involves epidemiology, public health, clinical medicine, veterinary medicine, medical microbiology and virology, immunology, ecology and evolution, environmental science, etc. In the 21st century, some emerging novel sciences and technologies joined in, notably molecular biology, bioinformatics, disease ecology, medical ecology, AI, and big data science and technology. The focus of this article is disease- and medical-ecology perspectives.
Arguably the most important mission in studying disease ecology of wildlife or zoonoses is to do with the emerging/reemerging infectious diseases (EID), which are frequently caused by pathogens originating from animal hosts, especially wildlife animals (Guégan et al., 2020). EID events are dominated by zoonoses (60.3% of EIDs): the majority of which (71.8%) originate in wildlife (e.g., SARS and Ebola virus), and are increasing significantly over time (Jones et al., 2008). Zoonoses are infectious diseases that are naturally transmittable from vertebrate animals to humans. Zoonotic viruses are not only the most frequently (constituting over 65% of pathogens discovered since 1980) newly emerging human pathogens, but also include some of the most heinous infectious diseases such as Ebola and Marburg virus, HIV-1 and HIV-2, Sin Nombre virus, Nipah, Hendra and Menangle virus, West Nile virus, Borrelia burgdorferi, and more recently (after 2000), SARS, Middle East respiratory syndrome (MERS) and several subtypes of avian influenza, as well as most recently and likely COVID-19. Some zoonoses requires intermediate vectors (also known as vector-borne pathogens: VBPs), including important human diseases such as malaria and dengue, as well as zoonotic diseases for which people are dead-end hosts, such as Lyme disease and West Nile virus (Taylor et al., 2001; Woolhouse et al., 2001; Woolhouse, 2002; Woolhouse and Gowtage-Sequeria, 2005; Kilpatrick, 2011; Kilpatrick and Randolph, 2012; Johnson et al., 2015a). Johnson et al. (2015a) survey suggested that wild animals were the major source (91% or 86 out of 95) transmission of zoonotic viruses, while only 34% (32 out of 95) transmitted by domestic animals, and 25% by both wild and domestic animals. For example, the Nipah virus (NIV) was introduced into pigs from wild animals, time after time, such as bats, leading to persistent enzootic infection in pigs. Eventually, spillover of NIV to livestock workers occurred (Walsh, 2015).
It was found that the majority (94%) (N = 162) of zoonotic viruses discovered before 2015 were RNA viruses, far more than the number of zoonotic DNA viruses. RNA viruses, such as influenza viruses, flaviviruses, enteroviruses, and coronaviruses, have inherently deficient or absent polymerase error-correction mechanisms and are transmitted as quasi-species or swarms of many, often hundreds or thousands of, genetic variants. Their genetic instability is particularly high, which allows for rapid microbial evolution in an extremely diverse population under natural selection (Morens and Fauci, 2020).
Wild rodents were identified as a spillover source of some zoonotic viruses, such as zoonotic arenaviruses and zoonotic bunyaviruses (Han et al., 2015). Bats belong to oldest mammals and contribute about 20% to mammalian diversity (Zhang et al., 1992; Johnson et al., 2020). Their vast diversity and long co-evolutionary relationships with pathogens maximize the opportunity for cross-species mixing and maintenance of quasi-species pools of viruses that may infect a range of hosts (Menachery et al., 2017). Bats appear to be the most active transmitters given they were more implicated for zoonotic paramyxoviruses and most zoonotic rhabdoviruses (Brook and Dobson, 2015), while primates were implicated as a transmission source of zoonotic retroviruses (Johnson et al., 2015a). Bats are also among the most abundant source for novel viral sequences (Anthony et al., 2013), which have been identified mainly from enteric samples (i.e., bat guano). The huge pools of viruses in bat guano may play a critical role in the bat microbiome to prime their immunity (Menachery et al., 2017).
Despite harboring exceptionally diverse kinds of viruses, surveyed bats rarely display signs of disease, a phenomenon similarly discovered in humans with herpes viruses (Barton et al., 2007). Understanding why bats seem to possess exceptional immunity against viruses is of obvious importance. One important reason may be that virus tropism differences between species and tissues may contribute to limiting disease in bats. Enteric infection can be a significantly different tissue than the respiratory tract in terms of disease and adaptive immunity. The enteric location may generate a dampened adaptive response that permits viral maintenance, while elements of adaptive immunity in bat species keep functional, similar to the members of the microbiome in human guts (Menachery et al., 2017).
The unique host environment of bats is also responsible for the broad diversity in corona-virus (CoV) quasi-species pools. During flight, bats can accumulate reactive oxygen species (ROS) for short periods of time, which may have mutagenic effects, possibly overwhelming CoV proofreading repair and/or altering viral polymerase fidelity and increasing species diversity. The mutagenic effects may also be critical for cross-species or spillover transmission (Seronello et al., 2011). Similarly, Zhou et al. (2016) found that the constitutive expression of type-I IFN (interferon or IFN-α) in bat hosts may select for advantageous viral mutations that enhance resistance to innate immune antiviral defense pathways and provide a replication advantage, especially after cross species transmission. That is, constitutively expressed IFN-α may result in the induction of a subset of IFN-stimulated genes associated with antiviral activity and resistance to DNA damage, suggesting a unique IFN system of bats that promotes their capacity to coexist with viruses (Zhou et al., 2016).
It should be noted that, in spite of previous discussed multiple suspicions bats are implicated, those animals should not be automatically labeled as “bad guys” in spillover events. It is a fact that bats harbor more viruses than many animals, and several studies already pointed important features of bat immune system as involved in both resistance to disease and viruses maintenance in bat populations (Zhou et al., 2016). In other words, bats may possess special mechanisms to “neutralize” disease risks from viruses they host. Actually, few data is available concerning other wildlife species eventually similarly or even more “spillover prone” than bats.
Phylogenetic analyses based on sequence similarity have been playing an important role in studies of the origin and cross-species transmission (spillover) of coronaviruses (e.g., Latinne et al., 2020). Munnink et al. (2020) conducted an in-depth investigation of SARS-CoV-2 infections in animals and humans working or living in 16 mink farms in the Netherlands. SARS-CoV-2 infections were detected in 68% (66 out of 97) of the owners, workers, and their close contacts. Their study provided evidence of SARS-CoV-2 spillover back and forth between animals and humans within mink farms given some people were infected with viral strains with an animal sequence signature. Theoretically, an animal species of sufficiently high population density to allow natural selection and a competent ACE2 protein for SARS-CoV-2 to “hook,” such as mink, would be a possible host of the direct progenitor of SARS-CoV-2 (Zhou and Shi, 2021). However, whether bats or pangolins, which carry coronaviruses with genomes that are ∼90 to 96% similar to human SARS-CoV-2, were the animal source of the first human outbreak, are still in hot debates because there is not certain evidence other than the previously mentioned genome similarity (Hu et al., 2020). Phylogenetic analyses of viral genomes from bats and pangolins revealed that further adaptations, either in animal hosts or in humans, must have occurred before the virus caused the COVID-19 pandemic, if SARS-CoV-2 was indeed spillover from bats or pangolins. SARS-CoV-2 antibodies were detected in human serum samples taken outside of China before the COVID-19 outbreak was detected, which indicates that SARS-CoV-2 had existed for some time before the first cases were described in Wuhan (Andersen et al., 2020). Therefore, it is possible that spillover of SARS-CoV-2 had occurred long before humans discovered its infections.
The zooanthroponosis of SARS-CoV-2 (COVID-19) has been confirmed by its successful detections in animals including domesticated cats, dogs, and ferrets, as well as captive-managed mink, lions, tigers, deer, and mice. Other than circumstantial evidence of zoonotic cases in mink farms in the Netherlands (Zhou and Shi, 2021), no cases of natural transmission from wild or domesticated animals to humans have been confirmed; therefore the zoonotic status of COVID-19 is still a conjecture (e.g., Dhama et al., 2020). Currently nearly 1/4 billion human COVID-19 infections documented seem to be exclusively via human–human transmissions. That is, SARS-CoV-2 virus and COVID-19 do not seems to satisfy the WHO (World Health Organization) definition for zoonoses. For this reason, Haider et al. (2020) suggested to classify SARS-CoV-2 (COVID-19) as an EID of probable animal origin. Compared with other emerging viruses, such as Ebola, avian H7N9, SARS-CoV, and MERS-CoV, SARS-CoV-2 is of relatively low pathogenicity and moderate transmissibility.
Ecology and Evolution of Cancers
Arguably, few other human diseases have been investigated more extensively from ecological and evolutionary perspectives than cancers. Perhaps, the only exceptional category has been the epidemiological studies of infectious diseases, and more recently the microbiome-associated diseases. Ecological concepts and principles have been extensively applied to study cancer and so does the evolutionary theory. Box 2 summarized 20 such concepts, principles, and theories that originated from ecological and evolutionary sciences and found applications in cancer research. Two particular points are worthy of special mentions here, as explained below:
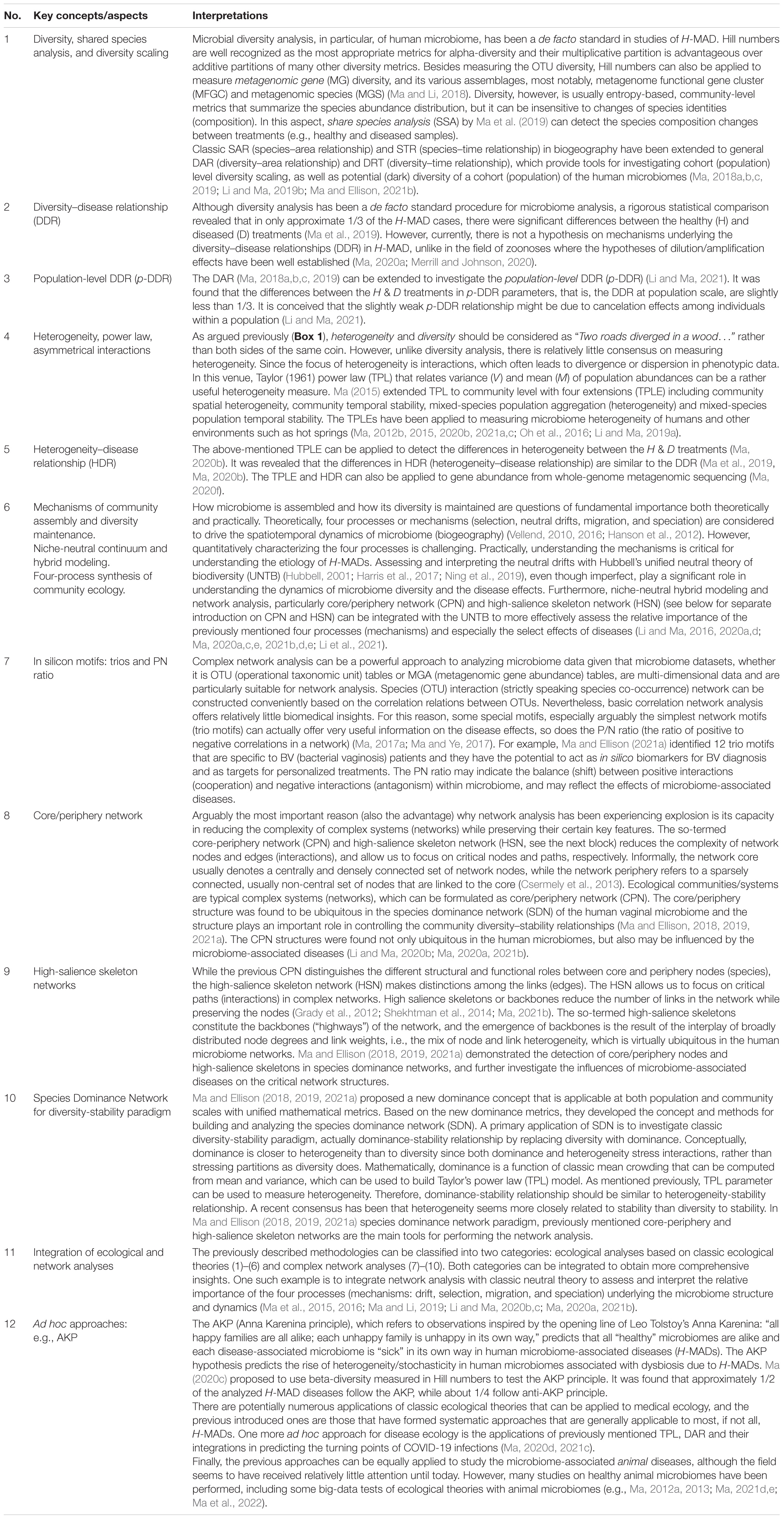
Box 3. Twelve selected key concepts and topics of the medical ecology of human microbiome associated diseases (H-MAD) (H, healthy treatment; D, diseased treatment) (also see Figure 4).
