Coupling Imaging and Omics in Plankton Surveys: State-of-the-Art, Challenges, and Future Directions
- 1Institut de Biologie de l’Ecole Normale Supérieure (IBENS), Ecole Normale Supérieure, Centre National de la Recherche Scientifique (CNRS), Institut National de la Santé Et de la Recherche Médicale (INSERM) Université Paris Sciences et Lettres (PSL), Paris, France
- 2Centre National de la Recherche Scientifique (CNRS) Research Federation for the study of Global Ocean Systems Ecology and Evolution, FR2022/Tara Oceans Global Ocean Systems Ecology and Evolution (GOSEE), Paris, France
- 3Sorbonne Universités, Centre National de la Recherche Scientifique (CNRS), Laboratoire d’Océanographie de Villefranche (LOV), Villefranche-sur-Mer, France, Institut Universitaire de France (IUF), Paris, France
- 4Department of Ecology, Environment and Plant Sciences, Stockholm University, Stockholm, Sweden
A major challenge in characterizing plankton communities is the collection, identification and quantification of samples in a time-efficient way. The classical manual microscopy counts are gradually being replaced by high throughput imaging and nucleic acid sequencing. DNA sequencing allows deep taxonomic resolution (including cryptic species) as well as high detection power (detecting rare species), while RNA provides insights on function and potential activity. However, these methods are affected by database limitations, PCR bias, and copy number variability across taxa. Recent developments in high-throughput imaging applied in situ or on collected samples (high-throughput microscopy, Underwater Vision Profiler, FlowCam, ZooScan, etc) has enabled a rapid enumeration of morphologically-distinguished plankton populations, estimates of biovolume/biomass, and provides additional valuable phenotypic information. Although machine learning classifiers generate encouraging results to classify marine plankton images in a time efficient way, there is still a need for large training datasets of manually annotated images. Here we provide workflow examples that couple nucleic acid sequencing with high-throughput imaging for a more complete and robust analysis of microbial communities. We also describe the publicly available and collaborative web application EcoTaxa, which offers tools for the rapid validation of plankton by specialists with the help of automatic recognition algorithms. Finally, we describe how the field is moving with citizen science programs, unmanned autonomous platforms with in situ sensors, and sequencing and digitalization of historical plankton samples.
Importance of Plankton Monitoring
Plankton are an extremely diverse group of organisms comprised of highly dynamic and interacting populations of viruses, bacteria, archaea, single-celled eukaryotes (e.g., protists) and animals that drift with the currents and span several orders of magnitude in size (from <0.1 µm to a few mm) (de Vargas et al., 2015). Photosynthetic plankton (phytoplankton) carry out almost half of the net primary production on our planet (Field et al., 1998) and fuel the biological carbon pump (i.e., the export of photosynthetically fixed carbon to the deep ocean) (Falkowski, 2012; Guidi et al., 2015). Plankton also form the base of food webs that sustain the complexity of life in the ocean and other aquatic environments (Azam et al., 1983). In marine and freshwater ecosystems, plankton are influenced by multiple anthropogenic impacts (i.e., plastics, pollutants, nutrient loading, invasive species) and global change (e.g., deoxygenation, warming, acidification, freshening, ocean circulation changes) (Williamson et al., 2009). Plankton can also function as sensitive indicators of change due to their relatively short life cycles (Hays et al., 2005) and can help determine the health status of an ecosystem (DeLong and Karl, 2005). Therefore, the biomass and diversity of plankton were recently identified as Essential Ocean, Biodiversity, and Climate Variables by three independent vital observational networks: the Global Ocean Observing System (GOOS), the Group on Earth Observations (GEO-BON), and the Global Climate Observing System (GCOS) (Miloslavich et al., 2018; Muller-Karger et al., 2018).
Evolution of Plankton Observation Workflows
Community assessment of environmental planktonic samples has evolved over the years (Pierella Karlusich et al., 2020a). For example, classically the field relied on plankton that could be collected by nets, easily preserved (e.g., using lugol's or paraformaldehyde), settled in specialized chambers, identified and enumerated by experienced taxonomists (Utermöhl, 1958). Later, chemical analyses by high-performance liquid chromatography (HPLC) allowed phytoplankton to be distinguished by their primary and accessory pigments (Jeffrey, 1974; Mackey et al., 1996; Jeffrey et al., 1999). An important adopted method from the biomedical sciences was flow cytometry that can sort populations by size and auto-fluorescence and led to important discoveries (Chisholm et al., 1988). Many of these methods continue to be important in regional biomonitoring programs, however most are time-consuming, require expert taxonomic knowledge and training, and are gradually being replaced by higher throughput methods, including automated plankton imaging instruments (e.g., Underwater Vision Profiler, Imaging FlowCytobot, FlowCam, ZooScan, etc; Figure 1) as well as genetic surveys (i.e., ‘omic’ approaches) (Pierella Karlusich et al., 2020a).
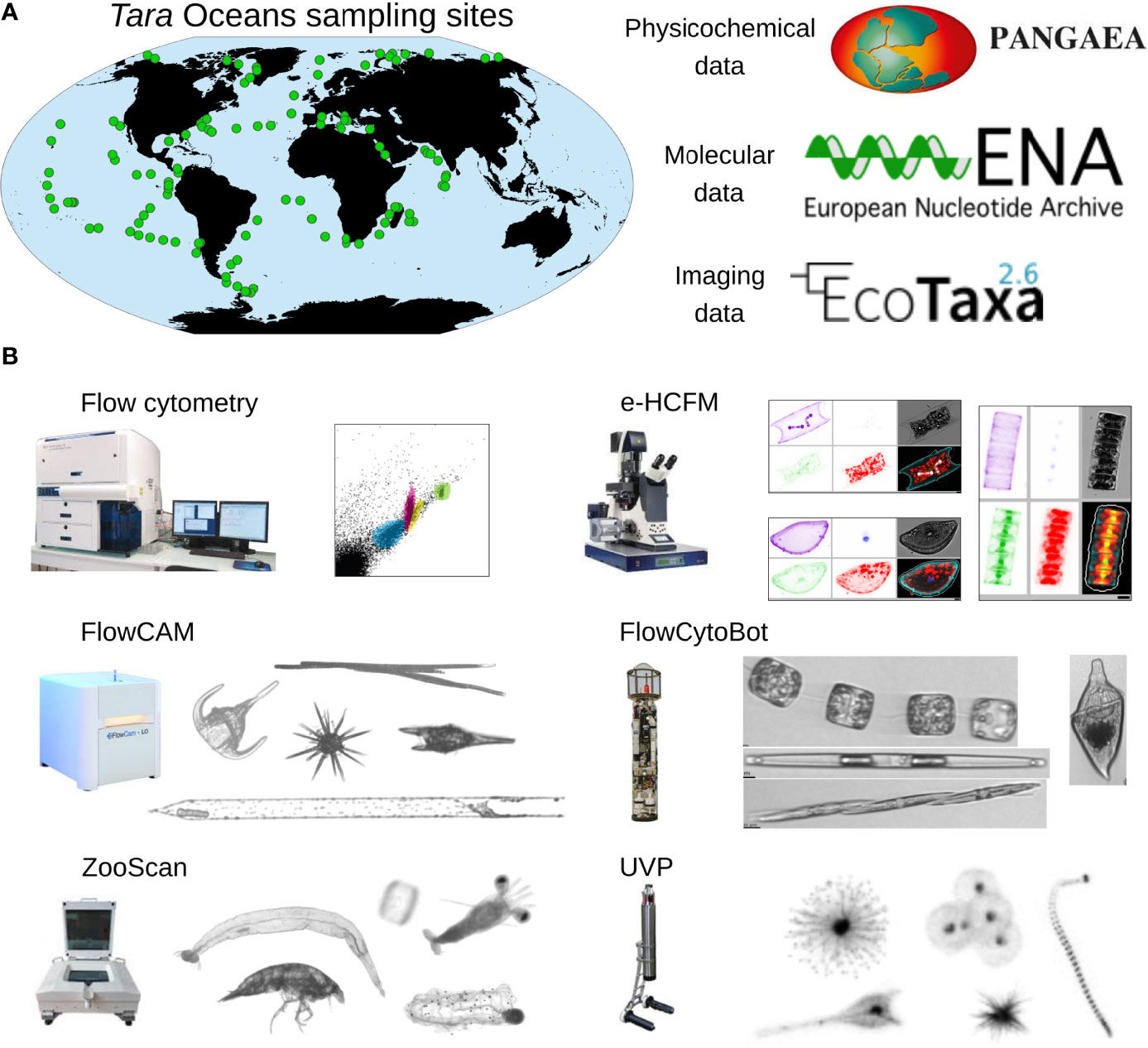
Figure 1 Datasets generated by Tara Oceans expeditions and imaging devices. (A) Tara Oceans expeditions (2009-2013) collected >35000 plankton samples from 210 sampling sites of the upper ocean, which were used for generating physicochemical contextual data as well as >60 terabases of DNA and RNA sequences and ~7 million images (Pierella Karlusich et al., 2020b; Sunagawa et al., 2020). The set of physicochemical and oceanographic parameters can be retrieved from Pangaea repository (https://www.pangaea.de/), the molecular data from the European Nucleotide Archive (ENA; https://www.ebi.ac.uk/ena/), and the imaging data from Ecotaxa (https://ecotaxa.obs-vlfr.fr/). (B) High throughput plankton imaging instruments used in the Tara Oceans expeditions. The flow cytometer is used to determine counts of picocyanobacteria, heterotrophic bacteria and eukaryotic picophytoplankton (Gasol and Morán, 2015). The environmental High-Content Fluorescence Microscopy (e-HCFM) method is based on an automated Leica SP8 TCS confocal laser scanning microscope that enables 3D multicolor imaging of cells (Colin et al., 2017). The instrument was developed at the European Molecular Biology Laboratory for the analysis of objects in the size range 2–500 µm, and is best suited to study preserved samples. The Imaging FlowCytobot (IFCB; McLane Research Laboratories, Inc., USA; Olson and Sosik, 2007) is designed for the analysis of objects in the size range 10–150 µm, and is appropriate to study live small-size protists such as flagellates, ciliates and diatoms. The FlowCam (Fluid Imaging Inc.; Sieracki et al., 1998) uses a similar imaging principle as the Imaging FlowCytobot. FlowCam works well with organisms between 20 and 300 μm, with FlowCam-nano and FlowCam-macro for smaller and larger organisms, respectively. The ZooScan (Hydroptics, France; Gorsky et al., 2010) is a benchtop scanner instrument useful for the analysis of objects in the size-range 300-5000 µm, and is best suited to study preserved samples of organisms such as large hard-shelled protists (e.g., Rhizaria) and metazoa. The Underwater Vision Profiler (UVP5, Hydroptics, France; Picheral et al., 2010) is designed to detect and count objects of >100 µm in size and to identify those of >600 µm in size. It is appropriate to study fragile aggregates such as marine snow particles and organisms that tend to break when sampled with nets, such as gelatinous metazoans.
High throughput imaging and omic methods have been used in large spatial marine surveys (Figure 1A) and at ocean time-series sites (Olson and Sosik, 2007; Sosik and Olson, 2007). However, the longest running large-scale marine plankton survey currently corresponds to a classical approach deployed since the 1930s: the Continuous Plankton Recorder (CPR) (Warner and Hays, 1994; Batten et al, 2019). This is possible because the CPR is sufficiently robust for deployments from commercial ships, unaccompanied by researchers, and makes sample collection cost-efficient over large ocean tracts (Warner and Hays, 1994; Batten et al, 2019).
