- 1Department of Environmental Systems Science, Institute of Biogeochemistry and Pollutant Dynamics, ETH Zurich - Swiss Federal Institute of Technology, Zurich, Switzerland
- 2Department of Surface Waters ‐ Research and Management, Eawag ‐ Swiss Federal Institute of Aquatic Science and Technology, Kastanienbaum, Switzerland
- 3Department of Biology, Institute of Molecular Health Sciences, ETH Zurich - Swiss Federal Institute of Technology, Zurich, Switzerland
In stratified lakes, methane-oxidizing bacteria (MOB) are strongly mitigating methane fluxes to the atmosphere by consuming methane entering the water column from the sediments. MOB communities in lakes are diverse and vertically structured, but their spatio-temporal dynamics along the water column as well as physico-chemical parameters and interactions with other bacterial species that drive the community assembly have so far not been explored in depth. Here, we present a detailed investigation of the MOB and bacterial community composition and a large set of physico-chemical parameters in a shallow, seasonally stratified, and sub-alpine lake. Four highly resolved vertical profiles were sampled in three different years and during various stages of development of the stratified water column. Non-randomly assembled MOB communities were detected in all compartments. We could identify methane and oxygen gradients and physico-chemical parameters like pH, light, available copper and iron, and total dissolved nitrogen as important drivers of the MOB community structure. In addition, MOB were well-integrated into a bacterial-environmental network. Partial redundancy analysis of the relevance network of physico-chemical variables and bacteria explained up to 84% of the MOB abundances. Spatio-temporal MOB community changes were 51% congruent with shifts in the total bacterial community and 22% of variance in MOB abundances could be explained exclusively by the bacterial community composition. Our results show that microbial interactions may play an important role in structuring the MOB community along the depth gradient of stratified lakes.
Introduction
Atmospheric concentrations of the potent greenhouse gas methane have steadily increased since the pre-industrial era from 722 to 1874 ppb.1 Freshwater lakes are significant natural methane sources, responsible for about 70% of the freshwater methane emissions (DelSontro et al., 2018; Sanches et al., 2019). Methane is primarily generated in their sediments by methanogenesis, but to a smaller extent, methane production can also occur in the oxic epilimnion of lakes via different suggested pathways (Grossart et al., 2011; Bogard et al., 2014; Tang et al., 2016; Bižić-Ionescu et al., 2018; Günthel et al., 2019). The magnitude of the methane fluxes to the atmosphere is under strong control of anaerobic and aerobic methane oxidation (Chistoserdova, 2011; Nordi et al., 2013; Graf et al., 2018; Martinez-Cruz et al., 2018). Aerobic methane-oxidizing bacteria (MOB) oxidize up to 90% of methane within the water column of freshwater systems (Bastviken et al., 2003, 2008). They mainly belong to Gammaproteobacteria and Alphaproteobacteria and are often referred to as type I and type II MOB, respectively (Hanson and Hanson, 1996; Chistoserdova and Lidstrom, 2013; Knief, 2015).
Freshwater lakes represent complex spatially and temporally structured environments that determine the composition of MOB assemblages and their activity in numerous ways. Temperate lakes usually develop a thermal stratification during summer months, under which anoxia can develop in the bottom waters allowing large amounts of methane to accumulate (Schubert et al., 2012). During this phase, the highest MOB activity can be detected at the bottom part of the oxycline, where methane and oxygen counter gradients meet (Bastviken et al., 2002; Sundh et al., 2005; Borrel et al., 2011). Such methane oxidation zones migrate within the water column as stratification progresses (Carini et al., 2005). Competitiveness under specific oxygen, methane, copper and iron concentrations can be a factor leading to MOB niche differentiation (Knief, 2015; Chidambarampadmavathy et al., 2017; Guggenheim et al., 2019; Mayr et al., 2019; Reis et al., 2020). This is thought to be partially coupled to the composition and property of the expressed methane monooxygenase (MMO), which initiates the methane oxidation process and exists in two main forms (Sirajuddin and Rosenzweig, 2015). The particulate MMO (pMMO) is a copper-dependent enzyme with high methane affinity but slower turnover rate (Lee et al., 2006; Ross et al., 2019). It appears to be almost ubiquitous among MOB (Knief, 2015). The soluble MMO (sMMO) uses iron in its active center and is only found in some MOB (Merkx et al., 2001; Tinberg and Lippard, 2011). In addition to the mentioned parameters, other physico-chemical variables can shape the MOB abundance, their community structure and ecosystem function. For instance, low water temperature and nitrogen-rich conditions favor the growth of type I over type II MOB (Tsutsumi et al., 2011; He et al., 2012a; Siljanen et al., 2012). Furthermore, lanthanides have been shown to be essential in the methane metabolism by MOB (Pol et al., 2014; Picone and Op den Camp, 2019). The so-called “lanthanide switch” induces the upregulation of the more efficient methanol dehydrogenase (MDH), which oxidizes methanol produced by MMO and may have a significant effect on MOB activity and their community composition (Vu et al., 2016; Yu and Chistoserdova, 2017; Chistoserdova, 2019). Methane oxidation by MOB under anoxic conditions has recently been shown to be important in stratified lakes (Deutzmann et al., 2014; Oswald et al., 2016a,b; Graf et al., 2018; van Grinsven et al., 2020). The relative importance of anaerobic to aerobic methane oxidation rates can differ due to given physico-chemical environmental conditions, such as the availabilities of nitrate, nitrite, sulfate, manganese, or iron within different depth zones (Oswald et al., 2016b; Roland et al., 2018; van Grinsven et al., 2020).
Apart from physico-chemical parameters, co-occurring organisms can also affect the MOB community composition directly or indirectly (Stein, 2020). Heterotrophic richness has been shown to enhance methane oxidation activity by MOB (Ho et al., 2014), as accompanying organisms can either remove inhibiting substances (e.g., methanol) or provide stimulating factors (e.g., essential nutrients such as cobalamin; Stock et al., 2013; Iguchi et al., 2015; Veraart et al., 2018; Singh et al., 2019). On the other hand, MOB also select for certain heterotrophs by providing organic metabolites (e.g., acetate) or by removing toxic compounds (e.g., formaldehyde; Morris et al., 2013; van der Ha et al., 2013; Oshkin et al., 2015; Gilman et al., 2017; Xing et al., 2018). Indeed, MOB play an integral role in transferring methane-derived carbon and other metabolites to the microbial pool and higher trophic levels of the food web (Jones and Grey, 2011; Sanseverino et al., 2012; Agasild et al., 2014). There is thus considerable evidence that ecological interactions can be important drivers in shaping the MOB community composition, but this hypothesis has so far not really been studied in the context of environmental data.
In this study, we investigated the combined effects of microbial communities and the physico-chemical environment on MOB community assemblies in order to improve our understanding of MOB-based ecosystem function (i.e., methane removal) under varying conditions. As the short literature review above has demonstrated, the potential drivers of MOB diversity and abundance are highly complex. Yet, few studies have so far been conducted that tried to systematically identify important drivers among the large set of potential factors. Over several years, we assembled a unique dataset of vertical profiles of the total bacterial and MOB community and a large set of physico-chemical variables in a eutrophic, seasonally stratified, and sub-alpine lake (Rotsee). Statistical analysis was used to link spatio-temporal fluctuations of physico-chemical variables and bacterial community composition to MOB abundance data with the goal of identifying key drivers of the MOB community structure. In particular, we aimed to determine the extent to which bacterial interactions vs. physico-chemical drivers determine the MOB community structure.
Materials and Methods
Site Description, in-situ Profiling, Sample Collection, and Analysis
Rotsee is a small (0.5 km2), eutrophic lake located in central Switzerland with a maximum depth of 16 m. Its wind-shielded location allows stable stratification from spring until mid to late autumn with an oxycline usually developed between 6 and 9 m depth. During stratification methane accumulates in the anoxic water column and reaches concentrations up to 1 mM (Schubert et al., 2010; Oswald et al., 2015). Sample collection was conducted close to the deepest point of the lake during 3 consecutive years at the beginning of stratification (June 2013), during peak stratification (August 2013), and shortly before the lake overturns (September 2014 and September 2015). Detailed methods of physico-chemical profiling, sampling, and analysis are reported in the Supplementary Material. Our physicochemical dataset includes the following parameters: conductivity (Cond), turbidity (Turb), depth (pressure), temperature (T), pH, photosynthetically active radiation (PAR, herein equated as light), concentrations of oxygen (O2), chlorophyll a (Chl-a), total sulphide (STot = H2S, HS−, S2−), dissolved organic carbon (DOC), total dissolved nitrogen (TDN), dissolved inorganic carbon (DIC), nitrite (NO2), nitrate (NO3), ammonium (NH4), sulphate (SO4), phosphate (PO4), dissolved (MDiss) and total (MTot) metal concentrations [copper (Cu), iron (Fe), manganese (Mn), zinc (Zn), chromium (Cr)], methane (CH4) as well as 13C/12C isotopic ratio of CH4 (δ13C-CH4). Particulate metal (MPart) concentrations were obtained by subtracting dissolved metal from total metal concentrations. Bioavailable metal fractions (MDGT) were measured via the Diffusive Gradients in Thin film (DGT) technique (Davison, 2016). Detailed information on the retrieval of the DGT data can be found in (Guggenheim et al., 2019).
DNA Sampling and Extraction, pmoA qPCR, Library Preparation, and Sequencing
Bacterial DNA was obtained by filtration of water samples and extraction from 0.2 μm polycarbonate filters using the PowerWater® DNA Isolation Kit (MoBio Laboratories, Carlsbad, CA, USA). Further details on DNA sampling and processing are reported in the Supplementary Material. The 16S rRNA and pmoA genes served as marker genes for the total bacterial community and MOB detection, respectively (Dumont, 2014). Quantitative polymerase chain reaction (qPCR) on the pmoA gene was conducted using the primer pair A189f and mb661r (5′-GGNGACTGGGACTTCTGG-3′, 5′-CCGGMGCAACGTCYTTACC-3′, Eurofins Genomics, Ebersberg, Germany), which covers most alphaproteobacterial and gammaproteobacterial MOB (Costello and Lidstrom, 1999). Although this primer set is specific for pmoA, it disfavors alphaproteobacterial MOB (Bourne et al., 2001). Verrucomicrobial pmoA sequences and sequences of NC10 phylum were not covered by the applied primer pair. pmoA amplicon libraries were prepared using the above-mentioned primers with Illumina Nextera overhang sequences at the 5′-end (Microsynth AG, Balgach, Switzerland). Amplicons were purified using AMPure XP beads (BeckmanCoulter Inc., Fullerton, CA, USA). Indexing and sequencing (MiSeq platform, Illumina Inc., San Diego, CA, USA) were conducted by the Genomics Facility Basel (Basel, Switzerland). Library preparation and Illumina sequencing of 16S rRNA genes were performed by Microsynth AG (Balgach, Switzerland). 16S rRNA genes were amplified using the primers S-D-Bact-0341-b-S-17 (5′-CCTACGGGNGGCWGCAG-3′, 341F) and S-D-Bact-0785-a-A-21 (5′-GACTACHVGGGTATCTAATCC-3′, 805R; Herlemann et al., 2011). Anaerobic methanotrophic archaea (ANME) or related archaea were excluded from the analysis as previous research showed they are of minor importance in the Rotsee water column (Oswald et al., 2015).
Sequence Processing, Phylogenetic Analysis, and Data Deposition
16S rRNA gene and pmoA sequencing data were analyzed by the Genomic Diversity Centre (GDC, Zurich, Switzerland). Raw data were quality controlled using FastQC (v0.11.4) and MultiQC (v0.7). 16S rRNA gene low quality ends of reads were trimmed with PRINSEQ-lite (v0.20.4) and merged using usearch (v8.1.1812_i86linux64). pmoA reads were trimmed and merged using usearch (v9.2.64_i86linux 64) and FLASH (v1.2.11), respectively. Merged reads were primer-site trimmed by cutadapt v1.5 and v1.12, respectively. PRINSEQ-lite (v0.20.4) was used to filter and size-select the amplicons. Sequences were clustered into operational taxonomic units (OTUs) at a 97% similarity cut-off level using the uparse (16S rRNA gene) and an 86% similarity cut-off level using the unoise (pmoA) workflow with usearch (Wen et al., 2016). Taxonomic assignment of the representative sequences was set using the UTAX classifier together with the GreenGene database (May 2013) for 16S rRNA gene, and the SINTAX classifier with the pmoA database from (Wen et al., 2016). All sequence data have been deposited at the ENA database under the accession numbers PRJEB28460 (16S rRNA gene) and PRJEB28505 (pmoA).
