- 1Programme Cartes d'Identité des Tumeurs, Ligue Nationale Contre le Cancer, Paris, France
- 2Centre de Recherche des Cordeliers, INSERM, Sorbonne Université, Université de Paris, Equipe inflammation, complément et cancer, Paris, France
Tumor cells constantly interact with their microenvironment, which comprises a variety of immune cells together with endothelial cells and fibroblasts. The composition of the tumor microenvironment (TME) has been shown to influence response to immune checkpoint blockade (ICB). ICB takes advantage of immune cell infiltration in the tumor to reinvigorate an efficacious antitumoral immune response. In addition to tumor cell intrinsic biomarkers, increasing data pinpoint the importance of the TME in guiding patient selection and combination therapies. Here, we review recent efforts in determining how various components of the TME can influence response and resistance to ICB. Although a large body of evidence points to the extent and functional orientation of the T cell infiltrate as important in therapy response, recent studies also confirm a role for other components of the TME, such as B cells, myeloid lineage cells, cancer-associated fibroblasts, and vasculature. If the ultimate goal of curative cancer therapies is to induce a long-term memory T cell response, the other components of the TME may positively or negatively modulate the induction of efficient antitumor immunity. The emergence of novel high-throughput methods for analyzing the TME, including transcriptomics, has allowed tremendous developments in the field, with the expansion of patient cohorts, and the identification of TME-based markers of therapy response. Together, these studies open the possibility of including TME-based markers for selecting patients that are likely to respond to specific therapies, and pave the way to personalized medicine in oncology.
Introduction
Cancers arise from the accumulation of genomic abnormalities in pre-malignant cells. These cells hijack key homeostasis functions to promote their survival and growth and avoid elimination by the immune system (1). The interplay between malignant cells and the immune system during cancer development has been proposed to comprise three steps: elimination, followed by an equilibrium phase, and escape from the immune control, termed the 3 Es of cancer immunoediting (2).
Indeed, malignant cells develop and evolve in a complex and strongly interconnected tumor microenvironment (TME), comprising a vast variety of immune cells and non-immune stromal cells such as endothelial cells and fibroblasts (3). Studying the TME is of paramount importance given the clinical impact of its composition and extent (4). For instance, a strong infiltration by CD8+ T cells is generally associated with a favorable prognosis (5–8), while the presence of M2-polarized macrophages is widely considered a negative prognostic marker (9–11). Moreover, the TME, through its many components, harbors a high diversity of possible targets for cancer treatment (4, 12, 13).
In recent years, therapeutic options for the treatment of cancer have changed tremendously with the development of immunotherapy. Among the various types of immunotherapy, immune checkpoint blockade (ICB) covers a range of monoclonal antibody-based therapies that aim at blocking the interaction of inhibitory receptors (immune checkpoints) expressed on the surface of immune cells, with their ligands. The main targets for these treatments are CTLA-4 and PD-1 or its ligand PD-L1. ICB has drawn considerable attention (14, 15), especially because of the durability of responses and effects on patients' overall survival. A key challenge is identifying patients who are the most likely to respond.
Several markers have recently been suggested to be associated with response to ICB. The PD-1/PD-L1 axis is at the forefront of interactions between immune, stromal and tumor cells. The expression of both PD-1 and PD-L1 was shown to be increased in melanoma patients who responded to PD-1 blockade (16). PD-L1 expression on tumor cells was associated with response to anti-PD-1 therapies in various malignancies (17, 18). To date, PD-L1 detection by immunohistochemical analysis is the only companion test approved by the FDA for ICB in NSCLC, urothelial carcinoma, cervical cancer, and triple-negative breast cancer (19). However, subsequent trials have reported conflicting results for the use of PD-L1 as a predictive biomarker (20), likely due to the heterogeneity of modalities used (such as the antibodies used for detection, or the PD-L1 positivity threshold). In addition, it was shown, initially in melanoma and non-small cell lung cancer (NSCLC) which are highly mutated tumor types (21), that the higher the mutational burden of a tumor, the more likely it is to respond to ICB (22–24). This was recently demonstrated to remain true in many malignancies (25). In particular, a high response rate to ICB was reported in tumors with mismatch-repair deficiency (26–28). However, this is only a general correlate that does not provide sufficient sensitivity or specificity in all cancer types (29). Recently, the gut microbiome was also shown to be associated with response to ICB (30–33), although many questions remain open in this area (34).
Here, we review recent advances in understanding the composition and functionality of the TME in response and resistance to ICB, and we discuss how these insights can facilitate the prediction of patient responses. The association of TME components with response to ICB is summarized in Table 1 (factors associated with response) and Table 2 (factors associated with resistance), as well as in Figure 1.
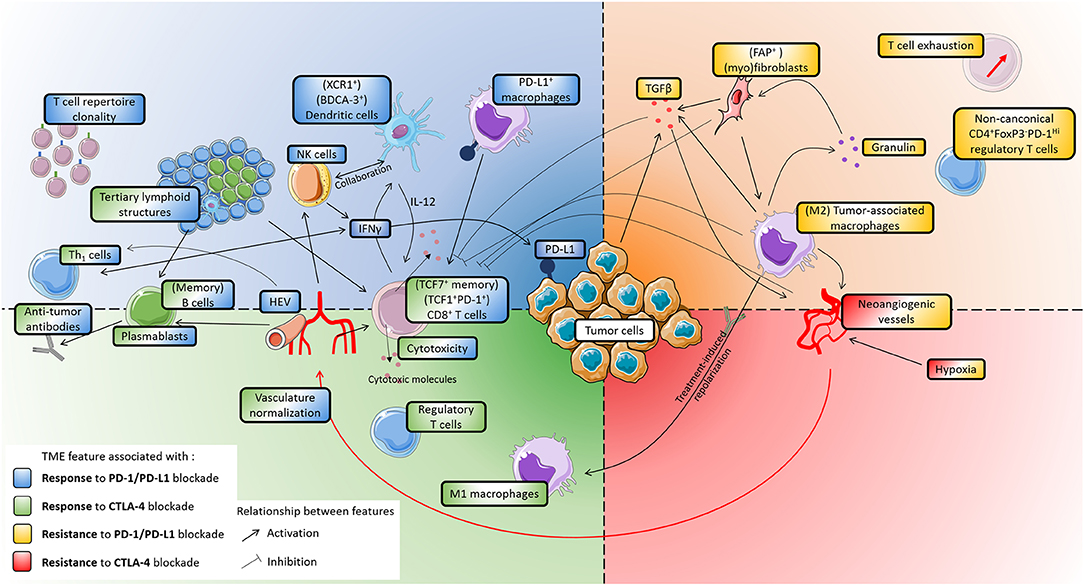
Figure 1. Main features of the tumor microenvironment that influence patients' response to immune checkpoint blockade. The figure is divided in four quarters, corresponding to association with either response or resistance, to either CTLA-4 or PD-1/PD-L1 blockade. Activating or inhibitory relationships between TME features are indicated by black arrows. Whenever phenotypes are indicated between brackets, it indicates that studies identified particular subsets as being associated with patients' response. PD-L1 is the only target of ICB that is shown, as it is the only one which expression was directly shown to associate with patients' response. Upper left (blue), features associated with increased response to PD-1/PD-L1 blockade: T cell repertoire clonality, NK cells, Dendritic cells, PD-L1+ macrophages, high endothelial venules, IFNγ. Lower left (green), features associated with increased response to CTLA-4 blockade: M1-polarized macrophages, regulatory T cells. (Left) (green and blue), some features are associated with an increased response to both CTLA-4 and PD-1/PD-L1 bloackade: CD8+ T cells, cytotoxicity, tertiary lymphoid structures, Th1 cells, B cells, plasmablasts, antibodies, vasculature normalization. Upper right (yellow), features associated with resistance to PD-1/PD-L1 blockade: M2-polarized macrophages, granulin, fibroblasts, TGFβ, T cell exhaustion, non-canonical regulatory T cells. Lower right (red): no markers were identified as being associated solely with resistance to CTLA-4 blockade. (Right) (yellow and red), features associated with resistance to both CTLA-4 and PD-1/PD-L1 blockade: hypoxia, neoangiogenic vessels. Cell drawings originate from Servier Medical Art (https://smart.servier.com), distributed under a CC-BY 3.0 Attribution license (https://creativecommons.org/licenses/by/3.0/).
Importance of the TME in Response and Resistance to Immune Checkpoint Blockade Therapy
T Cells Generally Favor ICB Efficacy but Their Diversity and Functionality Are Crucial
ICB therapies were designed to reinvigorate efficacious anti-tumor immune responses mainly mediated by T cells (80, 81). The relationship between the density and localization of CD8+ T cells and the response to PD-1 blockade in melanoma (16), as well as to PD-L1 blockade in various cancer types (35), has been analyzed, and a higher density of CD8+ T cells, both in the tumor core and in the invasive margin, was shown to correlate with an increased response to PD-1/PD-L1 blockade. A metric known as the Immunoscore (5), that accounts for the density of CD3+ and CD8+ T cells in the tumor core and the invasive margin and has prognostic value (6), could be used to predict patient response to ICB (82, 83).