Identifying and Enumerating Plankton by Nucleic Acid Surveys
Plankton are typically collected by filtering seawater. In molecular based applications it is also common to use a combination of filter membranes with different pore sizes (serial size-fractionation) to separate the organisms by cell diameter and aggregation forms (e.g., 0.2-3 µm, 0.8-5 µm, 5-20 µm, 20-180 µm, 180-2000 µm) (Pesant et al., 2015). Given the inverse logarithmic relationship between plankton size and abundance (Belgrano et al., 2002; Pesant et al., 2015), protocols usually consist of filtering higher water volumes for the larger size fractions (Pesant et al., 2015). Genetic surveys that characterize the structure and composition of microbial communities are performed by the extraction of nucleic acids from the plankton samples, then a PCR amplification step and sequencing of a marker gene (gene metabarcoding) (Burki et al., 2021). The resulting DNA (or RNA) sequences are taxonomically classified by comparisons with reference sequence databases (Pawlowski et al., 2012; Guillou et al., 2013; Quast et al., 2013). Therefore, the incompleteness of the reference database can impact the results.
Many studies have focused on taxonomically informative fragments of the hypervariable regions of the 16S (prokaryote and chloroplast) and/or 18S (eukaryotic nuclear) rRNA genes. These molecular markers are by far the most represented in reference databases (Pawlowski et al., 2012; Guillou et al., 2013; Quast et al., 2013). To a minor extent, others have used the internal transcribed spacer (ITS) region of the ribosomal operon, for example for fungi (Schoch et al., 2012) and oomycetes (Robideau et al., 2011). Still others have relied on functional gene markers such as the nitrogenase subunit gene (nifH) for nitrogen-fixers (Heller et al., 2014), the ribulose-1,5 bisphosphate carboxylase–oxygenase large subunit (rbcL) gene for photosynthetic organisms (Bailet et al., 2019; Armbrecht et al., 2021), and the mitochondrial cytochrome oxidase c subunit I (COI) gene for a range of microbial groups (Kucera and Saunders, 2008; Gall and Saunders, 2010; Stern et al., 2010).
In gene metabarcoding, the fraction of the obtained sequencing reads corresponding to a given taxon is then used as a proxy for its relative abundance. However, this approach generates biases due to several error sources: variable DNA extraction and sequencing efficiency (which also can affect other molecular methods), innate PCR biases, and copy number variability of the marker gene among species. PCR amplification bias due to template abundances and mismatches of the primers on the target sites of certain taxa can both generate differences between the observed and the genuine relative read abundances as large as 10-fold (Polz & Cavanaugh, 1998; Lefever et al., 2013; Parada et al., 2016; Bradley et al., 2016; Wear et al., 2018). In addition, the 18S rRNA gene copy number can differ by >5 orders of magnitude in protists, and thus overestimates certain taxa (Zhu et al., 2005; Godhe et al., 2008; de Vargas et al., 2015; Mäki et al., 2017).
Some of the mentioned biases can be avoided or lessened. For example, shotgun sequencing is a PCR-free alternative and unlike metabarcoding here and elsewhere, generates sequences for all the DNA present, thus it can be used to detect numerous marker genes in an environmental sample (also called here metagenomes) (Liu et al., 2007; Logares et al., 2014; Obiol et al., 2020; Pierella Karlusich et al., 2022). Moreover, the use of alternative marker genes with low-copy variability among taxa can also improve on the innate biases in quantification (Sunagawa et al., 2013; Pierella Karlusich et al., 2022).
In addition to taxonomic analyses, metagenomics can be used to reconstruct partial to full genomes (metagenome-assembled genomes or MAGs) for non-model and uncultivated organisms (Delmont et al., 2018; Tully et al., 2018; Delmont et al., 2021). Complementing information can be obtained by metatranscriptomics, which provide gene expression values as an indicator of functional activity, e.g. nifH for N2 fixation; rbcL for photosynthesis (Carradec et al., 2018).
Current popular high-throughput sequencing technologies (e.g., 454-pyrosequencing, Ion Torrent, Illumina) were limited in the lengths of the sequence fragments (maximum length of ~500 bp), and thus limited the phylogenetic resolution. A new generation of environmental sequencing has recently emerged that utilizes long-read technologies, e.g., Pacific Bioscience (PacBio) and Oxford Nanopore Technologies (ONT). Both can produce high-quality long-read metabarcoding and metagenomic datasets of between 1,500 and 5,000 bp (Heeger et al., 2018; Tedersoo et al., 2018; Orr et al., 2018; Jamy et al., 2020). These long reads can be used in phylogeny-aware annotations to account for uncertainty and missing data in the reference databases (Jamy et al., 2020).
Despite the different mentioned caveats, omic surveys have become relatively affordable and automated (Ji et al., 2013), allowing analyses of datasets of unprecedented size and taxonomic resolution. Such datasets have enabled the identification of cryptic species (not easily detected by morphology) (Šlapeta et al., 2006), and allowed for a simultaneous survey of all domains of life.
High-Throughput Imaging of Environmental Plankton Populations
Investigating plankton at large spatial and/or temporal scales is difficult. Sampling is often labor intensive and common collection methods, such as nets, sediment traps, bottles, and electric powered pumps, are not adapted for all sizes of plankton, nor can fragile organisms be sampled carefully (Remsen et al., 2004). In addition, collections largely represent ‘snapshots’ and require manual and time-consuming sorting of material, which makes the results difficult to scale up to pan-oceanic observations in situ (a classical approach which is an exception to this limitation is CPR). These issues also limit the number of samples that can be used to isolate DNA/RNA for omics and/or to manually count cells by microscopy. However, while omic analyses are relatively automated, manual microscopy is low throughput because each sample requires long processing time by specialized personnel (Ji et al., 2013). This reliance on human experts also implies some classification subjectivity (Culverhouse et al., 2003) but more importantly can also lead to low reproducibility.
These limitations have stimulated the development of numerous alternative and automated plankton monitoring tools and instruments for marine and freshwater ecosystems (Wiebe and Benfield, 2003; Lombard et al., 2019; Spanbauer et al., 2020; Orenstein et al., 2021; Irisson et al., 2022). These techniques generate data comparable to those obtained by manual light microscopy, but in a high-throughput way. Still, some differences can be detected due to the differences in manual vs automatic classification, sample preservation vs in situ observations, and between sampled seawater volumes (Olson and Sosik, 2007; Jakobsen & Carstensen, 2011; Álvarez et al., 2014; Schmid et al., 2016; Haraguchi et al., 2017; Detmer et al., 2019; Hrycik et al., 2019; Kraft et al., 2021).
Current plankton imaging instruments that are commercially available are shown in Figure 1B and a more complete list is available in Table 1 of Lombard et al., 2019. Additionally, a high-throughput microscopy denoted as environmental high content fluorescence microscopy (e-HCFM), has recently been described for generating 3D multi-channel images of nanoplankton (5-20 µm) and microplankton (20-180 µm) from preserved samples (Colin et al., 2017) (Figure 1B). These instruments deliver highly diverse and continuous image datasets from planktonic populations, and some can be deployed for in situ monitoring (e.g., for harmful algal blooms; Moore et al., 2017; Moore et al., 2019; Henrichs et al., 2021) and/or for in situ quantification of fragile organisms that are difficult to collect by nets (Hull et al., 2011; Biard et al., 2016; Gaskell et al., 2019). It is expected that the volume and complexity of marine data will increase by orders of magnitude in the coming years and the annotation rate of human experts is currently lagging behind the data generated. Therefore, advanced automated image recognition techniques, including the extraction of image features followed by machine learning classifiers (e.g. support vector machine, SVM; random forest, RF; artificial neural networks, ANNs) and the combination of the two steps by deep learning approaches (convolutional neural networks, CNN; Krizhevsky et al., 2012; Russakovsky et al., 2015) have been developed (Irisson et al., 2022 and references therein).
Machine learning models are only as good as the data they are trained on. Therefore, database limitations affect the annotation of high-throughput images, in the same way as it occurs in sequence analyses. A sure way to improve classifiers is through large, diverse, and high-quality training data sets. Most plankton data sets are severely unbalanced (e.g., one of a few dominant taxa). Hence to move forward, there is a need for large, publicly available data sets of identified images that contain realistic proportions of diverse planktonic taxa. Fortunately, there are a number of ongoing programs aiming to expand the current labeled set of plankton classes and create a larger publicly available plankton database. These include the Tara Oceans project (Figure 1), and numerous localized monitoring programs in freshwater (Kyathanahally et al., 2021) and marine environments (Luo et al., 2018; Plonus et al., 2021; PlanktonSet, Cowen et al., 2015; ZooScanNet, Elineau et al., 2018; WHOI-Plankton, Sosik et al., 2021; SYKE-plankton_IFCB_2022, https://b2share.eudat.eu/records/abf913e5a6ad47e6baa273ae0ed6617a).
Some of the mentioned machine learning approaches are integrated into specialized plankton software tools such as ZooProcess (Gorsky et al., 2010) combined with Plankton Identifier (Gasparini and Antajan, 2007) or ZooImage (Bell and Hopcroft, 2008; Grosjean et al., 2018), and Visual SpreadSheet (FlowCAM Manual, 2012). As an alternative to these locally installed applications that are specific to one or few instruments, is the recent development of the web application called EcoTaxa (Picheral et al., 2017). EcoTaxa brings the possibility to use machine learning approaches (including deep learning) with no technical knowledge or set up, enables users to work collaboratively to validate or correct the proposed annotations, and to share the resulting datasets to comply with the standards of Findability, Accessibility, Interoperability, and Reusability (FAIR principles; Wilkinson et al., 2016).
Visualizing and Classifying Plankton Using EcoTaxa
EcoTaxa was initially developed in France for exploiting the quantitative imaging data collected during the Tara Oceans expeditions (Figure 1A) (Pierella Karlusich et al., 2020b; Sunagawa et al., 2020). It has since expanded and now covers many other sampling campaigns. EcoTaxa functions as a centralized repository where individual images from environmental populations of (mainly) marine plankton generated by different technologies can be uploaded (>190 million images from >10 different instruments as of January 2022). Operators can train machine classifiers on the fly, using morphological features produced by the pre-processing software (such as the aforementioned ZooProcess) and/or features generated by deep learning networks included in EcoTaxa. The user selects and classifies a few images and the supervised machine learning algorithm predicts annotations for the remaining ones (a process akin to the common use of BLAST (Altschul et al., 1997) or HMMER (http://hmmer.org/) algorithms for searching nucleotide or protein sequences in omics databases). Then the graphical interface strives to efficiently validate or correct those predictions, resulting in a throughput of ~2000 to ~10,000 annotations per active hour depending on the dataset (Irisson et al., 2021). Visitors have free access to some datasets that have been identified by expert taxonomists and published with an open license. Similar to nucleic acid databases, users can navigate the image database along a taxonomic tree, or filter the images according to sampling criteria (location, season, time of day) (Figure 2A). Once access is granted by the dataset manager, resulting datasets can be easily downloaded and provides users with ecological data such as concentration and biovolume estimates at a given time and point (latitude, longitude, depth) for each taxon. These types of analyses are useful in research, training, and teaching (e.g. plankton lab exercises).
