- 1Department of Oncology, Chinese PLA General Hospital, Beijing, China
- 2Department of Health Management, Chinese PLA General Hospital, Beijing, China
- 3Department of Bio-therapeutic, Chinese PLA General Hospital, Beijing, China
Background: Immune and stromal cells in the tumor microenvironment (TME) significantly contribute to the prognosis of lung adenocarcinoma; however, the TME-related immune prognostic signature is unknown. The aim of this study was to develop a novel immune prognostic model of the TME in lung adenocarcinoma.
Methods: First, the immune and stromal scores among lung adenocarcinoma patients were determined using the ESTIMATE algorithm in accordance with The Cancer Genome Atlas (TCGA) database. Differentially expressed immune-related genes (IRGs) between high and low immune/stromal score groups were analyzed, and a univariate Cox regression analysis was performed to identify IRGs significantly correlated with overall survival (OS) among patients with lung adenocarcinoma. Furthermore, a least absolute shrinkage and selection operator (LASSO) regression analysis was performed to generate TME-related immune prognostic signatures. Gene set enrichment analysis was performed to analyze the mechanisms underlying these immune prognostic signatures. Finally, the functions of hub IRGs were further analyzed to delineate the potential prognostic mechanisms in comprehensive TCGA datasets.
Results: In total, 702 intersecting differentially expressed IRGs (589 upregulated and 113 downregulated) were screened. Univariate Cox regression analysis revealed that 58 significant differentially expressed IRGs were correlated with patient prognosis in the training cohort, of which three IRGs (CLEC17A, INHA, and XIRP1) were identified through LASSO regression analysis. A robust prognostic model was generated on the basis of this three-IRG signature. Furthermore, functional enrichment analysis of the high-risk-score group was performed primarily on the basis of metabolic pathways, whereas analysis of the low-risk-score group was performed primarily on the basis of immunoregulation and immune cell activation. Finally, hub IRGs CLEC17A, INHA, and XIRP1 were considered novel prognostic biomarkers for lung adenocarcinoma. These hub genes had different mutation frequencies and forms in lung adenocarcinoma and participated in different signaling pathways. More importantly, these hub genes were significantly correlated with the infiltration of CD4+ T cells, CD8+ T cells, macrophages, B cells, and neutrophils.
Conclusions: The robust novel TME-related immune prognostic signature effectively predicted the prognosis of patients with lung adenocarcinoma. Further studies are required to further elucidate the regulatory mechanisms of these hub IRGs in the TME and to develop new treatment strategies.
Introduction
Lung cancer is still the leading disease worldwide in terms of the threat to human life and health (1, 2), and lung adenocarcinoma is the most common pathological subtype. Studies in the past decade have reported that tyrosine kinase inhibitors (TKIs) targeting epidermal growth factor receptor (EGFR), anaplastic lymphoma kinase (ALK), and ROS proto-oncogene 1 (ROS1) are potential therapeutic targets for lung adenocarcinoma, upon genotyping (3–5). Molecular-targeted therapy based on these sensitive targets has considerably enhanced overall survival (OS) among patients with lung adenocarcinoma; however, this therapy is not suitable for all patients with lung adenocarcinoma. Furthermore, drug resistance is common among patients receiving molecular-targeted therapy, and their prognosis is poor (6, 7). Nonetheless, numerous studies have led to the advancement of immunotherapy for several cancers, including lung adenocarcinoma. Immunotherapy is different from targeted therapy; it has more durable clinical benefits. Furthermore, some antibodies used for immunotherapy have been successfully approved as first- and second-line treatments for advanced lung adenocarcinoma (8). In particular, the immune system reportedly plays an important role in the pathogenesis and prognosis of lung adenocarcinoma (9). Therefore, it is essential to understand the immune prognostic signature of lung adenocarcinoma.
Previous studies have investigated the prognostic role of immune-related genes (IRGs) in lung adenocarcinoma from the ImmPort database (10, 11); however, this database contains published data on IRGs, thus potentially not accounting for all IRGs. Moreover, these studies have reported no correlation between prognostic factors and OS among certain subgroups of patients with lung adenocarcinoma, indicating that this association is largely unknown. One of the important reasons may be the complex prognostic behavior of tumors; furthermore, when considering the characteristics of IRGs directly associated with tumors, it is also important to focus on the tumor microenvironment (TME) (12). The TME is closely associated with tumorigenesis and patient prognosis (13, 14). Moreover, accumulating evidence indicates that tumor-infiltrating immune cells and stromal cells (15, 16), as the primary nontumor components of the TME, play a significant role in lung cancer prognosis. These findings highlight the importance of understanding the association between the TME and lung adenocarcinoma prognosis. The development of a prognostic model of IRGs based on the TME might provide novel insights into the generation of a more accurate prognostic system.
Accurate management and appropriate personalized therapies for lung adenocarcinoma are required in accordance with prognostic stratification. Moreover, an enhanced understanding of IRGs involved in the TME would help elucidate their regulatory mechanisms in the TME and develop new treatment strategies. With advancements in machine learning, the ESTIMATE algorithm has been used to investigate IRGs in the TME, based on the immune and stromal scores of the TME, and to generate a TME-related immune prognostic model (17). Moreover, this algorithm can effectively predict the prognosis of patients with various cancers. Accordingly, in this study, we determined the immune and stromal scores of tumors using the ESTIMATE algorithm and developed a novel TME-related immune prognostic model of lung adenocarcinoma.
Materials and Methods
Acquisition of TCGA Data
Normalization of RNA sequence data, in terms of level 3 fragments per kilobase of exon per million fragments mapped (FPKM) reads, was performed for 594 samples obtained from The Cancer Genome Atlas (TCGA) database, including 535 adenocarcinoma and 59 normal lung samples, before December 15, 2019. Thereafter, the Ensemble IDs were converted to gene symbols in accordance with human gene annotations. Furthermore, clinical data of lung adenocarcinoma patients were obtained and merged into a single matrix for subsequent analysis. Patients with an incomplete follow-up duration or recorded date of death of any cause were excluded. Finally, 494 lung adenocarcinoma patients with expression profiles and clinical data were included.