However, the mere presence of CD8+ T cells is not entirely informative of whether patients are likely to respond to ICB. The phenotype and functionality of these cells also need to be analyzed. The development of single-cell RNA sequencing technology has highlighted the high variability that is inherent to tumor-infiltrating T cells in many malignancies (38, 84–91). In melanoma, dysfunctional CD8+ T cells were shown to form a major proliferative compartment (91). Interestingly, in mouse models, some dysfunctional CD8+ T cells are nonetheless tumor-specific (92) and a subpopulation can be reactivated (92, 93). Hence, the cytotoxicity of CD8+ T cells must be considered. A study investigating the changes in the TME during anti-PD-1 treatment of anti-CTLA-4-resistant melanoma patients reported that, although the density of tumor-infiltrating lymphocytes did not significantly change, their cytolytic activity increased (36). Pre-treatment expression of cytotoxicity-related genes, notably GZMA, is also related to PD-1 blockade response (43). In melanoma, a higher expression of gene signatures related to T cell cytotoxicity was associated with the clinical activity of the anti-CTLA-4 ipilimumab (37). Moreover, regardless of the overall T cell infiltration, an increased presence of memory-like CD8+TCF7+ T cells (as opposed to generally exhausted CD8+TCF7− T cells) is associated with an increased response to PD-1 blockade in patients with metastatic melanoma (38).
The TME harbors both tissue-resident T cells and newly infiltrating T cells that enter via the bloodstream. The involvement of tissue-resident T cells in ICB has been investigated. In lung cancer, tumors with a higher expression of tissue-resident memory cell marker CD103 on tumor-infiltrating cytotoxic lymphocytes displayed an enhanced T cell cytotoxicity, independently of an increased cytotoxic T cell density (94). In melanoma, a subset of T cells expressing TCF1 [a marker of self-renewable CD8+ T cells (54, 95) that proliferate after PD-1 blockade (54)] and PD-1 was identified (39). In mice, these Tcf1+PD-1+ tumor infiltrating lymphocytes were shown to be able to proliferate, and to mediate durable responses to ICB (39). Thus, rather than rejuvenating differentiated CD8+ T cells, ICB seems to act on less-differentiated memory-like CD8+ T cells that expand into a pool of effector CD8+ T cells.
CD4+ T cell subsets also play critical roles in tumor immunology and immunotherapy (96). Several subsets of effector CD4+ Th1 cells have been shown to be more abundant in melanoma tumors responding to CTLA-4 inhibition (40). Moreover, a recent study in a sarcoma mouse model revealed that presentation of peptides by tumor cells on class II major histocompatibility complex (MHC) and their recognition by CD4+ Th1 cells was crucial to generate functional CD8+ T cell responses during ICB with anti-PD-1, anti-CTLA-4, or combination of both (41).
CD4+FoxP3+ regulatory T cells (Treg) are generally associated with a poor prognosis in several cancers as suppressors of antitumor immune responses (97). However, since CTLA-4 is constitutively expressed on Tregs, their presence has been shown to be associated with response to CTLA-4 blockade (42). Interestingly, another study (98) revealed that the efficacy of CTLA-4 inhibition relied on Treg depletion during treatment. The impact of anti-CTLA-4 treatment on the relative composition of the T cell infiltrate is disputed. An increase in CD4+ and CD8+ T cell infiltration is observed, and in one study, the Treg compartment was not depleted (99), which was not the case in other works (98). Tregs have also been related to hyperprogression, i.e., ICB-related tumor growth acceleration. For instance, in gastric cancer, it was shown that PD-1 blockade increased Treg infiltration, which subsequently promoted hyperprogression (100). Non-conventional inhibitory T cells have also been studied with regards to ICB (73). These CD4+FoxP3−PD-1Hi (4PD1hi) T cells, which have immunosuppressive functions and present similarities with follicular helper T cells, have distinct behaviors in tumors treated by PD-1 or CTLA-4 blockade. CTLA-4 inhibition increases the number of 4PD1hi T cells while PD-1 blockade depletes them and mitigates their inhibitory functions. The persistence of these cells after PD-1 blockade is considered a negative prognostic factor (100).
To mediate a potential antitumor response, T cells must be tumor-reactive, i.e., recognize a peptide presented by the MHC on tumor cells. Several parts of this mechanism are closely related to ICB response (101). Some studies reported that T cell receptor repertoire clonality, i.e., the expansion of specific T cell clones, was associated with response to PD-1 blockade (16, 36, 43, 44). Similar results were reported for PD-L1 blockade in several lung, endometrial, colorectal and kidney malignancies, and this clonality was also observed in peripheral T cells, which may open the way for non-invasive testing (45). Downregulation of antigen presenting machinery and loss of heterozygosity of class I HLA genes also impair response to ICB (102–104). In melanoma, class II MHC profiling has been proposed as a biomarker of response to ICB, alone (105, 106) or in combination with PD-L1 detection (107).
The central position of T cells in immunotherapy is also highlighted by the pivotal role of interferon gamma (IFNγ) in the interplay between TME components and in mediating response to ICB. IFNγ can be secreted by many different cell types, notably cytotoxic T cells, Th1 and NK cells. It promotes cytotoxicity of CD8+ T cells and NK cells, favors Th1 polarization of CD4+ T cells, upregulates antigen presentation and activates M1 macrophages. Furthermore, it inhibits cell growth and favors apoptosis (108). IFNγ also upregulates PD-L1 expression on neighboring cells (109). IFNγ signaling was shown to be associated with a higher response rate to PD-(L)1 blockade in NSCLC (48, 49), urothelial carcinoma (48) and melanoma (46, 49). A T-cell inflamed gene expression signature strongly correlating with IFNγ signaling proved efficient in predicting response to PD-1 blockade in a pan-cancer study (47), suggesting this may have broader significance.
B Cells and Tertiary Lymphoid Structures: Emerging Factors Associated With Increased ICB Response
Although T cells are key effectors of antitumor immunity, there is a growing interest in the role of B cells for ICB. Although there have been reports that B cell absence or depletion did not prevent anti-PD-1 efficacy in melanoma (110), other studies have indicated a favorable impact of B cells on ICB. Single-cell studies of tumor-infiltrating immune cells revealed that melanoma tumors responding to PD-1 blockade exhibited increased numbers of B cells (38). A recent re-analysis of the same dataset revealed that subsets of B cells varied strongly between responders and non-responders, with higher infiltration by memory-like B cells and plasmablast-like cells in tumors of responders (55). An increased presence of plasmablasts had previously been described in the blood of melanoma, lung, and renal cell carcinomas patients responding to anti-CTLA-4 and anti-PD-1 therapies (56). A gene signature related to memory B cells (111) was reported to be associated with clinical benefit and improved survival in anti-PD-1- and anti-CTLA-4-treated melanoma patients and in anti-PD-L1-treated urothelial carcinoma (112). B-cell-secreted antibodies have also been related to ICB response. In particular, higher quantities of melanoma-specific antibodies were found in the serum of patients responding to CTLA-4 or PD-1 inhibition (58). In HPV-related cancers treated by PD-1 blockade, increased IgG and IgM antibody response was found in the serum of responders but not non-responders (59). In clear cell renal cell carcinoma, IgG-containing immune complexes could lead to classical complement pathway activation, which was associated with a higher expression of immune checkpoint molecules (113). This suggests a relationship between B cells, complement system activation and immune exhaustion to be further explored in light of ICB advances. Moreover, since B cells can also express immune checkpoint molecules such as PD-1, principally on regulatory B cells (114), or CTLA-4 (115), it is likely that ICB treatment can also have a direct effect on B cells. Murine models of HPV-induced head and neck squamous cell carcinoma showed that radiotherapy and PD-1 blockade induced memory B-cells, plasma cells, and antigen-specific B-cells, as well B-cell germinal center formation (59).
B cells are a crucial component of tertiary lymphoid structures (TLS), lymph-node-like lymphocytic aggregates that are sites for activation and maturation of antitumor immune responses (116). Recent studies addressed the question of the impact of tumor infiltration by B cells and presence of TLS on patient survival and response to PD-1 blockade in soft-tissue sarcoma (50) and to PD-1 and CTLA-4 blockade in melanoma (51, 52). These studies reveal that groups of patients marked by the presence of TLS and a stronger infiltration by B cells present a higher response rate to ICB. Work on murine models of breast cancer suggest an activation of B cells and follicular helper T cells (Tfh), a T cell subset found in TLS, by ICB, and an influence of these cells on response (53). Moreover, induction of TLS improved the efficacy of ICB in checkpoint-blockade-resistant tumors in a mouse model (57). Presence of CXCL13-producing PD-1Hi CD8+ T cells in TLS has been associated with response to PD-1 blockade in NSCLC (117). However, mice deficient for Tfh were shown to present an improved response to CTLA-4 blockade (73). Therefore, the impact of TLS on ICB response is likely to have a broad applicability, although not universal.