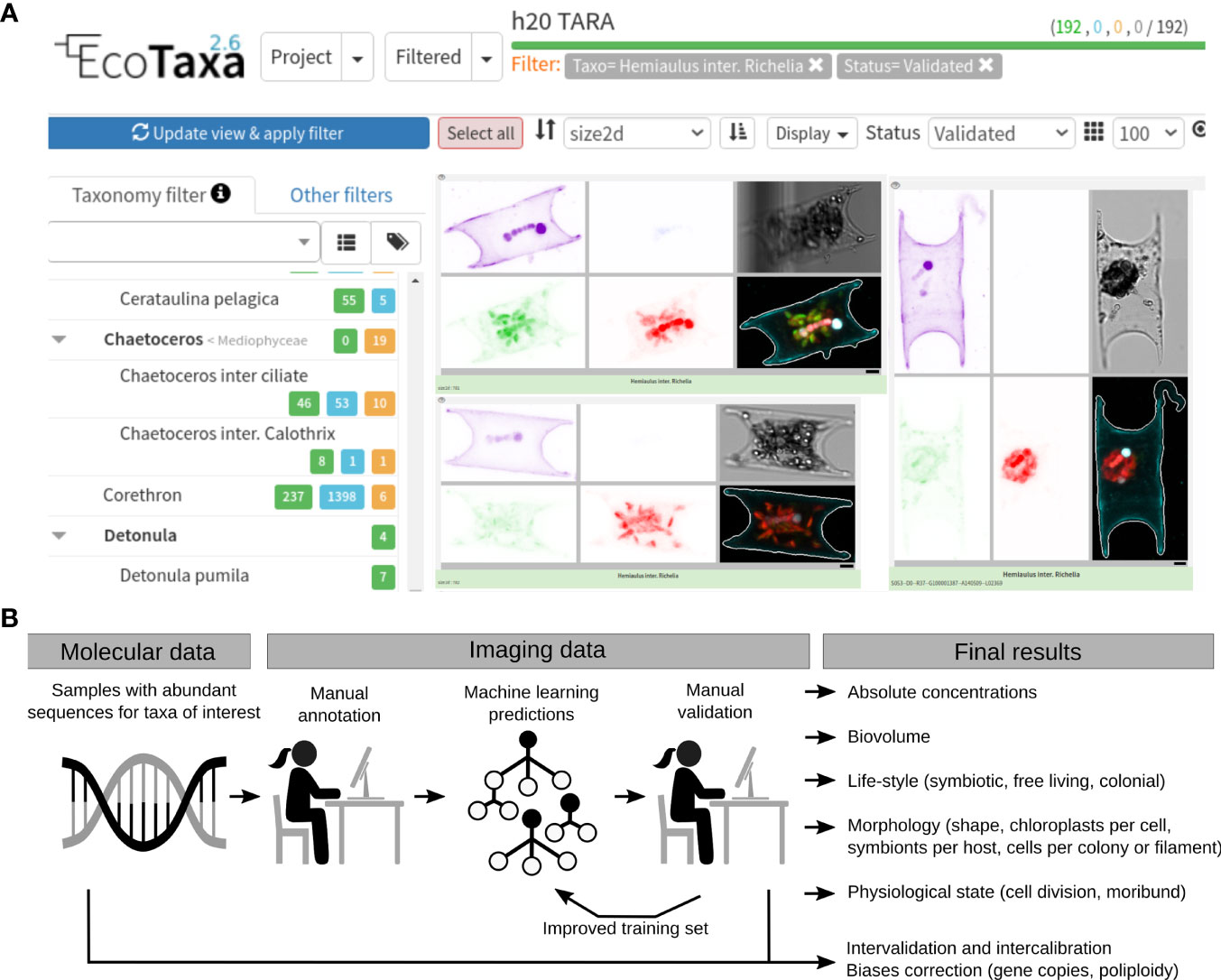
Figure 2 Integration of molecular and imaging datasets with the help of EcoTaxa. (A) User interface of EcoTaxa (https://ecotaxa.obs-vlfr.fr/). The user can navigate the database along a taxonomic tree (left panel of the website) or filter the images according to sampling criteria (location, time, depth) or morphological features (size, chlorophyll a content, etc). The concentration and biovolume for a taxon at a given geographical location and time point can be retrieved. For operators, easy-to-use tools are provided for the rapid identification of large numbers of images by a combination of machine learning and human validation. (B) Workflow for the integration of molecular and imaging data. This example is based on our recent analyses of free-living and symbiotic planktonic nitrogen-fixers in the Tara Oceans project (Pierella Karlusich et al., 2021). The molecular data was first used for helping to target the manual annotation workload of the imaging data. The strategy started with the mining of molecular data (metagenomes) to select a few samples where sequences from nitrogen-fixers were abundant, and therefore it was feasible to manually search the images from those samples through EcoTaxa. After obtaining a few manually annotated images, predictions were run for the whole dataset (all samples) and curated by visual inspection. These results were then used as a new training set for running new predictions. The imaging and molecular results were then compared to validate each other and to quantify biases (e.g., the variations in gene or genome copies per cell). Imaging-based information that cannot be determined by molecular data were also generated: absolute quantifications, biovolume, symbiont number per host cell, asymbiotic partners, chloroplast content, cell number per colony/filament.
Each image is associated with the geotemporal and processing metadata. Additionally, images generated by Tara Oceans are linked to a complete set of physicochemical and oceanographic parameters (dissolved nutrient concentrations, temperature, salinity, etc) archived in the Pangaea repository (https://www.pangaea.de/), and molecular data archived in the European Nucleotide Archive (ENA; https://www.ebi.ac.uk/ena/) (Figure 1A). In addition to contextual variables for each sample, EcoTaxa stores a collection of numeric features extracted from each image (roundness, perimeter, area, aspect ratio, texture, intensity, etc). Beyond their use for automated classification explained above, these features are increasingly valuable as data in their own right, i.e., for trait-based approaches (Vilgrain et al., 2021).
So far, examples of studies based on Ecotaxa have focused on specific taxonomic groups: the supergroup Rhizaria (Biard et al., 2016), the polychaete Poeobius sp. (Christiansen et al., 2018), the filamentous cyanobacterium Trichodesmium (Pierella Karlusich et al., 2021), and planktonic symbioses (Vincent et al., 2018; Pierella Karlusich et al., 2021). As an example, we briefly describe the process of image classification for such specific taxon cases. In each of these targeted studies, images of a taxon of interest were classified into accepted taxonomic units along a single tree, provided by EcoTaxa. In cases where the dorsal and lateral views or the different development stages of the same taxon look very different, the automated classifier is guided by splitting the images into different classes, defined as children of the target taxon. In the focused studies on specific taxa, the detailed sorting of some other parts of the dataset is not necessary, and therefore several image classes can be grouped under a single “catch all” term (e.g., ‘detritus’ that contains fecal pellets, aggregates and fibers). Yet, it is important that these “catch all” classes be thoroughly checked for objects belonging to the taxa of interest since their polymorphic nature tends to trip automated classifiers.
It is worth mentioning that Ecotaxa (as well as most studies and tools) have used supervised classifiers, which learn to classify new images based on a set of images already classified by human experts. While these supervised machine learning approaches are generally very fast and the most accurate for a predefined class of interest, they are limited to the set of classes present in the training data set of taxa/morphologies (González et al., 2017). Instead, unsupervised learning has been implemented which functions without predefined class labels for the images under study because the goal is to group similar images into clusters. Importantly, this enables novelty detection and facilitates the data-driven creation of possibly meaningful subcategories within what could have been considered as a single class in a supervised classification approach. An example tool for unsupervised classification is MorphoCluster (Schröder et al., 2020; https://github.com/morphocluster), which uses image features computed by a CNN for clustering. The primary use cases for unsupervised learning is the rapid annotation of huge volumes of images for further data analysis but also the initialization of a training set.
In Situ Sensors for Imaging and Omics in Unmanned, Autonomous, and Remote Sensing Platforms
Recently the field is advancing in the direction of deploying in situ sensors on longer-term platforms monitored by satellites (or ship cruises) (Whitt et al., 2020). Examples of imaging instruments include moored (Seegers et al., 2015; Yamahara et al., 2015; Ryan et al., 2017; Bowers et al., 2018), ship-based (Schofield et al., 2006), remotely operated underwater vehicles (ROVs) and autonomous underwater vehicles (AUVs) (Hails et al., 2009; Pargett et al., 2015; Beckler et al., 2019). These applications significantly advance the spatiotemporal coverage compared to earlier efforts with ship-based sampling.
A notable example is the nearly 16 year continuous time series of Imaging FlowCytobot (IFCB) at 4m depth at the Martha’s Vineyard Coastal Observatory (MVCO) (Olson and Sosik, 2007; Sosik and Olson, 2007). The instrument is able to operate unattended for months, using power and communications from a shore lab for real-time operation, monitoring and data download. Another example is the next generation of Underwater Vision Profiler (UVP6) currently deployed on Argo buoys, gliders and moorings (Picheral et al., 2021).
Molecular data can also be generated through in situ sensors on longer-term platforms. The Environmental Sample Processor (ESP) is a robotic device that can be programmed to automate water sample filtration and preservation of plankton material, or homogenize it for immediate in situ analyses (Scholin et al., 2017). It has has been deployed locally moored (Preston et al., 2011; Yamahara et al., 2015), free-drifting (Ottesen et al., 2013; Robidart et al., 2014), as well as in deep-water configurations (Ussler et al., 2013), and with AUVs (Pargett et al., 2015; Scholin et al., 2017). Published capabilities include in situ detection of specific planktonic species via nucleic acid probe hybridization arrays or quantitative PCR (Preston et al., 2011; Varaljay et al., 2015), and collection and fixation of samples for on-shore genomic and transcriptomic analyses (Ottesen et al., 2011; Aylward et al., 2015).
To date, high throughput omics technology has been miniaturized into Oxford Nanopore MinION devices (Jain et al., 2016; Lu et al., 2016). These sequencers are small and portable and capable of real-time analyses, and have been successfully applied in marine (Shin et al., 2018; Warwick-Dugdale et al., 2019) and freshwater environments (Urban et al., 2020). Thus, in the future, it should be feasible to have these miniaturized sequencers for monitoring plankton by their in situ DNA/RNA, simultaneously with in situ imaging. However, improved strategies for data retrieval from autonomous platforms are still required; for example Argo floats cannot send millions of images or sequences via satellite communication. Currently only data processed in the sensor and summarized (e.g. number of particles detected, size spectrum) can be obtained, unless the sensors are physically retrieved from the ocean, which is rare.
Citizen Science: Engaging the Public for Plankton Enumeration
In addition to the use of autonomous samplers for improving the spatiotemporal coverage of plankton populations, the field is developing a new generation of affordable tools and protocols that can be deployed in the thousands of citizen sailing boats, professional sailing yachts, cargo ships and fishing vessels which are navigating the world ocean every day.
An example is Plankton Planet, which is a recently established and ongoing citizen science project initiated by researchers from the CNRS (The National Centre for Scientific Research, France), together with the Tara Oceans consortium (de Vargas et al., 2020; Pollina et al., 2020; https://www.planktoscope.org/). The project aims to mobilize citizen sailors to collect samples and images of plankton from all over the world. Participants are provided with free training and encouraged to build their own Planktoscope (Pollina et al., 2020), a frugal high-throughput microscope platform for acquiring images of microplankton (20–200 µm). The participants take images which can then be uploaded to EcoTaxa for classification. Participants are also equipped with a basic sampling toolkit for collecting planktonic DNA for sequencing; sea surface plankton are collected by a net and rapidly transferred onto a filter membrane using a manual pumping system, and the filter is then heated and desiccated as part of a simple protocol for DNA preservation (De Vargas et al., 2020).