Immune Score and Stromal Score in the TME
Estimation of STromal and Immune cells in MAlignant Tumor tissues using Expression data (ESTIMATE) is a tool for predicting and estimating infiltrating immune and stromal cells in tumor tissues based on gene expression profiles. Herein, the ESTIMATE algorithm was used to analyze the characteristics of specific gene expression in immune and stromal cells for each tumor sample to predict their immune and stromal scores. Thereafter, the immune and stromal scores were analyzed using the estimate package in R software.
Screening of Differentially Expressed IRGs
Based on the median immune and stromal scores, patients with lung adenocarcinoma were divided into two groups: high and low immune/stromal score groups. Significant differences in OS between the high and low immune/stromal score groups were analyzed. On the basis of the significant differences in patient prognosis, differentially expressed IRGs between the two groups were assessed using the Limma package in R software. Finally, intersecting differentially expressed IRGs in both groups were considered for further analysis. A log(fold change) of >2 and an adjusted p-value of <0.05 were considered cutoffs. Heat maps and Venn diagrams were generated using R.
GO and KEGG Pathway Enrichment Analyses of Differentially Expressed IRGs
Gene Ontology (GO) and Kyoto Encyclopedia of Genes and Genomes (KEGG) pathway enrichment analyses were performed to understand gene functional annotation and functional enrichment, respectively. Common differentially expressed IRGs of GO and KEGG annotation were performed using Database for Annotation, Visualization, and Integrated Discovery (DAVID), an online database (https://david.ncifcrf.gov/), to predict functional domains and their biological implications. Fisher's exact test was performed to analyze pathways, diseases, and functions. A p-value of <0.05 indicated the significance of GO terms and KEGG pathway enrichment in genes herein. Furthermore, the top 10 GO terms and KEGG pathway enrichment results were mapped using Hmisc and ggplot2 in R software.
Construction of Prognostic Model for Lung Adenocarcinoma in the Training Set
A total of 494 patients with lung adenocarcinoma in the TCGA dataset were randomly divided into a training set and testing set at a ratio of 7:3 (training cohort, 346 patients; testing cohort, 148 patients).
First, univariate Cox proportional hazard regression analysis was performed to screen out prognostic IRGs in the training cohort (iteration = 1,000), with p < 0.05 indicating statistical significance. Second, the key IRGs were further selected from among significant prognostic IRGs on univariate analysis, through least absolute shrinkage and selection operator (LASSO) regression, a powerful tool for developing refined prognostic models, fitting generalized linear models, selecting variables, and regularizing complexity, using R software. Key IRGs were subjected to multivariate Cox regression analysis. Finally, the risk score formula was developed in accordance with the key IRGs identified through LASSO analysis.
Evaluation of the Prognostic Model in the Training Set
After the expression value of each specific gene was included, the risk score formula for each patient was weighted by its estimated regression coefficient on LASSO regression analysis. On the basis of the best separation of risk score, patients were divided into high-risk-score and low-risk-score groups. Survival differences between the two groups were assessed by Kaplan–Meier survival curves using log-rank tests. ROC curves were used to assess the accuracy of model prediction. Furthermore, LASSO regression analysis was performed to examine the role of the risk score in predicting clinical outcomes. Furthermore, the association between risk score and clinical stage was analyzed.
To further determine whether the independent prognostic model could be used as an independent prognostic factor, univariate and multivariate Cox regression analyses were performed to analyze the predictive value of age, sex, stage, TNM stage, and predictive model. In the univariate analysis, the correlation between some independent variables and dependent variables was considered, and some correlations might be masked by the influence of confounding factors. To avoid omitting important predictors of prognosis, the threshold in univariate analysis was relaxed to p < 0.1, while the p-value in multivariate analysis was still 0.05.
GSEA Analysis of Differences in Pathway Enrichment in the Training Set
To investigate the differences in the putative mechanism between the high-risk-score and low-risk-score groups, gene set enrichment analysis (GSEA) was performed to comprehensively analyze the differences in function enrichment. GSEA is a computational method of determining whether an a priori defined set of genes is significantly different between two biological states. The number of permutations was set to 1,000, and the permutation type was set to phenotype. In this study, all genes in the training set were sequenced according to the degree of differential expression in the high-risk-score group and the low-risk-score group. GSEA was used to comprehensively analyze differences in gene pathway enrichment between the two groups.
Validation of the Prognostic Model in the Testing Cohort
The prognostic model was further validated for the testing cohort (n = 148).
Similarly, the risk score of each patient was weighted on the basis of the risk score. Thereafter, based on the best separation of the risk score, patients in the testing cohort were divided into high-risk-score and low-risk-score groups. A Kaplan–Meier survival curve and ROC curve analysis were performed in the testing however.
Functional Analysis of IRG Signatures in the Model
To further analyze the mutation characteristics and the putative functional mechanisms of these hub IRGs in lung adenocarcinoma, gene expression profiles of patients with lung adenocarcinoma were imported from the following datasets: TCGA database (Broad, Cell 2012), Lung Adenocarcinoma (MSKCC, Science 2015), Lung Adenocarcinoma (TCGA, Firehose Legacy), Lung Adenocarcinoma (TSP, Nature 2008), and Non-Small-Cell Cancer (MSKCC, Cancer Discov 2017). A combined study of five datasets including 1,825 patients were included in this study.
GSCALite is an online cancer genomic analysis tool that integrates cancer genomics data for 33 cancer types from the TCGA and normal tissue data from GTEx (http://bioinfo.life.hust.edu.cn/web/GSCALite/), enabling gene set pathway analysis in data analysis. In this study, GSCALite was used to analyze the pathway of hub genes.
