- 1Department of Gastroenterology, Peking University People’s Hospital, Beijing, China
- 2Clinical Center of Immune-Mediated Digestive Diseases, Peking University People’s Hospital, Beijing, China
- 3Institute of Clinical Molecular Biology and Central Laboratory, Peking University People’s Hospital, Beijing, China
Background: The aim of this study was to clarify the effect of 5-aminosalicylic acid (5-ASA) treatment on gut bacterial microbiota in patients with ulcerative colitis (UC).
Methods: A total of 57 UC patients, including 20 untreated and 37 5-ASA-treated, were recruited into an exploration cohort. We endoscopically collected both non-inflamed and inflamed mucosal samples from all patients, and compared the gut bacterial profiles using 16S rDNA sequencing. Ten untreated UC patients were then treated with 5-ASA and subsequently recruited for an independent validation study to confirm the acquired data.
Results: In untreated UC patients, compared with those in non-inflamed mucosae, Firmicutes (such as Enterococcus) were decreased and Proteobacteria (e.g., Escherichia–Shigella) were increased in the inflamed mucosae. Compared with the inflamed mucosae of untreated UC patients, there was a higher abundance of Firmicutes (e.g., Enterococcus) and lower Proteobacteria (Escherichia–Shigella) in the inflamed mucosae of 5-ASA treated UC patients. In the validation cohort, after administration of 5-ASA, bacterial alteration was consistent with these data. Furthermore, there was a skewed negative correlation between Escherichia–Shigella and bacterial genera of Firmicutes in the inflamed mucosae. 5-ASA treatment decreased the strength of bacterial correlation and weakened the skewed negative correlation pattern.
Conclusion: The microbial dysbiosis (mainly characterized by an increased abundance in the Escherichia–Shigella genus) and the skewed negative correlation between Escherichia–Shigella and bacterial genera of Firmicutes are two characteristics of the inflamed mucosae of UC patients. 5-ASA treatment decreases Escherichia–Shigella and weakens the skewed correlations, which may be related to its treatment efficiency.
Introduction
Ulcerative colitis (UC), a major form of inflammatory bowel disease (IBD), is an idiopathic chronic inflammatory condition affecting the colon and rectum, which influences life quality of patient (Ungaro et al., 2017). Although the pathogenesis of IBD remains unclear, it is accepted that bacterial dysbiosis is an important cause (Sartor and Wu, 2017). Bacterial dysbiosis in IBD is characterized by a reduction in bacterial diversity, a decrease in the Firmicutes phylum (Faecalibacterium, Blautia, Roseburia, etc.), and an increase in the Proteobacteria phylum (Enterobacteriaceae, including Escherichia) (Ott et al., 2004; Liguori et al., 2016; Sokol et al., 2016; Takahashi et al., 2016). Compared with healthy subjects, an increase in fecal Proteobacteria and a decrease in Firmicutes have been observed in IBD patients; additionally, compared with patients in remission, the mucosae of patients in an active stage were colonized with a higher abundance of Proteobacteria and a lower abundance of Firmicutes (Liguori et al., 2016; Sokol et al., 2016). Apart from bacterial imbalance, there are some reports on the intestinal microbiome in IBD patients, including fungal and viral microbiomes (Li et al., 2014; Norman et al., 2015; Liguori et al., 2016; Sokol et al., 2016; Butto and Haller, 2017; Hedin et al., 2017; Pascal et al., 2017).
It is reported that there was relative connection between inflammation and bacterial dysbiosis in IBD pathogenesis. For example, intestinal dysbiotic microbiota triggers a sustained and uninhibited inflammatory response by inducing effective cells, such as type 1, 9, and 17 T helper cells and innate lymphoid cells, to produce pro-inflammatory cytokines like interferon-γ, IL-17 and tumor necrosis factor α (Kamada et al., 2013; Rooks and Garrett, 2016; Belkaid and Harrison, 2017; Ungaro et al., 2017). Adversely, it is also reported that inflammation drove microbial dysbiosis (Zeng et al., 2017). Several studies provide evidence that the inflammatory tissue facilitates a growth advantage for pathogens such as Citrobacter rodentium and Salmonella (Barman et al., 2008; Kamada et al., 2012; Zeng et al., 2017). Thus, it is plausible for regulating inflammatory status to affect the microbiota. Nevertheless, the effect of regulating inflammation on the bacterial microbiota has been rarely studied in IBD patients.
Currently, 5-aminosalicylic acid (5-ASA), an anti-inflammatory modulator, is the primary therapeutic regimen for controlling inflammation in IBD patients (Laharie, 2017; Ungaro et al., 2017). Andrews et al. (2011) reported that 5-ASA altered fecal bacterial microbiota in patients with irritable bowel syndrome (IBS). In this study, 12 women with diarrhea-predominant IBS received 5-ASA treatment. Data from 16S rRNA sequencing showed a decrease of bacterial operational taxonomic units (OTUs), and an increase in the abundance of Firmicutes (Andrews et al., 2011). However, the effect of 5-ASA on mucosal bacterial microbiota in UC patients is still unclear. Therefore, we aim to clarify the effect of 5-ASA treatment on the bacterial microbiota in this study to gain insight into the probable causal relationships associated with 5-ASA therapy.
Materials and Methods
Study Subjects and Biopsy Collection
This study was approved by the Institutional Medical Ethics Review Board of Peking University People’s Hospital. All UC patients were enrolled from Peking University People’s Hospital from January 2015 to January 2017. The diagnosis of UC was established according to the World Gastroenterology Organization Global Guidelines (Bernstein et al., 2016). Apart from patients who did not undergo mucosal sampling, 57 patients in the activate stage of UC were recruited for an exploration cohort. Two groups, untreated (n = 20) and 5-ASA-treated (n = 37), were included in this cohort. Only patients following regimens for at least 1 month were classified in the 5-ASA-treated group. Furthermore, 10 UC patients from the 20 untreated patients in the exploration cohort underwent 5-ASA treatment for approximately 6 months and showed a decrease in their Mayo Endoscopic score (described below), but not a clinical complete remission. All of them were recruited into a validation cohort to confirm the data acquired from the exploration cohort (Table 1 and Supplementary Table S1). All recruited patients were requested to avoid using probiotics and antibiotics for at least 2 weeks before sampling. After that, for each patient, inflamed mucosae and adjacent non-inflamed (2–4 mm3 of each mucosa sample) mucosae were obtained endoscopically and stored at -80°C after freezing in liquid nitrogen until DNA was extracted. We defined “non-inflamed” and “inflamed” by using endoscopic observation (Lewis et al., 2008). The mucosa with following characters was considered as “endoscopic inflamed,” including erythema, decreased/absent vascular pattern, friability, erosions, spontaneous bleeding, or ulceration. The mucosa without these symptoms was defined as “endoscopic non-inflamed.”
DNA Extraction
Microbial genomic DNA was extracted from biopsy samples using the QIAamp DNA Stool Mini Kit (Qiagen, Hilden, Germany) according to the manufacturer’s instructions, with minor modifications. Briefly, each biopsy sample was re-suspended in 200 μL phosphate-buffered saline with 80 μL enzyme solution (22.5 mg lysozyme powder [Sigma-Aldrich, United States] and 40 units of mutanolysin dissolved in 80 μL 10 mM Tris-HCl/1 mM ethylenediaminetetraacetic acid [Sigma, United States]). After a 40-min incubation at 37°C, 2 zirconium beads (0.1 mm) were added, and the mixtures were homogenized in a Mini-bead Beater (FastPrep, United States) (Chen et al., 2012). The subsequent genomic DNA-purification steps were performed according to the manufacturer’s instructions.
16S rDNA Amplification and Sequencing
After DNA extraction, bacterial 16S rDNA was amplified. Briefly, the V3–V4 region of 16S rDNA was amplified using paired primers (357F: CCTACGGGNBGCASCAG/806R: GACTACNVGGGTATCTAATCC). The 16S rDNA gene was PCR-amplified using the KAPA HiFi HotStart PCR Kit (Kapa Biosystems, United States) in a 25-μL reaction volume containing 0.5 μL KAPA HiFi HotStart DNA Polymerase, 5 μL GC buffer, 0.5 μL deoxyribonucleoside triphosphates, 0.5 μM of each primer, 2 μL genomic DNA, and 16 μL double-distilled water. The reaction was held at 95°C for 3 min, followed by 25 cycles at 95°C for 1 min, 55°C for 30 s, and 72°C for 30 s, with a final elongation step at 72°C for 5 min in an ABI thermocycler (Applied Biosystems 2720, United States). Each PCR product was purified and amplified again to link with sample-specific barcodes (NEXTflexTM DNA PCR Master Mix, Bioo Scientific, United States). After quantification using an ND-1000 v3.3.0 spectrophotometer (NanoDrop, United States), a paired-end sequencing (2 × 125 bp) was performed on an Illumina HiSeq 2500 sequencer in two lanes at the Center for Molecular Immunology of Chinese Academy of Sciences (Beijing, China).