One is that ecology and evolution are innately interwoven for cancer studies: using Hutchinson (1965) classic monograph titled “The Ecological Theatre and Evolutionary Play,” cancer evolution is played out in the ecological setting (the “theater”) of constraining and changing environments (Hall, 2017). The cancer cell environment includes other host cells, microbiomes, and the biochemical and physiological environments. Obviously, virtually everything in cancer environment is dynamic, and for this reason, cancer evolution, which is played out at ultra- micro-, micro-, macro-, and global scales as explained in Box 2. Environment also supplies the energy and materials that allows cancer cells to survive and evolve, until they evolve to their maximal fitness, which kills its host but ironically themselves too, or they are suppressed and/or eradicated by competitions from other somatic cells, predation by immune cells, and/or various medical treatments. For the interwoven nature between cancer ecology and evolution, evo-eco (or eco-evo) paradigm for cancer research has been suggested to play a similar role as the popular evo-devo in evolutionary developmental biology.
Another point to note is the somewhat mismatch between theoretical studies and clinic applications. Although the recognition of cancer as an evolutionary process occurred more than a half-century ago and the recognition of ecological theories have also occurred since the new century, and evo-eco thinking has indeed generated tremendous impacts on cancer research, we must admit that much of the research remain are at the stage of discussions on their parallels. Indeed, much of the ecological and evolutionary concepts in cancer ecology are analogies (parallels) drawn from ecology and evolution. For this reason, evo-eco thinking has not achieved tangible clinic success. As indicated by Korolev et al. (2014), it is crucial to pursue the identified parallels further by making them quantitative, testable, and eventually useful insights for devising therapy strategies and methods (Plutynski, 2021). DNA sequencing technologies, and experiments with microbes and animal models have created unprecedented opportunities to revolutionize the eco-evolutionary studies on cancers.
Cancer is so closely related to genes that it is considered to be a disease of human genome; recent findings suggest that human metagenome is also involved in cancer development (Poore et al., 2020). For this reason, beyond the points summarized in Box 2, in the remainder of this sub-section, we briefly discuss a more recent topic in cancer ecology, the relationship between cancer and human microbiomes. Human microbiomes are distributed not only within and on human bodies, but also within the tumor tissues. They can be neighbors of cancer cells as well as “insiders” within cancer cells. Therefore, microbiomes should have far reaching impacts on the cancers. Nevertheless, the field is still in its infancy stage with a history of slightly longer than a decade.
Bacteria and virus within tumors are localized within both cancer cells and immune cells. However, exact diagnostic implications of microbial contributions to different types of cancer were largely unknown until Nejman et al. (2020) and Poore et al. (2020). Poore et al. (2020) reanalyzed microbial reads from 18,116 samples from 10,481 patients belonging to 33 cancer types deposited in TCGA databases by utilizing machine learning algorithms. They found that there are unique microbial signatures in tissue and blood within and between most cancer types. In some cases, microbiome signature can be more sensitive than human genomic signature. Nejman et al. (2020) took largely experimental approaches to investigating the 1,526 tumor and adjacent normal tissues across seven cancer types covering breast, lung, ovary, pancreas, melanoma, bone, and brain tumors. Their findings are similar to those obtained from the bioinformatics (machine-learning) approaches by Poore et al. (2020). For example, Nejman et al. (2020) demonstrated from their 1,526 tumor microbiome samples that the beta-diversity within a given tumor type is smaller than the beta-diversity between tumor types, i.e., the within cancer type similarity (between tumor tissue and adjacent normal tissue) is larger than between cancer types.
Although bacteria were first detected in human tumors more than a century ago (Nejman et al., 2020), the studies on the relationship between microbiome and cancer is still in its early infancy, and answers to many of the fundamental questions are still open. The most intensively studied microbiome-cancer relationship has been focused on gut microbiome, but in recent years, attentions have been increasingly paid to tissue microbiome such as lung-tissue microbiome and lung cancer relationship. Understanding how microbes in the respiratory tract might influence lung carcinoma development and treatment efficacy may be instrumental for forecasting the risk of cancer development and to improve treatment efficacy and safety (Ramiìrez-Labrada et al., 2020). Cooperative interactions between microbiome and host might lead to microbial participation in host functions such as defense and metabolism. Furthermore, the same microbes that promote human health, under one circumstance, might induce disease and cancer development in another circumstance. In some other circumstances, the change of microbiome composition may cause disease.
In the case of lung cancer, it has been postulated that altered lung microbiome and chronic inflammation in lung tissue contribute to carcinogenesis (e.g., Clavel et al., 2017). The lung microbiome dysbiosis may modulate the risk of malignancy at multiple levels including chronic inflammation and oncogenes (e.g., Tsay et al., 2021). The correlation between repeated antibiotic exposure and increased risk of lung cancer has been investigated. Several bacteria including Mycobacterium tuberculosis have been found to relate with lung cancer.
The coevolution of the host immunity-microbiome interaction may have led to the development of regulatory pathways that modulate self-tolerance and tolerance against non-cancerous agents vs. elimination of pathogens and tumor cells. The delicate balance between tolerance and lung immune activation may be interrupted by changes in immunity-microbiome cooperation due to the antibiotic overuse, changes in diet, or chronic infections, and the loss of balance might raise the risk of lung cancer (e.g., Woodhams et al., 2020).
Medical Ecology of Human Microbiome Associated Diseases
General Introduction on Medical Ecology
The term medical ecology was coined nearly a century ago by eminent microbiologist, Rene Dubos.1 Dubos discovered gramicidin in 1939, together with Alexander Fleming’s discovery of penicillin in 1928, and their findings opened the way into the modern era of anti-microbial therapy. Inspired by their findings, in which soil microbes played a dominant role, Dubos embraced the concept that natural ecosystems, if explored properly, would provide for many of our needs, including treatments for diseases. Therefore, the ecological principles, if applied to the human condition, will provide a resolution to the dichotomy of the “man vs. nature” paradigm. According to the website of http://www.medicalecology.org/, “the medical ecology is an amalgam of principles borrowed from a wide variety of basic and applied sciences. This new hybrid science focuses on issues of human health in which environmental disturbances plays a central role.”
Ma (2012b,2017b,2021a) proposed a narrowed definition for medical ecology, with diseases limited to microbiome-associated diseases. As mentioned previously, medical ecology of human microbiome can be defined as cross-disciplinary studies of human microbiomes for the objectives to understand their implications to human health and diseases from the ecological perspective, which are supported by bioinformatics and computational biology, theoretical ecology, clinical medicine, and medical microbiology. In the case of medical ecology for human microbiomes, community ecology occupies particularly important position (Gilbert and Lynch, 2019). Figure 4 and Box 2 are aimed to illustrate the selected key concepts and aspects of medical ecology of human microbiome associated diseases.
To the best of our knowledge, the term human microbiome associated diseases do not have a formal definition for its scope. Nevertheless, many human diseases are indeed associated with the human microbiomes, indirectly at the minimum. To illustrate this opinion, in the remainder of this section, we review the evidence supporting the relationship between COVID-19 and human microbiomes.
COVID-19 and Human Microbiomes
COVID-19–Virome Interactions
It is estimated that the size of human virome is approximately 380 trillion, which is approximately 10 times of the size of bacterial microbiome, and the later is approximately 10 times of the somatic cell number of human body. The human virome may regulate host immunity and pathophysiology (Brown et al., 2019). Nevertheless, there are few reports on the interaction between COVID-19 and human virome, and to the best of our knowledge, Zuo et al. (2020b) may be the only exception. They found that both enteric RNA and DNA viromes were perturbed by SARS-CoV-2 by comparing stool samples from 98 COVID-19 patients with those from 78 healthy controls. They also suggested that gut microbiome may calibrate host immunity and regulate severity to SARS-CoV-2 infection (Zuo et al., 2020a).
SARS-CoV-2 and Gut Microbiome
Patients with COVID-19 may experience gastrointestinal disorders preceding or following the respiratory symptoms. Studies have confirmed that, alongside the respiratory tract, the gastrointestinal tract can be an entry and replication site for SARS-CoV-2 (Dhar and Mohanty, 2020; François and Harry, 2020). Since gut microbiome plays major roles in maintaining host homeostasis. It has been reported that many respiratory viral infections may alter the gut microbiome, including influenza virus and respiratory syncytial virus, and SARS-CoV-2 is, by no means, an exception (Zuo et al., 2020c).
Gu et al. (2020) found that SARS-CoV-2 infection may influence the composition of gut microbiome, e.g., leading to a significant reduction of bacterial diversity, a significantly higher relative abundance of opportunistic pathogens such as Streptococcus, Rothia, Veillonella, and Actinomyces, and a lower abundance of beneficial symbionts, compared with the control group.