Innate Immune Cells: Functionality Is the Key
Besides the adaptive immune cells presented above, the immune TME can also harbor various innate immune populations, such as dendritic cells, macrophages, or NK cells, with critical effects on tumor evolution. Increasing efforts focus on exploiting them to treat cancer (118). Moreover, functionality-based subtypes of each of these populations have been shown to influence response or resistance to ICB.
To exert effective antitumor activity, CD8+ T cells require priming by antigen presenting cells, such as dendritic cells (DC). Dendritic cells can be classified as classical dendritic cells (cDCs) and specialized type I interferon-producing plasmacytoid dendritic cells (pDCs). cDCs are further divided into cDC1 with enhanced exogenous antigen cross-presentation to CD8+ T cells, and cDC2 cells which favor presentation to CD4+ T cells inducing Th-2 or Th-17 responses (96, 119). A recent study using mouse models revealed that effective PD-1 blockade therapy requires the presence of IL-12-producing cDC1 (60). During anti-PD-1 treatment, DCs respond to IFNγ produced by neighboring T cells by secreting IL-12, thereby enhancing T-cell-mediated tumor cell killing (60). In renal cell carcinoma, a study reported that a higher expression of genes related to cross-presenting DCs, including XCR1, was associated with better survival of patients treated with PD-L1 blockade (62). A stimulatory DC subset, identifiable by expression of BDCA-3 and CLEC9A markers (120), is associated with anti-PD-1 immunotherapy response in melanoma patients (63). The BDCA-3+CLEC9A+ DC subset (which produces high levels of IFNα) induces Th1 polarization and exhibits superior capacity to cross-present dead cell-associated antigens (121), suggesting that it plays an essential role in the induction of antitumor responses. Interestingly, this subset also expresses chemokine receptor XCR1 and interacts with XCL1-producing NK cells, which were also found to be associated with anti-PD-1 response in melanoma (63). PD-L1 can also bind CD80, a co-stimulatory molecule expressed on DCs, and repress its availability (61). It was shown that PD-L1 inhibition could allow a higher expression of CD80 on DCs and therefore a stronger priming of T cell responses through the binding of CD80 with CD28 on T cells. In the same study, a DC gene signature (including XCR1, BATF3, FLT3, and IRF8) expression correlated with increased survival of patients treated with PD-L1 blockade in NSCLC and renal cell carcinoma (61).
Macrophages form another critical innate immune population, capable of phagocytosis, and antigen presentation. Although their functionality spectrum can be more complex, two opposite states of polarization are often distinguished. Classically activated M1 macrophages favor CD8+ T cell activation through antigen presentation and cytokine secretion. In contrast, alternatively activated M2 macrophages have pro-angiogenic and immunosuppressive properties and secrete TGFβ (122). In NSCLC, the expression of PD-L1 on immune cells is mostly found on the surface of CD68+ macrophages. This macrophage expression of PD-L1 is associated with an increased CD8+ T cell infiltration in tumors and an increased survival of patients when they are treated with PD-(L)1 blockade therapies (64). In lung squamous cell carcinoma, stromal macrophages, activated through CSF-1R (the receptor of colony-stimulating factor-1 (CSF-1), a macrophage growth factor), trap CD8+ T cells and prevent them from entering the tumor core, thereby limiting the efficacy of PD-1 blockade therapy (74). This phenomenon was also reported in liver metastases of pancreatic ductal adenocarcinoma, in which it was shown that CSF-1 induced granulin expression by macrophages. This led to the recruitment of myofibroblasts that retained CD8+ T cells outside of the tumor (75). Tumor-associated macrophages (TAMs) can also have other immunosuppressive effects (123), through secretion of IL-10 and TGFβ and the expression of immune checkpoint molecules PD-L1, PD-L2, CTLA-4 ligands CD80 and CD86 (124), and PD-1 (125). M2-polarized macrophages could also contribute to hyperprogression. Indeed, a study in NSCLC showed that patients with hyperprogression under PD-(L)1 inhibition showed increased tumor infiltration by M2 macrophages, and suggested that macrophage reprogramming to the M2 phenotype might have occurred as a result of the binding of treatment antibodies to macrophage Fc receptors (126). Interestingly, PD-1 can be expressed on macrophages that are mostly of the protumor M2 phenotype, and PD-(L)1-blockade therapy could revert their function toward an antitumor M1 phenotype that can engage in malignant cell phagocytosis (125). Moreover, in melanoma patients, a higher infiltration by CD68+CD16+ classically activated (M1) macrophages was found in tumors of responders to CTLA-4 as compared to non-responders (65).
NK cells are innate lymphocytes that do not have antigen-specific receptors. They are able to sense cells lacking expression of class I MHC and can exert cytotoxic functions or secrete cytokines. Evidence point to the presence of PD-1+ NK cells with an activated phenotype (127, 128). However, the binding with PD-L1 mediates a dysfunction of these NK cells (127, 129). Consequently, NK cells are likely to weigh in PD-1-axis inhibition. This is reinforced by the fact that, in mouse models, depletion of NK cells abolished the effects of PD-1 and PD-L1 inhibition (66). Notably, several novel checkpoint inhibitors targeting NK cells are being tested in clinical trials (118, 127).
Evolution of the TME During Treatment and Response
The composition of the TME is dynamic and evolves during ICB therapies. Several studies have pointed to differential evolution of the TME between responders and non-responders. In longitudinal studies of melanoma patients treated with ICB (36, 130, 131). Interestingly, the TME composition differences between responders and non-responders were found to be stronger early on-treatment than before ICB (130, 131). Indeed, the differences in the densities of CD4+ or CD8+ T cells were more profoundly visible after two or three anti-PD-1 doses than at baseline (130). Similarly, analysis of on-treatment biopsies revealed a difference between responders and non-responders in the expression of PD-1 (130) or PD-L1 (130, 131). These observations stand for either anti-CTLA-4-progressors treated with PD-1 blockade (130), or for patients treated with PD-1 inhibitor alone or in combination with CTLA-4 blockade (131). Response to ICB is accompanied by more global changes in the TME composition. An increase in the numbers of CD8+ T cells and NK cells in tumors of responders to PD-1 inhibitors, as well as a decrease in macrophage infiltration were shown (36). Tumors responding to ICB also revealed an increase in B cells and TLS (51, 52). T cell receptor (TCR) repertoire clonality of responders was found to be increased during therapy (36, 131). In a trial combining CTLA-4 blockade with an demethylating agent, an increase in CD8+ T cell and PD-1 expression was reported (132).
The functionality of the TME can also be significantly impacted by ICB. During PD-1 blockade, responders showed an increased expression of the T-cell exhaustion marker LAG3 (130). Conversely, the upregulation of exhausted T cells during PD-1 therapy, as seen by expression of alternative inhibitory checkpoints LAG-3 or TIM-3, has been linked to adaptive resistance to PD-1 treatment in lung cancer in humans (71) and in mouse models (72). In NSCLC and melanoma patients, a higher expression of CD38 in patients with high basal or treatment-induced T cell infiltration was associated with adaptive resistance to PD-(L)1 inhibition (133). Interestingly, PD-1 blockade was also found to induce clonal replacement preferentially of exhausted CD8+ T cells compared to any other T cell subset (134). This could mean that T cells present at baseline may show reduced proliferation, and that the response to ICB could be due to T cell clones that enter the tumor during the course of treatment.
Stromal Tumor Microenvironment: Complex Interactions With Immune and Tumor Cells
The TME forms a complex system in which tumor cells interact not only with immune cells but also with non-immune stromal cells. The two main stromal cell types are endothelial cells, forming vessels, and fibroblasts. Since blood and lymphatic vessels partly govern the extent of the immune infiltration of the tumor, their involvement in response to ICB has been analyzed. In hypoxic conditions, tumors activate neoangiogenesis, forming abnormal vasculature that has been proposed to reduce the infiltration of lymphocytes (135). This neoangiogenic vasculature can be specifically targeted to normalize the vasculature. Several studies have demonstrated that normalizing the vasculature facilitated the entry of immune cells into the tumor, and may therefore enhance responsiveness to ICB (67, 69). This approach is further supported by in breast and colon murine models treated with PD-1 or CTLA-4 blockade, response to ICB was associated with increased vessel perfusion, which is a measure of proper vascular function (68). This could explain the success of combinatory treatments with anti-angiogenic drugs and PD-1 or PD-L1 blockade in mouse tumor models (70, 136), which relies on the normalization of the tumor vasculature (136) and promotion of high endothelial venules (70), a vessel type specialized in lymphocyte trafficking and found in secondary lymphoid organs and TLS (137). Hypoxia, which leads to neoangiogenic vessel formation, is also implicated in response to ICB. In murine models of melanoma (76) and prostate cancer (77), targeting hypoxia products led to the sensitization of the tumors to ICB.