16S rDNA Sequence Analysis
The Illumina reads were sorted into different samples according to their barcoded index sequences. Fast Length Adjustment of SHort reads (FLASH) software was used to merge paired-end reads from the next-generation sequencing results (Magoc and Salzberg, 2011). Low-quality reads were filtered using the fastq_quality_filter (-p 90 -q 25 -Q33) in FASTX-Toolkit, v.0.0.14 (Caporaso et al., 2010) and chimera reads were removed with USEARCH 64 bit, v8.0.1517 (Edgar et al., 2011). The OTUs were aligned utilizing the UCLUST algorithm with a 97% identity and taxonomically classified using the SILVA database, v128 released on 29/09/2016 (Quast et al., 2013). Alpha and beta diversity were generated with the Quantitative Insights Into Microbial Ecology (QIIME) (Caporaso et al., 2010) pipeline and calculated based on weighted and unweighted Unifrac-distance matrices. The pivotal criterion to select core OTUs was an abundance higher than 10 reads in at least 1 sample. We used the linear discriminant analysis (LDA) effect size (LEfSe) method to identify species with statistically significant differential abundance among groups (Segata et al., 2011). We characterized species alpha diversity in the community by calculating the Shannon and Chao1 diversity indexes. A Venn diagram was drawn for analysis of group-specific bacterial microbiota. In addition, we analyzed bacterial beta diversity using partial-least squares discrimination analysis (PLS-DA) and non-metric multi-dimensional scaling (NMDS) (Caporaso et al., 2010). Additionally, the relative abundance of the various phyla, classes, orders, families, and genera in each sample was computed and compared among all groups.
Microbial Abundance-UC Severity Correlation in the Exploration Cohort
The Mayo Endoscopic Score (Lewis et al., 2008) was used to estimate the severity of UC patients. Based on the total Mayo Clinic score, UC patients were divided into four groups: inactive (Mayo score = 0, n = 0), mild (Mayo score = 1, n = 7), moderate (Mayo score = 2, n = 33), and severe (Mayo score = 3, n = 17). Linear regression was performed to analyze correlations between microbial abundance and UC severity.
Analysis of the Bacterial Interaction Patterns
To analyze bacterial-interaction patterns associated with different treatment strategies, pairwise bacterial abundance at the genus level was analyzed to determine correlations using Spearman’s method. Correlation coefficients were calculated using the pandas software package of Python, v.3.6.0. Gplots and pheatmap packages were launched in R 3.3.2 and Cytoscape 3.4.0, respectively, to visualize the patterns of microbial-interaction networks. Only significant correlations (p-value < 0.05 after false-discovery rate correlation) are shown.
Data Availability Statement
The sequences generated in the present study are available through the NCBI Sequence Read Archive (accession number SRP136321).
Statistical Analysis
GraphPad Prism, v.6.0c was used for data analysis and graph preparation. All data are expressed as the mean ± standard error of the mean. An analysis of variance (ANOVA) with Fisher’s least significant difference post hoc test was used for data analysis. Differences with a p-value < 0.05 were considered statistically significant.
Results
General Information on Recruited People
Fifty-seven UC patients, including untreated (n = 20) and 5-ASA treated (n = 37), were recruited for the exploration cohort. There was no significant difference in mean age between the untreated and 5-ASA treated groups (p = 0.796). The endoscopic mayo clinic score and Montreal classification of each patient were recorded (Table 1). The Mayo Endoscopic score in the untreated and 5-ASA treated groups was not significantly different (2.3 ± 0.6 vs. 2.1 ± 0.7, p = 0.516).
Ten UC patients were recruited for an independent validation study; the mean age was 33 ± 14 years, and four out of 10 patients were male. The mean therapy time was 6 months. Six patients were treated with 5-ASA for approximately 6 months, and four patients were treated for approximately 12 months. The total Mayo Endoscopic score of each patient was recorded before and after 5-ASA treatment. After 5-ASA treatment, the total mayo clinic score of UC patients was decreased significantly (9.2 ± 2.5 vs. 5.9 ± 1.8, p = 0.002) (Table 2).
Effect of 5-ASA on Bacterial Diversity in UC Patients
Different Traits of the Bacterial Diversity in Untreated and 5-ASA Treated UC Patients of the Exploration Cohort
The bacterial alpha diversity was analyzed using observed species, and Chao1 and Shannon’s indexes, and there was no significant difference between the Untreated/Non-inflamed and Untreated/Inflamed groups (Figure 1A). PLS-DA was carried out to estimate bacterial beta diversity. The bacterial microbiota clustered depending on non-inflamed and inflamed mucosae (Figure 1B).
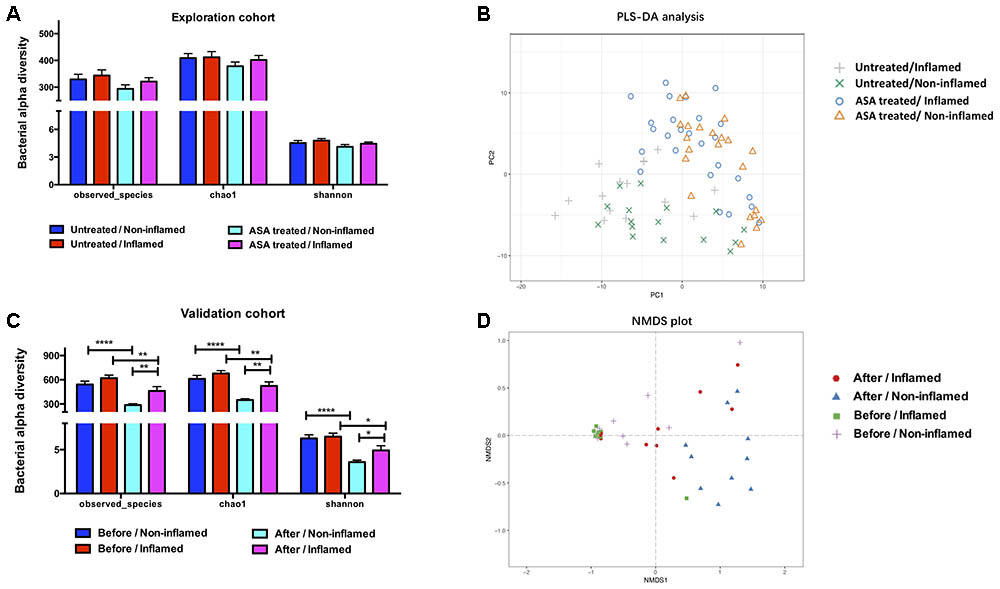
FIGURE 1. The bacterial diversity in the exploration and validation cohorts. (A) The bacterial alpha diversity in the exploration cohort. (B) Partial least-squares discrimination analysis (PLS-DA) of the bacterial beta diversity in exploration cohort. (C) The bacterial alpha diversity in the validation cohort. (D) Non-metric multidimensional scaling (NMDS) analysis of the bacterial beta diversity in the validation cohort. Untreated/Non-inflamed, non-inflamed mucosae of untreated UC patients; Untreated/Inflamed, inflamed mucosae of untreated UC patients; ASA-treated/Non-inflamed, non-inflamed mucosae of 5-ASA treated UC patients; ASA-treated/Inflamed, inflamed mucosae of 5-ASA treated UC patients. Before/Non-inflamed, non-inflamed mucosae before 5-ASA treatment; Before/Inflamed, inflamed mucosae before 5-ASA treatment; After/Non-inflamed, non-inflamed mucosae after 5-ASA treatment; After/Inflamed, inflamed mucosae after 5-ASA treatment.
We further analyzed the bacterial contents in the ASA-treated/Inflamed group. No significant difference in alpha diversity was found between the Untreated/Inflamed and ASA-treated/Inflamed groups (Figure 1A). Analyzing the bacterial beta diversity indicated that 5-ASA treatment drove separate clustering of all samples obtained from inflamed mucosae (Figure 1B).
5-ASA Treatment Altered the Bacterial Diversity of UC Patients in the Validation Cohort
Determining the Chao1 and Shannon indexes for the observed species revealed no statistical differences between the groups. Irrespective of whether the mucosae were non-inflamed or inflamed, 5-ASA treatment significantly decreased the bacterial alpha diversity. After 5-ASA treatment, however, higher alpha diversity was observed in After/Inflamed group compared with After/Non-inflamed group (Figure 1C).
In place of PLS-DA, NMDS analysis was performed to confirm the effect of 5-ASA treatment on bacterial beta diversity. We observed mucosal-type-dependent and treatment-dependent clustering in all mucosal samples (Figure 1D). These data indicated that 5-ASA treatment significantly altered the mucosal bacterial diversity.
Effect of 5-ASA on the Bacterial Composition in UC Patients
Different Traits of the Bacterial Composition in Untreated and 5-ASA Treated UC Patients of Exploration Cohort
Firmicutes, Bacteroidetes, and Proteobacteria phyla constituted the main part of the bacterial microbiota. These data were consistent with a previous study (Sokol et al., 2016). Of note, there were trends of abundant decrease in Firmicutes and increase in Proteobacteria in the Untreated/Inflamed group compared with the Untreated/Non-inflamed group (Figures 2A,B), although these differences were not significant. Additionally, at the genus level, most of the bacterial microbiota were comprised of Escherichia–Shigella, Bacteroides, and Faecalibacterium. Compared with the Untreated/Non-inflamed group, there were decreased trends in abundance of Enterococcus and Faecalibacterium, and increased trends in the abundance of Escherichia–Shigella and Prevotella_9 in the Untreated/Inflamed group (Figure 2C).