It was revealed that the gut microbiome of COVID-19 patients demonstrated increased functional capacity for nucleotide and amino acid biosynthesis and carbohydrate metabolism (Tang et al., 2020; Zuo et al., 2020b). Depleted symbionts and gut dysbiosis persisted even after the patient recovered from COVID-19. It was suggested that the differences in microbiota composition might be harnessed to differentiate the severity of COVID-19-related infections (Zuo et al., 2020a). SARS-CoV-2-induced shedding of angiotensin-converting enzyme II (ACE2), which is the cell surface receptor that virus binds to when entering host cells, may drive the dysbiosis of gut microbiota (Viana et al., 2020). In the meantime, gut microbiome dysbiosis, in turn, may raise the COVID-19 severity, particularly in elderly or obese patients (Belani, 2020; Viana et al., 2020). Furthermore, improving the profile of the gut microbiome may help to alleviate the COVID-19 symptoms in elderly and immune-compromised patients.
SARS-CoV-2 and Lung Microbiome
Lung microbiome is associated with several respiratory diseases and immunity; by activating an innate and adaptive immune response, it may change the risk and symptoms of COVID-19 disease. However, few existing studies have investigated the lung microbiome of COVID-19 patients (Fan et al., 2020). Shen and Bo (2020) found that COVID-19 patients experienced the enrichment of pathogenic and commensal bacteria. Han et al. (2020) suggested that the COVID-19 patients might have higher diversity of bacteria than the healthy controls. The abundance of lactic acid bacteria, including Lactobacillus fermentum, L. reuteri, L. delbrueckii, and L. salivarius, appeared to be higher in the COVID-19 patients than in the healthy control (Han et al., 2020).
The diversity of human oropharyngeal and intestinal microbiomes may influence the progression of pulmonary viral infection. The SARS-CoV-2 may aggravate lung disease by interacting with the lung or oral microbiota, and the aggravation mechanisms involve changes in cytokines, T cell responses, and the effects of host conditions such as aging, and the oral microbiome changes owing to some systemic diseases (Bao et al., 2020). For instance, Capnocytophaga, Veillonella, and other oral opportunistic pathogens have been found in the lung of the COVID-19 patients (Chen et al., 2020; Peddu et al., 2020; Ren et al., 2020; Shen and Bo, 2020; Wu et al., 2020). The nasopharyngeal bacterial population in COVID-19 patients differed with viral infection lengths, e.g., significant decline of Fusobacterium periodonticum 3 days after infection (Moore et al., 2020).
SARS-CoV-2 and Environmental Microbiome
Coronaviruses may live on in marine plankton with wastewater effluent. Mora et al. (2020) analyzed the metagenomic data from the dried-out Aral Sea basin in Uzbekistan and found that coronavirus-like sequences (including SARS-CoV-2 match) had existed in environmental samples before the current COVID-19 pandemic. Mordecai and Hewson (2020) suggested that SARS-CoV-2 might be present in coastal marine waters affected by sewage effluent, and the rates of their physical decay and loss of infectivity may be similar to other aquatic viruses.
The risk of COVID-19 infections may be influenced by the environmental microbiome diversity. Higher diversity of human microbiome is thought to render better immunity against external infections. Human microbiome diversity is in turn strongly influenced, if not dictated by environmental microbiome diversity. Kumar and Chander (2020) argued that high microbial exposure, particularly to Gram-negative bacteria, may induce interferon type-I that might have a protective effect against COVID-19. Their argument is that the countries with lower mortality also tend to be sanitation poor and to have high incidence of attendant diseases. Kumar and Chander (2020) continued that the populations of developing and underdeveloped countries might have higher resistance to COVID-19 due to high microbial load exposure and resulting microbial interference and/or immunity. They concluded that, high diversity of environmental microbiome appears to have a protective effect against external infection such as SARS-CoV-2 (Kumar and Chander, 2020).
Toward a Unified Medical Ecology of Human Diseases
Is an Ecological Unification Necessary?
At present, majority of studies in medical ecology has been centered on human diseases that are associated with human microbiomes. However, whether or not medical ecology approaches should play an important role in studies beyond human-microbiome-associated diseases are still open. We argue that ecology, especially theoretical ecology, should play a significant role in studies on human diseases, which should be similar to the role that medical genetics has been playing in bio- and clinical medicine since the 1960s. Therefore, medical ecology, in our opinion, should become a foundational discipline of modern medicine, similar to today’s medical genetics (Harper, 2004). Broadly speaking, disease ecology of zoonoses, IPM for agricultural and forest pests, and cancer ecology all have demonstrated the importance of ecology in the medical enterprises we humans have been endeavoring. Whatever terminologies from disease ecology, cancer ecology to medical ecology may be used, the common threads of ecological science are obvious. We suggest the term “medical ecology” is used for human diseases, while preserving the term “disease ecology” for wildlife and zoonoses. As to the ecology of plant and animal diseases (pests), there is no need to change their terminologies since we do not believe there is a need to extend the unification to the areas of plant protection (entomology, nematology, and plant pathology). Regarding the veterinary science for livestock diseases, our limited knowledge suggests that the ecology of livestock diseases should be situated somewhat between the medical ecology for human diseases and disease ecology of wildlife theoretically, and should also be similar to the ecology of IPM for plant protection from a practical perspective. For example, on the one hand, monitoring the emergence and/or reemergence of zoonoses should be conducted through the practices across wildlife conservation, veterinary service, and public health system; on the other hand, some practices in veterinary medicine such as the control of insect and mite pests are similar to the IPM for plant protection. Still, if one feels that a unified terminology is necessary, either disease ecology or medical ecology or their interchangeably usages can be useful; Figure 5 is a sketch from our attempt to view disease and medical ecology from a unified ecological perspective.
Box 4 summarizes the key commonalities and unique aspects of disease ecology, IPM, cancer ecology, medical ecology, and ecology of COVID-19 (as an example of infectious diseases). Although, we realize that it is neither feasible, nor necessary to unify all of the terminologies for ecological sciences compared in Box 4, we reiterate a common ecological perspective is critical for the studies of medical enterprises humans endeavor.
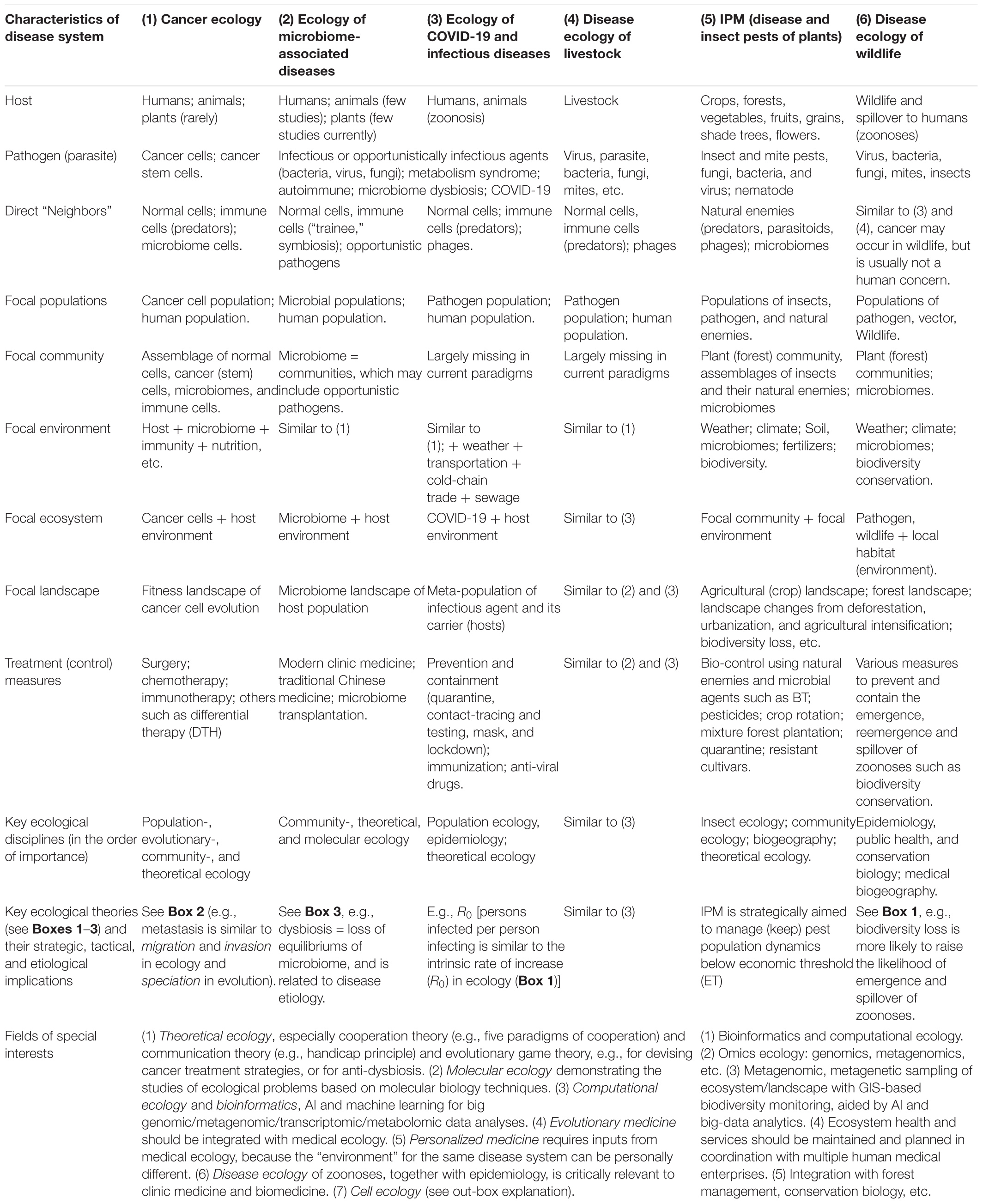
Box 4. Comparing the cancer ecology, medical ecology of human microbiome-associated diseases, medial ecology of COVID-19, disease ecology of livestock, IPM of plant pests, and disease ecology of wildlife.
Perspective—Areas for Promising Novel Breakthroughs
According the Ecological Society of America (ESA),2 “Ecology is the study of the relationships between living organisms, including humans, and their physical environment; it seeks to understand the vital connections between plants and animals and the world around them.” Traditionally, ecology includes several disciplines: autecology, population ecology, community ecology, ecosystem ecology, and landscape ecology in terms of the scale (level) of ecological entities, corresponding to individual (organism), population of individuals (organisms from same species), community of species (assemblage of populations from different species), and landscape (a cluster of interacting ecosystems). This is just one classification scheme for the ecological science, and other alternative schemes exist. For example, there are microbial ecology, plant ecology, insect ecology, and animal ecology in terms of taxa; molecular ecology, physiological ecology, chemical ecology, mathematical ecology, and evolutionary ecology in terms of cross-disciplinary classification. To fit cancer ecology into the ESA definition for ecology, we need to add cells to the list of ecological entities. To add medical ecology as a cross-disciplinary field of ecology, we need to add human host to the list of ecological environments, and, of course, recognize microbiomes as the counterparts of macrobiomes (or biomes in traditional literature) in the ecology and biogeography of plants and animals traditionally. The systematic studies of microbiome started in the new century, while the history of biome research can be traced back to 18th century at a minimum (von Humboldt, 1799, cited in Sanmartín, 2012). Studies in recent years have demonstrated that both microbiomes and macrobiomes should follow the same or similar ecological principles and laws. One such example is the extension of classic species–area relationship (SAR) in plant biogeography (Watson, 1835) to general diversity–area relationship (DAR) (Ma, 2018a,2019), which was first demonstrated with the human microbiomes.