Fibroblasts, which are the other main stromal population in the TME, can also play a critical role in response or resistance to ICB. Fibroblasts expressing the marker fibroblast activation protein-alpha (FAP) induced resistance to PD-1 inhibitors in colorectal cancer murine models by promoting immunosuppression, recruiting myeloid cells and inhibiting T cell activity (138). In metastatic pancreatic cancer, a decrease in αSMA+ fibroblast numbers by targeting macrophages has been associated with enhanced response to PD-1 blockade (75). Two recent studies point to the role of TGFβ in stroma-mediated resistance to PD-L1 blockade (78, 79). In a murine model of colorectal cancer with TGFβ-activated stroma, blockade of PD-L1 only leveraged limited responses, but resistance was overcome upon combination with TGFβ inhibition (78). Similar findings were observed in a model of metastatic liver cancer (78). This ICB-potentiating effect of TGFβ inhibition was also demonstrated in metastatic urothelial cancer patients (79). Increased TGFβ signaling was found in “immune-low” tumors, and TGFβ blockade co-administered with anti-PD-L1 showed anti-tumor strong responses in a murine urothelial cancer model, whereas neither treatment showed activity alone (79). Together, these results reveal the central role of stroma-derived TGFβ in inducing immune evasion and resistance to ICB.
Conclusions
Immune checkpoint blockade has promoted a revolution of cancer treatment. Its mechanism of action is tightly related to the action of cytotoxic CD8+ T cells. However, a growing body of evidence points to relationships between patients' response and other components of the tumor microenvironment. The TME is indeed an intricated assembly of many cell populations that influence the abundance and functionality of the neighboring cells. Each cell population found in the TME, immune or stromal, influences response to ICB. This is particularly striking with the role of tertiary lymphoid structures, which combine T cells, B cells, and dendritic cells to allow a maturation and activation of an antitumoral immune response.
In the past years, several promising immune checkpoints that could also be inhibited have emerged. These notably include T cell-related inhibitory molecules such as Tim-3 or Lag-3, and TIGIT, expressed both by T cells and NK cells in the TME (139). However, there is still a lack or robust validated markers associated with response or resistance to these emerging treatments.
The future of research for biomarkers of response may be to analyze the TME composition as a whole instead of analyzing each population separately. To do so, many different approaches are available (140), including many computational approaches harnessing tumor transcriptomics (141), and are now extended to single-cell analyses. These investigations will be complemented by further studies of the locations of cells in the TME, by high-throughput multispectral imaging or spatial transcriptomics (142–144). The integration of these different approaches should allow the emergence of novel composite biomarkers that are necessary to predict patients' responses to the combination of antitumor and anti-TME therapies in the future.
Author Contributions
FP, MM, and WF wrote the manuscript, which was amended and validated by all authors.
Funding
This project has received funding from the European Union's Horizon 2020 research and innovation programme under grant agreement No. 754923.
Disclaimer
The material presented and views expressed here are the responsibility of the authors only. The EU Commission takes no responsibility for any use made of the information set out.
Conflict of Interest
The authors declare that the research was conducted in the absence of any commercial or financial relationships that could be construed as a potential conflict of interest.
Acknowledgments
This work was supported by the Institut National de la Santé et de la Recherche Médicale, Université de Paris, Sorbonne Université and the Programme Cartes d'Identité des Tumeurs of the Ligue Nationale contre le cancer.
References
1. Hanahan D, Weinberg RA. Hallmarks of cancer: the next generation. Cell. (2011) 144:646–74. doi: 10.1016/j.cell.2011.02.013
2. Dunn GP, Old LJ, Schreiber RD. The three Es of cancer immunoediting. Annu Rev Immunol. (2004) 22:329–60. doi: 10.1146/annurev.immunol.22.012703.104803
3. Fridman WH, Pagès F, Sautès-Fridman C, Galon J. The immune contexture in human tumours: impact on clinical outcome. Nat Rev Cancer. (2012) 12:298–306. doi: 10.1038/nrc3245
4. Fridman WH, Zitvogel L, Sautès–Fridman C, Kroemer G. The immune contexture in cancer prognosis and treatment. Nat Rev Clin Oncol. (2017) 14:717–34. doi: 10.1038/nrclinonc.2017.101
5. Galon J, Costes A, Sanchez-Cabo F, Kirilovsky A, Mlecnik B, Lagorce-Pagès C, et al. Type, density, and location of immune cells within human colorectal tumors predict clinical outcome. Science. (2006) 313:1960–4. doi: 10.1126/science.1129139
6. Pagès F, Mlecnik B, Marliot F, Bindea G, Ou FS, Bifulco C, et al. International validation of the consensus immunoscore for the classification of colon cancer: a prognostic and accuracy study. Lancet. (2018) 391:2128–39. doi: 10.1016/S0140-6736(18)30789-X
7. Mahmoud SMA, Paish EC, Powe DG, Macmillan RD, Grainge MJ, Lee AHS, et al. Tumor-infiltrating CD8+ lymphocytes predict clinical outcome in breast cancer. J Clin Oncol. (2011) 29:1949–55. doi: 10.1200/JCO.2010.30.5037
8. Gao Q, Qiu SJ, Fan J, Zhou J, Wang XY, Xiao YS, et al. Intratumoral balance of regulatory and cytotoxic T cells is associated with prognosis of hepatocellular carcinoma after resection. J Clin Oncol. (2007) 25:2586–93. doi: 10.1200/JCO.2006.09.4565
9. Medrek C, Pontén F, Jirström K, Leandersson K. The presence of tumor associated macrophages in tumor stroma as a prognostic marker for breast cancer patients. BMC Cancer. (2012) 12:306. doi: 10.1186/1471-2407-12-306
10. Sugimoto M, Mitsunaga S, Yoshikawa K, Kato Y, Gotohda N, Takahashi S, et al. Prognostic impact of M2 macrophages at neural invasion in patients with invasive ductal carcinoma of the pancreas. Eur J Cancer. (2014) 50:1900–08. doi: 10.1016/j.ejca.2014.04.010
11. Jensen TO, Schmidt H, Møller HJ, Høyer M, Maniecki MB, Sjoegren P, et al. Macrophage markers in serum and tumor have prognostic impact in American joint committee on cancer stage I/II melanoma. J Clin Oncol. (2009) 27:3330–7. doi: 10.1200/JCO.2008.19.9919
12. Petitprez F, Vano YA, Becht E, Giraldo NA, Reyniès A, de Sautès-Fridman C, et al. Transcriptomic analysis of the tumor microenvironment to guide prognosis and immunotherapies. Cancer Immunol Immunother. (2017) 67:981–8. doi: 10.1007/s00262-017-2058-z
13. Fridman WH, Miller I, Sautès-Fridman C, Byrne AT. Therapeutic targeting of the colorectal tumor stroma. Gastroenterology. (2020) 158:303–21. doi: 10.1053/j.gastro.2019.09.045
14. Pardoll DM. The blockade of immune checkpoints in cancer immunotherapy. Nat Rev Cancer. (2012) 12:252–64. doi: 10.1038/nrc3239
15. Topalian SL, Drake CG, Pardoll DM. Immune checkpoint blockade: a common denominator approach to cancer therapy. Cancer Cell. (2015) 27:450–61. doi: 10.1016/j.ccell.2015.03.001
16. Tumeh PC, Harview CL, Yearley JH, Shintaku IP, Taylor EJM, Robert L, et al. PD-1 blockade induces responses by inhibiting adaptive immune resistance. Nature. (2014) 515:568–71. doi: 10.1038/nature13954
17. Topalian SL, Hodi FS, Brahmer JR, Gettinger SN, Smith DC, McDermott DF, et al. Safety, activity, and immune correlates of anti-PD-1 antibody in cancer. N Engl J Med. (2012) 366:2443–54. doi: 10.1056/NEJMoa1200690
18. Daud AI, Wolchok JD, Robert C, Hwu WJ, Weber JS, Ribas A, et al. Programmed death-ligand 1 expression and response to the anti-programmed death 1 antibody pembrolizumab in melanoma. J Clin Oncol. (2016) 34:4102–9. doi: 10.1200/JCO.2016.67.2477
19. Center for Devices and Radiological Health. List of Cleared or Approved Companion Diagnostic Devices (In Vitro and Imaging Tools). FDA (2019). Available online at: /medical-devices/vitro-diagnostics/list-cleared-or-approved-companion-diagnostic-devices-vitro-and-imaging-tools (accessed May 20, 2019)
20. Nishino M, Ramaiya NH, Hatabu H, Hodi FS. Monitoring immune-checkpoint blockade: response evaluation and biomarker development. Nat Rev Clin Oncol. (2017) 14:655–68. doi: 10.1038/nrclinonc.2017.88
21. Alexandrov LB, Nik-Zainal S, Wedge DC, Aparicio SAJR, Behjati S, Biankin AV, et al. Signatures of mutational processes in human cancer. Nature. (2013) 500:415–21. doi: 10.1038/nature12477
22. Snyder A, Makarov V, Merghoub T, Yuan J, Zaretsky JM, Desrichard A, et al. Genetic basis for clinical response to CTLA-4 blockade in melanoma. N Engl J Med. (2014) 371:2189–99. doi: 10.1056/NEJMoa1406498