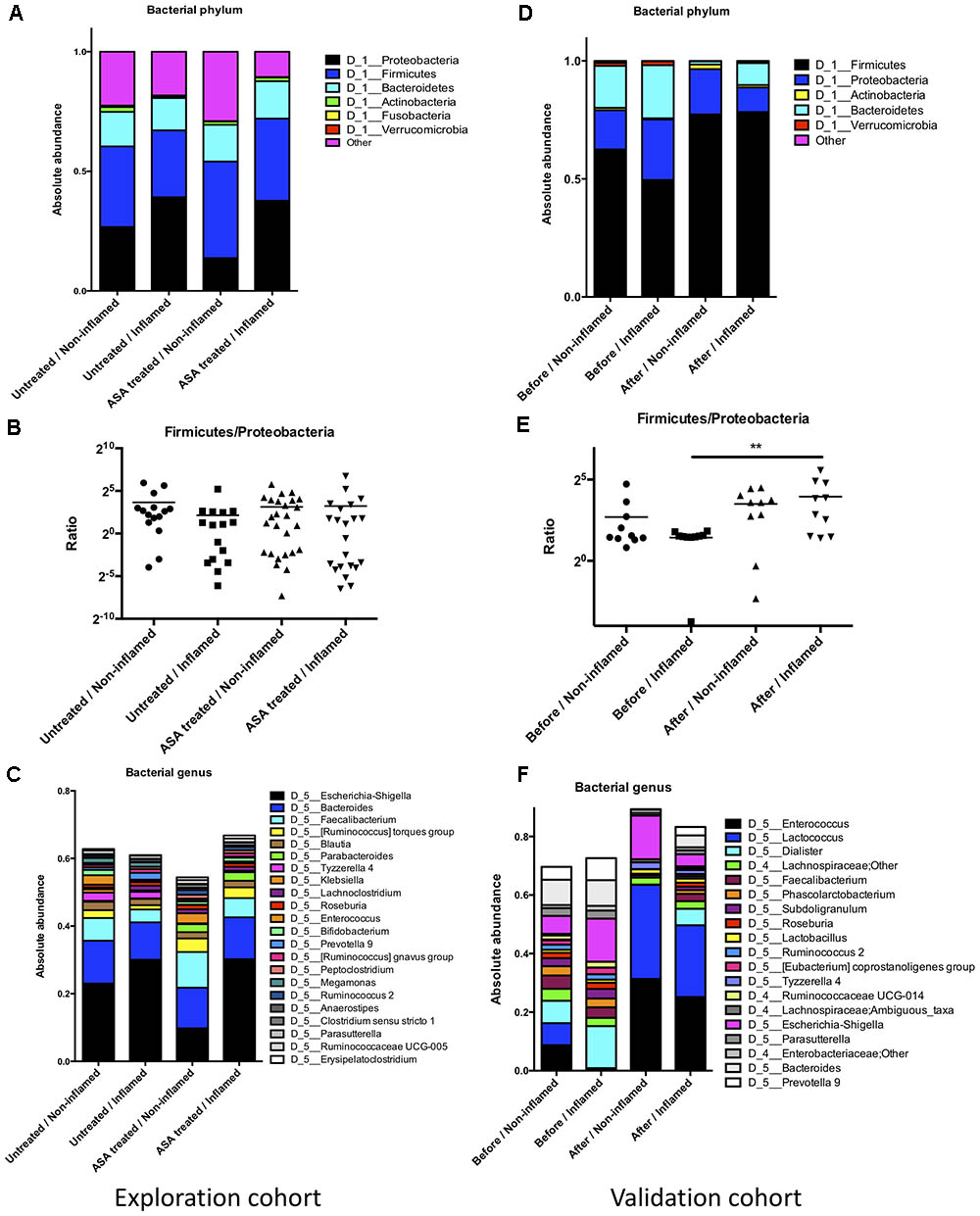
FIGURE 2. The bacterial composition in the exploration and validation cohorts. (A) The bacterial composition at the phylum level in the exploration cohort. (B) Firmicutes/Proteobacteria abundance ratio in the exploration cohort. (C) The bacterial composition at the genus level in the exploration cohort. (D) The bacterial composition at the phylum level in the validation cohort. (E) The bacterial composition at the phylum level in the validation cohort, ∗∗p ≤ 0.01. (F) Firmicutes/Proteobacteria abundance ratio in the validation cohort. Untreated/Non-inflamed, non-inflamed mucosae of untreated UC patients; Untreated/Inflamed, inflamed mucosae of untreated UC patients; ASA-treated/Non-inflamed, non-inflamed mucosae of 5-ASA treated UC patients; ASA-treated/Inflamed, inflamed mucosae of 5-ASA treated UC patients. Before/Non-inflamed, non-inflamed mucosae before 5-ASA treatment; Before/Inflamed, inflamed mucosae before 5-ASA treatment; After/Non-inflamed, non-inflamed.
Compared with the Untreated/Inflamed group, a lower abundance of Proteobacteria and a higher abundance of Firmicutes were noted in the ASA treated/Inflamed group at the phylum level, but these differences were not significant (Figures 2A,B). At the genus level, Faecalibacterium and Bifidobacterium were more abundant, and Escherichia–Shigella and Prevotella_9 were less abundant in the ASA treated/Inflamed group, compared with the Untreated/Inflamed group (Figure 2C).
5-ASA Treatment Altered the Bacterial Composition of UC Patients in the Validation Cohort
We further analyzed the bacterial composition in the validation cohort. At the phylum level, a lower abundance of Firmicutes and a higher abundance of Bacteroidetes and Proteobacteria were observed in the Before/Inflamed group compared with the Before/Non-inflamed group, although these differences were not statistically different. After 5-ASA treatment, the abundance of Firmicutes significantly increased, and the Bacteroidetes abundance significantly decreased in the inflamed mucosae (After/Inflamed vs. Before/Inflamed) (Figure 2D and Supplementary Table S2). In addition, the Firmicutes/Proteobacteria abundance ratio significantly increased in the inflamed mucosae (p = 0.004) (Figure 2F).
At the genus level, 19 bacterial genera were found with an average abundance of over 1%. These genera belonged to three phyla: Firmicutes (14), Proteobacteria (3), and Bacteroidetes (2) (Figure 2E). Thirty genera represented over 0.5% of the total and belonged to four phyla: Firmicutes (16), Proteobacteria (9), Bacteroidetes (4), and Verrucomicrobia (1) (Supplementary Table S3).
Before 5-ASA treatment, compared with the non-inflamed group, 4 bacterial genera (Prevotella_2, Prevotella_9, Dialister, and Klebsiella) significantly increased in the inflamed group. The abundance of some bacteria changed in the inflamed group without statistical significance. Trends toward alterations in these genera, such as Subdoligranulum, Roseburia, Eubacterium coprostanoligenes, and Escherichia-Shigella increased, and Enterococcus, Lactococcus, and Faecalibacterium decreased in inflamed mucosae (Supplementary Table S3).
Compared with the untreated groups, after 5-ASA treatment, the abundance of Enterococcus and Lactococcus increased significantly in both non-inflamed and inflamed mucosae. However, the abundance of several bacterial genera such as Bacteroides, Prevotella_9, Faecalibacterium, Phascolarctobacterium, Subdoligranulum, Roseburia, Ruminococcus_2, Eubacterium coprostanoligenes, Ruminococcaceae UCG-014, Ruminococcaceae_UCG_002, Dialister, Lachnospiraceae_NK4A136_group, Parasutterella, and Akkermansia significantly decreased after 5-ASA treatment (Supplementary Table S3). These data suggested that 5-ASA treatment altered the bacterial composition.
Based on these data, we primarily hypothesized that 5-ASA treatment affected the bacterial microbiota. To test this hypothesis, we subsequently performed a further confirmation study in the validation cohort.
5-ASA Treatment Altered the Representative Bacteria in the Validation Cohort
LEfSe analysis was performed to identify the differential bacteria composition. The difference in the bacterial microbiota between non-inflamed and inflamed mucosae was also explored using the Mann–Whitney U test at different taxon levels, including order, family and genus.
Before 5-ASA treatment, three representative bacterial genera were identified in the Before/Non-inflamed group and four representative genera were identified in the Before/Inflamed group. Tyzzerella_3, Atopobium, Klebsiella, and Dialister were enriched in the Before/Inflamed group (Figure 3A). To investigate the effect of 5-ASA treatment on mucosal microbiota, the differentiated taxa in the After/Non-inflamed and After/Inflamed groups were assessed. Forty representative genera were found in the After/Inflamed group, but no representative bacterium was found in the After/Non-inflamed group, at the genus level. Firmicutes members in the Clostridiales order (Christensenellaceae, Lachnospiraceae, and Ruminococcaceae) and Selenomonadales order (Veillonellaceae) were overrepresented in the After/Inflamed group. Among the Firmicutes, the Roseburia, Faecalibacterium, Ruminococcaceae UCG_002/003/004/009/010/013, Ruminococcus_2, and Lachnos piraceaeUCG_003 genera were associated with the After/Inflamed group (Figure 3B). These data indicated that Firmicutes were overrepresented in mucosae after 5-ASA treatment, especially in inflamed mucosae.