Depth Zones and Statistical Analysis
As a basis for ecological interpretation and statistical analysis, we divided the water column into four zones with contrasting environmental conditions (Figure 1). The “oxic” zone ranged from the surface to depths where O2 started to decrease and where CH4 remained stable at low concentrations, but epilimnetic CH4 sources might be present (Hofmann et al., 2010; Donis et al., 2017). This zone was followed by the “oxycline” zone, where O2 was present but declined with depth, while CH4 remained low. The lower boundary of the oxycline zone ended where CH4 accumulation began. Below the oxycline, O2 was below detection, but in Rotsee in-situ photosynthetic O2 production still provides oxygen (Oswald et al., 2015). The “oxidation zone,” in which CH4 was mainly consumed, was thus the zone from where CH4 began to increase and either O2 was present at measurable concentrations or light levels (PAR) allowed for photosynthesis. Therefore, this zone ended where PAR fell below detection limit. The dark bottom water was defined as the “anoxic” zone, where O2 for aerobic CH4 oxidation was lacking.
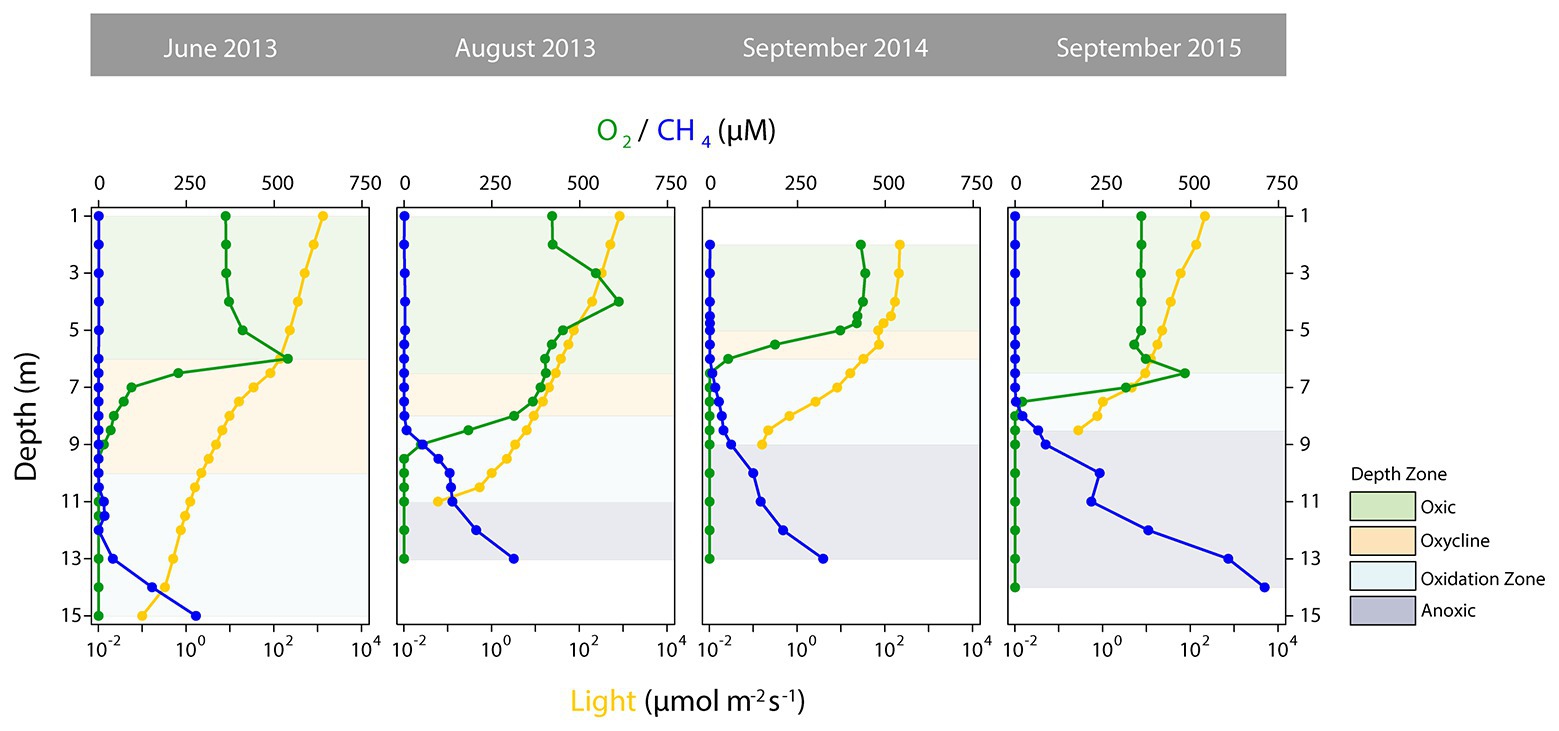
Figure 1. Depth profiles of selected physico-chemical parameters distinguishing the depth zones in Rotsee. Oxygen (O2) concentrations are shown in green, methane (CH4) concentrations in blue and photosynthetically active radiation (light, logarithmic scale) is depicted in dark yellow. Green shaded areas denote the oxic zones, yellow the oxyclines, blue the oxidation zones, and dark blue the anoxic zones.
Statistical analysis was performed within the R statistical software environment (version 3.4.3; R Core Team, 2020) using the vegan, phyloseq, mixOmics, EcoSimR, igraph, and picante packages (Csárdi and Nepusz, 2006; Kembel et al., 2010; McMurdie and Holmes, 2013; Gotelli et al., 2015; Rohart et al., 2017; Oksanen et al., 2018). 16S rRNA gene (bacterial community) and pmoA (MOB) reads occurring less than 3 or 50 times in at least three samples, respectively, were removed from the OTU tables. To compare relative OTU abundances, reads were standardized to the mean sequencing depth. 16S rRNA gene data assigned to MOB species was excluded in statistical models incorporating the pmoA data set to avoid redundant correlations. Alpha diversity measures were calculated using default vegan functions (richness, Shannon). To obtain profiles for comparison and for co-linearity assessment, a number of missing values were imputed using the missMDA package [10 complete profiles for NO2, NO3, NH4, SO4, PO4, DIC (all June 2013), Chl-a (September 2014 and 2015), δ13C-CH4 (June 2013, September 2015), and eight missing values for pH in September 2014; star labeled plots in Supplementary Figure S1; Josse and Husson, 2016]. Except for pH, the imputed profiles were not incorporated in the statistical models but are discussed in the context of their co-linearity with non-imputed variables. Predicted values were tested by multiple‐ and over-imputation (Supplementary Figure S2).
A principal component analysis (PCA) with the environmental variables was performed to summarize the changes in physico-chemical variables along the depth gradients during temporal succession. Pearson correlations with Holmes-corrected p-values of physico-chemical parameters were calculated to assess the influence of single physico-chemical variables on specific MOB species. The mvabund package was used to test environmental, total bacterial and MOB community structure differences between different depth zones (Wang et al., 2012). To assess the environmental influence on the MOB community structuring, we applied canonical correlation analysis (CCA) based on selected environmental parameters (forward, backward, and both, incorporating variables that were chosen at least in two selection strategies). Variables with variance inflation factors (VIFs) > 10 were removed prior to model selection and significance was assessed by permutation tests (9,999 permutations).
Randomness in co-occurrence of MOB and total bacterial community was tested with C-score metric and quasiswap algorithm in a null model using the EcoSimR package (Gotelli and Ulrich, 2012; Gotelli et al., 2015). To determine if phylogenetically related species cluster within specific sites in different depth zones, we assessed the standardized (z-score) effect size (SES) of mean pairwise distances (MPD) and the mean nearest taxon distances (MNTD; Webb et al., 2002). SES were compared to a null model (“richness” null model, 999 randomizations of the phylogenetic tree) and differences between zones SES were assessed by ANOVA after checking for variance homogeneity and normality of the data distributions, followed by Tukey’s HSD. MPD is sensitive to differences in phylogenetically more distant taxa, whereas MNTD is sensitive to differences of phylogenetically more closely related taxa (i.e., tip of a phylogenetic tree). Non-metric multidimensional scaling (NMDS) of Bray-Curtis dissimilarity matrix of the square root transformed Wisconsin standardized OTU tables (bacteria and MOB) were determined. Overall similarity of MOB and the total bacterial community was assessed by Procrustes analysis of the first two dimensions of the respective NMDS plots. Significance of the Procrustes was estimated by a correlation-like statistic based on the squared m12 algorithm (9,999 permutations; Peres-Neto and Jackson, 2001).
To evaluate the inter-correlations of the total bacterial community, MOB and environmental variables explaining the depth zonation, we used the supervised N-integration discriminant analysis with DIABLO from the mixOmics package in R (Rohart et al., 2017). This analysis extracts complementary information from several data sets measured on the same samples but across different data type platforms (i.e., pmoA, 16S rRNA gene, and environmental variables) and aims to understand the interplay between the different levels of data that were measured. Optimal sparsity parameters were determined by computing M-fold cross-validation scores. The relative influence of inter-correlations of the selected environmental variables and bacterial community members on MOB at an association relevance level > 0.5 was assessed by partial Bray-Curtis dissimilarity-based redundancy analysis using Hellinger standardized 16S rRNA gene and pmoA data and scaled and centered environmental data. Variables having a VIF > 5 (environmental data) and VIF > 3 (16S rRNA gene) were stepwise removed and selected (forward, backward, and both) prior to analysis. The results of the DIABLO approach were analyzed by building relevance networks visualized with Cytoscape 3.7.2 using the EClerize layout. Non-randomness of the networks was tested by comparing the network to 10,000 random Erdös-Réyni networks with similar numbers of edges and nodes (Ju et al., 2014; Weiss et al., 2016). The network GML files are available as Supplementary Materials. See also section Supplementary Material for additional statistical analysis and plots.
Results
Limnological Conditions and Zonation
The defined depth zones over all four field campaigns were consistently different from each other in terms of their overall physico-chemical properties (mvabund: Likelihood Ratio Test (LRT) = 778.8, p < 0.001; Figure 1), and sampling time contributed considerably to the variability in the dataset (see ordination of samples based on their physicochemical characteristics in Figure 2). The annual T-driven stratification during summer months resulted in a narrowing of the oxycline and the oxidation zone as the season progressed (see T(°C) profiles in Supplementary Figure S1). The surface water was always well-oxygenated and O2 concentrations fell below detection limit usually in the upper half of the oxidation zone (Figure 1). The anoxic zone was substantially enriched with CH4, while only small amounts of CH4 (0.12–1.07 μM) were detected in the oxic zone, which, however, was still oversaturated relative to the atmosphere (Schubert et al., 2010). In September 2015, the oxycline was combined with the oxidation zone as CH4 profiles already slightly increased, where O2 decreased. In June 2013, light was detected almost to the sediment, therefore an anoxic zone was not defined. Vertical profiles of additionally measured physico-chemical parameters are summarized in Supplementary Figure S1. Turbidity (Turb) maxima were mostly congruent with Chl-a throughout the water column as profiled in June and August 2013. The anoxic zone exhibited substantial concentrations of TDN, PO4, DIC, and reduced substances (FeDGT/Diss, MnDGT/Diss, STot, and NH4). CuDGT, CuDiss, and CuTot were found to be highest at the lake’s surface and decreased strongly in the lower oxic zone and in the oxycline, whereas CuPart concentrations usually peaked within the oxidation zone. Some variables exhibited pronounced co-linearity (see vectors in PCA Figure 2; Supplementary Figure S2).