Cells are building blocks of life except for viruses, from single-celled prokaryotes through to metazoans (multi-cellular organisms). To understand a multitude of biological processes, it is required to understand how cells behave, how they interact with each other and with their environment (Richards et al., 2019), which is obviously the mission of ecology. Richards et al. (2019) presented a working definition for single cell ecology: “the use of state-of-the-art approaches, often informed by physical and molecular methods, to study biological phenomena at the scale of a single cell with a focus on how individuals or groups of individuals of the same species interact with their environment, each other and cells of different species.”
One may wonder what is the relationship between the single cell ecology and cancer ecology. Cancer ecology has a history of near three decades; however, until the recent decade, the technology that is implemented at single-cell level for exploring ecological interactions was not available. Single cell sequencing methods refers to sequencing protocols that can sequence a single-cell genome or transcriptome, rather than sequencing the genome from mixed-cell samples as done traditionally (Tang et al., 2019). Compared with traditional sequencing technologies that can only produce the “average” genome of many cells, the single cell sequencing methods (e.g., Xu and Zhao, 2018; Tang et al., 2019) can assess heterogeneities among individual cells, make distinctions among a moderate number of cells, and delineate cell maps. When the single-cell sequencing methods are applied for microbiome/metagenome studies, they can easily link metabolic functions to specific species (hence providing both microbial species and functional diversities), generate a high-quality genome for species with relatively low abundances, which may be rather difficult to capture with traditional metagenomic sequencing (Xu and Zhao, 2018). After the microbial genomes are assembled, one can study genome rearrangement, gene insertion, deletion, duplication, and loss, intra-species variations (strains or sub-species diversity) and virus-host infection of uncultured microbes (Xu and Zhao, 2018). This enabling technology has been changing many fields of biology since its invention, for example: the heterogeneity in antibiotic responses within population of cells, evolution of cancer cell lines, transcriptome expression profiles during viral infection, cell cycles, physical properties of a cell, and microbial interaction with each other and their environment (Richards et al., 2019). In fact, many of the projects have been aimed to investigate diseases at single cell level (Tang et al., 2019). We expect that the single cell sequencing technology is likely to revolutionize the studies of cell ecology and consequently offers unprecedented opportunities to advance medical ecology, in particular, cancer ecology and studies on microbiome-associated diseases.
In the remainder of this review, we try to identify the additional disciplines or fields that are of critical significance for medical ecology, besides cell ecology. The completion of landmark human genome project (HGP) helped to transform the material basis of biological research into big, portable datasets; and simultaneously led to the full establishment of bioinformatics and computational biology (Ma, 2017b; Grote et al., 2021). It appears that biology research has turned from molecularization of life to perceived big-data- and omics-centric present (Grote et al., 2021). Somewhat ironically, this trend is opposite to the ancient question of how to relate ideas of “life” to those of “matter,” which may have to do with the traditional representations widely adopted by biologists, from imagery, metaphors, and scale of explanatory reasoning (Grote et al., 2021). Nowadays, biologists may often be subconscious that gene as code of life is essentially a metaphor and therefore bioinformatics is only a tool to link the code or information with the experimentally known underpinnings (Reynolds, 2018; Grote et al., 2021). Therefore, a point we wish to make is that bioinformatics, big-data analytics, and even arguably computational biology are more like microscope to biologists. They usually do not offer theories to develop hypotheses to explain the experimentally known underpinnings. A follow-up question is then what science can fill the gap to offer the theories? Our answer is the ecology, especially theoretical ecology.
The critical importance of physical sciences (including chemistry) to biology, and particularly molecular biology, has been well recognized, whether it is microscope, electron microscope, to today’s DNA sequencing technology. Nevertheless, virtually all the landmark contributions physical scientists made to biology seem to be on practical sides, rather than on theoretical sides. Arguably, the most important theory in biology, Darwin’s evolutionary theory appeared to have little connections with physical sciences. Instead, ecology and evolution are often perceived as twin in biological sciences (e.g., Department of Ecology and Evolution in many universities, and in sections of many academic journals). Hutchinson’s “Ecological Theater and Evolutionary Play” is another example, which also highlights the dependence between ecological and evolutionary sciences. As illustrated by Kingsland (1995) in her classic “Modeling Nature,” and many others, it is theoretical ecology or mathematical ecology that turns out to play a role similar to what theoretical physics (mathematical physics) does in physics. Indeed, many mathematical models in theoretical physics have been adapted to ecological modeling. Nevertheless, the mathematical models based on the principles of physics (such as thermodynamics) only achieved limited success in ecology. This should be due to the reality that life is fundamentally different from physical world, and physical environment is only part of ecosystem. Therefore, while bioinformatics and computational biology offer necessary supportive tools for medical ecology partially, theoretical ecology (mathematical ecology) present inspirations for constructing and testing important hypotheses (theories) in medical ecology.
In summary, we expect that cell ecology, bioinformatics and computational biology (including big-data analytics and AI), theoretical ecology should be among the most critical supporting disciplines (fields) for advancing medical ecology of human diseases, and equally important to disease ecology of wildlife and livestock, and the IPM of plants. A question slightly beyond the scope of this review is what are the significant contributions medical ecology can make to clinic- and biomedicines. Here, we list four fields that medical ecology can support: (1) etiological insights, especially for human microbiome associated diseases (e.g., Li and Ma, 2020d; Ma and Ellison, 2021a); (2) personalized precision medicine (e.g., Ma et al., 2011); (3) devising innovative treatment strategies and measures such as the immunotherapy and differential therapy (DTH) of cancers (Adler and Gordon, 2019; Solé and Aguadé-Gorgorió, 2021), and microbiome transplantations; (4) epidemiological forecasting of disease outbreaks and pandemic (e.g., Ma, 2020d).
Finally, a slightly off-topic to this review is the field of complexity science, which has enormous potential applications to medicine and medical/disease ecology such as those demonstrated with complex network analyses (e.g., Ma and Ellison, 2019, 2021a) and evolutionary game theory (e.g., Ma and Krings, 2011; Ma and Zhang, 2021; Ma and Yang, 2022). Ecosystems are typical complex systems, and ecological science is arguably one of the most successful scientific disciplines where complexity science has achieved extraordinary successes. We further argue that medical/disease ecology can help to establish strong and broad bridges between medical enterprises and complexity science.
Author Contributions
YPZ and ZSM conceived and outlined the review topics. ZSM wrote the draft. Both authors revised the draft and approved the submission.
Funding
This study received funding from the National Natural Science Foundation of China (Grant: #31970116).
Conflict of Interest
The authors declare that the research was conducted in the absence of any commercial or financial relationships that could be construed as a potential conflict of interest.
Publisher’s Note
All claims expressed in this article are solely those of the authors and do not necessarily represent those of their affiliated organizations, or those of the publisher, the editors and the reviewers. Any product that may be evaluated in this article, or claim that may be made by its manufacturer, is not guaranteed or endorsed by the publisher.
Footnotes
References
Adler, F. R., and Gordon, D. M. (2019). Cancer ecology and evolution: positive interactions and system vulnerability. Curr. Opin. Syst. Biol. 17, 1–7. doi: 10.1016/j.coisb.2019.09.001
Andersen, K. G., Rambaut, A., Lipkin, W. I., Holmes, E. C., and Garry, R. F. (2020). The proximal origin of SARS-CoV-2. Nat. Med. 26, 450–452. doi: 10.1038/s41591-020-0820-9
Anthony, S. J., Epstein, J. H., Murray, K. A., Navarrete-Macias, I., Zambrana-Torrelio, C. M., Solovyov, A., et al. (2013). A strategy to estimate unknown viral diversity in mammals. mBio 4, e598–e513. doi: 10.1128/mBio.00598-13
Araujo, A., Cook, L. M., Frieling, J. S., Tan, W., Copland, J. A. II, Kohli, M., et al. (2021). Quantification and optimization of standard-of-care therapy to delay the emergence of resistant bone metastatic prostate cancer. Cancers (Basel) 13:677. doi: 10.3390/cancers13040677
Bao, L., Zhang, C., Dong, J., Zhao, L., Li, Y., and Sun, J. (2020). Oral microbiome and SARS-CoV-2: beware of lung co-infection. Front. Microbiol. 11:1840. doi: 10.3389/fmicb.2020.01840
Barton, E. S., White, D. W., Cathelyn, J. S., Brett-McClellan, K. A., Engle, M., Diamond, M. S., et al. (2007). Herpesvirus latency confers symbiotic protection from bacterial infection. Nature 447, 326–329. doi: 10.1038/nature05762
Becker, D. J., Streicker, D. G., and Altizer, S. (2018). Using host species traits to understand the consequences of resource provisioning for host-parasite interactions. J. Anim. Ecol. 87, 511–525. doi: 10.1111/1365-2656.12765
Belani, A. (2020). Gut microbiome dysbiosis and endotoxemia-additional pathophysiological explanation for increased COVID-19 severity in obesity. Obes. Med. 20:100302. doi: 10.1016/j.obmed.2020.100302
Bernardo-Cravo, A., Schmeller, D. S., Chatzinotas, A., Vredenburg, V. T., and Loyau, A. (2020). Environmental factors and host microbiomes shape host-pathogen dynamics. Trends Parasitol. 36, 29–36. doi: 10.1016/j.pt.2020.04.010
Bloomfield, L. S. P., Mcintosh, T. L., and Lambin, E. F. (2020). Habitat fragmentation, livelihood behaviors, and contact between people and nonhuman primates in Africa. Landsc. Ecol. 35, 985–1000. doi: 10.1007/s10980-020-00995-w
Brook, C. E., and Dobson, A. P. (2015). Bats as ‘special’ reservoirs for emerging zoonotic pathogens. Trends Microbiol. 23, 172–180. doi: 10.1016/j.tim.2014.12.004
Brown, E. M., Kenny, D. J., and Xavier, R. J. (2019). Gut microbiota regulation of T cells during inflammation and autoimmunity. Annu. Rev. Immunol. 37, 599–624. doi: 10.1146/annurev-immunol-042718-041841
Bukkuri, A., and Adler, F. R. (2021). Viewing cancer through the lens of corruption: using behavioral ecology to understand cancer. Front. Ecol. Evol. 9:678533. doi: 10.3389/fevo.2021.678533
Cardinale, B. J., Duffy, J. E., Gonzalez, A., Hooper, D. U., Perrings, C., Venail, P., et al. (2012). Biodiversity loss and its impact on humanity. Nature 486, 59–67. doi: 10.1038/nature11148
Chen, N., Zhou, M., Dong, X., Qu, J., Gong, F., Han, Y., et al. (2020). Epidemiological and clinical characteristics of 99 cases of 019 novel coronavirus pneumonia in Wuhan, China: a descriptive study. Lancet 395, 507–513. doi: 10.1016/S0140-6736(20)30211-7
Civitello, D. J., Cohen, J., Fatima, H., Halstead, N. T., Liriano, J., McMahon, T. A., et al. (2015). Biodiversity inhibits parasites: broad evidence for the dilution effect. Proc. Nat. Acad. Sci. U.S.A. 112, 8667–8671. doi: 10.1073/pnas.1506279112
Clavel, T., Gomes-Neto, J. C., Lagkouvardos, I., and Ramer-Tait, A. E. (2017). Deciphering interactions between the gut microbiota and the immune system via microbial cultivation and minimal microbiomes. Immunol. Rev. 279, 8–22. doi: 10.1111/imr.12578
Creemers, J. H. A., Lesterhuis, W. J., Mehra, N., Gerritsen, W. R., Figdor, C. G., de Vries, I. J. M., et al. (2021). A tipping point in cancer-immune dynamics leads to divergent immunotherapy responses and hampers biomarker discovery. J. Immunother. Cancer 2021:e002032. doi: 10.1136/jitc-2020-002032
Csermely, P., London, A., Wu, L. Y., and Uzzi, B. (2013). Structure and dynamics of core-periphery networks. J. Complex Netw. 1, 93–123.