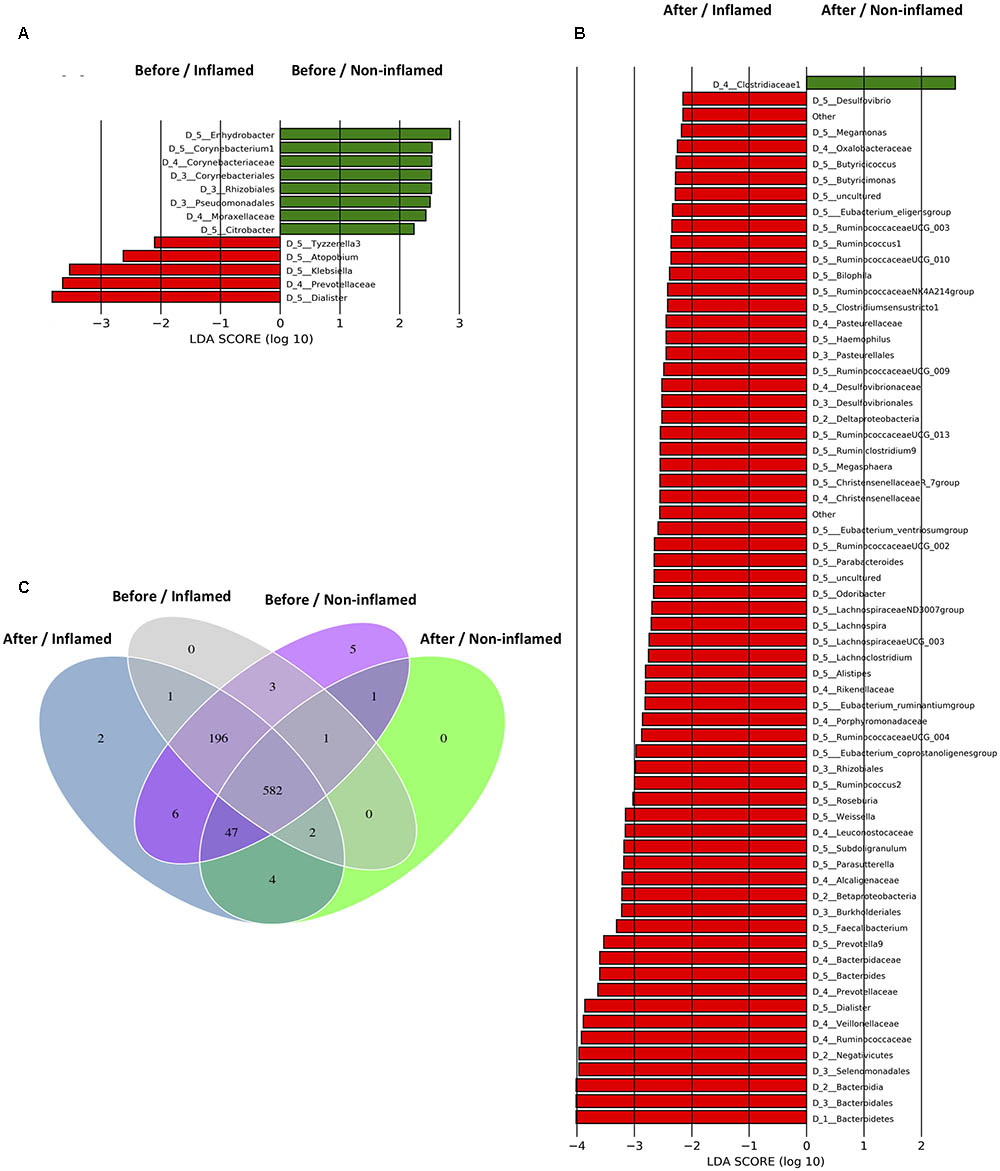
FIGURE 3. Taxonomic differences of bacterial microbiota and specific operational taxonomic units (OTUs) of samples in the validation cohort. (A) Bacterial taxa differentially abundant before 5-aminosalicylic acid (5-ASA) treatment. (B) Bacterial taxa differentially abundant after 5-ASA treatment. Differential-abundance microbial cladogram obtained by LEfSe. (C) Venn diagram representing the specific and shared bacterial microbiota. Before/Non-inflamed, non-inflamed mucosae before 5-ASA treatment; Before/Inflamed, inflamed mucosae before 5-ASA treatment; After/Non-inflamed, non-inflamed mucosae after 5-ASA treatment; After/Inflamed, inflamed mucosae after 5-ASA treatment.
Group-Specific Bacterial Microbiota in the Validation Cohort
To further investigate the effects of 5-ASA treatment on the bacterial microbiota, we analyzed group-specific OTUs before and after 5-ASA treatment. The group-specific OTUs were identified by matching data with the SILVA database. We identified 850 OTUs, 582 of which were shared by all four groups (Figure 3C).
Rhodanobacter (OTU420, Proteobacteria) uniquely existed in non-inflamed mucosae (Before/Non-inflamed and After/Non-inflamed groups), and OTU843 exclusively colonized inflamed mucosae (Before/Inflamed and After/Inflamed groups). Five specific OTUs were found in the Before/Non-inflamed group. Four of them were not identified in the SILVA database, although one OTU was identified in the database (Anaerosalibacter, a genus belonging to the Clostridiaceae family). Three OTUs, namely Phascolarctobacterium (OTU51, Firmicutes), Bacteroides (OTU146, Bacteroidetes), and OTU837, were shared by the Before/Non-inflamed and Before/Inflamed groups. No unique bacteria were detected in the Before/Inflamed and After/Non-inflamed groups. Several new OTUs were discovered after 5-ASA treatment. Ruminiclostridium (OTU164, Firmicutes) and OTU805 were found in the After/Inflamed group, and Clostridium sensu stricto 2 (OTU393, Firmicutes), Bacillus (OTU597, Firmicutes), Coprococcus 2 (OTU624, Firmicutes), and OTU821 were found in both the After/Non-inflamed and After/Inflamed groups (Supplementary Table S4).
These data suggested that more kinds of Firmicutes bacteria and less kinds of Proteobacteria and Bacteroidetes bacteria existed in the intestinal mucosae of UC patients after 5-ASA treatment.
5-ASA Treatment Altered the Bacterial Interaction Patterns in UC Patients
We further analyzed the effect of 5-ASA treatment on bacterial interaction patterns in non-inflamed and inflamed mucosae. Before 5-ASA treatment, the abundance of Lactococcus, Enterococcus, and Roseburia (belonging to Firmicutes) correlated negatively with Bacteroides and Propionibacterium in non-inflamed mucosae. Additionally, a low degree of bacterial correlation was found in the Before/Non-inflamed group. Notably, there was an extensive negative correlation of Escherichia–Shigella with Firmicutes (Ruminococcaceae, Faecalibacteria, Streptococcus, Lachnoclostridium, etc.) and Bacteroidetes (Prevotella, Alistipes, Bacteroides, and Parabacteroides) in the Before/Inflamed group. In addition, Bacteroides and Parabacteroides (belonging to the Bacteroidetes phylum) negatively correlated with Lactococcus and Enterococcus. These data indicated that increased Bacteroidetes and Proteobacteria abundance negatively correlated with the Firmicutes abundance in the Before/Inflamed group. These skewed bacterial correlations tended to be related to inflammatory responses in inflamed mucosae (Figure 4).
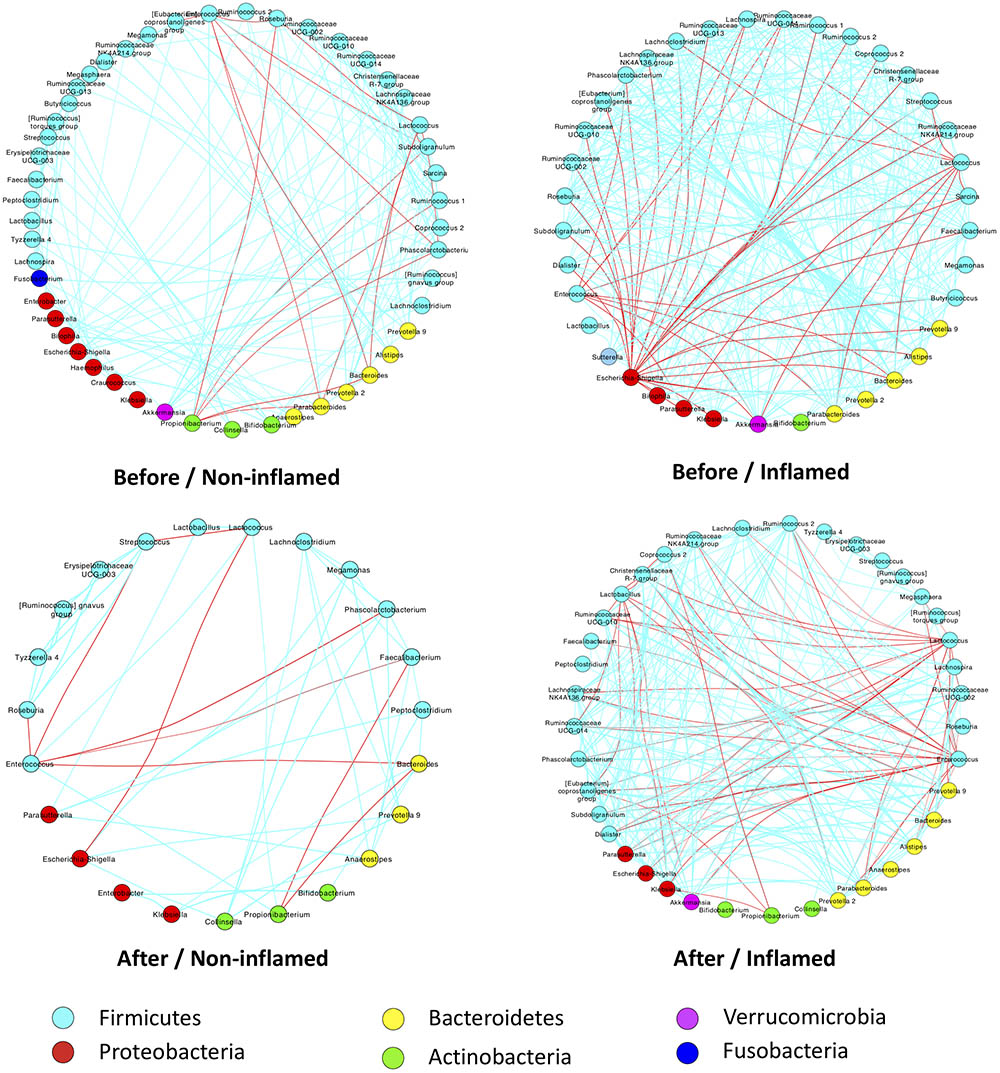
FIGURE 4. 5-ASA treatment altered bacterial-interaction patterns in UC patients of the validation cohort. Bacterial abundances were analyzed using Spearman’s test. Only significant correlations (p-value < 0.05) are displayed with an edge. The edge colors indicate positive (green) or negative (red) correlations, which depended on Spearman’s correlation coefficient. The nodes represent microbial genera – the colors of which represent bacterial phyla. Before/Non-inflamed, non-inflamed mucosae before 5-ASA treatment; Before/Inflamed, inflamed mucosae before 5-ASA treatment; After/Non-inflamed, non-inflamed mucosae after 5-ASA treatment; After/Inflamed, inflamed mucosae after 5-ASA treatment.