In addition to collecting samples, citizen science programs can help perform, or at least initiate, the taxonomic classification of images for example (Robinson et al., 2017; Kiko et al., 2018). Platforms like Ecotaxa and other specialized tools could be complemented with more user friendly interfaces geared towards a global community of citizen scientists, like the existing Plankton Portal app as part of the Zooniverse initiative (Robinson et al., 2017) or the PlanktonID website (Kiko et al., 2018). Still, collaboration with trained taxonomists is always necessary as the field moves forward and the lack of trained taxonomists is an important challenge (Pearson et al., 2011).
Historical Plankton Sampling
Planning for future ocean conditions requires historical data to establish more informed ecological baselines. Historical samples, preserved in formaldehyde, represent a treasure trove that can be used to compare plankton communities from the modern ocean with that of former decades (or even centuries, Fox et al., 2020).
A recent study compared the samples collected in the eastern Pacific Ocean by the HMS Challenger in September 1875 with those from Tara Oceans expedition in September 2011 (Fox et al., 2020). Notably, up to 76% reduction in shell thickness of calcifying foraminifera was measured, pointing to the potential effect of decreasing pH during the 140-year period that separated both expeditions. Although these results may be compounded by multiannual processes such as El Niño—La Niña cycles, this study illustrates the value of historical samples and simple morphological measures.
More complete datasets can be obtained with historical samples generated from time-series collections. An example was the building of historical plankton datasets by digitalization of preserved samples and classification of the resulting images using machine learning classifiers and human curation by García-Comas et al., 2011 and Vandromme et al., 2011. In addition, there are recent efforts in generating molecular data from preserved samples of the CPR survey (Stern et al., 2018; Stern et al., 2022), one of the longest running marine biological surveys.
Imaging vs Molecular Data to Estimate Plankton Diversity, Abundance and Biomass
Combining image and omics-based analyses provides a unique opportunity to relate plankton diversity, abundance, and biomass. In fact, the global ocean trends in the Shannon index, a diversity index that accounts for both richness and evenness (Calderón-Sanou et al., 2020), were highly congruent between molecular and imaging approaches (Ibarbalz et al., 2019). The trends included zooplankton imaging based on ZooScan, photosynthetic protist data obtained by confocal or light microscopy, and prokaryote data based on flow-cytometry (Ibarbalz et al., 2019). These global patterns of marine plankton diversity were used to infer the abiotic drivers and to predict the effects of severe warming of the surface ocean (Ibarbalz et al., 2019; Busseni et al., 2020). Thus, combining methods resulted in a robust estimate and prediction.
A second example of how combining imaging and omics has an advantage comes from the correlation between rRNA gene copy number and cell size. Here, it was proposed that the rRNA gene metabarcoding reads should reflect the relative proportion of biovolume for a given taxon (Lamb et al., 2019). Biovolume is often used as a proxy of biomass, which is a relevant parameter for energy and matter fluxes (e.g., food webs, biogeochemical cycles). However, there is still little consensus for using rRNA genes as a biovolume estimator due to poor correlations reported in many studies (e.g., Lamb et al., 2019; Santoferrara, 2019; Lavrinienko et al., 2021; van der Loos & Nijland, 2021; Pierella Karlusich et al., 2022). Thus, image based methods are still considered a stronger and consistent estimator for plankton biovolume.
Some have attempted to infer plankton relative cell abundances from rRNA gene metabarcoding by the establishment of correction factors. Cell abundance usually corresponds to species abundance for unicellular organisms, which is an important measure for inferring community assembly processes. However, the application of copy number corrections for the 16S rRNA gene in bacteria has limited accuracy (Kembel et al., 2012; Louca et al., 2018; Starke et al., 2020), and this correction is even more challenging for protists due to intraspecies variation in 18S rRNA gene copy number (Gong & Marchetti, 2019). Instead, marker genes with low-copy variability among taxa can drastically improve the quantifications (Pierella Karlusich et al., 2022).
Plankton identification and abundance estimates by image based approaches are most easily applied to microplankton (20-200 µm) or larger organisms that possess distinguishable morphological characters (Lombard et al., 2019). For example, many different taxonomic groups include flagellated, ciliated, or amoeboid cells that are usually <20 μm in size (Adl et al., 2019). On the contrary, the DNA-based methods remain preferable for pico- (0.2-3 µm) and nano-plankton (0.8-5 µm) because of their higher abundances and less separable phenotypes. This means that an integrated approach to quantifying all kinds of plankton still needs to be developed.
Using Molecular Data for Targeting the Manual Annotation Workload of Images
A workflow that analyzes in parallel images and sequences has been proposed to alleviate the “taxonomic bottleneck” (Riedel et al., 2013). As CNNs and feature-based methods utilize only the information contained in the image to predict the taxonomy, these annotations could be improved by the parallel DNA (and/or RNA) sequencing analyses for helping to target the manual annotation workload. A recent example includes our workflow to link a functional gene marker (nifH for N2 fixation) to plankton from the Tara Oceans e-HCFM images (Pierella Karlusich et al., 2021) (Figure 2B). The Tara Oceans project publicly archived >2 million images by e-HCFM, and presented a challenge to identify low-abundant organisms such as planktonic nitrogen-fixers. An important prerequisite here is that we already knew both the nifH gene and what the target diazotrophs looked like in microscopy images. However, in order to use the machine learning imaging prediction tools in EcoTaxa, a training set was still required for the RF model. Hence, using the molecular data (metagenomes), we selected a few stations where nifH sequences diagnostic of the target nitrogen-fixers were abundant, and then manually searched in the images from the parallel e-HCFM data (Figure 2B). Once we acquired a few manually annotated images from these selected samples, we started an iterative cycle: we ran predictions over the whole dataset (all samples) and curated the results by visual inspection, which were then used as a new example set for running new predictions.
The imaging results verified the molecular results (and vice versa), and additionally we obtained other valuable ecological information that cannot be determined by molecular data alone. For example, morphological and ecological features (biovolume, symbiont number per host cell, asymbiotic partners, chloroplast content, cell number per colony/filament) were measured. Since we also acquired absolute quantifications by the images, we were able to estimate biases in molecular approaches that are often overlooked or not clearly understood (e.g., the variations in gene or genome copies per cell; Milivojević et al., 2021). Finally, both molecular and imaging data validated global biogeographical patterns of nitrogen-fixers, and identified new high density (“hotspots”) areas in understudied and undersampled oceanic regions.
This workflow can also be used to target microorganisms that we do not know the phenotype (image) but have the phylotype (sequence). For example, we identified in numerous samples a high number of nifH sequences which were identical to symbiotic diazotrophs previously thought to be only present in freshwater environments. In the parallel e-HCFM datasets, we observed numerous images for potential symbiosis, and so we speculated that many of these nifH sequences could correspond to those images. These initial observations were recently confirmed by the isolation of the symbioses (Schvarcz et al., 2022). Therefore, this workflow advances our ability to link morphological features with genetic data, and holds promise for identifying new microbial interactions (e.g. symbiosis), and it is worth mentioning that it is directly applicable to other microbial populations.
Conclusions
High-throughput imaging and molecular technologies with adequate computational and statistical tools are complementary, where imaging is currently best suited for abundance and biomass estimates of limited groups and metabarcoding provides deeper estimates of taxonomic richness. Since the two methods are complementary, their combined use in the study of marine plankton communities provide much more reliable and accurate results. The challenge is to standardize an analytical pipeline where samples can be processed smoothly by both methodologies and their results combined to more accurately present microbial diversity, both qualitatively and quantitatively.
Indeed, recent reports illustrate how imaging combined with omics can generate valuable ecological information, such as the detection and quantification of symbioses, global biodiversity patterns, distribution patterns for biogeochemically relevant microbes and the estimation of standing stock plankton biomass. These are invaluable datasets necessary to constrain and improve ecosystem and biogeochemical models, and forecast changes in marine ecosystems in light of climate change. Moreover they also drive curiosity and generate hypotheses.
Automation techniques will become increasingly important given that most imaging modes are collecting quantities of data in real-time that are unfeasible to analyze and interpret manually. Future ocean observatories will include imaging and omics sensors deployed onto stationary and mobile platforms, ideally semi-autonomous and incorporated into an array of interoperable, web-enabled sensors for synoptic observations of the physical and chemical environment. In addition, affordable tools that can be deployed to thousands of citizen sailing boats will become more common. The field could not be more well poised for imaging and sequencing our most valuable aquatic assets: the plankton.
Data Availability Statement
The original contributions presented in the study are included in the article. Further inquiries can be directed to the corresponding authors.
Author Contributions
JJPK, CB, and RF designed the project. JJPK, FL, J-OI, CB, and RF wrote the manuscript. All authors contributed to the article and approved the submitted version.
Funding
This work has been supported by the FFEM - French Facility for Global Environment, French Government ‘Investissements d’Avenir’ programs OCEANOMICS (ANR-11-BTBR-0008), FRANCE GENOMIQUE (ANR-10-INBS-09-08), MEMO LIFE (ANR-10-LABX-54), and PSL Research University (ANR-11-IDEX-0001-02). JJPK acknowledges postdoctoral funding from the Fonds Français pour l’Environnement Mondial. RF is funded by Knut and Alice Wallenberg Foundation. This article is contribution number 135 of Tara Oceans.
Conflict of Interest
The authors declare that the research was conducted in the absence of any commercial or financial relationships that could be construed as a potential conflict of interest.
Publisher’s Note
All claims expressed in this article are solely those of the authors and do not necessarily represent those of their affiliated organizations, or those of the publisher, the editors and the reviewers. Any product that may be evaluated in this article, or claim that may be made by its manufacturer, is not guaranteed or endorsed by the publisher.
Acknowledgments
We would like to thank all colleagues from the Tara Oceans consortium as well as the Tara Ocean Foundation for their inspirational vision. We are also grateful to the three reviewers for their useful comments.