The TIMER database is a comprehensive tool for analyzing the immune cell infiltrates in tumors. The abundances of six immune infiltrates (B cells, CD4+ T cells, CD8+ T cells, neutrophils, macrophages, and dendritic cells) were estimated using the TIMER algorithm. In this study, the TIMER database was further applied to analyze the correlation between hub genes and immune cells. The correlation of hub gene expression with immune infiltration level was visualized in lung adenocarcinoma using the Gene module. The scatterplots were generated and displayed after hub genes and cancer type were submitted successfully, showing the purity-corrected partial Spearman's correlation and statistical significance.
Statistical Analysis
All statistical analyses were conducted with the R language (version 3.6.1). All statistical tests were bilateral, and p < 0.05 was statistically significant.
Results
Study Design and Workflow Overview
A total of 594 samples were obtained, including 535 adenocarcinoma and 59 normal lung samples. Data for a total of 494 lung adenocarcinoma patients with clinical information were retrieved (Table 1). The workflow for constructing and verifying the immune-related prognostic model is shown in Figure 1.
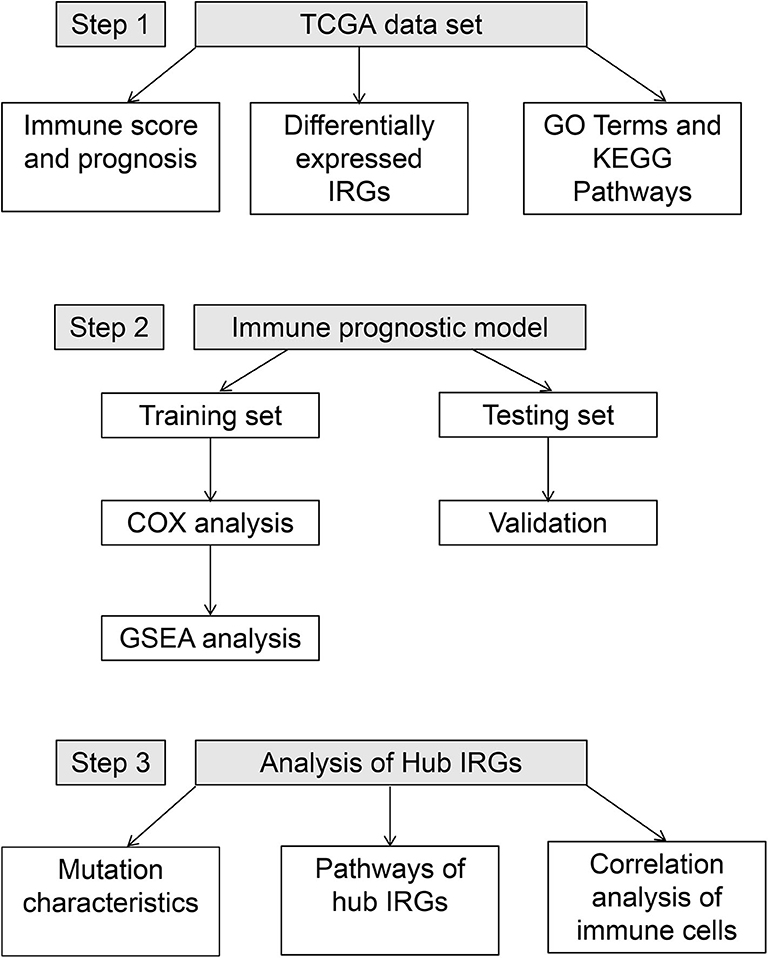
Figure 1. Workflow of the construction and verification of the immune-stromal score prognostic signature.
Immune Score and Stromal Score in the TME
The immune and stromal scores were analyzed using the ESTIMATE algorithm. The immune and stromal scores of lung adenocarcinoma are shown in Supplementary Table 1. On the basis of the median value of immune and stromal scores, patients with lung adenocarcinoma were divided into two groups: the high immune/stromal score group and the low score group. These results show that the high immune score group had better OS than the low immune score group for lung adenocarcinoma. In terms of stromal score, although patients with high stromal score had better prognosis than those with low stromal score, the difference was not statistically significant (Figures 2A–C).