23. Rizvi NA, Hellmann MD, Snyder A, Kvistborg P, Makarov V, Havel JJ, et al. Cancer immunology. mutational landscape determines sensitivity to PD-1 blockade in non-small cell lung cancer. Science. (2015) 348:124–8. doi: 10.1126/science.aaa1348
24. Van Allen EM, Miao D, Schilling B, Shukla SA, Blank C, Zimmer L, et al. Genomic correlates of response to CTLA-4 blockade in metastatic melanoma. Science. (2015) 350:207–11. doi: 10.1126/science.aad0095
25. Goodman AM, Kato S, Bazhenova L, Patel SP, Frampton GM, Miller V, et al. Tumor mutational burden as an independent predictor of response to immunotherapy in diverse cancers. Mol Cancer Ther. (2017) 16:2598–608. doi: 10.1158/1535-7163.MCT-17-0386
26. Le DT, Uram JN, Wang H, Bartlett BR, Kemberling H, Eyring AD, et al. PD-1 blockade in tumors with mismatch-repair deficiency. N Engl J Med. (2015) 372:2509–20. doi: 10.1056/NEJMoa1500596
27. Le DT, Durham JN, Smith KN, Wang H, Bartlett BR, Aulakh LK, et al. Mismatch repair deficiency predicts response of solid tumors to PD-1 blockade. Science. (2017) 357:409–13. doi: 10.1126/science.aan6733
28. Marabelle A, Le DT, Ascierto PA, Di Giacomo AM, De Jesus-Acosta A, Delord JP, et al. Efficacy of pembrolizumab in patients with noncolorectal high microsatellite instability/mismatch repair-deficient cancer: results from the phase II KEYNOTE-158 study. J Clin Oncol. (2020) 38:1–10. doi: 10.1200/JCO.19.02105
29. Colli LM, Machiela MJ, Myers TA, Jessop L, Yu K, Chanock SJ. Burden of nonsynonymous mutations among TCGA cancers and candidate immune checkpoint inhibitor responses. Cancer Res. (2016) 76:3767–72. doi: 10.1158/0008-5472.CAN-16-0170
30. Vétizou M, Pitt JM, Daillère R, Lepage P, Waldschmitt N, Flament C, et al. Anticancer immunotherapy by CTLA-4 blockade relies on the gut microbiota. Science. (2015) 350:1079–84. doi: 10.1126/science.aad1329
31. Routy B, Le Chatelier E, Derosa L, Duong CPM, Alou MT, Daillère R, et al. Gut microbiome influences efficacy of PD-1-based immunotherapy against epithelial tumors. Science. (2018) 359:91–97. doi: 10.1126/science.aan3706
32. Gopalakrishnan V, Spencer CN, Nezi L, Reuben A, Andrews MC, Karpinets TV, et al. Gut microbiome modulates response to anti-PD-1 immunotherapy in melanoma patients. Science. (2018) 359:97–103. doi: 10.1126/science.aan4236
33. Matson V, Fessler J, Bao R, Chongsuwat T, Zha Y, Alegre ML, et al. The commensal microbiome is associated with anti-PD-1 efficacy in metastatic melanoma patients. Science. (2018) 359:104–8. doi: 10.1126/science.aao3290
34. Elinav E, Garrett WS, Trinchieri G, Wargo J. The cancer microbiome. Nat Rev Cancer. (2019) 19:371–6. doi: 10.1038/s41568-019-0155-3
35. Herbst RS, Soria J-C, Kowanetz M, Fine GD, Hamid O, Gordon MS, et al. Predictive correlates of response to the anti-PD-L1 antibody MPDL3280A in cancer patients. Nature. (2014) 515:563–7. doi: 10.1038/nature14011
36. Riaz N, Havel JJ, Makarov V, Desrichard A, Urba WJ, Sims JS, et al. Tumor and microenvironment evolution during immunotherapy with nivolumab. Cell. (2017) 171:934–49.e16. doi: 10.1016/j.cell.2017.09.028
37. Ji R-R, Chasalow SD, Wang L, Hamid O, Schmidt H, Cogswell J, et al. An immune-active tumor microenvironment favors clinical response to ipilimumab. Cancer Immunol Immunother. (2012) 61:1019–31. doi: 10.1007/s00262-011-1172-6
38. Sade-Feldman M, Yizhak K, Bjorgaard SL, Ray JP, Boer CG, de Jenkins RW, et al. Defining T cell states associated with response to checkpoint immunotherapy in melanoma. Cell. (2018) 175:998–1013.e20. doi: 10.1016/j.cell.2018.10.038
39. Siddiqui I, Schaeuble K, Chennupati V, Fuertes Marraco SA, Calderon-Copete S, Pais Ferreira D, et al. Intratumoral Tcf1+PD-1+CD8+ T cells with stem-like properties promote tumor control in response to vaccination and checkpoint blockade immunotherapy. Immunity. (2019) 50:195–211.e10. doi: 10.1016/j.immuni.2018.12.021
40. Wei SC, Levine JH, Cogdill AP, Zhao Y, Anang N-AAS, Andrews MC, et al. Distinct cellular mechanisms underlie anti-CTLA-4 and anti-PD-1 checkpoint blockade. Cell. (2017) 170:1120–33.e17. doi: 10.1016/j.cell.2017.07.024
41. Alspach E, Lussier DM, Miceli AP, Kizhvatov I, DuPage M, Luoma AM, et al. MHC-II neoantigens shape tumour immunity and response to immunotherapy. Nature. (2019) 574:696–701. doi: 10.1038/s41586-019-1671-8
42. Hamid O, Schmidt H, Nissan A, Ridolfi L, Aamdal S, Hansson J, et al. A prospective phase II trial exploring the association between tumor microenvironment biomarkers and clinical activity of ipilimumab in advanced melanoma. J Trans Med. (2011) 9:204. doi: 10.1186/1479-5876-9-204
43. Inoue H, Park J-H, Kiyotani K, Zewde M, Miyashita A, Jinnin M, et al. Intratumoral expression levels of PD-L1, GZMA, and HLA-A along with oligoclonal T cell expansion associate with response to nivolumab in metastatic melanoma. Oncoimmunology. (2016) 5:e1204507. doi: 10.1080/2162402X.2016.1204507
44. Roh W, Chen P-L, Reuben A, Spencer CN, Prieto PA, Miller JP, et al. Integrated molecular analysis of tumor biopsies on sequential CTLA-4 and PD-1 blockade reveals markers of response and resistance. Sci Transl Med. (2017) 9:eaah3560. doi: 10.1126/scitranslmed.aah3560
45. Wu TD, Madireddi S, Almeida PE, de Banchereau R, Chen Y-JJ, Chitre AS, et al. Peripheral T cell expansion predicts tumour infiltration and clinical response. Nature. (2020) 579:274–8. doi: 10.1038/s41586-020-2056-8
46. Ayers M, Lunceford J, Nebozhyn M, Murphy E, Loboda A, Kaufman DR, et al. IFN-γ-related mRNA profile predicts clinical response to PD-1 blockade. J Clin Invest. (2017) 127:2930–40. doi: 10.1172/JCI91190
47. Cristescu R, Mogg R, Ayers M, Albright A, Murphy E, Yearley J, et al. Pan-tumor genomic biomarkers for PD-1 checkpoint blockade-based immunotherapy. Science. (2018) 362: eaar3593. doi: 10.1126/science.aar3593
48. Higgs BW, Morehouse C, Streicher KL, Brohawn P, Pilataxi F, Gupta A, et al. Interferon gamma messenger RNA signature in tumor biopsies predicts outcomes in patients with non-small-cell lung carcinoma or urothelial cancer treated with durvalumab. Clin Cancer Res. (2018) 24:3857–66. doi: 10.1158/1078-0432.CCR-17-3451
49. Karachaliou N, Gonzalez-Cao M, Crespo G, Drozdowskyj A, Aldeguer E, Gimenez-Capitan A, et al. Interferon gamma, an important marker of response to immune checkpoint blockade in non-small cell lung cancer and melanoma patients. Ther Adv Med Oncol. (2018) 10:1758834017749748. doi: 10.1177/1758834017749748
50. Petitprez F, Reyniès A, de Keung EZ, Chen TW-W, Sun C-M, Calderaro J, et al. B cells are associated with survival and immunotherapy response in sarcoma. Nature. (2020) 577:556–60. doi: 10.1038/s41586-019-1906-8
51. Helmink BA, Reddy SM, Gao J, Zhang S, Basar R, Thakur R, et al. B cells and tertiary lymphoid structures promote immunotherapy response. Nature. (2020) 577:549–55. doi: 10.1038/s41586-019-1922-8
52. Cabrita R, Lauss M, Sanna A, Donia M, Larsen MS, Mitra S, et al. Tertiary lymphoid structures improve immunotherapy and survival in melanoma. Nature. (2020) 577:561–5. doi: 10.1038/s41586-019-1914-8
53. Hollern DP, Xu N, Thennavan A, Glodowski C, Garcia-Recio S, Mott KR, et al. B cells and T follicular helper cells mediate response to checkpoint inhibitors in high mutation burden mouse models of breast cancer. Cell. (2019) 179:1191–206.e21. doi: 10.1016/j.cell.2019.10.028
54. Im SJ, Hashimoto M, Gerner MY, Lee J, Kissick HT, Burger MC, et al. Defining CD8+ T cells that provide the proliferative burst after PD-1 therapy. Nature. (2016) 537:417–21. doi: 10.1038/nature19330
55. Griss J, Bauer W, Wagner C, Simon M, Chen M, Grabmeier-Pfistershammer K, et al. B cells sustain inflammation and predict response to immune checkpoint blockade in human melanoma. Nat Commun. (2019) 10:1–14. doi: 10.1038/s41467-019-12160-2
56. DeFalco J, Harbell M, Manning-Bog A, Baia G, Scholz A, Millare B, et al. Non-progressing cancer patients have persistent B cell responses expressing shared antibody paratopes that target public tumor antigens. Clin Immunol. (2018) 187:37–45. doi: 10.1016/j.clim.2017.10.002
57. Johansson-Percival A, He B, Li Z-J, Kjellén A, Russell K, Li J, et al. De novo induction of intratumoral lymphoid structures and vessel normalization enhances immunotherapy in resistant tumors. Nat Immunol. (2017) 18:1207–17. doi: 10.1038/ni.3836
58. Fässler M, Diem S, Mangana J, Hasan Ali O, Berner F, Bomze D, et al. Antibodies as biomarker candidates for response and survival to checkpoint inhibitors in melanoma patients. J ImmunoTherapy Cancer. (2019) 7:50. doi: 10.1186/s40425-019-0523-2
59. Kim SS, Shen S, Miyauchi S, Sanders PD, Franiak-Pietryga I, Mell L, et al. B-cells improve overall survival in HPV-associated squamous cell carcinomas and are activated by radiation and PD-1 blockade. Clin Cancer Res. (2020). doi: 10.1158/1078-0432.CCR-19-3211. [Epub ahead of print].