References
Adl S. M., Bass D., Lane C. E., Lukeš J., Schoch C. L., Smirnov A., et al. (2019). Revisions to the Classification, Nomenclature, and Diversity of Eukaryotes. J. Eukaryotic Microbiol. 66 (1), 4–119. doi: 10.1111/jeu.12691
Altschul S. F., Madden T. L., Schaffer A. A., Zhang J., Zhang Z., Miller W., et al. (1997). Gapped BLAST and PSI-BLAST: A New Generation of Protein Database Search Programs. Nucleic Acids Res. 25, 3389–3402. doi: 10.1093/nar/25.17.3389
Álvarez E., Moyano M., López-Urrutia Á., Nogueira E., Scharek R. (2014). Routine Determination of Plankton Community Composition and Size Structure: A Comparison Between FlowCAM and Light Microscopy. J. Plankton Res. 36 (1), 170–184. doi: 10.1093/plankt/fbt069
Armbrecht L., Eisenhofer R., Utge J., Sibert E. C., Rocha F., Ward R., et al. (2021). Paleo-Diatom Composition From Santa Barbara Basin Deep-Sea Sediments: A Comparison of 18S-V9 and Diat-Rbcl Metabarcoding vs Shotgun Metagenomics. ISME Commun. 1 (1), 66. doi: 10.1038/s43705-021-00070-8
Aylward F. O., Eppley J. M., Smith J. M., Chavez F. P., Scholin C. A., et al. (2015). Microbial Community Transcriptional Networks Are Conserved in Three Domains at Ocean Basin Scales. Proc. Natl. Acad. Sci. 112 (17), 5443–5448. doi: 10.1073/pnas.1502883112
Azam F., Fenchel T., Field J. G., Gray J. S., Meyer-Reil L. A., Thingstad F.. (1983). The Ecological Role of Water-Column Microbes in the Sea. Marine Ecol. Prog. Ser. 10, 257–263. doi: 10.3354/meps010257
Bailet B., Bouchez A., Franc A., Frigerio J.-M., Keck F., Karjalainen S.-M., et al. (2019). Molecular Versus Morphological Data for Benthic Diatoms Biomonitoring in Northern Europe Freshwater and Consequences for Ecological Status. Metabarcoding Metagenomics 3, e34002. doi: 10.3897/mbmg.3.34002
Batten S. D., Abu-Alhaija R., Chiba S., Edwards M., Graham G., Jyothibabu R., et al. (2019). A Global Plankton Diversity Monitoring Program. Front. Marine Sci. 6, 321. doi: 10.3389/fmars.2019.00321
Beckler J. S., Arutunian E., Moore T., Currier B., Milbrandt E., Duncan S.. (2019). Coastal Harmful Algae Bloom Monitoring Via a Sustainable, Sail-Powered Mobile Platform. Front. Marine Sci. 6. doi: 10.3389/fmars.2019.00587
Belgrano A., Allen A. P., Enquist B. J., Gillooly J. F. (2002). Allometric Scaling of Maximum Population Density: A Common Rule for Marine Phytoplankton and Terrestrial Plants. Ecol. Lett. 5, 611–613. doi: 10.1046/j.1461-0248.2002.00364.x
Bell J. L., Hopcroft R. R. (2008). Assessment of ZooImage as a Tool for the Classification of Zooplankton. J. Plankton Res. 30 (12), 1351–1367. doi: 10.1093/plankt/fbn092
Biard T., Stemmann L., Picheral M., Mayot N., Vandromme P., Hauss H., et al. (2016). In Situ Imaging Reveals the Biomass of Giant Protists in the Global Ocean. Nature 532 (7600), 504–507. doi: 10.1038/nature17652
Bochinski E., Bacha G., Eiselein V., Walles T. J. W., Nejstgaard J. C., Sikora T., et al. (2019). “Deep Active Learning for In Situ Plankton Classification,” in Pattern Recognition and Information Forensics. Eds. Zhang Z., Suter D., Tian Y., Branzan Albu A., Sidère N., Jair Escalante H. (Switzerland: Springer: Cham), 5–15.
Bowers H. A., Ryan J. P., Hayashi K., Woods A. L., Marin R. III, Smith G. J., et al. (2018). Diversity and Toxicity of Pseudo-nitzschia Species in Monterey Bay: Perspectives From Targeted and Adaptive Sampling. Harmful Algae 78, 129–141. doi: 10.1016/j.hal.2018.08.006
Bradley I. M., Pinto A. J., Guest J. S. (2016). Design and Evaluation of Illumina MiSeq-compatible, 18s rRNA Gene-Specific Primers for Improved Characterization of Mixed Phototrophic Communities. Appl. Environ. Microbiol. 82, 5878–5891. doi: 10.1128/AEM.01630-16
Burki F., Sandin M. M., Jamy M. (2021). Diversity and Ecology of Protists Revealed by Metabarcoding. Curr. Biol. 31 (19), R1267–R1280. doi: 10.1016/j.cub.2021.07.066
Busseni G., Caputi L., Piredda R., Fremont P., Hay Mele B., Campese L., et al. (2020). Large Scale Patterns of Marine Diatom Richness: Drivers and Trends in a Changing Ocean. Global Ecol. Biogeogr. 29 (11), 1915–1928. doi: 10.1111/geb.13161
Carradec Q., Pelletier E., Da Silva C., Alberti A., Seeleuthner Y., Blanc-Mathieu R., et al. (2018). A Global Ocean Atlas of Eukaryotic Genes. Nat. Commun. 9 (1), 1–13. doi: 10.1038/s41467-017-02342-1
Calderón-Sanou I., Münkemüller T., Boyer F., Zinger L., Thuiller W.. (2020). From Environmental DNA Sequences to Ecological Conclusions: How Strong Is the Influence of Methodological Choices? J. Biogeogr. 47 (1), 193–206. doi: 10.1111/jbi.13681
Chisholm S. W., Olson R. J., Zettler E. R., Goericke R., Waterbury J. B., Welschmeyer N.A.. (1988). A Novel Free-Living Prochlorophyte Abundant in the Oceanic Euphotic Zone. Nature 334 (6180), 340–343. doi: 10.1038/334340a0
Christiansen S., Hoving H. J., Schütte F., Hauss H., Karstensen J., Körtzinger A., et al. (2018). Particulate Matter Flux Interception in Oceanic Mesoscale Eddies by the Polychaete. Poeobius sp’ Limnol. Oceanogr. 63 (5), 2093–2109. doi: 10.1002/lno.10926
Colin S., Coelho L. P., Sunagawa S., Bowler C., Karsenti E., Bork P., et al. (2017). Quantitative 3D-Imaging for Cell Biology and Ecology of Environmental Microbial Eukaryotes. eLife 6, e26066. doi: 10.7554/eLife.26066
Cowe R. K., Sponaugle S., Robinson K. L., Luo J. Y. (2015). PlanktonSet 1.0: Plankton Imagery Data Collected From F.G. Walton Smith in Straits of Florida From 2014-06-03 to 2014-06-06 and Used in the 2015 National Data Science Bowl (Ncei Accession 0127422) (National Centers for Environmental Information). Available at: https://www.ncei.noaa.gov/access/metadata/landing-page/bin/iso?id=gov.noaa.nodc:0127422.
Culverhouse P. F., Williams R., Reguera B., Herry V., González-Gil S. (2003). Do Experts Make Mistakes? A Comparison of Human and Machine Indentification of Dinoflagellates. Mar. Ecol. Prog. Ser. 247, 17–25. doi: 10.3354/meps247017
Delmont T. O., Quince C., Shaiber A., Esen Ö. C., Lee S., Rappé M. S., et al. (2018). Nitrogen-Fixing Populations of Planctomycetes and Proteobacteria Are Abundant in Surface Ocean Metagenomes. Nat. Microbiol. 3, 804–813. doi: 10.1038/s41564-018-0176-9
Delmont T. O., Pierella Karlusich J. J., Veseli I., Fuessel J., Eren A. M., Foster R. A., et al. (2021). Heterotrophic Bacterial Diazotrophs Are More Abundant Than Their Cyanobacterial Counterparts in Metagenomes Covering Most of the Sunlit Ocean. ISME J 16, 927–936. doi: 10.1038/s41396-021-01135-1
DeLong E. F., Karl D. M. (2005). Genomic Perspectives in Microbial Oceanography’. Nature 437 (7057), 336–342. doi: 10.1038/nature04157
Detmer T. M., Broadway K. J., Potter C. G., Collins S. F., Parkos J. J., Wahl D.H., et al. (2019). Comparison of Microscopy to a Semi-Automated Method (FlowCAM®) for Characterization of Individual-, Population-, and Community-Level Measurements of Zooplankton. Hydrobiologia 838 (1), 99–110. doi: 10.1007/s10750-019-03980-w
de Vargas C., Audic S., Henry N., Decelle J., Mahé F., Logares R., et al. (2015). Ocean Plankton. Eukaryotic Plankton Diversity in the Sunlit Ocean. Science 348 (6237), 1261605. doi: 10.1126/science.1261605
de Vargas C., Pollina T., Romac S., Le Bescot N., Henry N., Berger C., et al. (2020). Plankton Planet: “Seatizen” Oceanography to Assess Open Ocean Life at the Planetary Scale. bioRxiv. doi: 10.1101/2020.08.31.263442
Elineau A., Desnos C., Jalabert L., Olivier M., Romagnan J. B., Brandao M., et al. (2018). ZooScanNet: Plankton Images Captured With the Zooscan. SEANOE. doi: 10.17882/55741
Ellen J. S., Graff C. A., Ohman M. D. (2019). Improving Plankton Image Classification Using Context Metadata. Limnol. Oceanogr. Methods/ASLO 17 (8), 439–461. doi: 10.1002/lom3.10324
Falkowski P. (2012). Ocean Science: The Power of Plankton. Nature 483 (7387), S17–S20. doi: 10.1038/483S17a
Field C. B., Behrenfeld M. J., Randerson J. T., Falkowski P. (1998). Primary Production of the Biosphere: Integrating Terrestrial and Oceanic Components. Science 281, 237–240. doi: 10.1126/science.281.5374.237
FlowCAM Manual Version 3.2 (2012). Visual Spreadsheet 3.2, Portable, Open Benchtop, Benchtop, PV Models.’ Manual. Ed. Spaulding B. (Maine, USA:Laboratory Manager).
Fox L., Stukins S., Hill T., Miller C. G. (2020). Quantifying the Effect of Anthropogenic Climate Change on Calcifying Plankton. Sci. Rep. 10 (1), 1620. doi: 10.1038/s41598-020-58501-w
Gall L. L., Saunders G. W. (2010). DNA Barcoding is a Powerful Tool to Uncover Algal Diversity: A Case Study of the Phyllophoraceae (Gigartinales, Rhodophyta) in the Canadian Flora’. J. Phycology 46, 374–389. doi: 10.1111/j.1529-8817.2010.00807.x
García-Comas C., Stemmann L., Ibanez F., Berline L., Mazzocchi M. G., Gasparini S., et al. (2011). Zooplankton Long-Term Changes in the NW Mediterranean Sea: Decadal Periodicity Forced by Winter Hydrographic Conditions Related to Large-Scale Atmospheric Changes? J. Marine systems: J. Eur. Assoc. Mar. Sci. Techniques 87 (3-4), 216–226. doi: 10.1016/j.jmarsys.2011.04.003
Gaskell D. E., Ohman M. D., Hull P. M. (2019). Zooglider-Based Measurements of Planktonic Foraminifera in the California Current System. J. Foraminiferal Res. 49 (4), 390–404. doi: 10.2113/gsjfr.49.4.390
Gasol J. M., Morán X. A. G. (2015). Flow Cytometric Determination of Microbial Abundances and its Use to Obtain Indices of Community Structure and Relative Activity In McGenity T. J., Timmis K. N., Nogales B. (Eds.), Springer Protocols Handbooks (pp. 159–187). Springer Berlin Heidelberg: Springer. doi: 10.1007/8623_2015_139
Gasparini S., Antajan E. (2007). “Plankton Identifier: A Software for Automatic Recognition of Planktonic Organisms,” in User’s manual. Available at: http://www.obs-vlfr.fr/~gaspari/Plankton_Identifier/index.php.
Godhe A., Asplund M. E., Härnström K., Saravanan V., Tyagi A., Karunasagar I. (2008). Quantification of Diatom and Dinoflagellate Biomasses in Coastal Marine Seawater Samples by Real-Time Pcr. Appl. Environ. Microbiol. 74, 7174–7182. doi: 10.1128/AEM.01298-08
Gong W., Marchetti A. (2019). Estimation of 18S Gene Copy Number in Marine Eukaryotic Plankton Using a Next-Generation Sequencing Approach. Front. Mar. Sci. 6, 219. doi: 10.3389/fmars.2019.00219
González P., Álvarez E., Díez J., López-Urrutia Á., del Coz J.J. (2017). Validation Methods for Plankton Image Classification Systems. Limnol. Oceanogr. Methods 15 (3), 221–237. doi: 10.1002/lom3.10151
Gorsky G., Ohman M. D., Picheral M., Gasparini S., Stemmann L., Romagnan J. B., et al. (2010). Digital Zooplankton Image Analysis Using the ZooScan Integrated System. J. Plankton Res. 32 (3), 285–303. doi: 10.1093/plankt/fbp124
Grosjean P., Denis K., Wacquet G. (2018) Zooimage: Analysis of Numerical Plankton Images. Available at: https://cran.r-project.org/web/packages/zooimage/.