Cunningham, A. A., Daszak, P., and Wood, J. L. N. (2017). One Health, emerging infectious diseases and wildlife: two decades of progress? Philos. Trans. R. Soc. Lond. B Biol. Sci. 372:20160167. doi: 10.1098/rstb.2016.0167
Dhama, K., Khan, S., Tiwari, R., Sircar, S., Bhat, S., Malik, Y. S., et al. (2020). Coronavirus disease 2019 -COVID-19. Clin. Microbiol. Rev. 33, e28–e20.
Dhar, D., and Mohanty, A. (2020). Gut microbiota and Covid-19- possible link and implications. Virus Res. 285:198018. doi: 10.1016/j.virusres.2020.198018
Dobson, A. P., Pimm, S. L., Hannah, L., Kaufman, L., Ahumada, J. A., Ando, A. W., et al. (2020). Ecology and economics for pandemic prevention. Science 36, 379–381. doi: 10.1126/science.abc3189
Dua, R., Ma, Y., and Newton, P. K. (2021). Are adaptive chemotherapy schedules robust? A three-strategy stochastic evolutionary game theory model. Cancers 13:2880. doi: 10.3390/cancers13122880
Dujon, A. M., Aktipis, A., Alix-Panabières, C., Amend, S. R., Boddy, A. M., Brown, J. S., et al. (2021). Identifying key questions in the ecology and evolution of cancer. Evol. Appl. 14, 877–892. doi: 10.1111/eva.13190
Ellwanger, J. H., Kulmann-Leal, B., Kaminski, V. L., Valverde-Villegas, J. M., Veiga, A. B. G. D., Spilki, F. R., et al. (2020). Beyond diversity loss and climate change: impacts of amazon deforestation on infectious diseases and public health. An. Acad. Bras. Cienc. 92:e20191375. doi: 10.1590/0001-3765202020191375
Enard, D., and Petrov, D. A. (2020). Ancient RNA virus epidemics through the lens of recent adaptation in human genomes. Phil. Trans. R. Soc. B 375:20190575. doi: 10.1098/rstb.2019.0575
Ewers, R. M., and Didham, R. K. (2007). The effect of fragment shape and species’ sensitivity to habitat edges on animal population size. Conserv. Biol. 21, 926–936. doi: 10.1111/j.1523-1739.2007.00720.x
Fan, J., Li, X., Gao, Y., Zhou, J., Wang, S., Huang, B., et al. (2020). The lung tissue microbiota features of 20 deceased patients with COVID-19. J. Infect. 81, e64–e67. doi: 10.1016/j.jinf.2020.06.047
Fauci, A. S., and Morens, D. M. (2012). The perpetual challenge of infectious diseases. N. Engl. J. Med. 366, 454–461. doi: 10.1056/nejmra1108296
Faust, C. L., McCallum, H. I., Bloomfield, L. S. P., Gottdenker, N. L., Gillespie, T. R., Torney, C. J., et al. (2018). Pathogen spillover during land conversion. Ecol. Lett. 21, 471–483. doi: 10.1111/ele.12904
François, T., and Harry, Sl (2020). Potential causes and consequences of gastrointestinal disorders during a SARS-CoV-2 infection. Cell Rep. 32:107915. doi: 10.1016/j.celrep.2020.107915
Gatenby, R., and Brown, J. (2018). The evolution and ecology of resistance in cancer therapy. Cold Spring Harb. Perspect. Med. 8:a033415. doi: 10.1101/cshperspect.a033415
Gibb, R., Franklinos, L. H. V., Redding, D. W., and Jones, K. E. (2020b). Ecosystem perspectives are needed to manage zoonotic risks in a changing climate. BMJ 371:m3389. doi: 10.1136/bmj.m3389
Gibb, R., Redding, D. W., Chin, K. Q., Donnelly, C. A., Blackburn, T. M., Newbold, T., et al. (2020a). Zoonotic host diversity increases in human-dominated ecosystems. Nature 584, 398–421. doi: 10.1038/s41586-020-2562-8
Gilbert, J. A., and Lynch, S. V. (2019). Community ecology as a framework for human microbiome research. Nat. Med. 25, 884–889. doi: 10.1038/s41591-019-0464-9
Grady, D., Thiemann, C., and Brockmann, D. (2012). Robust classification of salient links in complex networks. Nat. Commun. 3:864. doi: 10.1038/ncomms1847
Gregg, C. (2021). Starvation and climate change—how to constrain cancer cell epigenetic diversity and adaptability to enhance treatment rfficacy. Front. Ecol. Evol. 9:693781. doi: 10.3389/fevo.2021.693781
Grote, M., Onaga, L., Creager, A. N. H., de Chadarevian, S., Liu, D., Surita, G., et al. (2021). The molecular vista: current perspectives on molecules and life in the twentieth century. Hist. Philos. Life Sci. 43:16. doi: 10.1007/s40656-020-00364-5
Gu, S., Chen, Y., Wu, Z., Chen, Y., Gao, H., Lv, L., et al. (2020). Alterations of the gut microbiota in patients with COVID-19 or H1N1 influenza. Clin. Infect. Dis. 71, 2669–2678. doi: 10.1093/cid/ciaa709
Guégan, J. F., Ayouba, A., Cappelle, J., and De Thoisy, B. (2020). Forests and emerging infectious diseases: unleashing the beast wthin. Environ. Res. Lett. 15:083007. doi: 10.1088/1748-9326/ab8dd7
Haider, N., Rothman-Ostrow, P., Osman, A. Y., Arruda, L. B., Macfarlane-Berry, L., Elton, L., et al. (2020). COVID-19-zoonosis or emerging infectious disease? Front. Public Health 8:596944. doi: 10.3389/fpubh.2020.596944
Hall, C. A. S. (2017). Energy Return on Investment: A Unifying Principle for Biology, Economics and Sustainability. Berlin: Springer.
Han, B. A., Schmidt, J. P., Bowden, S. E., and Drake, J. M. (2015). Rodent reservoirs of future zoonotic diseases. Proc. Natl. Acad. Sci. U.S.A. 112, 7039–7044. doi: 10.1073/pnas.1501598112
Han, Y., Jia, Z. L., Shi, J. L., Wang, W., and He, K. (2020). The active lung microbiota landscape of COVID-19 patients. medRxiv [Preprint]. doi: 10.1101/2020.08.20.20144014
Hansen, E., and Read, A. F. (2020). Modifying adaptive therapy to enhance competitive suppression. Cancers (Basel) 12:3556. doi: 10.3390/cancers12123556
Hanson, C. A., Fuhrman, J. A., Horner-Devine, M. C., and Martiny, J. B. H. (2012). Beyond biogeographic patterns: processes shaping the microbial landscape. Nat. Rev. Microbiol. 10, 497–506. doi: 10.1038/nrmicro2795
Harper, P. S. (2004). Landmarks in Medical Genetics: Classic Papers with Commentaries (Oxford Monographs on Medical Genetics Book 51), 1st Edition. Oxford: Oxford University Press.
Harris, K., Parsons, T. L., Ijaz, U. Z., Lahti, L., Holmes, I., and Quince, C. (2017). Linking statistical and ecological theory: hubbell’s unified neutral theory of biodiversity as a hierarchical dirichlet process. Proc. IEEE 105, 516–529. doi: 10.1109/jproc.2015.2428213
He, Y., Zeng, H., Fan, Y. Y., Ji, S. S., and Wu, J. J. (2019). Application of deep learning in integrated pest management: a real-time system for detection and diagnosis of oilseed rape pests. Mob. Inf. Syst. 2019:4570808.
Hu, B., Guo, H., Zhou, P., and Shi, Z. L. (2020). Characteristics of SARS-CoV-2 and COVID-19. Nat. Rev. Microbiol. 19, 141–154. doi: 10.1038/s41579-020-00459-7
Hubbell, S. P. (2001). The unified Neutral Theory of Biodiversity and Biogeography. Princeton, NJ: Princeton University Press.
Hutchinson, G. E. (1965). The Ecological Theatre and Evolutionary Play, 1st Edn. New Haven, CT: Yale University Press.