60. Garris CS, Arlauckas SP, Kohler RH, Trefny MP, Garren S, Piot C, et al. Successful anti-PD-1 cancer immunotherapy requires T cell-dendritic cell crosstalk involving the cytokines IFN-γ and IL-12. Immunity. (2018) 49:1148–61.e7. doi: 10.1016/j.immuni.2018.09.024
61. Mayoux M, Roller A, Pulko V, Sammicheli S, Chen S, Sum E, et al. Dendritic cells dictate responses to PD-L1 blockade cancer immunotherapy. Sci Trans Med. (2020) 12: eaav7431. doi: 10.1126/scitranslmed.aav7431
62. Mayoux M, Fransen MF, Roller A, Matos I, Pulko V, Karanikas V, et al. Abstract 3658: dendritic cells dictate the responsiveness of PD-L1 blockade in cancer. Cancer Res. (2017) 77:3658. doi: 10.1158/1538-7445.AM2017-3658
63. Barry KC, Hsu J, Broz ML, Cueto FJ, Binnewies M, Combes AJ, et al. A natural killer-dendritic cell axis defines checkpoint therapy-responsive tumor microenvironments. Nat Med. (2018) 24:1178–91. doi: 10.1038/s41591-018-0085-8
64. Liu Y, Zugazagoitia J, Ahmed FS, Henick BS, Gettinger S, Herbst RS, et al. Immune cell PD-L1 co-localizes with macrophages and is associated with outcome in PD-1 pathway blockade therapy. Clin Cancer Res. (2019) 26:Clincanres. doi: 10.1158/1078-0432.CCR-19-1040
65. Romano E, Kusio-Kobialka M, Foukas PG, Baumgaertner P, Meyer C, Ballabeni P, et al. Ipilimumab-dependent cell-mediated cytotoxicity of regulatory T cells ex vivo by nonclassical monocytes in melanoma patients. Proc Natl Acad Sci USA. (2015) 112:6140–5. doi: 10.1073/pnas.1417320112
66. Hsu J, Hodgins JJ, Marathe M, Nicolai CJ, Bourgeois-Daigneault M-C, Trevino TN, et al. Contribution of NK cells to immunotherapy mediated by PD-1/PD-L1 blockade. J Clin Invest. (2018) 128:4654–68. doi: 10.1172/JCI99317
67. Elia AR, Grioni M, Basso V, Curnis F, Freschi M, Corti A, et al. Targeting tumor vasculature with TNF leads effector T cells to the tumor and enhances therapeutic efficacy of immune checkpoint blockers in combination with adoptive cell therapy. Clin Cancer Res. (2018) 24:2171–81. doi: 10.1158/1078-0432.CCR-17-2210
68. Zheng X, Fang Z, Liu X, Deng S, Zhou P, Wang X, et al. Increased vessel perfusion predicts the efficacy of immune checkpoint blockade. J Clin Invest. (2018) 128:2104–115. doi: 10.1172/JCI96582
69. Calcinotto A, Grioni M, Jachetti E, Curnis F, Mondino A, Parmiani G, et al. Targeting TNF-α to neoangiogenic vessels enhances lymphocyte infiltration in tumors and increases the therapeutic potential of immunotherapy. J Immunol. (2012) 188:2687–94. doi: 10.4049/jimmunol.1101877
70. Allen E, Jabouille A, Rivera LB, Lodewijckx I, Missiaen R, Steri V, et al. Combined antiangiogenic and anti-PD-L1 therapy stimulates tumor immunity through HEV formation. Sci Transl Med. (2017) 9:eaak9679. doi: 10.1126/scitranslmed.aak9679
71. Thommen DS, Schreiner J, Müller P, Herzig P, Roller A, Belousov A, et al. Progression of lung cancer is associated with increased dysfunction of T cells defined by coexpression of multiple inhibitory receptors. Cancer Immunol Res. (2015) 3:1344–55. doi: 10.1158/2326-6066.CIR-15-0097
72. Koyama S, Akbay EA, Li YY, Herter-Sprie GS, Buczkowski KA, Richards WG, et al. Adaptive resistance to therapeutic PD-1 blockade is associated with upregulation of alternative immune checkpoints. Nat Commun. (2016) 7:10501. doi: 10.1038/ncomms10501
73. Zappasodi R, Budhu S, Hellmann MD, Postow MA, Senbabaoglu Y, Manne S, et al. Non-conventional inhibitory CD4+Foxp3-PD-1hi T cells as a biomarker of immune checkpoint blockade activity. Cancer Cell. (2018) 33:1017–32.e7. doi: 10.1016/j.ccell.2018.05.009
74. Peranzoni E, Lemoine J, Vimeux L, Feuillet V, Barrin S, Kantari-Mimoun C, et al. Macrophages impede CD8 T cells from reaching tumor cells and limit the efficacy of anti–PD-1 treatment. Proc Natl Acad Sci USA. (2018) 115:E4041–50. doi: 10.1073/pnas.1720948115
75. Quaranta V, Rainer C, Nielsen SR, Raymant ML, Ahmed MS, Engle DD, et al. Macrophage-derived granulin drives resistance to immune checkpoint inhibition in metastatic pancreatic cancer. Cancer Res. (2018) 78:4253–69. doi: 10.1158/0008-5472.CAN-17-3876
76. Chafe SC, McDonald PC, Saberi S, Nemirovsky O, Venkateswaran G, Burugu S, et al. Targeting hypoxia-induced carbonic anhydrase IX enhances immune-checkpoint blockade locally and systemically. Cancer Immunol Res. (2019) 7:1064–78. doi: 10.1158/2326-6066.CIR-18-0657
77. Jayaprakash P, Ai M, Liu A, Budhani P, Bartkowiak T, Sheng J, et al. Targeted hypoxia reduction restores T cell infiltration and sensitizes prostate cancer to immunotherapy. J Clin Invest. (2018) 128:5137–49. doi: 10.1172/JCI96268
78. Tauriello DVF, Palomo-Ponce S, Stork D, Berenguer-Llergo A, Badia-Ramentol J, Iglesias M, et al. TGFβ drives immune evasion in genetically reconstituted colon cancer metastasis. Nature. (2018) 554:538–43. doi: 10.1038/nature25492
79. Mariathasan S, Turley SJ, Nickles D, Castiglioni A, Yuen K, Wang Y, et al. TGFβ attenuates tumour response to PD-L1 blockade by contributing to exclusion of T cells. Nature. (2018) 554:544–8. doi: 10.1038/nature25501
80. Sharma P, Allison JP. The future of immune checkpoint therapy. Science. (2015) 348:56–61. doi: 10.1126/science.aaa8172
81. Ribas A, Wolchok JD. Cancer immunotherapy using checkpoint blockade. Science. (2018) 359:1350–5. doi: 10.1126/science.aar4060
82. Ascierto PA, Capone M, Urba WJ, Bifulco CB, Botti G, Lugli A, et al. The additional facet of immunoscore: immunoprofiling as a possible predictive tool for cancer treatment. J Transl Med. (2013) 11:54. doi: 10.1186/1479-5876-11-54
83. Galon J, Bruni D. Approaches to treat immune hot, altered and cold tumours with combination immunotherapies. Nat Rev Drug Discov. (2019) 18:197–218. doi: 10.1038/s41573-018-0007-y
84. Tirosh I, Izar B, Prakadan SM, Wadsworth MH, Treacy D, Trombetta JJ, et al. Dissecting the multicellular ecosystem of metastatic melanoma by single-cell RNA-seq. Science. (2016) 352:189–196. doi: 10.1126/science.aad0501
85. Lavin Y, Kobayashi S, Leader A, Amir ED, Elefant N, Bigenwald C, et al. Innate immune landscape in early lung adenocarcinoma by paired single-cell analyses. Cell. (2017) 169:750–65.e17. doi: 10.1016/j.cell.2017.04.014
86. Zheng C, Zheng L, Yoo J-K, Guo H, Zhang Y, Guo X, et al. Landscape of infiltrating T cells in liver cancer revealed by single-cell sequencing. Cell. (2017) 169:1342–56.e16. doi: 10.1016/j.cell.2017.05.035