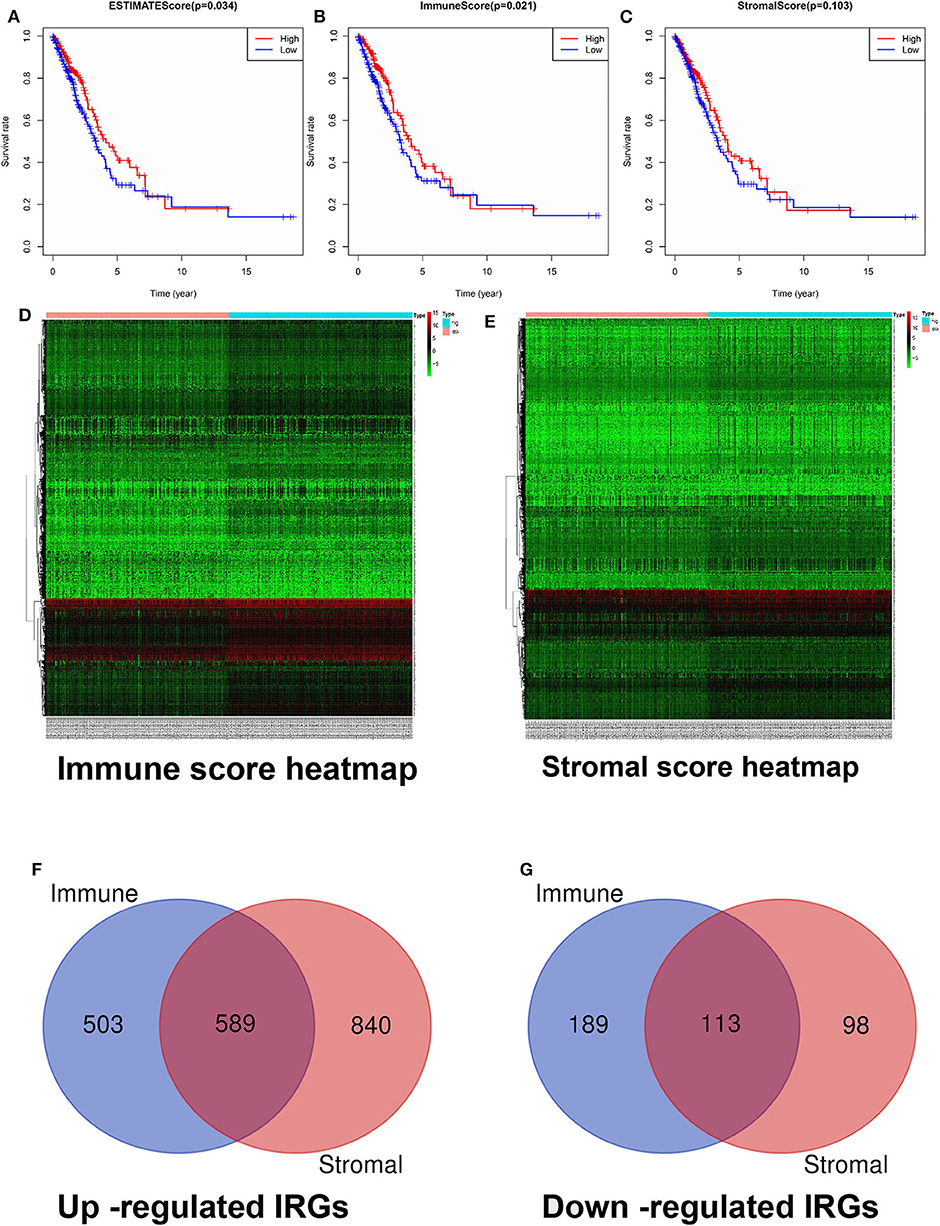
Figure 2. Screening and identification of differentially expressed IRGs. (A) Relationship between comprehensive immune score and OS. (B) Relationship between immune score and OS. (C) Relationship between stromal score and OS. (D) Heatmap of immune score groups. (E) Heatmap of stromal score groups. (F) The Venn diagram of the intersection of up-regulated IRGs between the immune and stromal score groups. (G) The Venn diagram of the intersection of down-regulated IRGs between the immune and stromal score groups. OS, overall survival; IRGs, immune-related genes.
Screening of Differentially Expressed IRGs
According to the criteria of a log(fold change) of >2 and an adjusted p-value of <0.05, our results showed that a total of 3,034 genes with significant differentially expressed IRGs were screened, including 2,521 upregulated IRGs and 513 downregulated IRGs. Among them, 1,394 differentially expressed IRGs (1,092 upregulated IRGs and 302 downregulated IRGs) were included in the immune score group (Supplementary Table 2), and 1,640 differentially expressed IRGs (1,429 upregulated IRGs and 211 downregulated IRGs) were included in the stromal score group (Supplementary Table 3). Heat maps and Venn diagrams are displayed in Figures 2D–G. In total, 702 intersecting differentially expressed IRGs (589 upregulated and 113 downregulated) in both groups are indicated in Figures 2F,G (Supplementary Table 4).
GO Terms and KEGG Pathway Enrichment Analysis of Differentially Expressed IRGs
GO terms are divided into three parts: biological processes, cellular components, and molecular functions. GO analysis showed that upregulated IRGs were mainly involved in the immune response (BP, GO: 0006955), external side of plasma membrane (CC, GO: 0009897), and antigen binding (MF, GO: 0003823). The downregulated IRGs were mainly involved in the cellular response to jasmonic acid stimulus (BP, GO: 0071395), neuronal cell body (CC, GO: 0043025), and oxidoreductase activity, and in acting on NAD(P)H, quinone or similar compounds as acceptors (MF, GO: 0016655) (Figures 3A–C,E; Table 2). The KEGG pathway enrichment results in the upregulated IRGs were mainly involved in cytokine–cytokine receptor interactions, chemokine signaling pathways, and cell adhesion molecules (CAMs). However, the KEGG pathway enrichment results of downregulated IRGs were mainly involved in arachidonic acid metabolism, metabolic pathways, and tyrosine metabolism (Figures 3D,F; Table 3).