Hyndman, T. H., Shilton, C. M., Stenglein, M. D., and Wellehan, J. F. X. Jr. (2018). Divergent bornaviruses from Australian carpet pythons with neurological disease date the origin of extant bornaviridae prior to the end-cretaceous extinction. PLoS Pathog 14:e1006881. doi: 10.1371/journal
Johnson, C. K., Hitchens, P. L., Pandit, P. S., Rushmore, J., Evans, T. S., Young, C. C. W., et al. (2020). Global shifts in mammalian population trends reveal key predictors of virus spillover risk. Proc. Biol. Sci. 287:20192736. doi: 10.1098/rspb.2019.2736
Johnson, C., Hitchens, P. L., Smiley Evans, T., Goldstein, T., Thomas, K., Clements, A., et al. (2015a). Spillover and pandemic properties of zoonotic viruses with high host plasticity. Sci. Rep. 5:14830. doi: 10.1038/srep14830
Johnson, P. T. J., Ostfeld, R. S., and Keesing, F. (2015b). Frontiers in research on biodiversity and disease. Ecol. Lett. 18, 1119–1133. doi: 10.1111/ele.12479
Jones, K. E., Patel, N. G., Levy, M. A., Storeygard, A., Balk, D., Gittleman, J. L., et al. (2008). Global trends in emerging infectious diseases. Nature 451, 990–993. doi: 10.1038/nature06536
Kareva, I. (2011). What can ecology teach us about cancer? Transl. Oncol. 4, 266–270. doi: 10.1593/tlo.11154
Kareva, I. (2015). Cancer ecology: niche construction, keystone species, ecological succession, and ergodic theory. Biol. Theory 10, 283–288. doi: 10.1007/s13752-015-0226-y
Keesing, F., Belden, L. K., Daszak, P., Dobson, A., Harvell, C. D., Holt, R. D., et al. (2010). Impacts of biodiversity on the emergence and transmission of infectious diseases. Nature 468, 647–652. doi: 10.1038/nature09575
Keesing, F., Holt, R. D., and Ostfeld, R. S. (2006). Effects of species diversity on disease risk. Ecol. Lett. 9, 485–498. doi: 10.1111/j.1461-0248.2006.00885.x
Kilpatrick, A. M. (2011). Globalization, land use, and the invasion of west nile virus. Science 334, 323–327. doi: 10.1126/science.1201010
Kilpatrick, A. M., and Randolph, S. E. (2012). Drivers, dynamics, and control of emerging vector-borne zoonotic diseases. Lancet 380, 1946–1955. doi: 10.1016/S0140-6736(12)61151-9
Klement, G. L. (2016). Eco-evolution of cancer resistance. Sci. Transl. Med. 8:327fs5. doi: 10.1126/scitranslmed.aaf3802
Kogan, M., and Jepson, P. (2007). Perspectives in Ecological Theory and Integrated Pest Management. Cambridge: Cambridge University Press.
Korolev, K. S., Xavier, J. B., and Gore, J. (2014). Turning ecology and evolution against cancer. Nat. Rev. Cancer 14, 371–380. doi: 10.1038/nrc3712
Kumar, P., and Chander, B. (2020). COVID 19 mortality: probable role of microbiome to explain disparity. Med. Hypotheses 144:110209. doi: 10.1016/j.mehy.2020.110209
Latinne, A., Hu, B., Olival, K. J., Zhu, G., Zhang, L., Li, H., et al. (2020). Origin and cross-species transmission of bat coronaviruses in China. Nat. Commun. 11:4235.
Li, L. W., and Ma, Z. S. (2016). Testing the neutral theory of biodiversity with human microbiome datasets. Sci. Rep. 6:31448. doi: 10.1038/srep31448
Li, L. W., and Ma, Z. S. (2019a). Comparative power law analysis for the spatial heterogeneity scaling of the hot-spring and human microbiomes. Mol. Ecol. 28, 2932–2943. doi: 10.1111/mec.15124
Li, W. D., and Ma, Z. S. (2019b). Diversity scaling of human vaginal microbial communities. Zool. Res. 40, 587–594. doi: 10.24272/j.issn.2095-8137.2019.068
Li, W. D., and Ma, Z. S. (2020a). A theoretic approach to the mechanism of gut microbiome translocation in SIV-infected Asian macaques. FEMS Microbiol. Ecol. 96:fiaa134. doi: 10.1093/femsec/fiaa134
Li, L. W., and Ma, Z. S. (2020d). Species sorting and neutral theory analyses reveal archaeal and bacterial communities are assembled differently in hot springs. Front. Bioeng. Biotechnol. 8:464. doi: 10.3389/fbioe.2020.00464
Li, W. D., and Ma, Z. S. (2020b). Dominance network analysis of the healthy human vaginal microbiome not dominated by Lactobacillus species. Comput. Struct. Biotechnol. J. 18, 3447–3456. doi: 10.1016/j.csbj.2020.10.033
Li, W. D., and Ma, Z. S. (2020c). FBA ecological guild: trio of firmicutes-bacteroidetes alliance against actinobacteria in human oral microbiome. Sci. Rep. 10:287. doi: 10.1038/s41598-019-56561-1
Li, L., Ning, P., and Ma, Z. S. (2021). Structure and dynamics of the breast tissue microbiomes under tumor influences: an approach with neutral, near-neutral, and niche-neutral hybrid models. Front. Microbiol. 12:614967. doi: 10.3389/fmicb.2021.614967
Li, W. D., and Ma, Z. S. (2021). Population-level diversity-disease relationship (DDR) in the human microbiome associated diseases. Comput. Struct. Biotechnol. J. 19, 2297–2306. doi: 10.1016/j.csbj.2021.04.032
Liu, X. P., Chang, X., Leng, S., Tang, H., Aihara, K., and Chen, L. N. (2019). Detection for disease tipping points by landscape dynamic network biomarkers. Nat. Sci. Rev. 6, 775–785. doi: 10.1093/nsr/nwy162
Lloyd-Smith, J. O., Cross, P. C., Briggs, C. J., Daugherty, M., Getz, W. M., Latto, J., et al. (2005). Should we expect population thresholds for wildlife disease? Trends Ecol. Evol. 20, 511–519. doi: 10.1016/j.tree.2005.07.004
Lloyd-Smith, J. O., George, D., Pepin, K. M., Pitzer, V. E., Pulliam, J. R., Dobson, A. P., et al. (2009). Epidemic dynamics at the human-animal interface. Science 326, 1362–1367. doi: 10.1126/science.1177345
Luis, A. D., Kuenzi, A. J., and Mills, J. N. (2018). Species diversity concurrently dilutes and amplifies transmission in a zoonotic host-pathogen system through competing mechanisms. Proc. Natl. Acad. Sci. U.S.A. 115, 7979–7984. doi: 10.1073/pnas.1807106115
Luis, A. D., O’Shea, T. J., Hayman, D. T. S., Wood, J. L. N., Cunningham, A. A., Gilbert, A. T., et al. (2015). Network analysis of host-virus communities in bats and rodents reveals determinants of cross-species transmission. Ecol. Lett. 18, 1153–1162. doi: 10.1111/ele.12491
Ma, Z. S. (2012a). Chaotic populations in genetic algorithms. Appl. Soft Comput. 12, 2409–2424. doi: 10.1016/j.asoc.2012.03.001
Ma, Z. S. (2012b). A note on extending taylor’s power law for characterizing human microbial communities: inspiration from comparative studies on the distribution patterns of insects and galaxies, and as a case study for medical ecology. arXiv [Preprint]. https://arxiv.org/abs/1205.3504 (accessed May 15, 2012).
Ma, Z. S. (2013). Stochastic populations, power law, and fitness aggregation in genetic algorithms. Fundam. Inform. 122, 173–206. doi: 10.3233/fi-2013-787
Ma, Z. S. (2015). Power law analysis of the human microbiome. Mol. Ecol. 24, 5428–5445. doi: 10.1111/mec.13394
Ma, Z. S. (2017a). The P/N (positive-to-negative links) ratio in complex networks—a promising in silico biomarker for detecting changes occurring in the human microbiome. Microb. Ecol. 75, 1063–1073. doi: 10.1007/s00248-017-1079-7
Ma, Z. S. (2018a). Extending species-area relationships (SAR) to diversity-area relationships (DAR). Ecol. Evol. 8, 10023–10038. doi: 10.1002/ece3.4425
Ma, Z. S. (2018b). Diversity time-period and diversity-time-area relationships exemplified by the human microbiome. Sci. Rep. 8:7214. doi: 10.1038/s41598-018-24881-3
Ma, Z. S. (2018c). Sketching the human microbiome biogeography with DAR (diversity-area relationship) profiles. Microb. Ecol. 77, 821–838. doi: 10.1007/s00248-018-1245-6
Ma, Z. S. (2019). A new DTAR (diversity–time–area relationship) model demonstrated with the indoor microbiome. J. Biogeogr. 46, 1–18. doi: 10.1111/jbi.13636
Ma, Z. S. (2020a). Critical network structures and medical ecology mechanisms underlying human microbiome-associated diseases. iScience 23:6. doi: 10.1016/j.isci.2020.101195
Ma, Z. S. (2020b). Heterogeneity-disease relationship in the human microbiome associated diseases. FEMS Microbiol. Ecol. 96:fiaa093. doi: 10.1093/femsec/fiaa093
Ma, Z. S. (2020f). Assessing and interpreting the metagenome heterogeneity with power law. Front. Microbiol. 11:648. doi: 10.3389/fmicb.2020.00648
Ma, Z. S. (2020c). Testing the anna karenina principle in human microbiome-associated diseases. iScience 23:101007. doi: 10.1016/j.isci.2020.101007
Ma, Z. S. (2020e). Niche-neutral theoretic approach to mechanisms underlying biodiversity and biogeography of human microbiomes. Evol. Appl. 14, 322–334. doi: 10.1111/eva.13116
Ma, Z. S. (2020d). Predicting the outbreak risks and inflection points of COVID-19 pandemic with classic ecological theories. Adv. Sci. 7:2001530. doi: 10.1002/advs.202001530
Ma, Z. S. (2021a). Spatial heterogeneity analysis of the human virome with Taylor’s power law. Comput. Struct. Biotechnol. J. 19, 2921–2927. doi: 10.1016/j.csbj.2021.04.069
Ma, Z. S. (2021c). Coupling power laws offers a powerful method for problems such as biodiversity and COVID-19 fatality predictions. arXiv [Preprint]. doi: 10.48550/arXiv.2105.11002
Ma, Z. S. (2021b). Towards a unified medical microbiome ecology of the OMU/OTU (operational metagenomic/taxonomic unit). Mol. Ecol. Resour.