Guidi L., Legendre L., Reygondeau G., Uitz J., Stemmann L., Henson S.A. (2015). A New Look at Ocean Carbon Remineralization for Estimating Deepwater Sequestration. Global Biogeochemical Cycles 29(7), 1044–1059. doi: 10.1002/2014gb005063
Guillou L., Bachar D., Audic S., Bass D., Berney C., Bittner L., et al. (2013). The Protist Ribosomal Reference Database (PR2): A Catalog of Unicellular Eukaryote Small Sub-Unit rRNA Sequences With Curated Taxonomy. Nucleic Acids Res. 41, D597–D604. doi: 10.1093/nar/gks1160
Hails A., Boyes C., Boyes A., Currier R. D., Henderson K., Kotlewski A., Kirkpatrick G.J., et al. (2009). “The Optical Phytoplankton Discriminator,” in Oceans 2009 (Biloxi, Mississippi, USA:IEEE). doi: 10.23919/oceans.2009.5422324
Haraguchi L., Jakobsen H. H., Lundholm N., Carstensen J. (2017). Monitoring Natural Phytoplankton Communities: A Comparison Between Traditional Methods and Pulse-Shape Recording Flow Cytometry. Aquat. Microbial Ecol. 80 (1), 77–92. doi: 10.3354/ame01842
Hays G. C., Richardson A. J., Robinson C. (2005). Climate Change and Marine Plankton. Trends Ecol. Evol. 20 (6), 337–344. doi: 10.1016/j.tree.2005.03.004
Heeger F., Bourne E. C., Baschien C., Yurkov A., Bunk B., Spröer C., et al. (2018). Long-Read DNA Metabarcoding of Ribosomal RNA in the Analysis of Fungi From Aquatic Environments. Mol. Ecol. Resour. 18, 1500–1514. doi: 10.1111/1755-0998.12937
Heller P., Tripp H. J., Turk-Kubo K., Zehr J. P. (2014). Arbitrator: A Software Pipeline for on-Demand Retrieval of Auto-Curated Nifh Sequences From Genbank. Bioinformatics 30 (20), 2883–2890. doi: 10.1093/bioinformatics/btu417
Henrichs D. W., Anglés S., Gaonkar C. C., Campbell L. (2021). Application of a Convolutional Neural Network to Improve Automated Early Warning of Harmful Algal Blooms. Environ. Sci. Pollution Res. Int. 28 (22), 28544–28555. doi: 10.1007/s11356-021-12471-2
Hrycik A. R., Shambaugh A., Stockwell J. D. (2019). ‘Comparison of FlowCAM and Microscope Biovolume Measurements for a Diverse Freshwater Phytoplankton Community. J. Plankton Res. 41 (6), 849–864. doi: 10.1093/plankt/fbz056
Hull P. M., Osborn K. J., Norris R. D., Robison B. H. (2011). Seasonality and Depth Distribution of a Mesopelagic Foraminifer, Hastigerinella Digitata, in Monterey Bay, California. Limnol. Oceanogr. 56 (2), 562–576. doi: 10.4319/lo.2011.56.2.0562
Ibarbalz F. M., Henry N., Brandáo M. C., Martini S., Busseni G., Byrne H, et al. (2019). Global Trends in Marine Plankton Diversity Across Kingdoms of Life. Cell 179 (5), 1084–1097. doi: 10.1016/j.cell.2019.10.008
Irisson J. O., Ayata S. D., Lindsay D. J., Karp-Boss L., Stemmann L. (2022). Machine Learning for the Study of Plankton and Marine Snow From Images. Annu. Rev. Mar. Sci. 14, 277–301. doi: 10.1146/annurev-marine-041921-013023
Jain M., Olsen H. E., Paten B., Akeson M. (2016). The Oxford Nanopore MinION: Delivery of Nanopore Sequencing to the Genomics Community. Genome Biol. 17 (1), 239. doi: 10.1186/s13059-016-1103-0
Jakobsen H. H., Carstensen J. (2011). FlowCAM: Sizing Cells and Understanding the Impact of Size Distributions on Biovolume of Planktonic Community Structure. Aquat. Microbial. Ecol. 65 (1), 75–87. doi: 10.3354/ame01539
Jamy M., Foster R., Barbera P., Czech L., Kozlov A., Stamatakis A., et al. (2020). Long-Read Metabarcoding of the Eukaryotic rDNA Operon to Phylogenetically and Taxonomically Resolve Environmental Diversity. Mol. Ecol. Resour. 20, 429–443. doi: 10.1111/1755-0998.13117
Jeffrey S. W. (1974). Profiles of Photosynthetic Pigments in the Ocean Using Thin-Layer Chromatography. Mar. Biol. 26(2), 101–110. doi: 10.1007/bf00388879
Jeffrey S. W., Wright S. W., Zapata M. (1999). Recent Advances in HPLC Pigment Analysis of Phytoplankton. Mar. Freshwater Res. 50 (8), 879. doi: 10.1071/MF99109
Ji Y., Ashton L., Pedley S. M., Edwards D. P., Tang Y., Nakamura A., et al. (2013). Reliable, Verifiable and Efficient Monitoring of Biodiversity Via Metabarcoding. Ecol. Lett. 16 (10), 1245–1257. doi: 10.1111/ele.12162
Kembel S. W., Wu M., Eisen J. A., Green J. L. (2012). Incorporating 16S Gene Copy Number Information Improves Estimates of Microbial Diversity and Abundance. PloS Comput. Biol. 8 (10), e1002743. doi: 10.1371/journal.pcbi.1002743
Kiko R., Christiansen S., Schröder S.-M., Koch R., Stemmann L. (2018) PlanktonID – Combining Deep Learning, In Situ Imaging and Citizen Science to Resolve the Distribution of Zooplankton in Major Upwelling Regions in 10th International Conference on Ecological Informatics-Translating Ecological Data Into Knowledge and Decisions in a Rapidly Changing World 2018 (ICEI 2018) (Jena, Ger.: Friedrich-Schiller-Univ. Jena).
Kraft K., Seppälä J., Hällfors H., Suikkanen S., Ylöstalo P., Anglès S., et al. (2021). First Application of IFCB High-Frequency Imaging-in-Flow Cytometry to Investigate Bloom-Forming Filamentous Cyanobacteria in the Baltic Sea. Front. Mar. Sci. 8, 282. doi: 10.3389/fmars.2021.594144
Krizhevsky A., Sutskever I., Hinton G. E. (2012). “ImageNet Classification With Deep Convolutional Neural Networks,” in Advances in Neural Information Processing Systems, vol. 25. (Red Hook, NY: Curran).
Kucera H., Saunders G. W. (2008). Assigning Morphological Variants of Fucus (Fucales, Phaeophyceae) in Canadian Waters to Recognized Species Using DNA Barcoding. Botany 86, 1065–1079. doi: 10.1139/B08-056
Kyathanahally S. P., Hardeman T., Merz E., Bulas T., Reyes M., Isles P., et al. (2021). Deep Learning Classification of Lake Zooplankton. Front. Microbiol. 12, 746297. doi: 10.3389/fmicb.2021.746297
Lamb P. D., Hunter E., Pinnegar J. K., Creer S., Davies R. G., Taylor M. I. (2019). How Quantitative Is Metabarcoding: A Meta-Analytical Approach. Mol. Ecol. 28 (2), 420–430. doi: 10.1111/mec.14920
Lavrinienko A., Jernfors T., Koskimäki J. J., Pirttilä A. M., Watts P. C. (2021). Does Intraspecific Variation in rDNA Copy Number Affect Analysis of Microbial Communities? Trends Microbiol. 29 (1), 19–2. doi: 10.1016/j.tim.2020.05.019
Lee H., Park M., Kim J. (2016) in 2016 IEEE International Conference on Image Processing (ICIP) (IEEE). doi: 10.1109/icip.2016.7533053
Lefever S., Pattyn F., Hellemans J., Vandesompele J. (2013). Single-Nucleotide Polymorphisms and Other Mismatches Reduce Performance of Quantitative PCR Assays. Clin. Chem. 59, 1470–1480. doi: 10.1373/clinchem.2013.203653
Liu Z., Lozupone C., Hamady M., Bushman F. D., Knight R. (2007). Short Pyrosequencing Reads Suffice for Accurate Microbial Community Analysis. Nucleic Acids Res. 35, e120. doi: 10.1093/nar/gkm541
Logares R., Sunagawa S., Salazar G., Cornejo-Castillo F. M., Ferrera I., Sarmento H., et al. (2014). Metagenomic 16S Rdna Illumina Tags Are a Powerful Alternative to Amplicon Sequencing to Explore Diversity and Structure of Microbial Communities. Environ. Microbiol. 16 (9), 2659–2671. doi: 10.1111/1462-2920.12250
Lombard F., Boss E., Waite A. M., Vogt M., Uitz J., Stemmann L., et al. (2019). Globally Consistent Quantitative Observations of Planktonic Ecosystems. Front. Mar. Sci. 6. doi: 10.3389/fmars.2019.00196
Louca S., Doebeli M., Parfrey L. W. (2018). Correcting for 16S rRNA Gene Copy Numbers in Microbiome Surveys Remains an Unsolved Problem. Microbiome 6 (1), 41. doi: 10.1186/s40168-018-0420-9
Lu H., Giordano F., Ning Z. (2016). Oxford Nanopore MinION Sequencing and Genome Assembly. Genomics Proteomics Bioinf. 14 (5), 265–279. doi: 10.1016/j.gpb.2016.05.004
Lumini A., Nanni L. (2019). Deep Learning and Transfer Learning Features for Plankton Classification. Ecol. Inf. 51, 33–43. doi: 10.1016/j.ecoinf.2019.02.007
Luo J. Y., Irisson J. O., Graham B., Guigand C., Sarafraz A., Mader C., et al. (2018). Automated Plankton Image Analysis Using Convolutional Neural Networks. Limnol. Oceanogr. Methods/ASLO 16 (12), 814–827. doi: 10.1002/lom3.10285
Mackey M. D., Mackey D. J., Higgins H. W., Wright S. W. (1996). CHEMTAX - A Program for Estimating Class Abundances From Chemical Markers:Application to HPLC Measurements of Phytoplankton. Mar. Ecol. Prog. Ser. 144, 265–283. doi: 10.3354/meps144265
Mäki A., Salmi P., Mikkonen A., Kremp A., Tiirola M. (2017). Sample Preservation, DNA or RNA Extraction and Data Analysis for High-Throughput Phytoplankton Community Sequencing. Front. Microbiol. 8, 1848. doi: 10.3389/fmicb.2017.01848
Milivojević T., Rahman S. N., Raposo D., Siccha M., Kucera M., Morard R. (2021). High Variability in SSU rDNA Gene Copy Number Among Planktonic Foraminifera Revealed by Single-Cell Qpcr. ISME Commun. 1, 63. doi: 10.1038/s43705-021-00067-3
Miloslavich P., Bax N. J., Simmons S. E., Klein E., Appeltans W., Aburto-Oropeza O., et al. (2018). Essential Ocean Variables for Global Sustained Observations of Biodiversity and Ecosystem Changes. Global Change Biol. 24 (6), 2416–2433. doi: 10.1111/gcb.14108
Moore T. S., Mouw C. B., Sullivan J. M., Twardowski M. S., Burtner A. M., Ciochetto A. B., et al. (2017). Bio-Optical Properties of Cyanobacteria Blooms in Western Lake Erie. Front. Marine Sci. 4. doi: 10.3389/fmars.2017.00300
Moore T. S., Churnside J. H., Sullivan J. M., Twardowski M. S., Nayak A. R., McFarland M. N., et al. (2019). Vertical Distributions of Blooming Cyanobacteria Populations in a Freshwater Lake From LIDAR Observations. Remote Sens. Environ. 225, 347–367. doi: 10.1016/j.rse.2019.02.025
Muller-Karger F. E., Miloslavich P., Bax N. J., Simmons S., Costello M. J., Sousa Pinto I., et al. (2018). Advancing Marine Biological Observations and Data Requirements of the Complementary Essential Ocean Variables (Eovs) and Essential Biodiversity Variables (Ebvs) Frameworks. Front. Mar. Sci. 5. doi: 10.3389/fmars.2018.00211
Obiol A., Giner C. R., Sánchez P., Duarte C. M., Acinas S. G., Massana R., et al. (2020). A Metagenomic Assessment of Microbial Eukaryotic Diversity in the Global Ocean. Mol. Ecol. Resour. 20 (3), 718–731. doi: 10.1111/1755-0998.13147
Olson R. J., Sosik H. M. (2007). A Submersible Imaging-in-Flow Instrument to Analyze Nano-and Microplankton: Imaging Flowcytobot. Limnol. Oceanography: Methods/ASLO 5 (6), 195–203. doi: 10.4319/lom.2007.5.195
Orenstein E., Ayata S. D., Maps F., Biard T., Becker É., Benedetti F., et al. (2021). Machine Learning Techniques to Characterize Functional Traits of Plankton From Image Data. ffhal–03482282f.