After 5-ASA treatment, a few bacterial correlations were observed in the After/Non-inflamed group. Compared with the Before/Inflamed group, fewer bacterial correlations were observed in the After/Inflamed group. Escherichia–Shigella did not extensively correlate with Firmicutes. However, the abundance of Lactococcus and Enterococcus increased and negatively correlated with Bacteroidetes (including Prevotella and Parabacteroides) and Proteobacteria (including Escherichia–Shigella, Klebsiella and Parasutterella). These data suggested that 5-ASA treatment alleviated the skewed negative correlation between Escherichia–Shigella and Firmicutes. In addition, increased Firmicutes exerted a suppressive effect on Bacteroidetes and Proteobacteria (Figure 4).
Relationship Between 5-ASA Efficiency and the Bacterial Microbiota
The Correlation Between UC Severity and Bacterial Abundance in the Exploration Cohort
We assumed that, irrespective of 5-ASA treatment, the abundance of some bacteria correlated with UC severity. To test this hypothesis, linear-regression analysis was performed to analyze the correlation between bacterial abundance and UC severity in the exploration cohort.
We found that bacterial abundance in non-inflamed mucosae poorly correlated with UC severity. In inflamed mucosae, the abundance of Firmicutes (R = 0.2603, p = 0.0549) negatively correlated with UC severity, and Proteobacteria (R = 0.2576, p = 0.0576) positively correlated with UC severity at the phylum level. Analysis at the genus level showed that the abundance of Faecalibacterium (R = 0.2873, p = 0.0334), Roseburia (R = 0.3416, p = 0.0107), and Bifidobacterium (R = 0.3536, p = 0.0081) negatively correlated with UC severity, while Enterobacteriaceae (R = 0.2873, p = 0.0179) and Escherichia–Shigella (R = 0.3175, p = 0.0182) positively correlated with UC severity (Figure 5A and Supplementary Table S5). These data indicated that the bacterial abundance might be a reliable factor for evaluating UC severity.
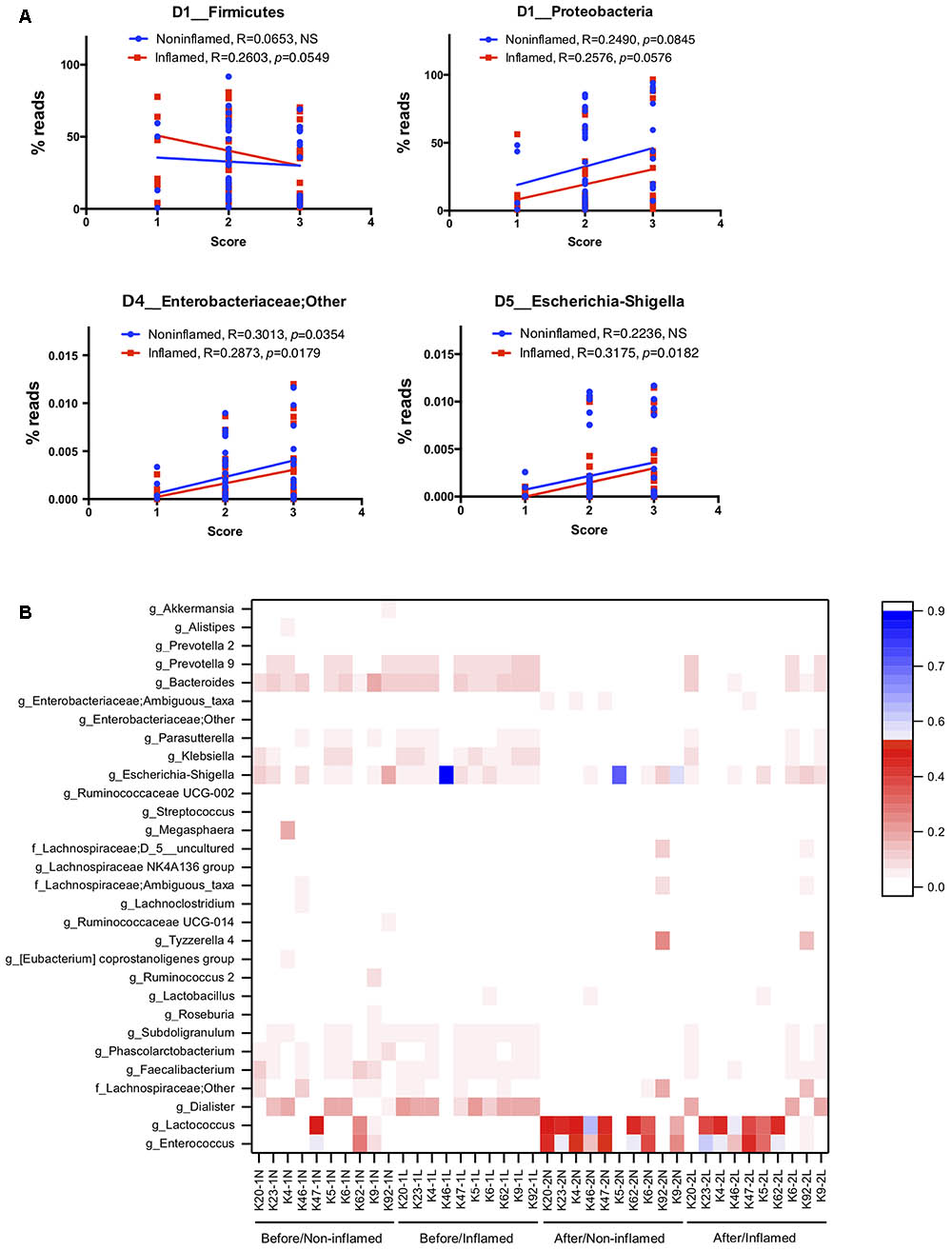
FIGURE 5. The relationship between 5-ASA treatment efficiency and bacterial microbiota. (A) The linear-regression analysis of the correlation between IBD severity and bacterial abundance at the phylum and genus levels in the exploration cohort. (B) Clustering heatmap of the bacterial abundance at the genus level in the validation cohort. Apart from unidentified OTUs, 50 bacterial genera of the highest abundance are displayed in this heatmap. Before/Non-inflamed (-1N), non-inflamed mucosae before 5-ASA treatment; Before/Inflamed (-1L), inflamed mucosae before 5-ASA treatment; After/Non-inflamed (-2N), non-inflamed mucosae after 5-ASA treatment; After/Inflamed (-2L), inflamed mucosae after 5-ASA treatment.
Bacterial Abundance Reflected the Efficiency of 5-ASA Treatment
To investigate the relationship between 5-ASA treatment efficiency and bacterial microbiota, we clustered samples by analyzing bacterial abundance at the genus level (Figure 5B). We found obvious clustering depending on 5-ASA treatment. Before 5-ASA treatment, bacteria such as Escherichia–Shigella, Dialister, Bacteroides, Prevotella_9, and Klebsiella colonized in the non-inflamed and inflamed mucosae with high abundance. The abundance of these bacteria decreased after 5-ASA treatment, and increase in Enterococcus, Lactococcus, and Lactobacillus was detected. Additionally, compared with the untreated group, several bacteria such as Faecalibacterium, Subdoligranulum, Parasutterella, Roseburia, Ruminococcus_2, Lachnoclostridium, Prevotella_2, Akkermansia, Phascolarctobacterium, and Ruminococcaceae UCG-014 decreased after 5-ASA treatment (Figure 5B).