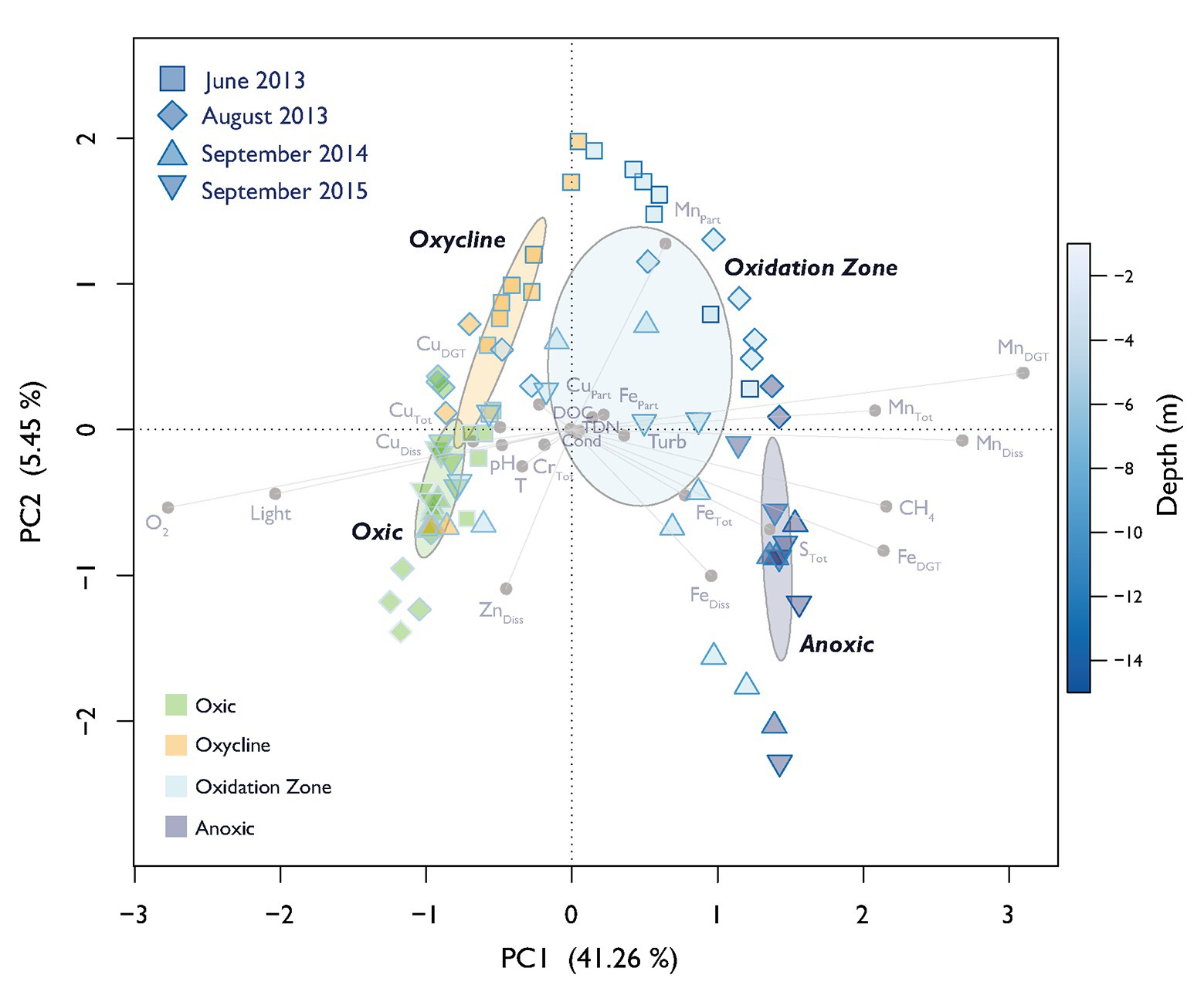
Figure 2. Principal component analysis (PCA) of physico-chemical variables. Dispersion ellipses depict the standard error of weighted average scores of depth zone groupings (confidence limits = 0.95). Symbols show scores of individual samples. The sampling date and assigned depth zones are colored accordingly. The color of the symbol outline illustrates the depth of the respective sample according to the depicted color gradient. The color of the symbol filling encodes the affiliation to the specific depth zone. Environmental variable loadings are depicted in light gray. Explained variance of PC1 and PC2 are given in parenthesis.
Microbial Community Structure (16S rRNA Gene)
An average of 41,951 reads per sample were assigned to 1,829 unique bacterial OTUs after filtering. Alpha diversity over all campaigns increased from around 400 OTUs in the surface water to approximately 1,000 OTUs close to the sediment (see alpha diversity plots in Supplementary Figure S3). Sixteen OTUs were assigned to proteobacterial MOB and five OTUs to potential verrucomicrobial MOB. Together they represented 1.07% of all filtered reads. These OTUs were removed in analysis for co-occurrence that incorporated both 16S rRNA gene and pmoA data to avoid trivial correlations. Actinobacteria, Bacteroidetes, non-MOB Proteobacteria, and Verrucomicrobia dominated the communities during all campaigns (Figure 3). Actinobacteria and Proteobacteria abundance tended to decrease or increase with depth, respectively. Cyanobacteria were abundant in both the oxic zone and oxycline in September 2015 and were present in lower proportions in the other three campaigns. Firmicutes were detected in the oxidation and anoxic zone. OD1 were highly abundant in the oxidation and anoxic zone in August 2013 and in September 2014 and 2015. Planctomycetes inhabited the oxic zone and oxycline mainly in August 2013. Microbial community structures were different between the sampling dates (mvabund: LRT = 29,741, p < 0.001) and not randomly distributed among depths over all campaigns (C-score metric for randomness in co-occurrence = 14.16, p < 0.001, SES = 24.75). Bacterial communities were further structured along the depth gradient during all campaigns and were significantly different between the depth zones (mvabund: LRT = 45,650, p < 0.001; see NMDS ordination in Supplementary Figure S4).
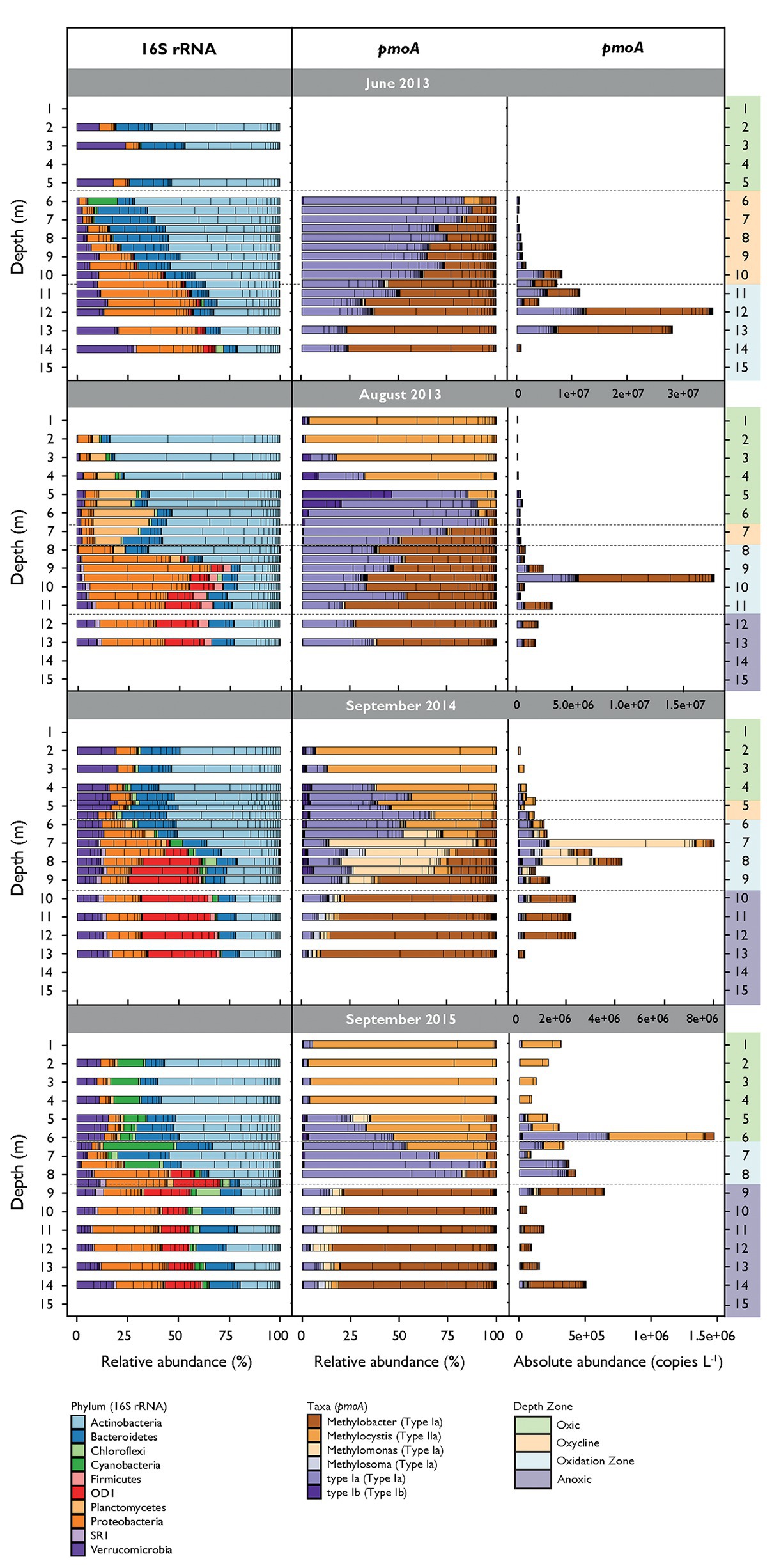
Figure 3. Relative abundance of most abundant bacterial phyla (16S rRNA gene) and methane-oxidizing bacteria (MOB) genera (pmoA) along the depth gradient during the different sampling dates. Bacteria phyla represent sequence reads that occur at least in 10% of the samples and represent at least 1% of total read counts. Bacterial and MOB relative abundances are standardized to the mean sequencing depth. MOB absolute abundances are calculated as relative abundance multiplied by pmoA copy numbers per liter determined by quantitative polymerase chain reaction (qPCR).
MOB Community Structure (pmoA)
We obtained an average of 89,816 pmoA reads per sample, which resulted in 121 OTUs after removing sparse OTUs (i.e., less than 50 reads in at least three samples). Alpha diversity increased with depth from 21 OTUs in the surface waters to 110 OTUs in deeper waters (Supplementary Figure S3). Sequences from type I (Gammaproteobacteria) and type II (Alphaproteobacteria) MOB were identified (Figure 3). Type I MOB were assigned to Methylobacter, Methylomonas, Methylosoma, and various environmental clusters (type Ia and type Ib), whereas type II MOB comprised only Methylocystis. MOB communities were structured along the depth gradient and differed between the depth zones and campaigns (mvabund: LRT = 3,134/2,900, p < 0.001; see NMDS ordination in Supplementary Figure S5). June 2013 was dominated by Methylobacter and type Ia (herein, we refer to type Ia excluding Methylobacter, Methylomonas, and Methylosoma) predominantly inhabiting the lower oxycline and the oxidation zone (Figure 3). Methylobacter was abundant in August 2013 in the oxidation and anoxic zone, whereas type Ia occurred from the oxycline on downward. In September 2014 and 2015, Methylobacter was also detected within anoxic waters. Methylocystis was found mainly in the oxic part of the lake, with the highest relative abundance in September 2015. Methylomonas and Methylosoma were most abundant in the CH4 oxidation zone of September 2014. Type Ib MOB were detected in low numbers in August 2013 in the lower part of the oxic zone and could also be found more dispersed within the oxic zone and the oxycline in September 2014 and 2015.
Statistical testing confirmed that MOB communities were not randomly distributed within the water column (C-score = 118.93, p < 0.001, SES = 98.4) and showed phylogenetic relatedness higher than expected by chance in each sample within the different zones (SES of MPD < 0, SES of MNTD < 0, SES are shown in Supplementary Figure S6). Phylogenetic relatedness at higher node levels changed between specific samples in the oxycline compared to the anoxic zone [ANOVA F(3,66) = 3.70, p < 0.05, Tukey’s HSD < 0.01], whereas phylogenetic relatedness at the tree-tip level (i.e., lower node levels) was highest within the oxidation and anoxic zone [ANOVA F(3,66) = 7.17, p < 0.001, Tukey’s HSD < 0.01]. These statistics indicate that MOB clades that are phylogenetically highly similar coexist within specific sites in the oxycline, whereas phylogenetically less similar MOB tend to be mutually exclusive. Inversely, sites within the anoxic zone have a narrower phylogenetic structuring but highly similar MOB tend to be mutually exclusive. Broad phylogenetic correlation with environmental structuring is evident, but some MOB show environmental preferences that are distinct from their close phylogenetic relatives (see color coded bar for genus in Figure 4).