Ma, Z. S. (2021d). Cross-scale analyses of animal and human gut microbiome assemblies from metacommunity to global landscape. mSystems 6:e0063321. doi: 10.1128/mSystems.00633-21
Ma, Z. S. (2021e). Philosophical skepticism concerning the neutral theory or randomness: misplaced or misconceived? A reply to madison, “stochasticity and randomness in community assembly: real or as-if?”. mSystems 6, e1014–e1021. doi: 10.1128/mSystems.01014-21
Ma, Z. S., and Ellison, A. M. (2018). A unified concept of dominance applicable at both community and species scale. Ecosphere 9:e02477. doi: 10.1002/ecs2.2477
Ma, Z. S., and Ellison, A. M. (2019). Dominance network analysis provides a new framework for studying the diversity-stability relationship. Ecol. Monogr. 89:1358. doi: 10.1002/ecm.1358
Ma, Z. S., and Ellison, A. M. (2021b). Towards a unified diversity-area relationship (DAR) of species- and gene-diversity. bioRxiv [Preprint]. doi: 10.1101/2020.05.16.099861v1
Ma, Z. S., and Ellison, A. M. (2021a). In silico trio-biomarkers for bacterial vaginosis revealed by species dominance network analysis. Comput. Struct. Biotechnol. J. 19, 2979–2989. doi: 10.1016/j.csbj.2021.05.020
Ma, Z. S., and Krings, A. W. (2011). Dynamic hybrid fault modeling and extended evolutionary game theory for reliability, survivability and fault tolerance analyses. IEEE Trans. Reliab. 60, 180–196. doi: 10.1109/tr.2011.2104997
Ma, Z. S., and Li, L. W. (2018). Measuring metagenome diversity and similarity with Hill numbers. Mol. Ecol. Resour. 18, 1339–1355. doi: 10.1111/1755-0998.12923
Ma, Z. S., and Li, W. D. (2019). How man and woman are different in their microbiome: ecological and network analyses of the microgenderome. Adv. Sci. 6:1902054. doi: 10.1002/advs.201902054
Ma, Z. S., and Taylor, R. A. J. (2020). Human reproductive system microbiomes exhibited significantly different heterogeneity scaling with gut microbiome, but the intra-system scaling is invariant. Oikos 129, 903–911. doi: 10.1111/oik.07116
Ma, Z. S., and Yang, L. X. (2022). CDC (cindy and david’s conversations) game: advising president to survive pandemic. medRixv [Preprint]. doi: 10.1101/2022.03.14.22272381v1
Ma, Z. S., and Ye, D. D. (2017). Trios—promising in silico biomarkers for differentiating the effect of disease on the human microbiome network. Sci. Rep. 7:13259. doi: 10.1038/s41598-017-12959-3
Ma, Z. S., and Zhang, Y. P. (2021). To mask, or not to mask, alice and bob’s dating dilemma. medRixv [Preprint]. doi: 10.1101/2022.04.14.22273886
Ma, Z. S., Zhang, Z., Xu, H., Wen, X., et al. (1992). Toward a decision support system for integrated pest management of pine caterpillar. J. Beijing Forestry University 1992, 107–113.
Ma, Z. S., Guan, Q., Ye, C., Zhang, C., Foster, J. A., and Forney, L. J. (2015). Network analysis suggests a potentially ‘evil’ alliance of opportunistic pathogens inhibited by a cooperative network in human milk bacterial communities. Sci. Rep. 5:8275. doi: 10.1038/srep08275
Ma, Z. S., Li, L. W., and Gotelli, N. J. (2019). Diversity-disease relationships and shared species analyses for human microbiome-associated diseases. ISME J. 13, 1911–1919. doi: 10.1038/s41396-019-0395-y
Ma, Z. S., Li, L. W., Li, W., Li, J., and Chen, H. (2016). Integrated network-diversity analyses suggest suppressive effect of Hodgkin’s lymphoma and slightly relieving effect of chemotherapy on human milk microbiome. Sci. Rep. 6:28048. doi: 10.1038/srep28048
Ma, Z. S., Li, W. D., and Shi, P. (2022). Microbiome-host phylogeny relationships in animal gastrointestinal tract microbiomes. FEMS Microbiol. Ecol. 98:fiac021. doi: 10.1093/femsec/fiac021
Ma, Z. S., Zaid, A., and Forney, L. J. (2011). Caring about trees in the forest: incorporating frailty in risk analysis for personalized medicine. Per. Med. 8, 681–688. doi: 10.2217/pme.11.72
Maley, C. C., Aktipis, A., Graham, T. A., Sottoriva, A., Boddy, A. M., and Janiszewska, M. (2017). Classifying the evolutionary and ecological features of neoplasms. Nat. Rev. Cancer 17, 605–619. doi: 10.1038/nrc.2017.69
McGuire, B. (2002). Global Catastrophes: A Very Short Introduction. Oxford: Oxford University Press.
Menachery, V. D., Graham, R. L., and Baric, R. S. (2017). Jumping species-a mechanism for coronavirus persistence and survival. Curr. Opin Virol. 23, 1–7. doi: 10.1016/j.coviro.2017.01.002
Merrill, T. S., and Johnson, P. J. (2020). Toward a mechanistic understanding of competence: a missing link in diversity-disease research. Parasitology 147, 1159–1170. doi: 10.1017/S0031182020000943
Miller, A. K., Brown, J. S., Enderling, H., Basanta, D., and Whelan, C. J. (2021). The evolutionary ecology of dormancy in nature and in cancer. Front. Ecol. Evol. 9:676802. doi: 10.3389/fevo.2021.676802
Moore, S. C., Penrice-Randal, R., Alruwaili, M., Dong, X., Pullan, S. T., Carter, D. P., et al. (2020). Amplicon based MinION sequencing of SARS-CoV-2 and metagenomic characterisation of nasopharyngeal swabs from patients with COVID-19. medRxiv [Preprint]. doi: 10.1101/2020.03.05.20032011
Mora, M., Egamberdieva, D., Krause, R., Martinez, J.-L., Cernava, T., Berg, G., et al. (2020). Highly matching coronavirus-like short sequences can be retrieved from environmental metagenomes. Res. Square 1–9. doi: 10.21203/rs.3.rs-44155/v1
Mordecai, G. J., and Hewson, I. (2020). Coronaviruses in the sea. Front. Microbiol. 11:1795. doi: 10.3389/fmicb.2020.01795
Morens, D. M., and Fauci, A. S. (2020). Emerging pandemic diseases: how we got to COVID-19. Cell 182, 1077–1092. doi: 10.1016/j.cell.2020.08.021
Munnink, B. B. O., Sikkema, R. S., Nieuwenhuijse, D. F., Molenaar, R. J., Munger, E., Molenkamp, R., et al. (2020). Transmission of SARS-CoV-2 on mink farms between humans and mink and back to humans. Science 371:172. doi: 10.1126/science.abe5901
Nejman, D., Livyatan, I., Fuks, G., Gavert, N., Zwang, Y., Geller, L. T., et al. (2020). The human tumor microbiome is composed of tumor type–specific intracellular bacteria. Science 368, 973–980. doi: 10.1126/science.aay9189
Newbold, T., Hudson, L. N., Contu, S., Hill, S. L. L., Beck, J., Liu, Y., et al. (2018). Widespread winners and narrow-ranged losers: land use homogenizes biodiversity in local assemblages worldwide. PLoS Biol. 16:e2006841. doi: 10.1371/journal.pbio.2006841
Newbold, T., Hudson, L. N., Hill, S. L., Contu, S., Lysenko, I., Senior, R. A., et al. (2015). Global effects of land use on local terrestrial biodiversity. Nature. 520, 45–50. doi: 10.1038/nature14324
Ning, D., Deng, Y., Tiedje, J. M., and Zhou, J. (2019). A general framework for quantitatively assessing ecological stochasticity. PNAS 116, 16892–16898. doi: 10.1073/pnas.1904623116
Oh, J., Byrd, A. L., Park, M., NISC Comparative Sequencing Program, Kong, H. H., and Segre, J. A. (2016). Temporal stability of the human skin microbiome. Cell 165, 854–866. doi: 10.1016/j.cell.2016.04.008
Pacheco, J. M., Santos, F. C., and Dingli, D. (2014). The ecology of cancer from an evolutionary game theory perspective. Interface Focus 4:20140019. doi: 10.1098/rsfs.2014.0019
Patz, J. A., Campbell-Lendrum, D., Holloway, T., and Foley, J. A. (2005). Impact of regional climate change on human health. Nature 438, 310–317. doi: 10.1038/nature04188
Peddu, V., Shean, R. C., Xie, H., Shrestha, L., Perchetti, G. A., Minot, S. S., et al. (2020). Metagenomic analysis reveals clinical SARS-CoV-2 infection and bacterial or viral superinfection and colonization. Clin. Chem. 66, 966–972. doi: 10.1093/clinchem/hvaa106
Peplinski, J., Malone, M. A., Fowler, K. J., Potratz, E. J., Pergams, A. G., Charmoy, K. L., et al. (2021). Ecology of fear: spines, armor and noxious chemicals deter predators in cancer and in nature. Front. Ecol. Evol. 9:682504. doi: 10.3389/fevo.2021.682504
Plowright, R. K., Parrish, C. R., McCallum, H., Hudson, P. J., Ko, A. I., Graham, A. L., et al. (2017). Pathways to zoonotic spillover. Nat. Rev. Microbiol. 15, 502–510. doi: 10.1038/nrmicro.2017.45
Plutynski, A. (2021). Testing multi-task cancer evolution: how do we test ecological hypotheses in cancer? Front. Ecol. Evol. 9:666262. doi: 10.3389/fevo.2021.666262
Poore, G. D., Kopylova, E., Zhu, Q., Carpenter, C., Fraraccio, S., Wandro, S., et al. (2020). Microbiome analyses of blood and tissues suggest cancer diagnostic approach. Nature 579, 567–574. doi: 10.1038/s41586-020-2095-1
Pressley, M., Salvioli, M., Lewis, D. B., Richards, C. L., Brown, J. S., and Stanková, K. (2021). Evolutionary dynamics of treatment-induced resistance in cancer informs understanding of rapid evolution in natural systems. Front. Ecol. Evol. 9:681121. doi: 10.3389/fevo.2021.681121
Rahman, M. T., Sobur, M. A., Islam, M. S., Ievy, S., Hossain, M. J., El Zowalaty, M. E., et al. (2020). Zoonotic diseases: etiology, impact, and control. Microorganisms 8:1405. doi: 10.3390/microorganisms8091405
Ramiìrez-Labrada, A. G., Isla, D., Artal, A., Arias, M., Rezusta, A., Pardo, J., et al. (2020). The influence of lung microbiota on lung carcinogenesis, immunity, and immunotherapy. Trends Cancer 6, 86–97. doi: 10.1016/j.trecan.2019.12.007
Randolph, S., and Dobson, A. (2012). Pangloss revisited: a critique of the dilution effect and the biodiversity-buffers-disease paradigm. Parasitology 139, 847–863. doi: 10.1017/S0031182012000200
Ren, L. L., Wang, Y. M., Wu, Z. Q., Xiang, Z. C., Guo, L., Xu, T., et al. (2020). Identification of a novel coronavirus causing severe pneumonia in human: a descriptive study. Chin. Med. J. (Engl). 133, 1015–1024. doi: 10.1097/CM9.0000000000000722
Reynolds, A. S. (2018). The Third Lens: Metaphor and the Creation of Modern Cell Biology. Chicago, IL: University of Chicago Press.