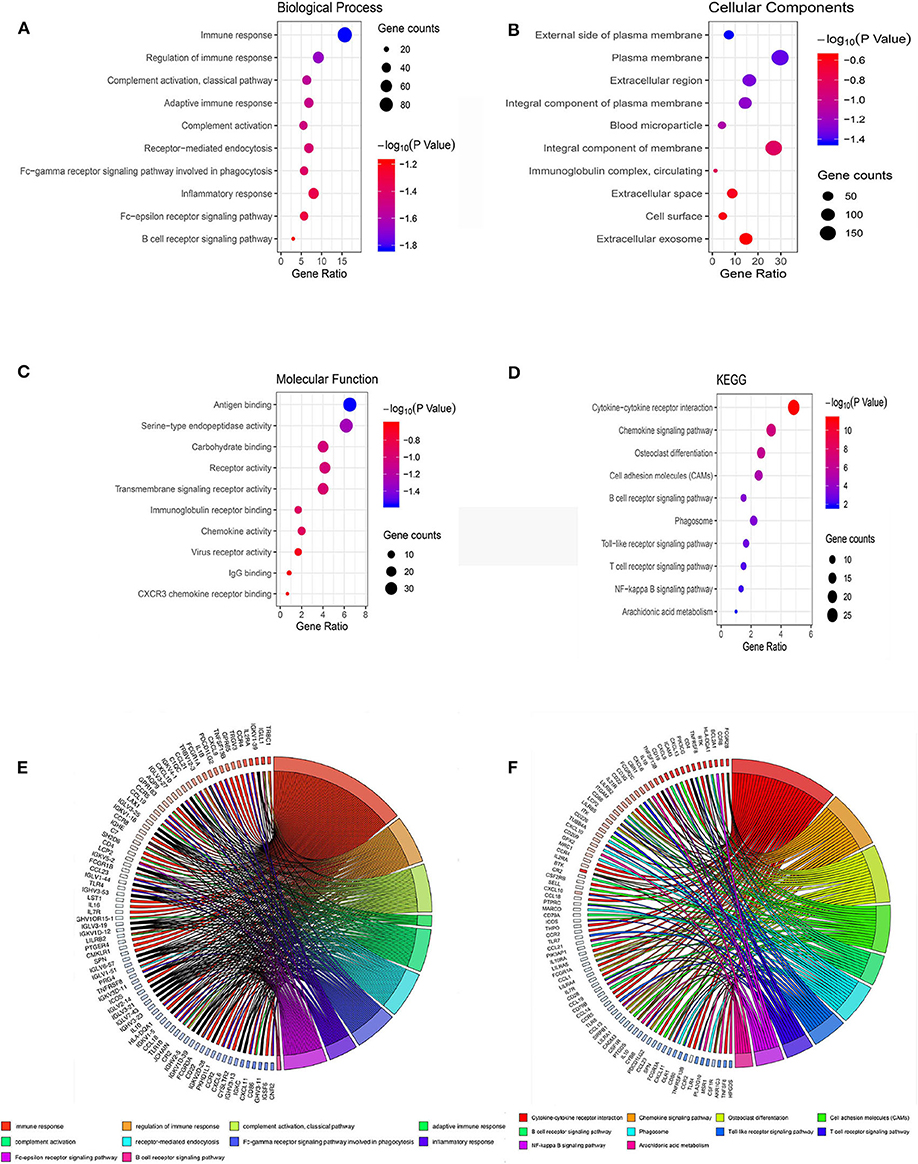
Figure 3. Bubble map of the top 10 GO terms and KEGG pathway enrichment analysis data of differentially expressed IRGs. (A) GO analysis of differentially expressed IRGs in biological processes. (B) GO analysis of differentially expressed IRGs in cellular components. (C) GO analysis of differentially expressed IRGs in terms of molecular function. (D) KEGG enrichment analysis of differentially expressed IRGs. A high gene ratio represents a high level of enrichment. The size of the dot indicates the number of target genes in the pathway, and the color of the dot reflects the p-value range. (E) GO chord plot of differentially expressed IRGs. (F) KEGG chord plot of differentially expressed IRGs.
Development of a Prognostic Model for the Training Cohort
To generate a prognostic model for lung adenocarcinoma, univariate regression analysis was first performed to screen the key prognostic genes in the training cohort. Thereafter, 58 significantly differentially expressed IRGs correlated with prognosis were considered for LASSO regression analysis. Finally, three key IRGs (CLEC17A, INHA, and XIRP1) were selected to generate an immune prognostic model. The results of the multivariate Cox regression analysis are summarized in Table 4. The risk score was determined using the following formula:
[Expression level of CLEC17A × (−0.13549042)] + [Expression level of INHA × (0.01207179)] + [Expression level of XIRP1 × (0.6263501)].
Evaluation of the Prognostic Model in the Training Cohort
LASSO regression analysis was performed to construct and evaluate the prognostic model (Figures 4A,B). Patients were divided into high- and low-risk-score groups in accordance with the best separation of risk scores. The high-risk-score group had significantly worse OS than the low-risk-score group (p < 0.0001; Figure 4C). The area under the ROC curve for predicting the 1-, 3-, and 5-year survival of lung adenocarcinoma was 0.699, 0.631, and 0.669, respectively (Figure 4D).
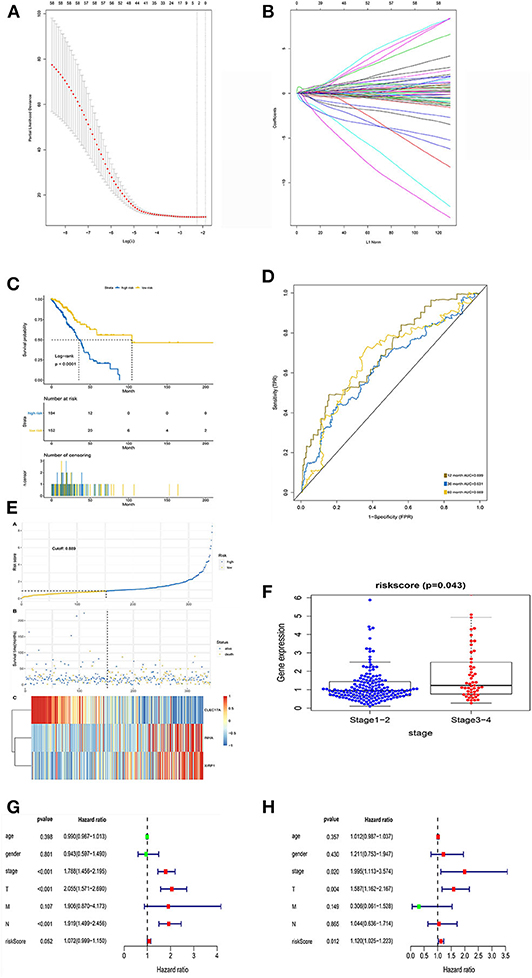
Figure 4. LASSO regression analysis and identification of prognostic signatures in the training set. (A) Ten-fold cross-validation for turning parameter selection in the LASSO Cox regression model. (B) Coefficient profiles in the LASSO Cox regression model. (C) Survival curve of low- and high-risk groups stratified by immune-stromal score signature. (D) ROC analysis of the TCGA dataset for prognostic signature. (E) LASSO regression analysis of low- and high-risk groups. (F) Relationship between risk score and clinical stage. (G) The forest plot of the prognostic signature by univariate analysis. (H) The forest plot of the prognostic signature by multivariate Cox proportional regression analysis.