In our exploration study, we found that UC severity positively correlated with the abundance of Escherichia–Shigella in inflamed mucosae. In the validation study, although the difference was not significant, we still found a decreased abundance of Escherichia–Shigella and other genera belonging to Enterobacteriaceae after 5-ASA treatment (Supplementary Table S5). These data indicated that bacterial abundance (e.g., Escherichia–Shigella) might reflect the efficiency of 5-ASA treatment.
Discussion
Compared with fecal microbiota (passersby), mucosal microbiota act as residents that can continuously activate the host immune system and induce chronic gut inflammation (Tang et al., 2015). To investigate the characteristics of the gut microbiota of UC patients undergoing 5-ASA treatment, we analyzed mucosal samples. Additionally, because of ethical limitations, we did not compare the mucosal bacterial microbiota of UC patients to healthy controls. Previous studies have reported microbial differences between non-inflamed and inflamed mucosae, and between remission and inflamed mucosae (Li et al., 2014; Liguori et al., 2016). Therefore, we compared the bacterial microbiota in inflamed and non-inflamed mucosae.
Gut microbiota is a complex biosystem affected by several factors. It has been reported that the location of the mucosal sampling sites influenced the microbial composition (Aguirre de Carcer et al., 2011; Gu et al., 2013; Costa et al., 2015; He et al., 2018). According to the Montreal classification, we enrolled approximately the same percentage of patients with each type to verify this effect. Furthermore, a previous study also reported a sex-based effect on mucosa-associated bacteria along the human colon (Aguirre de Carcer et al., 2011). We found that there was a significant difference in the sex percentage in our exploration cohort. To avoid the sex-based bias in our study, we further confirmed the results obtained from the exploration cohort within our validation cohort.
Bacterial dysbiosis is related to IBD development (Wlodarska et al., 2015; Imhann et al., 2016). In IBD patients, the bacterial diversity and the percentage of the Firmicutes phylum decreased and that of the Proteobacteria phylum increased (Ott et al., 2004; Liguori et al., 2016; Sokol et al., 2016; Takahashi et al., 2016). Our data were in agreement with these previous studies. Recently, some IgA-coated bacteria have been shown as dominant pathogens in IBD pathogenesis (Okai et al., 2017). Escherichia (belonging to the Proteobacteria phylum) is a genus of IgA-coated bacteria (Viladomiu et al., 2017). In addition, Escherichia coli (in particular, the AIEC pathotype) has been implicated in IBD pathogenesis (Nguyen et al., 2014). It has been reported that chronic infection with Escherichia induced cell cycle disorder, DNA damage, and inflammation. These processes play pivotal roles in IBD development (Khan et al., 2017; Viladomiu et al., 2017). Apart from Escherichia, a high abundance of Bacteroidetes was discovered in the inflamed mucosa of untreated UC patients, especially in the validation cohort. This finding suggests that the pathogenesis is related to Bacteroidetes species, such as Bacteroides, Prevotella_2, Prevotella_9, and Parabacteroides. Bacteroides fragilis has been reported to produce a toxin termed B. fragilis toxin that induced IBD and even colorectal cancer (Sears, 2009). Prevotella falsenii and Parabacteroides distasonis, two peptidoglycan recognition protein-regulated gut microbes, were also reported as aggravating colitis (Dziarski et al., 2016). In our exploration study, we also found that UC severity correlated with the Proteobacteria abundance, especially Enterobacteriaceae and Escherichia–Shigella in inflamed mucosa. We also assessed the bacterial microbiota in UC patients after 5-ASA treatment in our validation cohort. We found that it reduced the abundance of bacteria associated with inflammation after 5-ASA treatment, such as Escherichia–Shigella, Bacteroides, Prevotella_9, Prevotella_2, and Klebsiella. These data indicate that 5-ASA treatment might affect inflammation-associated bacteria colonizing the gut mucosa of UC patients.
Short-chain fatty acids (SCFAs), especially butyrate, provide up to 60% of the energy needed to colonic epithelium and gut immune cells, and play beneficial roles in anti-inflammation, anti-carcinogenesis, mucosal protection, and healing (Cook and Sellin, 1998; Cani et al., 2013; van der Beek et al., 2017). SCFAs levels are reduced in IBD patients, and this reduction is associated with a decrease in SCFAs-producing bacteria (Machiels et al., 2014). In Firmicutes, SCFA-producing bacteria include Blautia, Roseburia, Ruminococcus, Clostridium, Faecalibacterium, etc. In the exploration study, we found that the abundance of Roseburia, Ruminococcus, Clostridium, Faecalibacterium, and Dorea decreased in the inflamed mucosae of untreated UC patients. These data were in accord with those of previous studies (Machiels et al., 2014; Lopez-Siles et al., 2015, 2016). Additionally, we also showed that UC severity negatively correlated with bacteria such as Faecalibacterium, Roseburia, and Bifidobacterium in our exploration cohort. Although we could not perform a statistical analysis with our validation cohort because of the small sample size, these data were consistent with previous reports (Ott et al., 2004; Morgan et al., 2012; Sokol et al., 2016; Takahashi et al., 2016), and they validated the methods used in this study. Notably, increased colonization of Firmicutes, such as Ruminiclostridium, Clostridium sensu stricto 2, Coprococcus 2, and Bacillus, was found after 5-ASA treatment. The former three genera belong to the Clostridiales order and have been associated with SCFAs production (Spalinger et al., 2015). Bacillus clausii, a probiotic Bacillus spp., is utilized to treat small intestinal bacterial overgrowth (Gabrielli et al., 2009). These data suggest that the gut mucosa developed to a suitable state for colonization of some beneficial Firmicutes after 5-ASA treatment.
Sokol et al. (2017) reported a skewed microbial interaction pattern in IBD patients. He found that the concomitant analysis of microbiota showed a dense and homogenous correlation network in healthy subjects, but an unbalanced network in IBD patients (Sokol et al., 2017). In our validation cohort, the skewed negative correlation between Escherichia–Shigella and bacterial genera of Firmicutes was also found in the inflamed mucosae of UC patients before 5-ASA treatment. Many of the involved Firmicutes bacteria were SCFA-producing bacteria. It has been reported that the translocation of E. coli across epithelia was reduced by SCFAs, especially butyrate (Lewis et al., 2010; Byndloss et al., 2017). We inferred that the decrease in anti-inflammatory SCFA-producing bacteria, such as some Firmicutes bacteria, and the increase of pro-inflammatory Escherichia–Shigella represent two fundamental traits in the mucosal inflammation of UC patients. Thus, the skewed negative correlation between Escherichia–Shigella and bacterial genera of Firmicutes in inflamed mucosae may play a key role in gut inflammation in UC patients. After 5-ASA treatment, we found that the skewed interaction disappeared, which partly indicated a therapeutic effect of 5-ASA.
It is widely accepted that there is an interaction between host immunity and microbiota. The gut microbiota plays an instrumental role in the development and education of the host immune system early in life (Gensollen et al., 2016; Gomez de Aguero et al., 2016). Through its symbiotic relationship with immune cells, colonizing microbiota can stimulate host immunity to prevent pathogen invasion (Walker, 2014). The interaction between the immune system and microbiota is essential for the immune defense system of the host in a healthy state (Belkaid and Harrison, 2017). These reports highlighted a microbiota–immunity interaction. Nevertheless, interruption of host immunity–microbiota interactions plays a pivotal role in triggering inflammation in IBD, which is mediated by the host immune system (Du et al., 2015; Wlodarska et al., 2015; Kim et al., 2017; Kramer and Genco, 2017; Pickard et al., 2017); however, we are not clear as to whether inflammatory status affects gut microbiota. We observed in our study that there were parallel changes between the decrease in the Mayo Endoscopic score and bacterial alteration (e.g., decreased Escherichia–Shigella) of UC patients after 5-ASA treatment in our validation cohort. A previous study also indicated that the proposed anti-inflammatory and bacteriological effects of 5-ASA were well aligned with factors implicated in IBS pathogenesis (Andrews et al., 2011). We did not use sulfasalazine (SASP) in our research as its sulfonamide group might directly exert an antimicrobial effect. Unlike SASP, 5-ASA exerts its anti-inflammatory effect mainly by inhibiting TNF-α-regulated IκB degradation and NF-κB activation (Yan and Polk, 1999). Thus, we inferred that 5-ASA treatment might alter bacterial microbiota through regulating inflammatory status, although this needs further investigation.
In this study, we showed the microbial dysbiosis (mainly characterized by an abundant increase of Escherichia–Shigella) and the skewed negative correlation between Escherichia–Shigella and bacterial genera of Firmicutes in the inflamed mucosa of UC patients. In addition, we found that 5-ASA treatment altered the diversity, composition, and bacterial interaction patterns in mucosal samples of UC patients.
Ethics Statement
This study was carried out in accordance with the World Medical Association’s Declaration of Helsinki. The protocol was approved by the Institutional Medical Ethics Review Board of Peking University People’s Hospital (Document No. 2016PHB024-01). All subjects gave written informed consent in accordance with the Declaration of Helsinki.
Author Contributions
YL and JX designed the study. JX and NC performed acquisition of clinical data. JX, ZW, YS, NW, FZ, and XR performed analysis and interpretation of data. JX and YZ drew the figures. JX and YL wrote the manuscript. NC, ZW, and YS revised the manuscript for important intellectual content. NC and YL supervised the study.
Funding
This study was supported by Capital Characteristic Clinic Project (Z161100000516161) and National Natural Science Foundation of China (81670499).