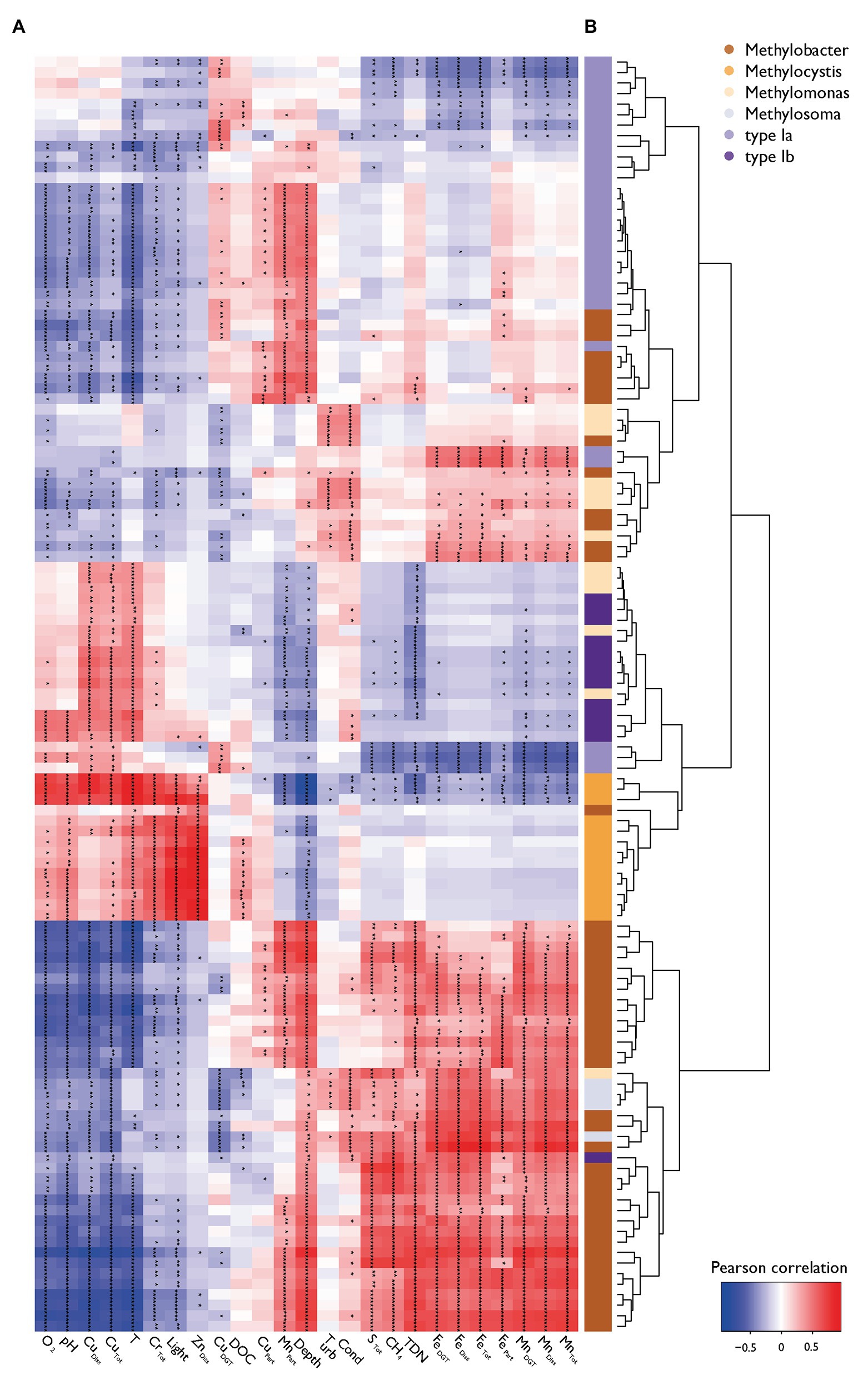
Figure 4. Pearson correlation heatmap of MOB (pmoA) and environmental variables. (A) The heatmap of the Pearson correlation of specific MOB OTUs abundance with physico-chemical variables is ordered by its column and row means. Asterisks indicate levels of Holmes corrected values of p (* p < 0.05, ** p < 0.01, *** p < 0.001). (B) A hierarchical environmental clustering dendrogram of MOB and their color coding according to their taxonomic affiliation are depicted on the right.
Identifying Environmental and Microbial Drivers of MOB Communities
A parsimonious CCA based on selected environmental variables explained a total of 69% of the MOB community structure over all campaigns with 42% of variation being explained on the first two axes (Figure 5). CH4 and O2 were the expected strong antipodal drivers. Light, CuDiss, pH, FeDGT, and TDN also contributed to the first canonical axis. Procrustes analysis showed statistically significant similarity between structuring of MOB and the rest of the bacterial community along the depth gradients over all sampling dates (Procrustes correlation = 0.51, p < 0.001). This could indicate either biological interactions between MOB and other microbes or similar niche preferences.
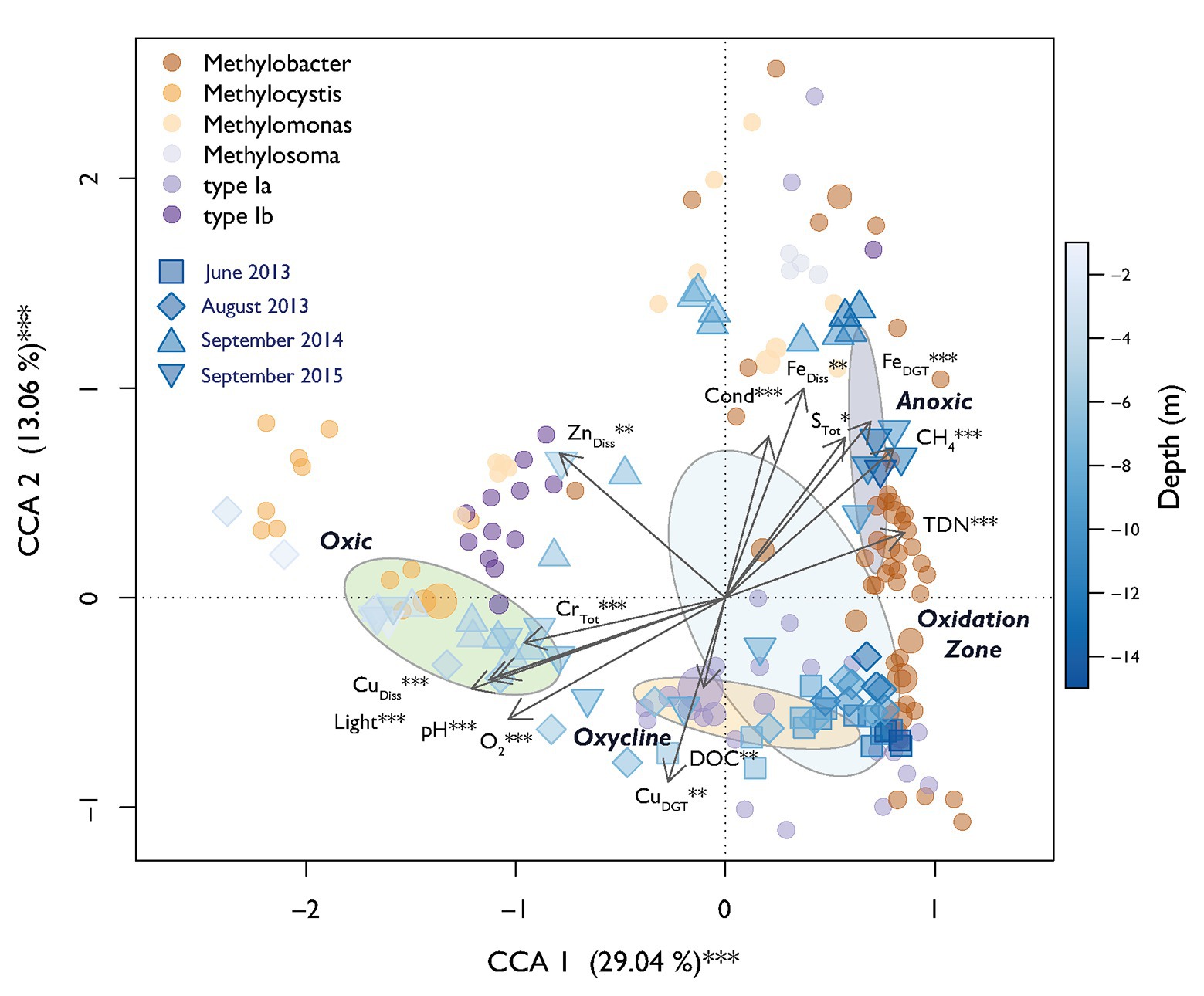
Figure 5. Canonical correspondence analysis (CCA) biplot of relative MOB (pmoA) abundance. Dots indicate specific MOB OTUs and are colored according to their taxonomic affiliation. The diameter of the dots is relative to the square root of the sums of the read counts standardized to the mean sequencing depth. Squares and triangles depict scores of specific sampling depths during different sampling dates. The fill color declares the depth of the respective sample according to the depicted color gradient. Dispersion ellipses show standard errors of weighted average scores of depth zones (confidence limits = 0.95). Environmental variables are fitted as arrows and the explained variance for canonical correlation analysis (CCA) axes 1 and 2 are given. Asterisks represent significance of permutational ANOVAS of the single variables and axes (** p < 0.01, *** p < 0.001).
Network analysis provides a framework to study associations between several classes of variables (Barberán et al., 2012; Comte et al., 2016). We constructed a relevance network to analyze the connection of MOB and the bacterial community composition (pmoA data and 16S rRNA gene data excluding 16S rRNA gene MOB OTUs from the latter) and physico-chemical variables (Figure 6A, for network characteristics see Supplementary Table S1). Fifty-nine MOB, 271 bacterial OTUs, and 17 physico-chemical variables formed distinct networks, with sub-networks that conformed to the depth zonation of Rotsee. This confirms that the originally hypothesized zonation can be broadly reconstructed from the dataset. The network architecture showed positive inter-correlations of Methylocystis with O2, CuDiss, light, pH, T, and ZnDiss in the surface waters (oxic zone). Type Ia MOB in the oxycline were connected to MnPart and CuDGT, and with CuPart and Turb in the oxidation zone. Within greater depths (anoxic zone), Methylobacter was linked to a larger set of bacteria and physico-chemical variables (MnDiss, CH4, FeDiss, TDN, STot, FeDGT, and MnDGT). Connections of MOB with bacterial OTU were common in all depth zone subclusters and are summarized in Figure 6A (i.e., number of connections of specific phylum within the network).
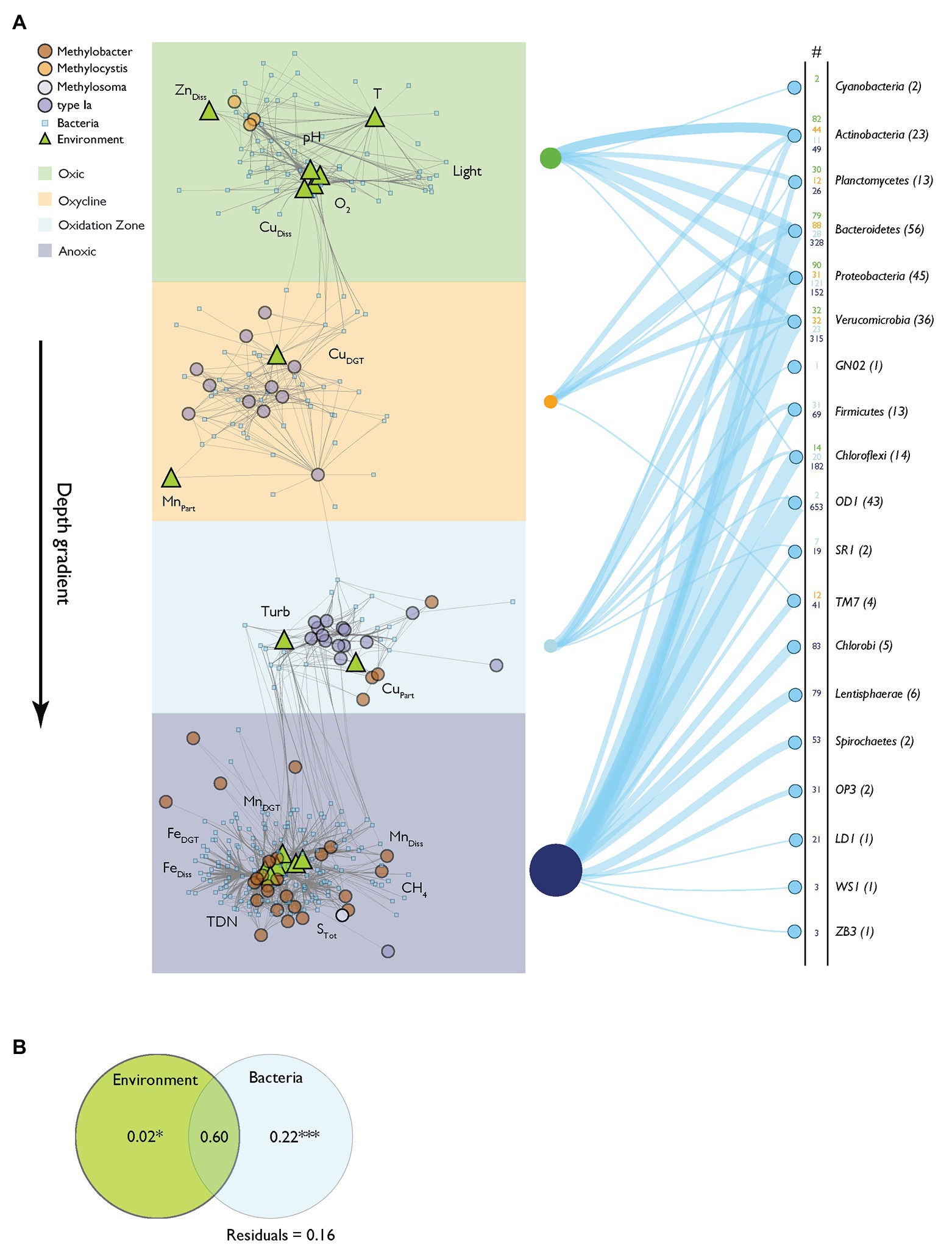
Figure 6. Relevance network based on N-integration discriminant analysis. (A) Relevance network of positive correlations between MOB, bacteria and physico-chemical variables. The network architecture reflects the assigned depth zones depicted as colored backgrounds. Gray network edges represent association relevance > 0.5. Nodes use symbols and color according to their source group: MOB (pmoA OTUs) are depicted as circles colored according to their phylogenetic group. Environmental variables are annotated as light green triangles. Bacterial 16S rRNA gene operational taxonomic units (OTUs) are shown as small, light blue squares. The right side of the panel shows the connectivity of distinct bacterial phyla to MOB within the different depth zones of the network. The numbers are colored according to the depth zones and connections are depicted as blue lines having relative widths according to the numbers of connections. Numbers in parentheses show the total numbers of specific bacterial phyla found in the network. (B) Partial redundancy analysis of model selected associated network variables (bacteria and physico-chemical variables) assessing influence on MOB occurrence. Significance levels of testable fractions are annotated (*** p < 0.001).