87. Azizi E, Carr AJ, Plitas G, Cornish AE, Konopacki C, Prabhakaran S, et al. Single-cell map of diverse immune phenotypes in the breast tumor microenvironment. Cell. (2018) 174:1293–308.e36. doi: 10.1016/j.cell.2018.05.060
88. Guo X, Zhang Y, Zheng L, Zheng C, Song J, Zhang Q, et al. Global characterization of T cells in non-small-cell lung cancer by single-cell sequencing. Nat Med. (2018) 24:978–85. doi: 10.1038/s41591-018-0045-3
89. Savas P, Virassamy B, Ye C, Salim A, Mintoff CP, Caramia F, et al. Single-cell profiling of breast cancer T cells reveals a tissue-resident memory subset associated with improved prognosis. Nat Med. (2018) 24:986–93. doi: 10.1038/s41591-018-0078-7
90. Zhang L, Yu X, Zheng L, Zhang Y, Li Y, Fang Q, et al. Lineage tracking reveals dynamic relationships of T cells in colorectal cancer. Nature. (2018) 564:268–272. doi: 10.1038/s41586-018-0694-x
91. Li H, van der Leun AM, Yofe I, Lubling Y, Gelbard-Solodkin D, van Akkooi ACJ, et al. Dysfunctional CD8 T cells form a proliferative, dynamically regulated compartment within human melanoma. Cell. (2019) 176:775–89.e18. doi: 10.1016/j.cell.2018.11.043
92. Philip M, Fairchild L, Sun L, Horste EL, Camara S, Shakiba M, et al. Chromatin states define tumour-specific T cell dysfunction and reprogramming. Nature. (2017) 545:452–6. doi: 10.1038/nature22367
93. Singer M, Wang C, Cong L, Marjanovic ND, Kowalczyk MS, Zhang H, et al. A distinct gene module for dysfunction uncoupled from activation in tumor-infiltrating T cells. Cell. (2016) 166:1500–11.e9. doi: 10.1016/j.cell.2016.08.052
94. Ganesan A-P, Clarke J, Wood O, Garrido-Martin EM, Chee SJ, Mellows T, et al. Tissue-resident memory features are linked to the magnitude of cytotoxic T cell responses in human lung cancer. Nat Immunol. (2017) 18:940–50. doi: 10.1038/ni.3775
95. Kratchmarov R, Magun AM, Reiner SL. TCF1 expression marks self-renewing human CD8+ T cells. Blood Adv. (2018) 2:1685–90. doi: 10.1182/bloodadvances.2018016279
96. Borst J, Ahrends T, Babała N, Melief CJM, Kastenmüller W. CD4+ T cell help in cancer immunology and immunotherapy. Nat Rev Immunol. (2018) 18:635–47. doi: 10.1038/s41577-018-0044-0
97. Takeuchi Y, Nishikawa H. Roles of regulatory T cells in cancer immunity. Int Immunol. (2016) 28:401–9. doi: 10.1093/intimm/dxw025
98. Arce Vargas F, Furness AJS, Litchfield K, Joshi K, Rosenthal R, Ghorani E, et al. Fc effector function contributes to the activity of human anti-CTLA-4 antibodies. Cancer Cell. (2018) 33:649–63.e4. doi: 10.1016/j.ccell.2018.02.010
99. Sharma A, Subudhi SK, Blando J, Scutti J, Vence L, Wargo J, et al. Anti-CTLA-4 immunotherapy does not deplete FOXP3+ regulatory T Cells (Tregs) in human cancers. Clin Cancer Res. (2019) 25:1233–8. doi: 10.1158/1078-0432.CCR-18-0762
100. Kamada T, Togashi Y, Tay C, Ha D, Sasaki A, Nakamura Y, et al. PD-1+ regulatory T cells amplified by PD-1 blockade promote hyperprogression of cancer. Proc Natl Acad Sci USA. (2019) 116:9999–10008. doi: 10.1073/pnas.1822001116
101. Havel JJ, Chowell D, Chan TA. The evolving landscape of biomarkers for checkpoint inhibitor immunotherapy. Nat Rev Cancer. (2019) 19:133–50. doi: 10.1038/s41568-019-0116-x
102. Sade-Feldman M, Jiao YJ, Chen JH, Rooney MS, Barzily-Rokni M, Eliane J-P, et al. Resistance to checkpoint blockade therapy through inactivation of antigen presentation. Nat Commun. (2017) 8:1136. doi: 10.1038/s41467-017-01062-w
103. Gettinger S, Choi J, Hastings K, Truini A, Datar I, Sowell R, et al. Impaired HLA class I antigen processing and presentation as a mechanism of acquired resistance to immune checkpoint inhibitors in lung cancer. Cancer Discov. (2017) 7:1420–35. doi: 10.1158/2159-8290.CD-17-0593
104. Chowell D, Morris LGT, Grigg CM, Weber JK, Samstein RM, Makarov V, et al. Patient HLA class I genotype influences cancer response to checkpoint blockade immunotherapy. Science. (2018) 359:582–7. doi: 10.1126/science.aao4572
105. Johnson DB, Estrada MV, Salgado R, Sanchez V, Doxie DB, Opalenik SR, et al. Melanoma-specific MHC-II expression represents a tumour-autonomous phenotype and predicts response to anti-PD-1/PD-L1 therapy. Nat Commun. (2016) 7:10582. doi: 10.1038/ncomms10582
106. Rodig SJ, Gusenleitner D, Jackson DG, Gjini E, Giobbie-Hurder A, Jin C, et al. MHC proteins confer differential sensitivity to CTLA-4 and PD-1 blockade in untreated metastatic melanoma. Sci Transl Med. (2018) 10: eaar3342. doi: 10.1126/scitranslmed.aar3342
107. Johnson DB, Bordeaux J, Kim JY, Vaupel C, Rimm DL, Ho TH, et al. Quantitative spatial profiling of PD-1/PD-L1 interaction and HLA-DR/IDO-1 predicts improved outcomes of Anti-PD-1 therapies in metastatic melanoma. Clin Cancer Res. (2018) 24:5250–60. doi: 10.1158/1078-0432.CCR-18-0309
108. Akdis M, Aab A, Altunbulakli C, Azkur K, Costa RA, Crameri R, et al. Interleukins (from IL-1 to IL-38), interferons, transforming growth factor β, and TNF-α: receptors, functions, and roles in diseases. J Allergy Clin Immunol. (2016) 138:984–1010. doi: 10.1016/j.jaci.2016.06.033
109. Garcia-Diaz A, Shin DS, Moreno BH, Saco J, Escuin-Ordinas H, Rodriguez GA, et al. Interferon receptor signaling pathways regulating PD-L1 and PD-L2 expression. Cell Rep. (2017) 19:1189–201. doi: 10.1016/j.celrep.2017.04.031
110. Damsky W, Jilaveanu L, Turner N, Perry C, Zito C, Tomayko M, et al. B cell depletion or absence does not impede anti-tumor activity of PD-1 inhibitors. J ImmunoTher Cancer. (2019) 7:153. doi: 10.1186/s40425-019-0613-1
111. Varn FS, Wang Y, Mullins DW, Fiering S, Cheng C. Systematic pan-cancer analysis reveals immune cell interactions in the tumor microenvironment. Cancer Res. (2017) 77:1271–82. doi: 10.1158/0008-5472.CAN-16-2490
112. Varn FS, Wang Y, Cheng C. A B cell-derived gene expression signature associates with an immunologically active tumor microenvironment and response to immune checkpoint blockade therapy. Oncoimmunology. (2018) 8:e1513440. doi: 10.1080/2162402X.2018.1513440
113. Roumenina LT, Daugan MV, Noé R, Petitprez F, Vano YA, Sanchez-Salas R, et al. Tumor cells hijack macrophage-produced complement c1q to promote tumor growth. Cancer Immunol Res. (2019) 7:1091–105. doi: 10.1158/2326-6066.CIR-18-0891
114. Schildberg FA, Klein SR, Freeman GJ, Sharpe AH. Coinhibitory pathways in the B7-CD28 ligand-receptor family. Immunity. (2016) 44:955–72. doi: 10.1016/j.immuni.2016.05.002
115. Kuiper HM, Brouwer M, Linsley PS, van Lier RA, Other departments. Activated T cells can induce high levels of CTLA-4 expression on B cells. J Immunol. (1995) 155:1776–83.