Additionally, the risk curve indicated that the high-risk-score group had a higher mortality and worse prognosis than the low-risk-score group (cutoff value: 0.889; Figure 4E). Further analysis of the relationship between risk score and pathological stage revealed that patients with early-stage lung adenocarcinoma (stages 1 and 2) scored lower than those with advanced stage lung adenocarcinoma (p = 0.043; Figure 4F).
Univariate Cox analysis showed that pathological staging and risk score had statistical significance, while age and sex had no statistical significance (Figure 4G). However, multivariate Cox analysis showed that pathological stage (HR, 1.995; 95% CI, 1.113–3.574; p = 0.020) and risk score (HR, 1.120; 95% CI, 1.025–1.223; p = 0.012) were independent prognostic factors (Figure 4H).
GSEA of the Mechanism Underlying the Prognostic Differences Between the Two Groups
In this study, the possible molecular mechanisms of the prognosis difference between the two groups of patients were analyzed by GSEA analysis. The results showed that the GO and pathway enrichment in the high-risk-score group was mainly involved in metabolism-related pathways (Figures 5A,C). However, GO and pathway enrichment in the low-risk-score group was primarily focused on immunoregulation and immune cell activation (Figures 5B,D). The detailed GSEA results are described in Table 5.
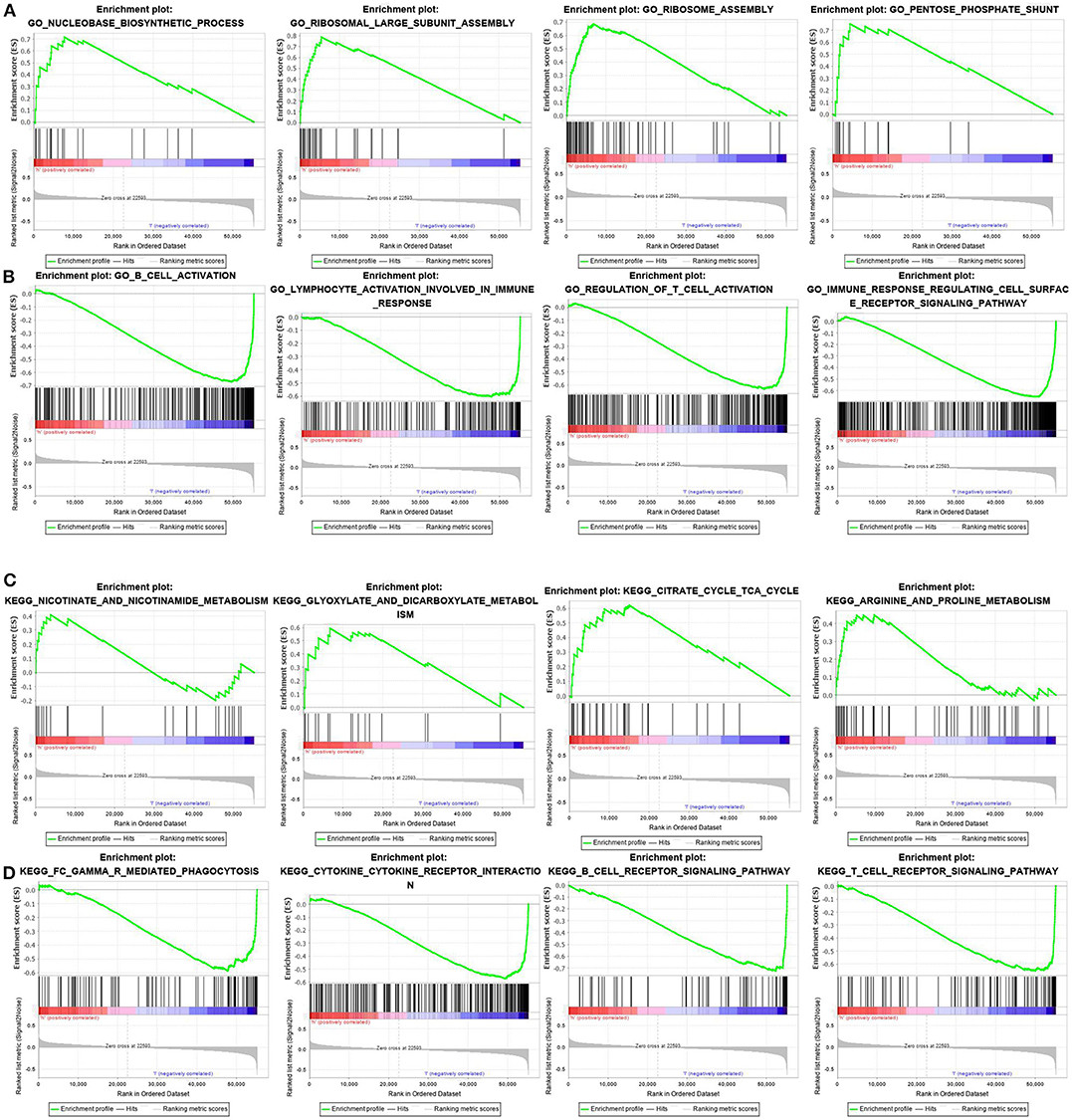
Figure 5. GSEA analysis of differences in pathway enrichment in the training set. (A,B) GO terms in the high- and low-risk score groups. (C,D) KEGG pathway enrichment in the high- and low-risk score groups.