Conflict of Interest Statement
The authors declare that the research was conducted in the absence of any commercial or financial relationships that could be construed as a potential conflict of interest.
Acknowledgments
The authors thank Zhu Baoli, Lv Na and Liu Fei from the Institute of Microbiology, Chinese Academy of Sciences for their warmhearted assistance in microbial genomics sequencing and analysis.
Supplementary Material
The Supplementary Material for this article can be found online at: https://www.frontiersin.org/articles/10.3389/fmicb.2018.01274/full#supplementary-material
References
Aguirre de Carcer, D., Cuiv, P. O., Wang, T., Kang, S., Worthley, D., Whitehall, V., et al. (2011). Numerical ecology validates a biogeographical distribution and gender-based effect on mucosa-associated bacteria along the human colon. ISME J. 5, 801–809. doi: 10.1038/ismej.2010.177
Andrews, C. N., Griffiths, T. A., Kaufman, J., Vergnolle, N., Surette, M. G., and Rioux, K. P. (2011). Mesalazine (5-aminosalicylic acid) alters faecal bacterial profiles, but not mucosal proteolytic activity in diarrhoea-predominant irritable bowel syndrome. Aliment. Pharmacol. Ther. 34, 374–383. doi: 10.1111/j.1365-2036.2011.04732.x
Barman, M., Unold, D., Shifley, K., Amir, E., Hung, K., Bos, N., et al. (2008). Enteric salmonellosis disrupts the microbial ecology of the murine gastrointestinal tract. Infect. Immun. 76, 907–915. doi: 10.1128/IAI.01432-07
Belkaid, Y., and Harrison, O. J. (2017). Homeostatic immunity and the microbiota. Immunity 46, 562–576. doi: 10.1016/j.immuni.2017.04.008
Bernstein, C. N., Eliakim, A., Fedail, S., Fried, M., Gearry, R., Goh, K. L., et al. (2016). World gastroenterology organisation global guidelines inflammatory bowel disease: update august 2015. J. Clin. Gastroenterol. 50, 803–818. doi: 10.1097/MCG.0000000000000660
Butto, L. F., and Haller, D. (2017). Dysbiosis in Crohn’s disease - Joint action of stochastic injuries and focal inflammation in the gut. Gut Microbes 8, 53–58. doi: 10.1080/19490976.2016.1270810
Byndloss, M. X., Olsan, E. E., Rivera-Chavez, F., Tiffany, C. R., Cevallos, S. A., Lokken, K. L., et al. (2017). Microbiota-activated PPAR-gamma signaling inhibits dysbiotic Enterobacteriaceae expansion. Science 357, 570–575. doi: 10.1126/science.aam9949
Cani, P. D., Everard, A., and Duparc, T. (2013). Gut microbiota, enteroendocrine functions and metabolism. Curr. Opin. Pharmacol. 13, 935–940. doi: 10.1016/j.coph.2013.09.008
Caporaso, J. G., Kuczynski, J., Stombaugh, J., Bittinger, K., Bushman, F. D., Costello, E. K., et al. (2010). QIIME allows analysis of high-throughput community sequencing data. Nat. Methods 7, 335–336. doi: 10.1038/nmeth.f.303
Chen, W., Liu, F., Ling, Z., Tong, X., and Xiang, C. (2012). Human intestinal lumen and mucosa-associated microbiota in patients with colorectal cancer. PLoS One 7:e39743. doi: 10.1371/journal.pone.0039743
Cook, S. I., and Sellin, J. H. (1998). Review article: short chain fatty acids in health and disease. Aliment. Pharmacol. Ther. 12, 499–507. doi: 10.1046/j.1365-2036.1998.00337.x
Costa, M. C., Silva, G., Ramos, R. V., Staempfli, H. R., Arroyo, L. G., Kim, P., et al. (2015). Characterization and comparison of the bacterial microbiota in different gastrointestinal tract compartments in horses. Vet. J. 205, 74–80. doi: 10.1016/j.tvjl.2015.03.018
Du, Z., Hudcovic, T., Mrazek, J., Kozakova, H., Srutkova, D., Schwarzer, M., et al. (2015). Development of gut inflammation in mice colonized with mucosa-associated bacteria from patients with ulcerative colitis. Gut Pathog. 7:32. doi: 10.1186/s13099-015-0080-2
Dziarski, R., Park, S. Y., Kashyap, D. R., Dowd, S. E., and Gupta, D. (2016). Pglyrp-Regulated Gut Microflora Prevotella falsenii, Parabacteroides distasonis and Bacteroides eggerthii enhance and Alistipes finegoldii attenuates colitis in mice. PLoS One 11:e0146162. doi: 10.1371/journal.pone.0146162
Edgar, R. C., Haas, B. J., Clemente, J. C., Quince, C., and Knight, R. (2011). UCHIME improves sensitivity and speed of chimera detection. Bioinformatics 27, 2194–2200. doi: 10.1093/bioinformatics/btr381
Gabrielli, M., Lauritano, E. C., Scarpellini, E., Lupascu, A., Ojetti, V., Gasbarrini, G., et al. (2009). Bacillus clausii as a treatment of small intestinal bacterial overgrowth. Am. J. Gastroenterol. 104, 1327–1328. doi: 10.1038/ajg.2009.91
Gensollen, T., Iyer, S. S., Kasper, D. L., and Blumberg, R. S. (2016). How colonization by microbiota in early life shapes the immune system. Science 352, 539–544. doi: 10.1126/science.aad9378
Gomez de Aguero, M., Ganal-Vonarburg, S. C., Fuhrer, T., Rupp, S., Uchimura, Y., Li, H., et al. (2016). The maternal microbiota drives early postnatal innate immune development. Science 351, 1296–1302. doi: 10.1126/science.aad2571
Gu, S., Chen, D., Zhang, J. N., Lv, X., Wang, K., Duan, L. P., et al. (2013). Bacterial community mapping of the mouse gastrointestinal tract. PLoS One 8:e74957. doi: 10.1371/journal.pone.0074957
He, J., Yi, L., Hai, L., Ming, L., Gao, W., and Ji, R. (2018). Characterizing the bacterial microbiota in different gastrointestinal tract segments of the Bactrian camel. Sci. Rep. 8:654. doi: 10.1038/s41598-017-18298-7
Hedin, C. R., van der Gast, C. J., Stagg, A. J., Lindsay, J. O., and Whelan, K. (2017). The gut microbiota of siblings offers insights into microbial pathogenesis of inflammatory bowel disease. Gut Microbes 8, 359–365. doi: 10.1080/19490976.2017.1284733
Imhann, F., Vich Vila, A., Bonder, M. J., Fu, J., Gevers, D., Visschedijk, M. C., et al. (2016). Interplay of host genetics and gut microbiota underlying the onset and clinical presentation of inflammatory bowel disease. Gut 67, 108–119. doi: 10.1136/gutjnl-2016-312135
Kamada, N., Kim, Y. G., Sham, H. P., Vallance, B. A., Puente, J. L., Martens, E. C., et al. (2012). Regulated virulence controls the ability of a pathogen to compete with the gut microbiota. Science 336, 1325–1329. doi: 10.1126/science.1222195
Kamada, N., Seo, S. U., Chen, G. Y., and Nunez, G. (2013). Role of the gut microbiota in immunity and inflammatory disease. Nat. Rev. Immunol. 13, 321–335. doi: 10.1038/nri3430
Khan, A. A., Khan, Z., Malik, A., Kalam, M. A., Cash, P., Ashraf, M. T., et al. (2017). Colorectal cancer-inflammatory bowel disease nexus and felony of Escherichia coli. Life Sci. 180, 60–67. doi: 10.1016/j.lfs.2017.05.016
Kim, D., Zeng, M. Y., and Nuìnþez, G. (2017). The interplay between host immune cells and gut microbiota in chronic inflammatory diseases. Exp. Mol. Med. 49:e339. doi: 10.1038/emm.2017.24
Kramer, C. D., and Genco, C. A. (2017). Microbiota, immune subversion, and chronic inflammation. Front. Immunol. 8:255. doi: 10.3389/fimmu.2017.00255
Laharie, D. (2017). Towards therapeutic choices in ulcerative colitis. Lancet 390, 98–99. doi: 10.1016/S0140-6736(17)31263-1
Lewis, J. D., Chuai, S., Nessel, L., Lichtenstein, G. R., Aberra, F. N., and Ellenberg, J. H. (2008). Use of the noninvasive components of the Mayo score to assess clinical response in ulcerative colitis. Inflamm. Bowel Dis. 14, 1660–1666. doi: 10.1002/ibd.20520
Lewis, K., Lutgendorff, F., Phan, V., Soderholm, J. D., Sherman, P. M., and McKay, D. M. (2010). Enhanced translocation of bacteria across metabolically stressed epithelia is reduced by butyrate. Inflamm. Bowel Dis. 16, 1138–1148. doi: 10.1002/ibd.21177
Li, Q., Wang, C., Tang, C., He, Q., Li, N., and Li, J. (2014). Dysbiosis of gut fungal microbiota is associated with mucosal inflammation in Crohn’s disease. J. Clin. Gastroenterol. 48, 513–523.