116. Sautès-Fridman C, Petitprez F, Calderaro J, Fridman WH. Tertiary lymphoid structures in the era of cancer immunotherapy. Nat Rev Cancer. (2019) 19:307–25. doi: 10.1038/s41568-019-0144-6
117. Thommen DS, Koelzer VH, Herzig P, Roller A, Trefny M, Dimeloe S, et al. A transcriptionally and functionally distinct PD-1+ CD8+ T cell pool with predictive potential in non-small cell lung cancer treated with PD-1 blockade. Nat Med. (2018) 24:994–1004. doi: 10.1038/s41591-018-0057-z
118. Demaria O, Cornen S, Daëron M, Morel Y, Medzhitov R, Vivier E. Harnessing innate immunity in cancer therapy. Nature. (2019) 574:45–56. doi: 10.1038/s41586-019-1593-5
119. Guilliams M, Ginhoux F, Jakubzick C, Naik SH, Onai N, Schraml BU, et al. Dendritic cells, monocytes and macrophages: a unified nomenclature based on ontogeny. Nat Rev Immunol. (2014) 14:571–8. doi: 10.1038/nri3712
120. Broz ML, Binnewies M, Boldajipour B, Nelson AE, Pollack JL, Erle DJ, et al. Dissecting the tumor myeloid compartment reveals rare activating antigen-presenting cells critical for T cell immunity. Cancer Cell. (2014) 26:638–52. doi: 10.1016/j.ccell.2014.09.007
121. van der Aa E, van Montfoort N, Woltman AM. BDCA3+CLEC9A+ human dendritic cell function and development. Semi Cell Dev Biol. (2015) 41:39–48. doi: 10.1016/j.semcdb.2014.05.016
122. Murray PJ, Wynn TA. Protective and pathogenic functions of macrophage subsets. Nat Rev Immunol. (2011) 11:723–37. doi: 10.1038/nri3073
123. Cassetta L, Pollard JW. Targeting macrophages: therapeutic approaches in cancer. Nat Rev Drug Discov. (2018) 17:887–904. doi: 10.1038/nrd.2018.169
124. Noy R, Pollard JW. Tumor-associated macrophages: from mechanisms to therapy. Immunity. (2014) 41:49–61. doi: 10.1016/j.immuni.2014.06.010
125. Gordon SR, Maute RL, Dulken BW, Hutter G, George BM, McCracken MN, et al. PD-1 expression by tumour-associated macrophages inhibits phagocytosis and tumour immunity. Nature. (2017) 545:495–9. doi: 10.1038/nature22396
126. Russo GL, Moro M, Sommariva M, Cancila V, Boeri M, Centonze G, et al. Antibody–Fc/FcR interaction on macrophages as a mechanism for hyperprogressive disease in non–small cell lung cancer subsequent to PD-1/PD-L1 blockade. Clin Cancer Res. (2019) 25:989–99. doi: 10.1158/1078-0432.CCR-18-1390
127. Pesce S, Greppi M, Grossi F, Del Zotto G, Moretta L, Sivori S, et al. PD/1-PD-Ls checkpoint: insight on the potential role of NK cells. Front Immunol. (2019) 10:1242. doi: 10.3389/fimmu.2019.01242
128. Pesce S, Greppi M, Tabellini G, Rampinelli F, Parolini S, Olive D, et al. Identification of a subset of human natural killer cells expressing high levels of programmed death 1: a phenotypic and functional characterization. J Allergy Clin Immunol. (2017) 139:335–46.e3. doi: 10.1016/j.jaci.2016.04.025
129. Concha-Benavente F, Kansy B, Moskovitz J, Moy J, Chandran U, Ferris RL. PD-L1 Mediates dysfunction in activated PD-1+ NK cells in head and neck cancer patients. Cancer Immunol Res. (2018) 6:1548–60. doi: 10.1158/2326-6066.CIR-18-0062
130. Chen P-L, Roh W, Reuben A, Cooper ZA, Spencer CN, Prieto PA, et al. Analysis of immune signatures in longitudinal tumor samples yields insight into biomarkers of response and mechanisms of resistance to immune checkpoint blockade. Cancer Discov. (2016) 6:827–37. doi: 10.1158/2159-8290.CD-15-1545
131. Amaria RN, Reddy SM, Tawbi HA, Davies MA, Ross MI, Glitza IC, et al. Neoadjuvant immune checkpoint blockade in high-risk resectable melanoma. Nat Med. (2018) 24:1649–54. doi: 10.1038/s41591-018-0197-1
132. Giacomo AMD, Covre A, Finotello F, Rieder D, Danielli R, Sigalotti L, et al. Guadecitabine plus ipilimumab in unresectable melanoma: the NIBIT-M4 clinical trial. Clin Cancer Res. (2019) 25:7351–62. doi: 10.1158/1078-0432.CCR-19-1335
133. Chen L, Diao L, Yang Y, Yi X, Rodriguez BL, Li Y, et al. CD38-mediated immunosuppression as a mechanism of tumor cell escape from PD-1/PD-L1 blockade. Cancer Discov. (2018) 8:1156–175. doi: 10.1158/2159-8290.CD-17-1033
134. Yost KE, Satpathy AT, Wells DK, Qi Y, Wang C, Kageyama R, et al. Clonal replacement of tumor-specific T cells following PD-1 blockade. Nat Med. (2019) 25:1251–9. doi: 10.1038/s41591-019-0522-3
135. Piali L, Fichtel A, Terpe HJ, Imhof BA, Gisler RH. Endothelial vascular cell adhesion molecule 1 expression is suppressed by melanoma and carcinoma. J Exp Med. (1995) 181:811–16. doi: 10.1084/jem.181.2.811
136. Schmittnaegel M, Rigamonti N, Kadioglu E, Cassará A, Wyser Rmili C, Kiialainen A, et al. Dual angiopoietin-2 and VEGFA inhibition elicits antitumor immunity that is enhanced by PD-1 checkpoint blockade. Sci Transl Med. (2017) 9: eaak9670. doi: 10.1126/scitranslmed.aak9670
137. Martinet L, Garrido I, Filleron T, Le Guellec S, Bellard E, Fournie J-J, et al. Human solid tumors contain high endothelial venules: association with T- and B-lymphocyte infiltration and favorable prognosis in breast cancer. Cancer Res. (2011) 71:5678–87. doi: 10.1158/0008-5472.CAN-11-0431
138. Chen L, Qiu X, Wang X, He J. FAP positive fibroblasts induce immune checkpoint blockade resistance in colorectal cancer via promoting immunosuppression. Biochem Biophys Res Commun. (2017) 487:8–14. doi: 10.1016/j.bbrc.2017.03.039
139. Qin S, Xu L, Yi M, Yu S, Wu K, Luo S. Novel immune checkpoint targets: moving beyond PD-1 and CTLA-4. Mol Cancer. (2019) 18:155. doi: 10.1186/s12943-019-1091-2
140. Petitprez F, Sun C-M, Lacroix L, Sautès-Fridman C, de Reyniès A, Fridman WH. Quantitative analyses of the tumor microenvironment composition and orientation in the era of precision medicine. Front Oncol. (2018) 8:390. doi: 10.3389/fonc.2018.00390
141. Sturm G, Finotello F, Petitprez F, Zhang JD, Baumbach J, Fridman WH, et al. Comprehensive evaluation of transcriptome-based cell-type quantification methods for immuno-oncology. Bioinformatics. (2019) 35:i436–45. doi: 10.1093/bioinformatics/btz363
142. Ståhl PL, Salmén F, Vickovic S, Lundmark A, Navarro JF, Magnusson J, et al. Visualization and analysis of gene expression in tissue sections by spatial transcriptomics. Science. (2016) 353:78–82. doi: 10.1126/science.aaf2403
143. Rodriques SG, Stickels RR, Goeva A, Martin CA, Murray E, Vanderburg CR, et al. Slide-seq: a scalable technology for measuring genome-wide expression at high spatial resolution. Science. (2019) 363:1463–7. doi: 10.1126/science.aaw1219
Keywords: tumor microenvironment, immunotherapy, immune checkpoint blockade, response, prediction
Citation: Petitprez F, Meylan M, de Reyniès A, Sautès-Fridman C and Fridman WH (2020) The Tumor Microenvironment in the Response to Immune Checkpoint Blockade Therapies. Front. Immunol. 11:784. doi: 10.3389/fimmu.2020.00784
Received: 10 February 2020; Accepted: 07 April 2020;
Published: 07 May 2020.
Edited by:
Salem Chouaib, Institut Gustave Roussy, FranceReviewed by:
Carlos Alfaro, Independent Researcher, Pamplona, SpainKaty Rezvani, University of Texas MD Anderson Cancer Center, United States
Copyright © 2020 Petitprez, Meylan, de Reyniès, Sautès-Fridman and Fridman. This is an open-access article distributed under the terms of the Creative Commons Attribution License (CC BY). The use, distribution or reproduction in other forums is permitted, provided the original author(s) and the copyright owner(s) are credited and that the original publication in this journal is cited, in accordance with accepted academic practice. No use, distribution or reproduction is permitted which does not comply with these terms.
*Correspondence: Florent Petitprez, florent.petitprez@ligue-cancer.net; Wolf H. Fridman, herve.fridman@crc.jussieu.fr