We used partial redundancy analysis to distinguish the relative contributions of physico-chemical variables and bacterial interactions on the variance of MOB abundance. According to this analysis, out of a total of 84% of explained variance in MOB occurrence, 22% can be explained exclusively by bacterial interactions, but only 2% exclusively by environmental drivers (Figure 6B). Interestingly, most of the explained variance is shared by physico-chemical variables and the bacterial community (60%). Specific bacterial OTUs affiliated with MOB in the network analysis are illustrated in the Cytoscape file (Supplementary File “Relevance network.cys”).
Discussion
MOB throughout the water column of Rotsee play a prominent role in mitigating CH4 emissions to the atmosphere (Schubert et al., 2010; Oswald et al., 2015). The dominance of type I MOB (Methylobacter, Methylomonas, Methylosoma, type Ia, and type Ib) over type II MOB (Methylocystis) is consistent with previously reported patterns in Rotsee and other freshwater lakes (Borrel et al., 2011; Bornemann et al., 2016; Oswald et al., 2016b; Rissanen et al., 2018; Mayr et al., 2019; Cabrol et al., 2020; Reis et al., 2020). Evidence for the presence of anaerobic MOB in Rotsee, such as NC10 (Methylomirabilis sp.), was not found (Mayr et al., 2019). Anyway, we identified five Methylacidiphilales in the 16S rRNA gene data set belonging to Methylacidimicrobium cyclopophantes, Methylacidimicrobium tartarophylas, and Methylacidimicrobium fagopyrum.
Our depth zones, defined based on coarse physico-chemical characteristics (CH4, O2, and light availability), broadly classify MOB habitat preferences during the sampling campaigns (Figures 1, 3), which confirms the broad ecological niches for MOB in stratified lakes proposed by Mayr et al. (2019). We found Rotsee to have a highly structured MOB distribution with groups of phylogenetically related MOB being present within the relatively small spatial scales of our defined depth zones. Mayr et al. previously observed MOB sharing similar depth distribution patterns in the same lake and it remained an open question how this diversity is maintained against competitive exclusion among organisms with a comparatively simple energy metabolism.
In this work, we focused on analyzing a large dataset of environmental parameters and bacterial (OTU) abundance to identify additional drivers of the MOB community structure throughout the whole stratified water column of Rotsee. This analysis suggested that in addition to CH4 and O2, also light, metal-species (Cu and Fe), pH, TDN, and STot played a significant role in further structuring MOB communities, explaining up to 69% of community variability according to the CCA (Figure 5). These parameters additionally showed high importance in the relevance network of MOB, other bacteria and the environment (Figure 6A).
Metals Are Important Drivers of MOB Community Composition
The availability of Cu as a co-factor of pMMO’s active site can restrict enzymatic activity in MOB communities and thus limit their growth (Semrau et al., 2010, 2018). Previous work on the role of Cu for MOB in Rotsee has already established a likely role of Cu scarcity and competition for Cu in the lake (Guggenheim et al., 2019). The present work provides additional evidence for Cu as an important factor controlling MOB community assembly: we observed positive correlations of MOB with changing importance of Cu-species along the depth gradient of Rotsee. CuDiss correlated with Methylomonas and type Ib OTUs, but most strongly with Methylocystis in the surface water (Figures 4, 5). These genera are able to use Cu acquisition mechanisms based on complexing agents to deal with low bioavailable Cu supply conditions (Ul-Haque et al., 2015). Such auxiliary peptides could mobilize Cu from the non-bioavailable CuDiss fraction (Dassama et al., 2017; Kenney and Rosenzweig, 2018). Additionally, most members of this taxon possess a high CH4 affinity copper-dependent pMMO isozyme, which would support oxidizing CH4 at the sub-micromolar CH4 concentrations prevalent in the surface water (Baani and Liesack, 2008; Reis et al., 2020). Methylocystis is often found in warmer waters (Borrel et al., 2011; Tsutsumi et al., 2011) and it is suggested that high T selects for type II over type I MOB (Sundh et al., 2005). Indeed, the warmer surface water of Rotsee seems to favor the presence of Methylocystis. It is thus likely that Methylocystis contribute to CH4 consumption in the oxic zone.
During late stratified periods, when CH4 has accumulated in the hypolimnion of Rotsee, the highest MOB abundance was found at the lower end of the oxycline and in the oxidation zone (Figure 3). Under these conditions CuDGT and CuPart correlated mostly with type Ia and Methylobacter OTUs (Figures 4, 5). It is thought that CuDGT is the bioavailable Cu fraction, whereas CuPart mainly represents Cu incorporated into biomass (Guggenheim et al., 2019). CuDGT concentrations decrease substantially from the oxycline toward the oxidation zone (Supplementary Figure S1). In situ studies focusing on the influence of Cu on MOB community structures are rare, but it has been elucidated that MOB able to express pMMO thrive even under very low levels of bioavailable Cu (< 50 nM; Cantera et al., 2016). In addition, as mentioned before, certain MOB species possess special Cu uptake mechanisms to increase the bioavailable Cu fraction.
Previous studies also reported the occurrence of Methylobacter species in the anoxic zones of stratified lakes (Biderre-Petit et al., 2011; Blees et al., 2014; Milucka et al., 2015; van Grinsven et al., 2020). Bioavailable and dissolved Fe (FeDGT and FeDiss) correlated with most Methylobacter species (Figures 4, 5), although cultivated representatives are not able to express the iron-dependent sMMO enzyme (Knief, 2015) and sMMO appears to be rare in Rot(see Guggenheim et al., 2019). However, it is possible that these MOB rely on Fe for other enzymatic pathways (e.g., formate dehydrogenase; Glass and Orphan, 2012). Furthermore, it has been suggested that the mechanism of Fe-coupled anaerobic CH4 oxidation is accomplished by a complex microbe-mineral reaction network in which both, MOB and iron-reducing organisms (bacteria and archaea) are directly and indirectly involved (Bar-Or et al., 2017; Cabrol et al., 2020). For example, besides methanogens being able to produce CH4, they are additionally involved in reducing Fe-oxides at high CH4 concentrations leading to intermediates, which are required by MOB for CH4 oxidation (Bar-Or et al., 2017). MnDGT and MnDiss also correlated with Methylobacter OTUs within the anoxic zone (Figures 4, 5). It has been proposed that an alternative anaerobic CH4 oxidation lifestyle proceeding via Fe(III) or Mn(IV) reduction could be relevant in CH4-rich anoxic zones of lakes, which could explain the increase in FeDiss and MnDiss in the deeper waters of Rotsee and its correlation with Methylobacter (Crowe et al., 2008; Oswald et al., 2016a; Supplementary Figure S1).
Interestingly, ZnDiss was also suggested as a significant driver by our analysis and was not co-linear with other environmental variables (Figures 4, 5; Supplementary Figure S2). There was a positive correlation of Methylocystis with ZnDiss although Zn potentially inhibits pMMO activity (Sirajuddin et al., 2014). However, the highest measured ZnDiss concentrations in Rotsee were ~100 times lower than those tested experimentally by Sirajuddin et al. The close network connectivity within the oxic zone between Methylocystis and other bacteria (i.e., linkage to Actinobacteria, Bacteria, Proteobacteria, and Bacteroidetes; Figure 6B) might indicate indirect effects mediated by Zn. For example, the bacterial OTU1 could be assigned to the order Candidatus Nanopelagicus within Actinobacteria isolated from Lake Zurich (Neuenschwander et al., 2018; see also the cytoscape file). The isolate showed a reduced genome and thus might have strong metabolic dependencies on co-occurring bacteria (i.e., MOB) for lost metabolic functions that have to be provided by functional leakage (Morris et al., 2012). As Actinobacteria exhibit Zn concentration linked gene regulation mechanisms, this might mirrors a reverse effect of Methylocystis on this Candidatus Nanopelagicus (Choi et al., 2017), mediated by, i.e., the release of riboflavin, nicotinamide, and thiamine of the Candidatus Nanopelagicus. Anyway, further work will be necessary to confirm the role of Zn and to investigate potential mechanisms linking its availability to MOB dynamics.
Other Environmental Controls
The highly significant contribution of total dissolved nitrogen (TDN) as an explanatory variable for MOB abundance indicates links between MOB and nitrogen availability (Figures 4, 5). In particular, TDN contributed to the position of Methylobacter in the relevance network (Figure 6A). The observed gradient of TDN strongly correlated with NH4 and exhibited similar concentration ranges suggesting that NH4 contributes the largest part of TDN (Supplementary Figures S1, S2). NH4 is a central nutrient in aquatic systems, hence a positive correlation between NH4 and MOB could be due to the fact that MOB assimilate NH4 for growth. Previously, a laboratory study with littoral wetland samples from a boreal lake has demonstrated that nitrogen load in form of NH4NO3 changed the MOB community structure and favored activity of type I MOB, particularly Methylobacter cells (Siljanen et al., 2012). The evolutionary linkage of the genetic sequence of pmoA and amoA, which encodes for the ammonia monooxygenase (AMO), endows most MOB, especially type I MOB, with the ability to oxidize NH4 through the pMMO enzyme (Knief, 2015; Khadka et al., 2018), which may be another explanation for the link between MOB and NH4.
CH4 oxidation can also be coupled directly to the nitrogen cycle. Anaerobic oxidation of CH4 via NO2-/NO3-reduction (n-damo) has normally been attributed to Methylomirabilis species of the NC10 phylum, which according to previous data do not appear in Rot(see Mayr et al., 2019). However, recent whole genome and environmental metagenome analysis have revealed that various assimilatory and dissimilatory nitrogen reduction genes, such as those encoding NO2‐ and NO3-reductases, are also found among gammaproteobacterial MOB, especially Methylobacter and Methylomonas species (Chistoserdova, 2015; Zhu et al., 2016). This would suggest that NO2 and NO3 can be used by MOB to oxidize CH4. However, NO2 and NO3 were not detected below the oxidation zone in Rot(see Supplementary Figure S1).
Co-Occurrence of MOB With Bacteria and Possible Ecological Interactions
Spatio-temporal shifts in the MOB community were highly congruent with the changing composition of the total bacterial community (OTU level, Procrustes 51%). The restrictive and congruent localization of MOB and bacterial OTUs throughout the stratified periods suggests that biological interactions between MOB and other bacteria need to be analyzed in more detail.
In our network analysis we focused exclusively on positive MOB co-occurrence patterns with environmental variables and the bacterial community. This means that observed associations can arise from a number of causes: species co-occurrence can be driven by direct biological interactions such as synergism (i.e., cooperation) but also by similar niche preferences (neutral effects; Faust and Raes, 2012; Ho et al., 2016). Additionally, positive feedback loops between MOB and the total bacterial community interacting with their environment may also result in co-occurrence. It is noteworthy that positive interactions with bacteria alone (excluding impact of the environment) explained a relevant part of the MOB occurrence (22%; Figure 6B). This indicates that at least some of the correlations are through actual microbial interactions and do not just reflect overlapping niche preferences. Anyway, it is possible that these interactions are mediated through an effect chain of non-measured variables, i.e., by the influence of trophic levels ultimately influencing MOB dynamics.
However, the majority of variance in MOB abundance within the network was explained jointly by physico-chemical parameters and the bacterial community (60%; Figure 6B), while according to CCA environmental parameters explained 69% of MOB abundance. While this underscores the importance of physico-chemical parameters as drivers of the bacterial and MOB community structure, positive correlations of this type could still involve biological interactions. Physico-chemical variables may, e.g., affect the bacterial community structure, which subsequently influences the MOB community by synergistic effects or vice versa. The available data do not allow us to distinguish between these possibilities. It is further possible that unmeasured environmental variables contribute to shaping of the MOB community structure. However, it is noteworthy that bacterial and physico-chemical inter-connectivities showed high complexity in the surface layer and the hypolimnion of the lake (i.e., number of connections in Figure 6A), while inter-connectivities within the oxycline and oxidation zones were less distinct.