Liguori, G., Lamas, B., Richard, M. L., Brandi, G., da Costa, G., Hoffmann, T. W., et al. (2016). Fungal dysbiosis in mucosa-associated microbiota of Crohn’s disease patients. J. Crohns Colitis 10, 296–305. doi: 10.1093/ecco-jcc/jjv209
Lopez-Siles, M., Martinez-Medina, M., Abella, C., Busquets, D., Sabat-Mir, M., Duncan, S. H., et al. (2015). Mucosa-associated Faecalibacterium prausnitzii phylotype richness is reduced in patients with inflammatory bowel disease. Appl. Environ. Microbiol. 81, 7582–7592. doi: 10.1128/AEM.02006-15
Lopez-Siles, M., Martinez-Medina, M., Suris-Valls, R., Aldeguer, X., Sabat-Mir, M., Duncan, S. H., et al. (2016). Changes in the abundance of Faecalibacterium prausnitzii Phylogroups I and II in the intestinal mucosa of inflammatory bowel disease and patients with colorectal cancer. Inflamm. Bowel Dis. 22, 28–41. doi: 10.1097/MIB.0000000000000590
Machiels, K., Joossens, M., Sabino, J., De Preter, V., Arijs, I., Eeckhaut, V., et al. (2014). A decrease of the butyrate-producing species Roseburia hominis and Faecalibacterium prausnitzii defines dysbiosis in patients with ulcerative colitis. Gut 63, 1275–1283. doi: 10.1136/gutjnl-2013-304833
Magoc, T., and Salzberg, S. L. (2011). FLASH: fast length adjustment of short reads to improve genome assemblies. Bioinformatics 27, 2957–2963. doi: 10.1093/bioinformatics/btr507
Morgan, X. C., Tickle, T. L., Sokol, H., Gevers, D., Devaney, K. L., Ward, D. V., et al. (2012). Dysfunction of the intestinal microbiome in inflammatory bowel disease and treatment. Genome Biol. 13:R79. doi: 10.1186/gb-2012-13-9-r79
Nguyen, H. T., Dalmasso, G., Muller, S., Carriere, J., Seibold, F., and Darfeuille-Michaud, A. (2014). Crohn’s disease-associated adherent invasive Escherichia coli modulate levels of microRNAs in intestinal epithelial cells to reduce autophagy. Gastroenterology 146, 508–519. doi: 10.1053/j.gastro.2013.10.021
Norman, J. M., Handley, S. A., Baldridge, M. T., Droit, L., Liu, C. Y., Keller, B. C., et al. (2015). Disease-specific alterations in the enteric virome in inflammatory bowel disease. Cell 160, 447–460. doi: 10.1016/j.cell.2015.01.002
Okai, S., Usui, F., Ohta, M., Mori, H., Kurokawa, K., Matsumoto, S., et al. (2017). Intestinal IgA as a modulator of the gut microbiota. Gut Microbes 8, 486–492. doi: 10.1080/19490976.2017.1310357
Ott, S. J., Musfeldt, M., Wenderoth, D. F., Hampe, J., Brant, O., Folsch, U. R., et al. (2004). Reduction in diversity of the colonic mucosa associated bacterial microflora in patients with active inflammatory bowel disease. Gut 53, 685–693. doi: 10.1136/gut.2003.025403
Pascal, V., Pozuelo, M., Borruel, N., Casellas, F., Campos, D., Santiago, A., et al. (2017). A microbial signature for Crohn’s disease. Gut 66, 813–822. doi: 10.1136/gutjnl-2016-313235
Pickard, J. M., Zeng, M. Y., Caruso, R., and Nunez, G. (2017). Gut microbiota: role in pathogen colonization, immune responses, and inflammatory disease. Immunol. Rev. 279, 70–89. doi: 10.1111/imr.12567
Quast, C., Pruesse, E., Yilmaz, P., Gerken, J., Schweer, T., Yarza, P., et al. (2013). The SILVA ribosomal RNA gene database project: improved data processing and web-based tools. Nucleic Acids Res. 41, D590–D596. doi: 10.1093/nar/gks1219
Rooks, M. G., and Garrett, W. S. (2016). Gut microbiota, metabolites and host immunity. Nat. Rev. Immunol. 16, 341–352. doi: 10.1038/nri.2016.42
Sartor, R. B., and Wu, G. D. (2017). Roles for intestinal bacteria, viruses, and fungi in pathogenesis of inflammatory bowel diseases and therapeutic approaches. Gastroenterology 152, 327.e–339.e. doi: 10.1053/j.gastro.2016.10.012
Sears, C. L. (2009). Enterotoxigenic Bacteroides fragilis: a rogue among symbiotes. Clin. Microbiol. Rev. 22, 349–369. doi: 10.1128/CMR.00053-08
Segata, N., Izard, J., Waldron, L., Gevers, D., Miropolsky, L., Garrett, W. S., et al. (2011). Metagenomic biomarker discovery and explanation. Genome Biol. 12:R60. doi: 10.1186/gb-2011-12-6-r60
Sokol, H., Leducq, V., Aschard, H., Pham, H. P., Jegou, S., Landman, C., et al. (2016). Fungal microbiota dysbiosis in IBD. Gut 66, 1039–1048. doi: 10.1136/gutjnl-2015-310746
Sokol, H., Leducq, V., Aschard, H., Pham, H. P., Jegou, S., Landman, C., et al. (2017). Fungal microbiota dysbiosis in IBD. Gut 66, 1039–1048. doi: 10.1136/gutjnl-2015-310746
Spalinger, M. R., Kasper, S., Chassard, C., Raselli, T., Frey-Wagner, I., Gottier, C., et al. (2015). PTPN2 controls differentiation of CD4+ T cells and limits intestinal inflammation and intestinal dysbiosis. Mucosal Immunol. 8, 918–929. doi: 10.1038/mi.2014.122
Takahashi, K., Nishida, A., Fujimoto, T., Fujii, M., Shioya, M., Imaeda, H., et al. (2016). Reduced abundance of butyrate-producing bacteria species in the fecal microbial community in Crohn’s disease. Digestion 93, 59–65. doi: 10.1159/000441768
Tang, M. S., Poles, J., Leung, J. M., Wolff, M. J., Davenport, M., Lee, S. C., et al. (2015). Inferred metagenomic comparison of mucosal and fecal microbiota from individuals undergoing routine screening colonoscopy reveals similar differences observed during active inflammation. Gut Microbes 6, 48–56. doi: 10.1080/19490976.2014.1000080
Ungaro, R., Mehandru, S., Allen, P. B., Peyrin-Biroulet, L., and Colombel, J. F. (2017). Ulcerative colitis. Lancet 389, 1756–1770. doi: 10.1016/S0140-6736(16)32126-2
van der Beek, C. M., Dejong, C. H. C., Troost, F. J., Masclee, A. A. M., and Lenaerts, K. (2017). Role of short-chain fatty acids in colonic inflammation, carcinogenesis, and mucosal protection and healing. Nutr. Rev. 75, 286–305. doi: 10.1093/nutrit/nuw067
Viladomiu, M., Kivolowitz, C., Abdulhamid, A., Dogan, B., Victorio, D., Castellanos, J. G., et al. (2017). IgA-coated E. coli enriched in Crohn’s disease spondyloarthritis promote TH17-dependent inflammation. Sci. Transl. Med. 9:eaaf9655. doi: 10.1126/scitranslmed.aaf9655
Walker, A. (2014). Intestinal colonization and programming of the intestinal immune response. J. Clin. Gastroenterol. 48(Suppl. 1), S8–S11. doi: 10.1097/MCG.0000000000000230
Wlodarska, M., Kostic, A. D., and Xavier, R. J. (2015). An integrative view of microbiome-host interactions in inflammatory bowel diseases. Cell Host Microbe 17, 577–591. doi: 10.1016/j.chom.2015.04.008
Yan, F., and Polk, D. B. (1999). Aminosalicylic acid inhibits IkappaB kinase alpha phosphorylation of IkappaBalpha in mouse intestinal epithelial cells. J. Biol. Chem. 274, 36631–36636. doi: 10.1074/jbc.274.51.36631
Keywords: ulcerative colitis, 5-aminosalicylic acid, mucosal microbiota, bacterial dysbiosis, bacterial correlation
Citation: Xu J, Chen N, Wu Z, Song Y, Zhang Y, Wu N, Zhang F, Ren X and Liu Y (2018) 5-Aminosalicylic Acid Alters the Gut Bacterial Microbiota in Patients With Ulcerative Colitis. Front. Microbiol. 9:1274. doi: 10.3389/fmicb.2018.01274
Received: 23 March 2018; Accepted: 24 May 2018;
Published: 13 June 2018.
Edited by:
Benoit Chassaing, Georgia State University, United StatesReviewed by:
Bruno Bonaz, Centre Hospitalier Universitaire de Grenoble, FranceYuji Naito, Kyoto Prefectural University of Medicine, Japan
Simon Andrew Hirota, University of Calgary, Canada
Copyright © 2018 Xu, Chen, Wu, Song, Zhang, Wu, Zhang, Ren and Liu. This is an open-access article distributed under the terms of the Creative Commons Attribution License (CC BY). The use, distribution or reproduction in other forums is permitted, provided the original author(s) and the copyright owner are credited and that the original publication in this journal is cited, in accordance with accepted academic practice. No use, distribution or reproduction is permitted which does not comply with these terms.
*Correspondence: Yulan Liu, liuyulan@pkuph.edu.cn
†These authors have contributed equally to this work.