Orr R. J., Zhao S., Klaveness D., Yabuki A., Ikeda K., Watanabe M. M., Shalchian-Tabrizi K., et al. (2018). Enigmatic Diphyllatea Eukaryotes: Culturing and Targeted PacBio RS Amplicon Sequencing Reveals a Higher Order Taxonomic Diversity and Global Distribution. BMC Evol. Biol. 18, 115. doi: 10.1186/s12862-018-1224-z
Ottesen E. A., Marin R., Preston C. M., Young C. R., Ryan J. P., Scholin C. A., DeLong E. F. (2011). Metatranscriptomic Analysis of Autonomously Collected and Preserved Marine Bacterioplankton. ISME J. 5, 1881–1895. doi: 10.1038/ismej.2011.70
Ottesen E. A., Young C. R., Eppley J. M., Ryan J. P., Chavez F. P., Scholin C. A., DeLong E. F. (2013). Pattern and Synchrony of Gene Expression Among Sympatric Marine Microbial Populations. Proc. Natl. Acad. Sci. U.S.A. 110, E488–E497. doi: 10.1073/pnas.1222099110
Parada A. E., Needham D. M., Fuhrman J. A. (2016). Every Base Matters: Assessing Small Subunit rRNA Primers for Marine Microbiomes With Mock Communities, Time Series and Global Field Samples. Environ. Microbiol. 18 (5), 1403–1414. doi: 10.1111/1462-2920.13023
Pargett D. M., Birch J. M., Preston C. M., Ryan J. P., Zhang Y., Scholin C.A. (2015). “Development of a Mobile Ecogenomic Sensor,” in Oceans 2015 (Washington: MTS/IEEE). doi: 10.23919/oceans.2015.7404361
Pawlowski J., Audic S., Adl S., Bass D., Belbahri L., Berney C., et al. (2012). Cbol Protist Working Group: Barcoding Eukaryotic Richness Beyond the Animal, Plant, and Fungal Kingdoms. PloS Biol. 10, e1001419. doi: 10.1371/journal.pbio.1001419
Pearson D. L., Hamilton A. L., Erwin T. L. (2011). Recovery Plan for the Endangered Taxonomy Profession. Bioscience 61 (1), 58–63. doi: 10.1525/bio.2011.61.1.11
Pesant S., Not F., Picheral M., Kandels-Lewis S., Le Bescot N., Gorsky G., et al. (2015). Open Science Resources for the Discovery and Analysis of Tara Oceans Data. Sci. Data 2, 150023. doi: 10.1038/sdata.2015.23
Picheral M., Guidi L., Stemmann L., Karl D. M., Iddaoud G., Gorsky G. (2010). The Underwater Vision Profiler 5: An Advanced Instrument for High Spatial Resolution Studies of Particle Size Spectra and Zooplankton. Limnol. Oceanography Methods/ASLO 8 (9), 462–473. doi: 10.4319/lom.2010.8.462
Picheral M., Catalano C., Brousseau D., Claustre H., Coppola L., Leymarie E., et al. (2021). The Underwater Vision Profiler 6: An Imaging Sensor of Particle Size Spectra and Plankton, for Autonomous and Cabled Platforms. Limnol. Oceanography Methods/ASLO 20(2), 115–129. doi: 10.1002/lom3.10475
Picheral M., Colin S., Irisson J.-O. (2017). EcoTaxa, a Tool for the Taxonomic Classification of Images. Available at:http://ecotaxa.obs-vlf.
Pierella Karlusich J. J., Pelletier E., Lombard F., Carsique M., Dvorak E., Colin S., et al. (2021). Global Distribution Patterns of Marine Nitrogen-Fixers by Imaging and Molecular Methods. Nat. Commun. 12 (1), 1–18. doi: 10.1038/s41467-021-24299-y
Pierella Karlusich J. J., Pelletier E., Zinger L., Lombard F., Zingone A., Colin S., et al. (2022). A Robust Approach to Estimate Relative Phytoplankton Cell Abundances From Metagenomes. Mol. Ecol. Resour. doi: 10.1111/1755-0998.13592
Pierella Karlusich J. J., Ibarbalz F. M., Bowler C. (2020a). Exploration of Marine Phytoplankton: From Their Historical Appreciation to the Omics Era. J. Plankton Res. 42 (6), 595–612. doi: 10.1093/plankt/fbaa049
Pierella Karlusich J. J., Ibarbalz F. M., Bowler C. (2020b). Phytoplankton in the Tara Ocean. Annu. Rev. Mar. Sci. 12, 233–265. doi: 10.1146/annurev-marine-010419-010706
Plonus R. M., Conradt J., Harmer A., Janßen S., Floeter J.. (2021). Automatic Plankton Image Classification—Can Capsules and Filters Help Cope With Data Set Shift? Limnol. Oceanography Methods/ASLO 19 (3), 176–195. doi: 10.1002/lom3.10413
Pollina T., Larson A. G., Lombard F., Li H., Colin S., de Vargas C., Prakash M. (2020). Planktonscope: Affordable Modular Imaging Platform for Citizen Oceanography. bioRxiv. doi: 10.1101/2020.04.23.056978
Polz M. F., Cavanaugh C. M. (1998). Bias in Template-to-Product Ratios in Multitemplate PCR. Appl. Environ. Microbiol. 64 (10), 3724–3730. doi: 10.1128/AEM.64.10.3724-3730.1998
Preston C. M., Harris A., Ryan J. P., Roman B., Marin R. III, Jensen S., et al. (2011). Underwater Application of Quantitative PCR on an Ocean Mooring. PloS One 6, e22522. doi: 10.1371/journal.pone.0022522
Quast C., Pruesse E., Yilmaz P., Gerken J., Schweer T., Yarza P., et al. (2013). The SILVA Ribosomal RNA Gene Database Project: Improved Data Processing and Web-Based Tools. Nucleic Acids Res. 41, D590–D596. doi: 10.1093/nar/gks1219
Remsen A., Hopkins T. L., Samson S. (2004). What You See Is Not What You Catch: A Comparison of Concurrently Collected Net, Optical Plankton Counter, and Shadowed Image Particle Profiling Evaluation Recorder Data From the Northeast Gulf of Mexico. Deep-sea Res. Part I Oceanographic Res. Papers 51 (1), 129–151. doi: 10.1016/j.dsr.2003.09.008
Riedel A., Sagata K., Suhardjono Y. R., Tänzler R., Balke M. (2013). Integrative Taxonomy on the Fast Track - Towards More Sustainability in Biodiversity Research. Front. Zool 10 (1), 15. doi: 10.1186/1742-9994-10-15
Robidart J. C., Church M. J., Ryan J. P., Ascani F., Wilson S. T., Bombar D., et al. (2014). Ecogenomic Sensor Reveals Controls on N2-fixing Microorganisms in the North Pacific Ocean. ISME J. 8, 1175–1185. doi: 10.1038/ismej.2013.244
Robideau G. P., De Cock A. W., Coffey M. D., Voglmayr H., Brouwer H., Bala K., et al. (2011). DNA Barcoding of Oomycetes With Cytochrome C Oxidase Subunit I and Internal Transcribed Spacer. Mol. Ecol. Resour. 11, 1002–1011. doi: 10.1111/j.1755-0998.2011.03041.x
Robinson K. L., Luo J. Y., Sponaugle S., Guigand C., Cowen R. K. (2017). A Tale of Two Crowds: Public Engagement in Plankton Classification. Front. Marine Sci. 11 (4), 82. doi: 10.3389/fmars.2017.00082
Russakovsky O., Deng J., Su H., Krause J., Satheesh S., Ma S., et al. (2015). ImageNet Large Scale Visual Recognition Challenge. Int. J. Comput. Vision 115, 211–252. doi: 10.1007/s11263-015-0816-y
Ryan J. P., Kudela R. M., Birch J. M., Blum M., Bowers H. A., Chavez F. P., et al. (2017). Causality of an Extreme Harmful Algal Bloom in Monterey Bay, California, During the 2014–2016 Northeast Pacific Warm Anomaly’, Geophys. Res. Lett. 44(11), 5571–5579. doi: 10.1002/2017GL072637
Santoferrara L. F. (2019). Current Practice in Plankton Metabarcoding: Optimization and Error Management. J. Plankton Res. 41 (5), 571–582. doi: 10.1093/plankt/fbz041
Schmid M. S., Aubry C., Grigor J., Fortier L. (2016). The LOKI Underwater Imaging System and an Automatic Identification Model for the Detection of Zooplankton Taxa in the Arctic Ocean. Methods Oceanography 15, 129–160. doi: 10.1016/j.mio.2016.03.003
Schoch C. L., Seifert K. A., Huhndorf S., Robert V., Spouge J. L., Levesque C. A., et al. (2012). Nuclear Ribosomal Internal Transcribed Spacer (Its) Region as a Universal DNA Barcode Marker for Fungi. Proc. Natl. Acad. Sci. U.S.A. 109, 6241–6246. doi: 10.1073/pnas.1117018109
Schofield O., Kerfoot J., Mahoney K., Moline M., Oliver M., Lohrenz S., Kirkpatrick G. (2006). Vertical Migration of the Toxic Dinoflagellate Karenia Brevis and the Impact on Ocean Optical Properties. J. Geophysical Res. 111, C06009. doi: 10.1029/2005JC003115
Scholin C. A., Birch J., Jensen S., Marin R. III, Massion E., Pargett D., et al. (2017). The Quest to Develop Ecogenomic Sensors: A 25-Year History of the Environmental Sample Processor (ESP) as a Case Study. Oceanogr. 30 (4), 100–113. doi: 10.5670/oceanog.2017.427
Schröder S.-M., Kiko R., Koch R. (2020). MorphoCluster: Efficient Annotation of Plankton Images by Clustering. Sensors 20 (11), 3060. doi: 10.3390/s20113060
Schvarcz C. R., Wilson S. T., Caffin M., Stancheva R., Li Q., Turk-Kubo K. A., et al. (2022). Overlooked and Widespread Pennate Diatom-Diazotroph Symbioses in the Sea. Nat. Commun. 13 (1), 799. doi: 10.1038/s41467-022-28065-6
Seegers B. N., Birch J. M., Marin R. III, Scholin C. A., Caron D. A., Seubert E. L., et al. (2015). Subsurface Seeding of Surface Harmful Algal Blooms Observed Through the Integration of Autonomous Gliders, Moored Environmental Sample Processors, and Satellite Remote Sensing in Southern California. Limnol. Oceanography 60 (3), 754–764. doi: 10.1002/lno.10082
Shin H., Lee E., Shin J., Ko S. R., Oh H. S., Ahn C. Y., et al. (2018). Elucidation of the Bacterial Communities Associated With the Harmful Microalgae Alexandrium Tamarense and Cochlodinium Polykrikoides Using Nanopore Sequencing. Sci. Rep. 8 (1), 5323. doi: 10.1038/s41598-018-23634-6
Sieracki C. K., Sieracki M. E., Yentsch C. S. (1998). An Imaging-in-Flow System for Automated Analysis of Marine Microplankton. Marine Ecol. Prog. Ser. 168, 285–296. doi: 10.3354/meps168285
Sosik H. M., Olson R. J. (2007). Automated Taxonomic Classification of Phytoplankton Sampled With Imaging-in-Flow Cytometry. Limnol. Oceanography Methods/ASLO 5 (6), 204–216. doi: 10.4319/lom.2007.5.204
Sosik H. M., Peacock E. E., Brownlee E. F. (2021a). WHOI-Plankton: Annotated Plankton Images - Dataset for Developing and Evaluating Classification Methods (Woods Hole Open Access Server). Available at: http://hdl.handle.net/1912/7341.