Hereafter, we suggest some examples of possible positive bacterial interaction mechanisms taking place in the water column of Rotsee. Within the oxidation zone, part of the type Ia and Methylobacter communities correlated with Turbidity (Turb), a possible indicator for primary producers as Turb showed some overlapping patterns with Chl-a depth profiles (Supplementary Figure S2). MOB can form mutualistic interactions with oxygenic phototrophs in light penetrated anoxic layers enabling CH4 oxidation, while potentially providing carbon dioxide in return (Milucka et al., 2015). It seems likely that these MOB clusters are the main CH4 consumers in Rotsee as the main aerobic CH4 oxidation process in Rotsee during stratification happens within the oxycline and oxidation zone and might be predominantly coupled to oxygenic primary production (Oswald et al., 2015; Brand et al., 2016). There is also evidence that CH4 oxidation by MOB under O2 limitation is indirectly connected to the reduction of alternative terminal electron acceptors by other organisms in the anoxic waters of the lake. NO2 and NO3 were not measured in the anoxic waters of Rotsee, however, NO2 could have been produced by Fe-/Mn-dependent anaerobic NH4-oxidizing bacteria, and readily used by gammaproteobacterial MOB for oxidizing CH4 (Ferousi et al., 2017; Kuypers et al., 2018). Due to the previously mentioned homology between pMMO and AMO, MOB and nitrifying bacteria are capable of both, CH4 and NH4 oxidation. Hence, the coexistence of MOB and nitrifying bacteria under anoxic conditions could be explained on the basis of similar niche preferences (Costa et al., 2019). Co-occurrence of MOB in the same areas as non-methanotrophic methylotrophs might be beneficial for MOB. Since high methanol concentrations inhibit MOB performance, co-occurring methylotrophs, which are able to assimilate methanol, remove this compound to levels that enable MOB to thrive (Denfeld et al., 2016). A recent in-situ study shows that non-methanotrophic methylotrophs induce a change in the expression of MOB MDHs via putative secretory compounds leading to an increased loss of methanol, which is readily taken up by the methylotrophs (He et al., 2012b; Biderre-Petit et al., 2019). For example, we found highly connected methylotrophs belonging to the family Methylophilaceae and the Verrucomicrobia subdivision 6 in the oxic zone in the relevance network. In the anoxic zone, we found members of the S-BQ2-57 soil group belonging to Verrucomicrobia that showed high connectivity within the network. Methanol also plays a significant role between the coupling of aerobic CH4 oxidation and denitrification by the cooperation between MOB and denitrifying bacteria. Organic metabolites (i.e., methanol, citrate, acetate, formaldehyde, and formate) released by MOB could serve as electron donors for denitrification, where methanol is thermodynamically considered as the ideal trophic link (Kalyuhznaya et al., 2009; Zhu et al., 2016).
Conclusion
In summary, our results indicate that the MOB community assembly in Rotsee is sensitively linked to environmental conditions and the greater bacterial community. The distinct zonation of MOB throughout the water column, which is thought to be driven by CH4 and O2 counter gradients, was additionally linked to several physico-chemical variables and their interactive effect with parts of the bacterial community. Considering the three-way relation of MOB, bacteria and environment, our analysis revealed that bacteria alone could explain significantly more of the MOB structure (22%) than the isolated physico-chemical variability (2%; Figure 6B). However, the mode of action underlying the correlations could not be unambiguously unraveled. Future studies with a strong focus on microbial interdependency that incorporate deep sequencing metagenomic and transcriptomic as well as metabolic and anabolic analysis tools will help to disentangle the mode of actions of the herein presented inter-connectivity (Zheng and Chistoserdova, 2019). Understanding the mechanisms of these biotic and abiotic interactions will help to predict the responses of MOB community functioning under diverse conditions.
Data Availability Statement
The datasets presented in this study can be found in online repositories. The names of the repository/repositories and accession number(s) can be found at: https://www.ebi.ac.uk/ena, PRJEB28460, PRJEB28505.
Author Contributions
CG, RF, HB, and BW planned the experiments. CG, HB, KB, and MM performed laboratory and field work. CG and RF analyzed the data. CG, RF, HB, and BW wrote the manuscript with support and input of all coauthors. All authors contributed to the article and approved the submitted version.
Funding
This study was made possible through research grants (no. 153091, 156759) by the Swiss National Science Foundation.
Conflict of Interest
The authors declare that the research was conducted in the absence of any commercial or financial relationships that could be construed as a potential conflict of interest.
Acknowledgments
We are grateful to Andreas Brand, Christian Dinkel, Kirsten Oswald, Hannah Bruderer, Tanja Beck, and Rohini Athavale for their help during field and lab work. We wish to thank Laura Sigg and Niksa Odzak for mentoring support in DGT preparation and interpretation of its results. David Kistler, Serge Robert, Gijs Nobbe (R.I.P.), and Patrick Kathriner are thanked for their assistance in ICP-MS, GC, IC and FIA analysis. Special thanks goes to Kirsten Oswald and Rohini Athavale for making several methane, nutrients, ammonium, and total sulphide profiles available. We are grateful to Carsten Schubert for valuable inputs and discussions and for the use of his laboratory for isotope analysis. We appreciate the help of the Genetic Diversity Centre at ETH Zurich, particularly Jean-Claude Walser, in preparing and analysing next-generation sequencing data. We thank Christian Beisel from the Genomics Facility Basel for his help with pmoA library preparation and sequencing. Feng Ju is acknowledged for giving input on co-occurrence analysis.
Supplementary Material
The Supplementary Material for this article can be found online at: https://www.frontiersin.org/articles/10.3389/fmicb.2020.579427/full#supplementary-material
Footnotes
References
Agasild, H., Zingel, P., Tuvikene, L., Tuvikene, A., Timm, H., Feldmann, T., et al. (2014). Biogenic methane contributes to the food web of a large, shallow lake. Freshw. Biol. 59, 272–285. doi: 10.1111/fwb.12263
Baani, M., and Liesack, W. (2008). Two isozymes of particulate methane monooxygenase with different methane oxidation kinetics are found in Methylocystis sp. strain SC2. Proc. Natl. Acad. Sci. 105, 10203–10208. doi: 10.1073/pnas.0702643105
Barberán, A., Bates, S. T., Casamayor, E. O., and Fierer, N. (2012). Using network analysis to explore co-occurrence patterns in soil microbial communities. ISME J. 6, 343–351. doi: 10.1038/ismej.2011.119
Bar-Or, I., Elvert, M., Eckert, W., Vigderovich, H., Zhu, Q., Ben-Dov, E., et al. (2017). Iron-coupled anaerobic oxidation of methane performed by a mixed bacterial-archaeal community based on poorly reactive minerals. Environ. Sci. Technol. 51, 12293–12301. doi: 10.1021/acs.est.7b03126
Bastviken, D., Cole, J. J., Pace, M. L., and Van de Bogert, M. C. (2008). Fates of methane from different lake habitats: connecting whole-lake budgets and CH4 emissions. J. Geophys. Res. 113:G02024. doi: 10.1029/2007JG000608
Bastviken, D., Ejlertsson, J., Sundh, I., and Tranvik, L. (2003). Methane as a source of carbon and energy for lake pelagic food webs. Ecology 84, 969–981. doi: 10.1890/0012-9658(2003)084[0969:MAASOC]2.0.CO;2
Bastviken, D., Ejlertsson, J., and Tranvik, L. J. (2002). Measurement of methane oxidation in lakes: a comparison of methods. Environ. Sci. Technol. 36, 3354–3361. doi: 10.1021/es010311p
Biderre-Petit, C., Jézéquel, D., Dugat-Bony, E., Lopes, F., Kuever, J., Borrel, G., et al. (2011). Identification of microbial communities involved in the methane cycle of a freshwater meromictic lake. FEMS Microbiol. Ecol. 77, 533–545. doi: 10.1111/j.1574-6941.2011.01134.x
Biderre-Petit, C., Taib, N., Gardon, H., Hochart, C., and Debroas, D. (2019). New insights into the pelagic microorganisms involved in the methane cycle in the meromictic Lake Pavin through metagenomics. FEMS Microbiol. Ecol. 95, 1–14. doi: 10.1093/femsec/fiy183
Bižić-Ionescu, M., Klintzsch, T., Ionescu, D., Hindiyeh, M. Y., Günthel, M., Muro-Pastor, A. M., et al. (2018). Widespread formation of methane by Cyanobacteria in aquatic and terrestrial environments. bioRxiv. doi: 10.1101/398958, [Preprint]
Blees, J., Niemann, H., Wenk, C. B., Zopfi, J., Schubert, C. J., Kirf, M. K., et al. (2014). Micro-aerobic bacterial methane oxidation in the chemocline and anoxic water column of deep south-Alpine Lake Lugano (Switzerland). Limnol. Oceanogr. 59, 311–324. doi: 10.4319/lo.2014.59.2.0311
Bogard, M. J., Del Giorgio, P. A., Boutet, L., Chaves, M. C. G., Prairie, Y. T., Merante, A., et al. (2014). Oxic water column methanogenesis as a major component of aquatic CH4 fluxes. Nat. Commun. 5, 1–9. doi: 10.1038/ncomms6350
Bornemann, M., Bussmann, I., Tichy, L., Deutzmann, J. S., Schink, B., and Pester, M. (2016). Methane release from sediment seeps to the atmosphere is counteracted by highly active Methylococcaceae in the water column of deep oligotrophic Lake Constance. FEMS Microbiol. Ecol. 92:fiw123. doi: 10.1093/femsec/fiw123
Borrel, G., Jézéquel, D., Biderre-Petit, C., Morel-Desrosiers, N., Morel, J. P., Peyret, P., et al. (2011). Production and consumption of methane in freshwater lake ecosystems. Res. Microbiol. 162, 833–847. doi: 10.1016/j.resmic.2011.06.004
Bourne, D. G., McDonald, I. R., and Murrell, J. C. (2001). Comparison of pmoA PCR primer sets as tools for investigating methanotroph diversity in three Danish soils. Appl. Environ. Microbiol. 67, 3802–3809. doi: 10.1128/AEM.67.9.3802-3809.2001
Brand, A., Bruderer, H., Oswald, K., Guggenheim, C., Schubert, C. J., and Wehrli, B. (2016). Oxygenic primary production below the oxycline and its importance for redox dynamics. Aquat. Sci. 78, 727–741. doi: 10.1007/s00027-016-0465-4
Cabrol, L., Thalasso, F., Gandois, L., Sepulveda-Jauregui, A., Martinez-Cruz, K., Teisserenc, R., et al. (2020). Anaerobic oxidation of methane and associated microbiome in anoxic water of Northwestern Siberian lakes. Sci. Total Environ. 736:139588. doi: 10.1016/j.scitotenv.2020.139588
Cantera, S., Lebrero, R., García-Encina, P. A., and Muñoz, R. (2016). Evaluation of the influence of methane and copper concentration and methane mass transport on the community structure and biodegradation kinetics of methanotrophic cultures. J. Environ. Manag. 171, 11–20. doi: 10.1016/j.jenvman.2016.02.002
Carini, S., Bano, N., LeCleir, G., and Joye, S. B. (2005). Aerobic methane oxidation and methanotroph community composition during seasonal stratification in Mono Lake, California (USA). Environ. Microbiol. 7, 1127–1138. doi: 10.1111/j.1462-2920.2005.00786.x
Chidambarampadmavathy, K., Karthikeyan, O. P., Huerlimann, R., Maes, G. E., and Heijmans, K. (2017). Responses of mixed methanotrophic consortia to variable Cu2+/Fe2+ ratios. J. Environ. Manag. 197, 159–166. doi: 10.1016/j.jenvman.2017.03.063
Chistoserdova, L. (2011). Methylotrophy in a lake: from metagenomics to single-organism physiology. Appl. Environ. Microbiol. 77, 4705–4711. doi: 10.1128/AEM.00314-11
Chistoserdova, L. (2015). Methylotrophs in natural habitats: current insights through metagenomics. Appl. Microbiol. Biotechnol. 99, 5763–5779. doi: 10.1007/s00253-015-6713-z
Chistoserdova, L. (2019). New pieces to the lanthanide puzzle. Mol. Microbiol. 111, 1127–1131. doi: 10.1111/mmi.14210
Chistoserdova, L., and Lidstrom, M. E. (2013). “Aerobic methylotrophic prokaryotes” in The prokaryotes: Prokaryotic physiology and biochemistry. eds. E. Rosenberg, E. F. De Long, F. Thompson, S. Lory, and E. Stackebrandt (Berlin, Heidelberg: Springer-Verlag), 267–285.