Reynolds, B. A., Oli, M. W., and Oli, M. K. (2020). Eco-oncology: applying ecological principles to understand and manage cancer. Ecol. Evol. 10, 8538–8553. doi: 10.1002/ece3.6590
Richards, T. A., Massana, R., Pagliara, S., and Hall, N. (2019). Single cell ecology. Phil. Trans. R. Soc. B 374:20190076. doi: 10.1098/rstb.2019.0076
Rohr, J. R., Civitello, D. J., Halliday, F. W., Hudson, P. J., Lafferty, K. D., Wood, C. L., et al. (2020). Towards common ground in the biodiversity-disease debate. Nat. Ecol. Evol. 4, 24–33. doi: 10.1038/s41559-019-1060-6
Rosenberg, E., and Zilber-Rosenberg, I. (2018). The hologenome concept of evolution after 10 years. Microbiome 6:78. doi: 10.1186/s40168-018-0457-9
Salkeld, D. J., Padgett, K., and Jones, J. (2013). A meta-analysis suggesting that the relationship between biodiversity and risk of zoonotic pathogen transmission is idiosyncratic. Ecol. Lett. 16, 679–686. doi: 10.1111/ele.12101
Sanmartín, I. (2012). Historical biogeography: evolution in time and space. Evo. Edu. Outreach 5, 555–568. doi: 10.1007/s12052-012-0421-2
Schmeller, D. S., Courchamp, F., and Killeen, G. (2020). Biodiversity loss, emerging pathogens and human health risks. Biodivers. Conserv. 29, 3095–3102. doi: 10.1007/s10531-020-02021-6
Schmidt, K. A. (2017). Information thresholds, habitat loss and population persistence in breeding birds. Oikos 126, 651–659. doi: 10.1111/oik.03703
Seronello, S., Montanez, J., Presleigh, K., Barlow, M., Park, S. B., and Choi, J. (2011). Ethanol and reactive species increase basal sequence heterogeneity of hepatitis C virus and produce variants with reduced susceptibility to antivirals. PLoS One 6:e27436. doi: 10.1371/journal.pone.0027436
Shah, H. A., Huxley, P., Elmes, J., and Murray, K. A. (2019). Agricultural land-uses consistently exacerbate infectious disease risks in Southeast Asia. Nat. Commun. 10:4299. doi: 10.1038/s41467-019-12333-z
Shavit, A., Kolumbus, A., and Ellison, A. M. (2016). Two roads diverge in a wood: indifference to the difference between ‘diversity’ and ‘heterogeneity’ should be resisted on epistemic and moral grounds. Phil Sci Arch.
Shen, C. M., and Bo, X. M. (2020). Gut microbiota may underlie the predisposition of healthy individuals to COVID-19. medRxiv [Preprint]. doi: 10.21203/rs.3.rs-45991/v1
Shekhtman, L. M., Berezin, Y., Danziger, M. M., and Havlin, S. (2014). Robustness of a network formed of spatially embedded networks. Phys. Rev. E Stat. Nonlin. Soft Matt. Phys. 90:012809.
Simon, J. C., Marchesi, J. R., Mougel, C., and Selosse, M. A. (2019). Host-microbiota interactions: from holobiont theory to analysis. Microbiome 7:5. doi: 10.1186/s40168-019-0619-4
Solé, R., and Aguadé-Gorgorió, G. (2021). The ecology of cancer differentiation therapy. J. Theor. Biol. 511:110552. doi: 10.1016/j.jtbi.2020.110552
Somarelli, J. A. (2021). The hallmarks of cancer as ecologically driven phenotypes. Front. Ecol. Evol. 9:661583. doi: 10.3389/fevo.2021.661583
Swift, T. L., and Hannon, S. J. (2010). Critical thresholds associated with habitat loss: a review of the concepts, evidence, and applications. Biol. Rev. 85, 35–53. doi: 10.1111/j.1469-185X.2009.00093.x
Tang, L., Gu, S., Gong, Y., Li, B., Lu, H., Li, Q., et al. (2020). Clinical significance of the correlation between changes in the major intestinal bacteria species and COVID-19 severity. Engineering 6, 1178–1184. doi: 10.1016/j.eng.2020.05.013
Tang, X. N., Huang, Y. M., Lei, J. L., Luo, H., and Zhu, X. (2019). The single-cell sequencing: new developments and medical applications. Cell Biosci. 9:53. doi: 10.1186/s13578-019-0314-y
Taylor, R. A. J. (2019). Taylor’s Power Law: Order and Pattern in Nature. London: Academic Press, 657.
Taylor, L. H., Latham, S. M., and Woolhouse, M. E. (2001). Risk factors for human disease emergence. Phil.Trans. R. Soc. Lond. B 356, 983–989. doi: 10.1098/rstb.2001.0888
Tiwari, R., Dhama, K., Sharun, K., Iqbal Yatoo, M., Malik, Y. S., Singh, R., et al. (2020). COVID-19: animals, veterinary and zoonotic links. Vet. Q. 40, 169–182. doi: 10.1080/01652176.2020.1766725
Tsay, J. J., Wu, B. G., Sulaiman, I., Gershner, K., Schluger, R., Li, Y., et al. (2021). lower airway dysbiosis affects lung cancer progression. Cancer Discov. 11, 293–307. doi: 10.1158/2159-8290.CD-20-0263
Ujvari, B., Fitzpatrick, J., Raven, N., Osterkamp, J., and Thomas, F. (2019). “The ecology of cancer,” in Cancer and Society, ed. E. Bernicker (Berlin: Springer), doi: 10.1007/978-3-030-05855-5_12
Vellend, M. (2010). Conceptual synthesis in community ecology. Q. Rev. Biol. 85, 183–206. doi: 10.1086/652373
Vellend, M. (2016). The Theory of Ecological Communities. Princeton, NJ: Princeton University Press.
Viana, S. D., Nunes, S., and Reis, F. (2020). ACE2 imbalance as a key player for the poor outcomes in COVID-19 patients with age-related comorbidities – role of gut microbiota dysbiosis. Ageing Res. Rev. 62:101123. doi: 10.1016/j.arr.2020.101123
Walsh, M. G. (2015). Mapping the risk of nipah virus spillover into human populations in south and Southeast Asia. Trans. R. Soc.Trop. Med. Hyg. 109, 563–571. doi: 10.1093/trstmh/trv055
Whelan, C. J., Avdieiev, S. S., and Gatenby, R. A. (2020). Insights from the ecology of information to cancer control. Cancer Control. 27:1073274820945980. doi: 10.1177/1073274820945980
Wood, C. L., Lafferty, K. D., Deleo, G., Young, H. S., Hudson, P. J., and Kuris, A. M. (2014). Does biodiversity protect humans against infectious disease. Ecology 95, 817–832. doi: 10.1890/13-1041.1
Woodhams, D. C., Bletz, M. C., Becker, C. G., Bender, H. A., Buitrago-Rosas, D., Diebboll, H., et al. (2020). Host-associated microbiomes are predicted by immune system complexity and climate. Genome Biol. 21:23. doi: 10.1186/s13059-019-1908-8
Woolhouse, M. E. J. (2002). Population biology of emerging and re-emerging pathogens. Trends Microbiol. 10, S3–S7. doi: 10.1016/s0966-842x(02)02428-9
Woolhouse, M. E. J., and Gowtage-Sequeria, S. (2005). Host range and emerging and reemerging pathogens. Emerg. Infect. Dis. 11, 1842–1847. doi: 10.3201/eid1112.050997
Woolhouse, M. E. J., Taylor, L. H., and Haydon, D. T. (2001). Population biology of multihost pathogens. Science 292, 1109–1112. doi: 10.1126/science.1059026
Wu, C. I., Wang, H. Y., Ling, S., and Lu, X. (2016). The ecology and evolution of cancer: the ultra-microevolutionary process. Ann. Rev. Genet. 50:347. doi: 10.1146/annurev-genet-112414-054842
Wu, F., Zhao, S., Yu, B., Chen, Y. M., Wang, W., Song, Z. G., et al. (2020). A new coronavirus associated with human respiratory disease in China. Nature 579, 265–269.
Xu, Y., and Zhao, F. Q. (2018). Single-cell metagenomics: challenges and applications. Protein Cell 9, 501–510. doi: 10.1007/s13238-018-0544-5
Zeng, D., Cao, Z. D., and Neill, D. B. (2021). Artificial intelligence enabled public health surveillance—from local detection to global epidemic monitoring and control. Artif. Intell. Med. 2021, 437–453. doi: 10.1016/B978-0-12-821259-2.00022-3
Zhang, X. M., Kousoulas, K. G., and Storz, J. (1992). The hemagglutinin/esterasegene of human coronavirus strain OC43: phylogenetic relationships to bovine and murine coronaviruses and influenza C virus. Virology 186, 318–323. doi: 10.1016/0042-6822(92)90089-8
Zhou, P., and Shi, Z. L. (2021). SARS-CoV-2 spillover events: spillover from mink to humans highlights SARS-CoV-2 transmission routes from animals. Science 371, 120–121. doi: 10.1126/science.abf6097
Zhou, P., Tachedjian, M., Wynne, J. W., Boyd, V., Cui, J., Smith, I., et al. (2016). Contraction of the type I IFN locus and unusual constitutive expression of IFN-alpha in bats. Proc. Natl. Acad. Sci. U.S A. 113, 2696–2701. doi: 10.1073/pnas.1518240113
Zuo, T., Liu, Q., Zhang, F., Lui, G. C., Tso, E. Y., Yeoh, Y. K., et al. (2020b). Original research: depicting SARS-CoV-2 faecal viral activity in association with gut microbiota composition in patients with COVID-19. Gut Microbiota 70, 276–284. doi: 10.1136/gutjnl-2020-322294
Zuo, T., Liu, Q., Zhang, F., Yeho, Y. K., Wan, Y., Zhan, H., et al. (2020a). Temporal Landscape of Human Gut Virome in SARS-CoV-2 Infection and Severity. Rochester, NY: Social Science Electronic Publishing.
Keywords: medical ecology, disease ecology, cancer ecology, integrated pest management (IPM), theoretical ecology, cell ecology, computational biology and bioinformatics, genomics and metagenomics
Citation: Ma ZS and Zhang YP (2022) Ecology of Human Medical Enterprises: From Disease Ecology of Zoonoses, Cancer Ecology Through to Medical Ecology of Human Microbiomes. Front. Ecol. Evol. 10:879130. doi: 10.3389/fevo.2022.879130
Received: 18 February 2022; Accepted: 19 April 2022;
Published: 15 June 2022.
Edited by:
Alexandro Guterres, Oswaldo Cruz Foundation (Fiocruz), BrazilReviewed by:
Ana Cláudia Coelho, University of Trás-os-Montes and Alto Douro, PortugalJose Artur Chies, Federal University of Rio Grande do Sul, Brazil
Copyright © 2022 Ma and Zhang. This is an open-access article distributed under the terms of the Creative Commons Attribution License (CC BY). The use, distribution or reproduction in other forums is permitted, provided the original author(s) and the copyright owner(s) are credited and that the original publication in this journal is cited, in accordance with accepted academic practice. No use, distribution or reproduction is permitted which does not comply with these terms.
*Correspondence: Zhanshan (Sam) Ma, ma@vandals.uidaho.edu; Ya-Ping Zhang, zhangyp@mail.kiz.ac.cn