Validation of the Prediction Model in the Testing Cohort
The Kaplan–Meier results showed that the high-risk group had worse OS than the low-risk group (p < 0.0001) (Figure 6A). The area under the ROC curve for predicting the 1-, 3-, and 5-year survival of lung adenocarcinoma was 0.725, 0.712, and 0.660, respectively (Figure 6B). Additionally, risk curve revealed that the high-risk-score group had a worse prognosis than the low-risk-score group (Figure 6C). These results were consistent with the results of the training set.
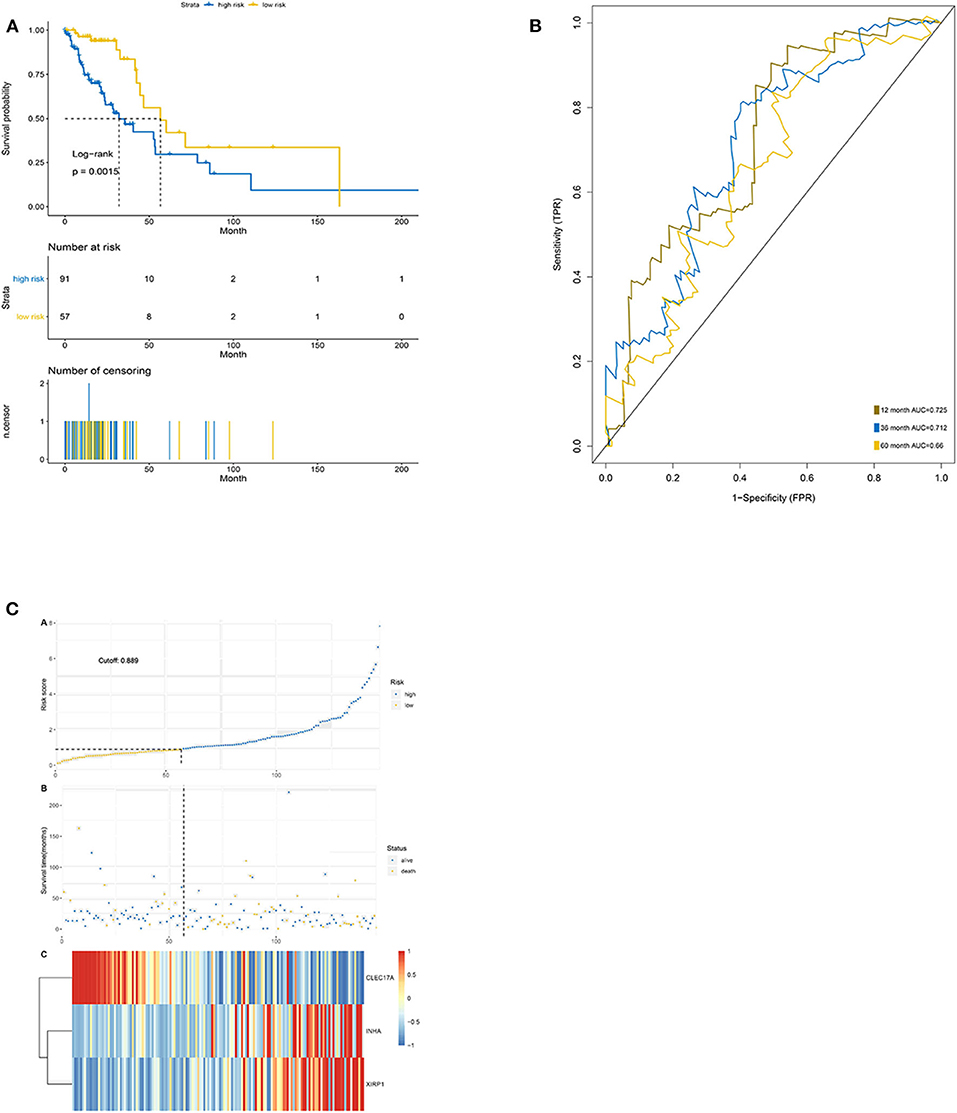
Figure 6. Validation of the prediction features. (A) Survival curve of low- and high-risk groups stratified by immune-stromal score signature. (B) ROC analysis of the TCGA dataset for prognostic signature. (C) LASSO regression analysis of low- and high-risk groups.
The Mechanism of Action of Hub IRGs in the TCGA Database
To further analyze the potential function of hub IRGs, our results were verified using the TCGA and GTEx databases. First, the mutation characteristics of these hub IRGs were analyzed among patients with lung adenocarcinoma. The mutation rates of these hub IRGs in patients with lung adenocarcinoma were 0.8, 1, and 2.6% (Figure 7A). Moreover, each hub gene had different mutation forms, including mutation, deletion, and amplification, in lung adenocarcinoma. For example, the mutation form of CLEC17A was mainly amplification, the mutation form of INHA was mainly amplification and missense mutation, while the mutation form of XIRP1 was mainly deep deletion and missense mutation (Figure 7B).