Choi, S. H., Lee, K. L., Shin, J. H., Cho, Y. B., Cha, S. S., and Roe, J. H. (2017). Zinc-dependent regulation of zinc import and export genes by zur. Nat. Commun. 8, 1–11. doi: 10.1038/ncomms15812
Comte, J., Lovejoy, C., Crevecoeur, S., and Vincent, W. F. (2016). Co-occurrence patterns in aquatic bacterial communities across changing permafrost landscapes. Biogeosciences 13, 175–190. doi: 10.5194/bg-13-175-2016
Costa, R. B., Okada, D. Y., Delforno, T. P., and Foresti, E. (2019). Methane-oxidizing archaea, aerobic methanotrophs and nitrifiers coexist with methane as the sole carbon source. Int. Biodeterior. Biodegrad. 138, 57–62. doi: 10.1016/j.ibiod.2019.01.005
Costello, A. M., and Lidstrom, M. E. (1999). Molecular characterization of functional and phylogenetic genes from natural populations of methanotrophs in lake sediments. Appl. Environ. Microbiol. 65, 5066–5074. doi: 10.1128/AEM.65.11.5066-5074.1999
Crowe, S. A., O’Neill, A. H., Katsev, S., Hehanussa, P., Haffner, G. D., Sundby, B., et al. (2008). The biogeochemistry of tropical lakes: a case study from Lake Matano. Indonesia. Limnol. Oceanogr. 53, 319–331. doi: 10.4319/lo.2008.53.1.0319
Csárdi, G., and Nepusz, T. (2006). The igraph software package for complex network research. InterJ. Complex Syst. 1695, 1–9. doi: 10.3724/SP.J.1087.2009.02191
Dassama, L. M. K., Kenney, G. E., and Rosenzweig, A. C. (2017). Methanobactins: from genome to function. Metallomics 9, 7–20. doi: 10.1039/C6MT00208K
Davison, W. (2016). Diffusive gradients in thin-films for environmental measurements. Cambridge: Cambridge University Press.
DelSontro, T., Beaulieu, J. J., and Downing, J. A. (2018). Greenhouse gas emissions from lakes and impoundments: upscaling in the face of global change. Limnol. Oceanogr. Lett. 3, 64–75. doi: 10.1002/lol2.10073
Denfeld, B. A., Ricão Canelhas, M., Weyhenmeyer, G. A., Bertilsson, S., Eiler, A., and Bastviken, D. (2016). Constraints on methane oxidation in ice-covered boreal lakes. J. Geophys. Res. Biogeosci. 121, 1924–1933. doi: 10.1002/2016JG003382
Deutzmann, J. S., Stief, P., Brandes, J., and Schink, B. (2014). Anaerobic methane oxidation coupled to denitrification is the dominant methane sink in a deep lake. Proc. Natl. Acad. Sci. 111, 18273–18278. doi: 10.1073/pnas.1411617111
Donis, D., Flury, S., Stöckli, A., Spangenberg, J. E., Vachon, D., and McGinnis, D. F. (2017). Full-scale evaluation of methane production under oxic conditions in a mesotrophic lake. Nat. Commun. 8:1661. doi: 10.1038/s41467-017-01648-4
Dumont, M. G. (2014). “Primers: functional marker genes for methylotrophs and methanotrophs” in Hydrocarbon and lipid microbiology protocols. eds. T. J. McGenity, K. N. Timmis, and B. Nogales (Berlin, Heidelberg: Springer-Verlag Berlin Heidelberg), 57–77.
Faust, K., and Raes, J. (2012). Microbial interactions: from networks to models. Nat. Rev. Microbiol. 10, 538–550. doi: 10.1038/nrmicro2832
Ferousi, C., Lindhoud, S., Baymann, F., Kartal, B., Jetten, M. S. M., and Reimann, J. (2017). Iron assimilation and utilization in anaerobic ammonium oxidizing bacteria. Curr. Opin. Chem. Biol. 37, 129–136. doi: 10.1016/j.cbpa.2017.03.009
Gilman, A., Fu, Y., Hendershott, M., Chu, F., Puri, A. W., Smith, A. L., et al. (2017). Oxygen-limited metabolism in the methanotroph Methylomicrobium buryatense 5GB1C. PeerJ 5:e3945. doi: 10.7717/peerj.3945
Glass, J. B., and Orphan, V. J. (2012). Trace metal requirements for microbial enzymes involved in the production and consumption of methane and nitrous oxide. Front. Microbiol. 3:61. doi: 10.3389/fmicb.2012.00061
Gotelli, N. J., Hart, E. M., and Ellison, A. M. (2015). Package “EcoSimR”—null model analysis for ecological data. R package version 0.1.0. doi: 10.5281/zenodo.16522.
Gotelli, N. J., and Ulrich, W. (2012). Statistical challenges in null model analysis. Oikos 121, 171–180. doi: 10.1111/j.1600-0706.2011.20301.x
Graf, J. S., Mayr, M. J., Marchant, H. K., Tienken, D., Hach, P. F., Brand, A., et al. (2018). Bloom of a denitrifying methanotroph, ‘Candidatus Methylomirabilis limnetica’, in a deep stratified lake. Environ. Microbiol. 20, 2598–2614. doi: 10.1111/1462-2920.14285
Grossart, H. -P., Frindte, K., Dziallas, C., Eckert, W., and Tang, K. W. (2011). Microbial methane production in oxygenated water column of an oligotrophic lake. Proc. Natl. Acad. Sci. 108, 19657–19661. doi: 10.1073/pnas.1110716108
Guggenheim, C., Brand, A., Bürgmann, H., Sigg, L., and Wehrli, B. (2019). Aerobic methane oxidation under copper scarcity in a stratified lake. Sci. Rep. 9:4817. doi: 10.1038/s41598-019-40642-2
Günthel, M., Donis, D., Kirillin, G., Ionescu, D., Bizic, M., McGinnis, D. F., et al. (2019). Contribution of oxic methane production to surface methane emission in lakes and its global importance. Nat. Commun. 10:5497. doi: 10.1038/s41467-019-13320-0
He, R., Wooller, M. J., Pohlman, J. W., Catranis, C., Quensen, J., Tiedje, J. M., et al. (2012a). Identification of functionally active aerobic methanotrophs in sediments from an arctic lake using stable isotope probing. Environ. Microbiol. 14, 1403–1419. doi: 10.1111/j.1462-2920.2012.02725.x
He, R., Wooller, M. J., Pohlman, J. W., Quensen, J., Tiedje, J. M., and Leigh, M. B. (2012b). Diversity of active aerobic methanotrophs along depth profiles of arctic and subarctic lake water column and sediments. ISME J. 6, 1937–1948. doi: 10.1038/ismej.2012.34
Herlemann, D. P. R., Labrenz, M., Jürgens, K., Bertilsson, S., Waniek, J. J., and Andersson, A. F. (2011). Transitions in bacterial communities along the 2000 km salinity gradient of the Baltic Sea. ISME J. 5, 1571–1579. doi: 10.1038/ismej.2011.41
Ho, A., Angel, R., Veraart, A. J., Daebeler, A., Jia, Z., Kim, S. Y., et al. (2016). Biotic interactions in microbial communities as modulators of biogeochemical processes: methanotrophy as a model system. Front. Microbiol. 7:1285. doi: 10.3389/fmicb.2016.01285
Ho, A., de Roy, K., Thas, O., De Neve, J., Hoefman, S., Vandamme, P., et al. (2014). The more, the merrier: heterotroph richness stimulates methanotrophic activity. ISME J. 8, 1945–1948. doi: 10.1038/ismej.2014.74
Hofmann, H., Federwisch, L., and Peeters, F. (2010). Wave-induced release of methane: littoral zones as source of methane in lakes. Limnol. Oceanogr. 55, 1990–2000. doi: 10.4319/lo.2010.55.5.1990
Iguchi, H., Yurimoto, H., and Sakai, Y. (2015). Interactions of methylotrophs with plants and other heterotrophic bacteria. Microorganisms 3, 137–151. doi: 10.3390/microorganisms3020137
Jones, R. I., and Grey, J. (2011). Biogenic methane in freshwater food webs. Freshw. Biol. 56, 213–229. doi: 10.1111/j.1365-2427.2010.02494.x
Josse, J., and Husson, F. (2016). missMDA: a package for handling missing values in multivariate data analysis. J. Stat. Softw. 70, 1–31. doi: 10.18637/jss.v070.i01
Ju, F., Xia, Y., Guo, F., Wang, Z., and Zhang, T. (2014). Taxonomic relatedness shapes bacterial assembly in activated sludge of globally distributed wastewater treatment plants. Environ. Microbiol. 16, 2421–2432. doi: 10.1111/1462-2920.12355
Kalyuhznaya, M. G., Martens-Habbena, W., Wang, T., Hackett, M., Stolyar, S. M., Stahl, D. A., et al. (2009). Methylophilaceae link methanol oxidation to denitrification in freshwater lake sediment as suggested by stable isotope probing and pure culture analysis. Environ. Microbiol. Rep. 1, 385–392. doi: 10.1111/j.1758-2229.2009.00046.x
Kembel, S. W., Cowan, P. D., Helmus, M. R., Cornwell, W. K., Morlon, H., Ackerly, D. D., et al. (2010). Picante: R tools for integrating phylogenies and ecology. Bioinformatics 26, 1463–1464. doi: 10.1093/bioinformatics/btq166
Kenney, G. E., and Rosenzweig, A. C. (2018). Methanobactins: maintaining copper homeostasis in methanotrophs and beyond. J. Biol. Chem. 293, 4606–4615. doi: 10.1074/jbc.TM117.000185
Khadka, R., Clothier, L., Wang, L., Lim, C. K., Klotz, M. G., and Dunfield, P. F. (2018). Evolutionary history of copper membrane monooxygenases. Front. Microbiol. 9, 1–13. doi: 10.3389/fmicb.2018.02493
Knief, C. (2015). Diversity and habitat preferences of cultivated and uncultivated aerobic methanotrophic bacteria evaluated based on pmoA as molecular marker. Front. Microbiol. 6:1346. doi: 10.3389/fmicb.2015.01346
Kuypers, M. M. M., Marchant, H. K., and Kartal, B. (2018). The microbial nitrogen-cycling network. Nat. Rev. Microbiol. 16, 263–276. doi: 10.1038/nrmicro.2018.9
Lee, S.-W., Keeney, D. R., Lim, D. H., Dispirito, A. A., and Semrau, J. D. (2006). Mixed pollutant degradation by Methylosinus trichosporium OB3b expressing either soluble or particulate methane monooxygenase: can the tortoise beat the hare. Appl. Environ. Microbiol. 72, 7503–7509. doi: 10.1128/AEM.01604-06
Martinez-Cruz, K., Sepulveda-Jauregui, A., Casper, P., Anthony, K. W., Smemo, K. A., and Thalasso, F. (2018). Ubiquitous and significant anaerobic oxidation of methane in freshwater lake sediments. Water Res. 144, 332–340. doi: 10.1016/j.watres.2018.07.053
Mayr, M. J., Zimmermann, M., Guggenheim, C., Brand, A., and Bürgmann, H. (2019). Niche partitioning of methane-oxidizing bacteria in the oxygen-methane counter gradient of stratified lakes. ISME J. 14, 274–287. doi: 10.1038/s41396-019-0515-8
McMurdie, P. J., and Holmes, S. P. (2013). Phyloseq: an R package for reproducible interactive analysis and graphics of microbiome census data. PLoS One 8:e61217. doi: 10.1371/journal.pone.0061217
Merkx, M., Kopp, D. A., Sazinsky, M. H., Blazyk, J. L., Müller, J., and Lippard, S. J. (2001). Dioxygen activation and methane hydroxylation by soluble methane monooxygenase: a tale of two irons and three proteins. Angew. Chem. Int. Ed. 40, 2782–2807. doi: 10.1002/1521-3773(20010803)40:15<2782::AID-ANIE2782>3.0.CO;2-P
Milucka, J., Kirf, M., Lu, L., Krupke, A., Lam, P., Littmann, S., et al. (2015). Methane oxidation coupled to oxygenic photosynthesis in anoxic waters. ISME J. 9, 1991–2002. doi: 10.1038/ismej.2015.12
Morris, B. E. L., Henneberger, R., Huber, H., and Moissl-Eichinger, C. (2013). Microbial syntrophy: interaction for the common good. FEMS Microbiol. Rev. 37, 384–406. doi: 10.1111/1574-6976.12019
Morris, J. J., Lenski, R. E., and Zinser, E. R. (2012). The black queen hypothesis: evolution of dependencies through adaptive gene loss. MBio 3, e00036–e00012. doi: 10.1128/mBio.00036-12
Neuenschwander, S. M., Ghai, R., Pernthaler, J., and Salcher, M. M. (2018). Microdiversification in genome-streamlined ubiquitous freshwater Actinobacteria. ISME J. 12, 185–198. doi: 10.1038/ismej.2017.156
Nordi, K. Á., Thamdrup, B., and Schubert, C. J. (2013). Anaerobic oxidation of methane in an iron-rich Danish freshwater lake sediment. Limnol. Oceanogr. 58, 546–554. doi: 10.4319/lo.2013.58.2.0546
Oksanen, A. J., Blanchet, F. G., Kindt, R., Legendre, P., Minchin, P. R., Hara, R. B. O., et al. (2018). vegan: community ecology package. R package version 2.4-4.