Spanbauer T. L., Briseño-Avena C., Pitz K. J., Suter E.. (2020). Salty Sensors, Fresh Ideas: The Use of Molecular and Imaging Sensors in Understanding Plankton Dynamics Across Marine and Freshwater Ecosystems. Limnol. Oceanography Lett. 5 (2), 169–184. doi: 10.1002/lol2.10128
Starke R., Pylro V. S., Morais D. K. (2020). 16s rRNA Gene Copy Number Normalization Does Not Provide More Reliable Conclusions in Metataxonomic Surveys. Microbial Ecol. 81 (2), 535–539. doi: 10.1007/s00248-020-01586-7
Stern R., Moore S. K., Trainer V. L., Bill B. D., Fischer A., Batten S. (2018). Spatial and Temporal Patterns of Pseudo-nitzschia Genetic Diversity in the North Pacific Ocean From the Continuous Plankton Recorder Survey. Mar. Ecol. Prog. Ser. 606, 7–28. doi: 10.3354/meps12711
Stern R., Schroeder D., Highfield A., Al-Kandari M., Vezzulli L., Richardson A. (2022). “Uses of Molecular Taxonomy in Identifying Phytoplankton Communities From the Continuous Plankton Recorder Survey,” in Advances in Phytoplankton Ecology (Elsevier), 47–79. Available at: https://www.sciencedirect.com/book/9780128228616/advances-in-phytoplankton-ecology
Stern R. F., Horak A., Andrew R. L., Coffroth M.-A., Andersen R. A., Veron B., et al. (2010). Environmental Barcoding Reveals Massive Dinoflagellate Diversity in Marine Environments. PloS One 5, e13991. doi: 10.1371/journal.pone.0013991
Sunagawa S., Mende D. R., Zeller G., Izquierdo-Carrasco F., Berger S. A., Kultima J. R., et al. (2013). Metagenomic Species Profiling Using Universal Phylogenetic Marker Genes. Nat. Methods 10 (12), 1196–1199. doi: 10.1038/nmeth.2693
Sunagawa S., Acinas S. G., Bork P., Bowler C., Eveillard D., Gorsky G., et al. (2020). Tara Oceans: Towards Global Ocean Ecosystems Biology’, Nature Reviews. Microbiology 18 (8), 428–445. doi: 10.1038/s41579-020-0364-5
Šlapeta J., López-García P., Moreira D. (2006). Global Dispersal and Ancient Cryptic Species in the Smallest Marine Eukaryotes. Mol. Biol. Evol. 23(1), 23–29. doi: 10.1093/molbev/msj001
Tedersoo L., Tooming-Klunderud A., Anslan S. (2018). PacBio Metabarcoding of Fungi and Other Eukaryotes: Errors, Biases and Perspectives. New Phytol. 217, 1370–1385. doi: 10.1111/nph.14776
Tully B., Graham E., Heidelberg J. (2018). The Reconstruction of 2,631 Draft Metagenome-Assembled Genomes From the Global Oceans. Sci. Data 5, 170203. doi: 10.1038/sdata.2017.203
Urban L., Holzer A., Baronas J. J., Hall M. B., Braeuninger-Weimer P., Scherm M. J., et al. (2020). Freshwater Monitoring by Nanopore Sequencing. Elife 10, e61504. doi: 10.7554/eLife.61504.sa2
Ussler W., Preston C., Tavormina P., Pargett D., Jensen S., Roman B., et al. (2013). Autonomous Application of Quantitative PCR in the Deep Sea: In Situ Surveys of Aerobic Methanotrophs Using the Deep-Sea Environmental Sample Processor. Environ. Sci. Technol. 47, 9339–9346. doi: 10.1021/es4023199
Utermöhl H. (1958). Zur Vervollkommnung Der Quantitativen Phytoplankton-Methodik. SIL Commun. 1953-1996, 1–38. doi: 10.1080/05384680.1958.11904091
van der Loos L. M., Nijland R. (2021). Biases in Bulk: DNA Metabarcoding of Marine Communities and the Methodology Involved. Mol. Ecol. 30 (13), 3270–3288. doi: 10.1111/mec.15592
Vandromme P., Stemmann L., Berline L., Gasparini S., Mousseau L., Prejger F., et al. (2011). Inter-Annual Fluctuations of Zooplankton Communities in the Bay of Villefranche-sur-mer From 1995 to 2005 (Northern Ligurian Sea, France). Biogeosciences 8 (11), 3143–3158. doi: 10.5194/bg-8-3143-2011
Varaljay V. A., Robidart J., Preston C. M., Gifford S. M., Durham B. P., Burns A. S., et al. (2015). Single-Taxon Field Measurements of Bacterial Gene Regulation Controlling DMSP Fate. ISME J. 9 (1), 677–671. doi: 10.1038/ismej.2015.23
Vilgrain L., Maps F., Picheral M., Babin M., Aubry C., Irisson J. O., Ayata S. D.. (2021). Trait-Based Approach Using In Situ Copepod Images Reveals Contrasting Ecological Patterns Across an Arctic Ice Melt Zone. Limnol. Oceanogr. 1155–1167. doi: 10.1002/lno.11672
Vincent F. J., Colin S., Romac S., Scalco E., Bittner L., Garcia Y., et al. (2018). The Epibiotic Life of the Cosmopolitan Diatom Fragilariopsis Doliolus on Heterotrophic Ciliates in the Open Ocean. ISME J. 12 (4), 1094–1108. doi: 10.1038/s41396-017-0029-1
Warner A. J., Hays G. C. (1994). Sampling by the Continuous Plankton Recorder Survey. Prog. Oceanography 34, 237–256. doi: 10.1016/0079-6611(94)90011-6
Warwick-Dugdale J., Solonenko N., Moore K., Chittick L., Gregory A. C., Allen M. J., et al. (2019). Long-Read Viral Metagenomics Captures Abundant and Microdiverse Viral Populations and Their Niche-Defining Genomic Islands. PeerJ 7, e6800. doi: 10.7717/peerj.6800
Wear E. K., Wilbanks E. G., Nelson C. E., Carlson C. A. (2018). Primer Selection Impacts Specific Population Abundances But Not Community Dynamics in a Monthly Time-Series 16S rRNA Gene Amplicon Analysis of Coastal Marine Bacterioplankton. Environ. Microbiol. 20, 2709–2726. doi: 10.1111/1462-2920.14091
Whitt C., Pearlman J., Polagye B., Caimi F., Muller-Karger F., Copping A., et al. (2020). Future Vision for Autonomous Ocean Observations. Front. Mar. Sci. 7. doi: 10.3389/fmars.2020.00697
Wiebe P. H., Benfield M. C. (2003). From the Hensen Net Toward Four-Dimensional Biological Oceanography. Prog. Oceanogr. 56 (1), 7–136. doi: 10.1016/S0079-6611(02)00140-4
Wilkinson M. D., Dumontier M., Aalbersberg I. J., Appleton G., Axton M., Baak A., et al. (2016). The FAIR Guiding Principles for Scientific Data Management and Stewardship. Sci. Data 3, 160018. doi: 10.1038/sdata.2016.18
Williamson C. E., Saros J. E., Schindler D. W. (2009). Climate Change. Sentinels of Change. Science 323 (5916), 887–888. doi: 10.1126/science.1169443
Yamahara K. M., Demir-Hilton E., Preston C. M., Marin R. III, Pargett D., Roman B., et al. (2015). Simultaneous Monitoring of Faecal Indicators and Harmful Algae Using an in-Situ Autonomous Sensor. Lett. Appl. Microbiol. 61 (2), 130–138. doi: 10.1111/lam.12432
Keywords: plankton, metabarcoding, metagenomics, high-throughput imaging, machine learning, EcoTaxa
Citation: Pierella Karlusich JJ, Lombard F, Irisson J-O, Bowler C and Foster RA (2022) Coupling Imaging and Omics in Plankton Surveys: State-of-the-Art, Challenges, and Future Directions. Front. Mar. Sci. 9:878803. doi: 10.3389/fmars.2022.878803
Received: 18 February 2022; Accepted: 27 April 2022;
Published: 03 June 2022.
Edited by:
Danny Ionescu, Leibniz-Institute of Freshwater Ecology and Inland Fisheries (IGB), GermanyReviewed by:
Michal Kucera, University of Bremen, GermanyKaisa Kraft, Finnish Environment Institute (SYKE), Finland
Lumi Haraguchi, Finnish Environment Institute (SYKE), Finland
Copyright © 2022 Pierella Karlusich, Lombard, Irisson, Bowler and Foster. This is an open-access article distributed under the terms of the Creative Commons Attribution License (CC BY). The use, distribution or reproduction in other forums is permitted, provided the original author(s) and the copyright owner(s) are credited and that the original publication in this journal is cited, in accordance with accepted academic practice. No use, distribution or reproduction is permitted which does not comply with these terms.
*Correspondence: Juan José Pierella Karlusich, pierella@biologie.ens.fr; Rachel A. Foster, rachel.foster@su.se
†ORCID: Juan José Pierella Karlusich, orcid.org/0000-0003-1739-4424
Fabien Lombard, orcid.org/0000-0002-8626-8782
Jean-Olivier Irisson, orcid.org/0000-0003-4920-3880
Chris Bowler, orcid.org/0000-0003-3835-6187
Rachel A. Foster, orcid.org/0000-0002-8696-1835