Oshkin, I. Y., Beck, D. A. C., Lamb, A. E., Tchesnokova, V., Benuska, G., Mctaggart, T. L., et al. (2015). Methane-fed microbial microcosms show differential community dynamics and pinpoint taxa involved in communal response. ISME J. 9, 1119–1129. doi: 10.1038/ismej.2014.203
Oswald, K., Jegge, C., Tischer, J., Berg, J., Brand, A., Miracle, M. R., et al. (2016a). Methanotrophy under versatile conditions in the water column of the ferruginous meromictic Lake La Cruz (Spain). Front. Microbiol. 7:1762. doi: 10.3389/fmicb.2016.01762
Oswald, K., Milucka, J., Brand, A., Hach, P., Littmann, S., Wehrli, B., et al. (2016b). Aerobic gammaproteobacterial methanotrophs mitigate methane emissions from oxic and anoxic lake waters. Limnol. Oceanogr. 61, 101–118. doi: 10.1002/lno.10312
Oswald, K., Milucka, J., Brand, A., Littmann, S., Wehrli, B., Kuypers, M. M. M., et al. (2015). Light-dependent aerobic methane oxidation reduces methane emissions from seasonally stratified lakes. PLoS One 10:e0132574. doi: 10.1371/journal.pone.0132574
Peres-Neto, P. R., and Jackson, D. A. (2001). How well do multivariate data sets match? The advantages of a procrustean superimposition approach over the Mantel test. Oecologia 129, 169–178. doi: 10.1007/s004420100720
Picone, N., and Op den Camp, H. J. M. (2019). Role of rare earth elements in methanol oxidation. Curr. Opin. Chem. Biol. 49, 39–44. doi: 10.1016/j.cbpa.2018.09.019
Pol, A., Barends, T. R. M., Dietl, A., Khadem, A. F., Eygensteyn, J., Jetten, M. S. M., et al. (2014). Rare earth metals are essential for methanotrophic life in volcanic mudpots. Environ. Microbiol. 16, 255–264. doi: 10.1111/1462-2920.12249
R Core Team (2020). R: A language and environment for statistical computing. Vienna: R Foundation for Statistical Computing.
Reis, P. C. J., Thottathil, S. D., Ruiz-González, C., and Prairie, Y. T. (2020). Niche separation within aerobic methanotrophic bacteria across lakes and its link to methane oxidation rates. Environ. Microbiol. 22, 738–751. doi: 10.1111/1462-2920.14877
Rissanen, A. J., Saarenheimo, J., Tiirola, M., and Peura, S. (2018). Gammaproteobacterial methanotrophs dominate methanotrophy in aerobic and anaerobic layers of boreal lake waters. Aquat. Microb. Ecol. 81, 257–276. doi: 10.3354/ame01874
Rohart, F., Gautier, B., Singh, A., and Lê Cao, K.-A. (2017). mixOmics: an R package for ‘omics feature selection and multiple data integration. PLoS Comput. Biol. 13:e1005752. doi: 10.1371/journal.pcbi.1005752
Roland, F. A. E., Morana, C., Darchambeau, F., Crowe, S. A., Thamdrup, B., Descy, J. -P., et al. (2018). Anaerobic methane oxidation and aerobic methane production in an east African great lake (Lake Kivu). J. Great Lakes Res. 44, 1183–1193. doi: 10.1016/j.jglr.2018.04.003
Ross, M. O., MacMillan, F., Wang, J., Nisthal, A., Lawton, T. J., Olafson, B. D., et al. (2019). Particulate methane monooxygenase contains only mononuclear copper centers. Science 364, 566–570. doi: 10.1126/science.aav2572
Sanches, L. F., Guenet, B., Marinho, C. C., Barros, N., and de Assis Esteves, F. (2019). Global regulation of methane emission from natural lakes. Sci. Rep. 9, 1–10. doi: 10.1038/s41598-018-36519-5
Sanseverino, A. M., Bastviken, D., Sundh, I., Pickova, J., and Enrich-Prast, A. (2012). Methane carbon supports aquatic food webs to the fish level. PLoS One 7:e42723. doi: 10.1371/journal.pone.0042723
Schubert, C. J., Diem, T., and Eugster, W. (2012). Methane emissions from a small wind shielded lake determined by Eddy covariance, flux chambers, anchored funnels, and boundary model calculations: a comparison. Environ. Sci. Technol. 46, 4515–4522. doi: 10.1021/es203465x
Schubert, C. J., Lucas, F. S., Durisch-Kaiser, E., Stierli, R., Diem, T., Scheidegger, O., et al. (2010). Oxidation and emission of methane in a monomictic lake (Rotsee, Switzerland). Aquat. Sci. 72, 455–466. doi: 10.1007/s00027-010-0148-5
Semrau, J. D., DiSpirito, A. A., Gu, W., and Yoon, S. (2018). Metals and methanotrophy. Appl. Environ. Microbiol. 84, e02289–e02217. doi: 10.1128/AEM.02289-17
Semrau, J. D., DiSpirito, A. A., and Yoon, S. (2010). Methanotrophs and copper. FEMS Microbiol. Rev. 34, 496–531. doi: 10.1111/j.1574-6976.2010.00212.x
Siljanen, H. M. P., Saari, A., Bodrossy, L., and Martikainen, P. J. (2012). Effects of nitrogen load on the function and diversity of methanotrophs in the littoral wetland of a boreal lake. Front. Microbiol. 3:39. doi: 10.3389/fmicb.2012.00039
Singh, R., Ryu, J., and Kim, S. W. (2019). Microbial consortia including methanotrophs: some benefits of living together. J. Microbiol. 57, 939–952. doi: 10.1007/s12275-019-9328-8
Sirajuddin, S., Barupala, D., Helling, S., Marcus, K., Stemmler, T. L., and Rosenzweig, A. C. (2014). Effects of zinc on particulate methane monooxygenase activity and structure. J. Biol. Chem. 289, 21782–21794. doi: 10.1074/jbc.M114.581363
Sirajuddin, S., and Rosenzweig, A. C. (2015). Enzymatic oxidation of methane. Biochemistry 54, 2283–2294. doi: 10.1021/acs.biochem.5b00198
Stein, L. Y. (2020). The long-term relationship between microbial metabolism and greenhouse gases. Trends Microbiol. 28, 500–511. doi: 10.1016/j.tim.2020.01.006
Stock, M., Hoefman, S., Kerckhof, F. -M., Boon, N., De Vos, P., De Baets, B., et al. (2013). Exploration and prediction of interactions between methanotrophs and heterotrophs. Res. Microbiol. 164, 1045–1054. doi: 10.1016/j.resmic.2013.08.006
Sundh, I., Bastviken, D., and Tranvik, L. J. (2005). Abundance, activity, and community structure of pelagic methane-oxidizing bacteria in temperate lakes. Appl. Environ. Microbiol. 71, 6746–6752. doi: 10.1128/AEM.71.11.6746-6752.2005
Tang, K. W., McGinnis, D. F., and Grossart, H. -P. (2016). Methane production in oxic lake waters potentially increases aquatic methane flux to air. Environ. Sci. Technol. Lett. 3, 227–233. doi: 10.1021/acs.estlett.6b00150
Tinberg, C. E., and Lippard, S. J. (2011). Dioxygen activation in soluble methane monooxygenase. Acc. Chem. Res. 44, 280–288. doi: 10.1021/ar1001473
Tsutsumi, M., Iwata, T., Kojima, H., and Fukui, M. (2011). Spatiotemporal variations in an assemblage of closely related planktonic aerobic methanotrophs. Freshw. Biol. 56, 342–351. doi: 10.1111/j.1365-2427.2010.02502.x
Ul-Haque, M. F., Kalidass, B., Vorobev, A. V., Baral, B. S., DiSpirito, A. A., and Semrau, J. D. (2015). Methanobactin from Methylocystis sp. strain SB2 affects gene expression and methane monooxygenase activity in Methylosinus trichosporium OB3b. Appl. Environ. Microbiol. 81, 2466–2473. doi: 10.1128/aem.03981-14
van der Ha, D., Vanwonterghem, I., Hoefman, S., De Vos, P., and Boon, N. (2013). Selection of associated heterotrophs by methane-oxidizing bacteria at different copper concentrations. Antonie Van Leeuwenhoek 103, 527–537. doi: 10.1007/s10482-012-9835-7
van Grinsven, S., Sinninghe Damsté, J. S., Abdala Asbun, A., Engelmann, J. C., Harrison, J., and Villanueva, L. (2020). Methane oxidation in anoxic lake water stimulated by nitrate and sulfate addition. Environ. Microbiol. 22, 766–782. doi: 10.1111/1462-2920.14886
Veraart, A. J., Garbeva, P., Van Beersum, F., Ho, A., Hordijk, C. A., Meima-Franke, M., et al. (2018). Living apart together—bacterial volatiles influence methanotrophic growth and activity. ISME J. 12, 1163–1166. doi: 10.1038/s41396-018-0055-7
Vu, H. N., Subuyuj, G. A., Vijayakumar, S., Good, N. M., Martinez-Gomez, N. C., and Skovran, E. (2016). Lanthanide-dependent regulation of methanol oxidation systems in Methylobacterium extorquens AM1 and their contribution to methanol growth. J. Bacteriol. 198, 1250–1259. doi: 10.1128/JB.00937-15
Wang, Y., Naumann, U., Wright, S. T., and Warton, D. I. (2012). Mvabund—an R package for model-based analysis of multivariate abundance data. Methods Ecol. Evol. 3, 471–474. doi: 10.1111/j.2041-210X.2012.00190.x
Webb, C. O., Ackerly, D. D., Mcpeek, M. A., and Donoghue, M. J. (2002). Phylogenies and community ecology. Annu. Rev. Ecol. Syst. 33, 475–505. doi: 10.1146/annurev.ecolsys.33.010802.150448
Weiss, S., Treuren, W., Van Lozupone, C., Faust, K., Friedman, J., Deng, Y., et al. (2016). Correlation detection strategies in microbial data sets vary widely in sensitivity and precision. ISME J. 10, 1669–1681. doi: 10.1038/ismej.2015.235
Wen, X., Yang, S., and Liebner, S. (2016). Evaluation and update of cutoff values for methanotrophic pmoA gene sequences. Arch. Microbiol. 198, 629–636. doi: 10.1007/s00203-016-1222-8
Xing, Z., Zhao, T., Zhang, L., Gao, Y., Liu, S., and Yang, X. (2018). Effects of copper on expression of methane monooxygenases, trichloroethylene degradation, and community structure in methanotrophic consortia. Eng. Life Sci. 18, 236–243. doi: 10.1002/elsc.201700153
Yu, Z., and Chistoserdova, L. (2017). Communal metabolism of methane and the rare earth element switch. J. Bacteriol. 199, e00328–e00317. doi: 10.1128/JB.00328-17
Zheng, Y., and Chistoserdova, L. (2019). “Multi-omics understanding of methanotrophs” in Methanotrophs: Microbiology fundamentals and biotechnological applications. ed. E. Y. Lee (Cham: Springer International Publishing), 121–138.
Keywords: methanotrophs, methane oxidation, pmoA , bacterial interactions, environmental factors, diversity, habitat specificity
Citation: Guggenheim C, Freimann R, Mayr MJ, Beck K, Wehrli B and Bürgmann H (2020) Environmental and Microbial Interactions Shape Methane-Oxidizing Bacterial Communities in a Stratified Lake. Front. Microbiol. 11:579427. doi: 10.3389/fmicb.2020.579427
Edited by:
David A. Walsh, Concordia University, CanadaReviewed by:
Ludmila Chistoserdova, University of Washington, United StatesSophie Crevecoeur, Environment and Climate Change (Canada), Canada
Copyright © 2020 Guggenheim, Freimann, Mayr, Beck, Wehrli and Bürgmann. This is an open-access article distributed under the terms of the Creative Commons Attribution License (CC BY). The use, distribution or reproduction in other forums is permitted, provided the original author(s) and the copyright owner(s) are credited and that the original publication in this journal is cited, in accordance with accepted academic practice. No use, distribution or reproduction is permitted which does not comply with these terms.
*Correspondence: Carole Guggenheim, carole_guggenheim@hotmail.com; Remo Freimann, remofreimann@gmail.com
†These authors have contributed equally to this work