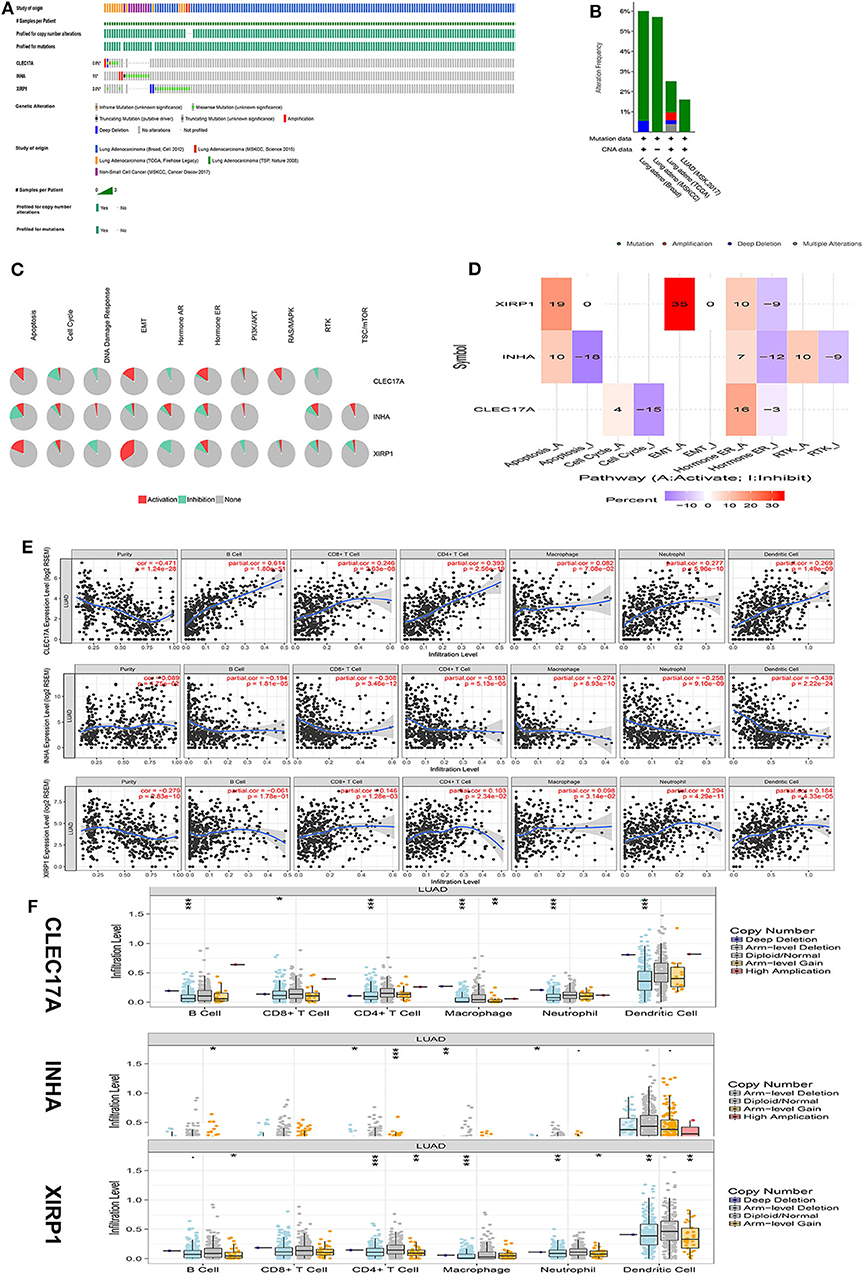
Figure 7. Mechanism analysis of hub genes in the TCGA database. (A) Matrix heat map shows genomic alterations of hub genes in five lung datasets. (B) The alteration frequencies of hub genes across five studies on lung adenocarcinoma. (C) The pathways of hub genes were analyzed by the GSCALite tool. (D) Heatmap percentage of hub genes. (E) The correlation between the hub gene and immune cells was analyzed in the TIMER database. (F) The relationship between copy number variation (CNV) of hub genes and immune cell infiltration was further analyzed.
In addition, analysis of pathways in the GSCALite database revealed that CLEC17A is primarily involved in the activation of the epithelial–mesenchymal transition (EMT) and RAS pathways and cell cycle inhibition. INHA is primarily involved in the activation of the mTOR pathway and inhibition of the apoptosis pathway. XIRP1 was mainly involved in the activation of the apoptosis and the EMT pathways and inhibition of the DNA damage and the PI3K pathways (Figures 7C,D).
Finally, the TIMER database was used to analyze the correlation between hub IRGs and immune cells. The results showed that these key genes were significantly correlated with the infiltration of CD4+ T cells, CD8+ T cells, macrophages, B cells, and neutrophils. Assuming that a correlation coefficient >0.3 was considered a strong correlation, further analysis showed that CLEC17A was positively correlated with the infiltration of B cells and CD4+ T cells. However, INHA was negatively correlated with the infiltration of CD8+ T and dendritic cells. However, there was no strong correlation between XIRP1 and the infiltration of immune cells (Figure 7E). Moreover, the relationship between copy number variation (CNV) of hub IRGs and immune cell infiltration was further analyzed. The results showed that there were significant differences between the CNV of these hub IRGs and immune cell infiltration. Arm-level deletion of the CLEC17A gene was closely related to the infiltration of B cells, CD4+ T cells, macrophages, neutrophils, and dendritic cells. Arm-level gain of the INHA gene was closely related to the infiltration of CD4+ T cells. Arm-level deletion of the XIRP1 gene was closely related to the infiltration of CD4+ T cells and macrophages (Figure 7F).
Discussion
Tumor-infiltrating immune cells in the TME significantly contribute to the prognosis of lung adenocarcinoma. Therefore, it is essential to develop a TME-related immune prognostic model for appropriate clinical management of lung adenocarcinoma. Accordingly, the aim of this study was to develop a novel TME-related immune prognostic model for lung adenocarcinoma.
Although some studies have explored the prognostic value of TME-related IRGs in lung adenocarcinoma, certain key issues of these models remain to be resolved.
Yang et al. (18) used the CIBERSORT algorithm to analyze a TME-related prognostic immunity-based model for lung adenocarcinoma. This model was developed to evaluate the relative levels of the 22 immune cell phenotypes, primarily including B cells, T cells, macrophages, dendritic cells, plasma cells, natural killer cells, and mast cells. Moreover, this algorithm is primarily used to evaluate immune cells; however, it cannot be used to evaluate stromal cells in the TME. Yue et al. (19) used the ESTIMATE algorithm to investigate the TME-related immune prognostic characteristics of lung adenocarcinoma. However, they directly enumerated immune and stromal cells from their expression profiles of all genes expressed in lung adenocarcinoma and normal tissues. Moreover, differentially expressed IRGs were analyzed using Wilcoxon correlation analysis between tumor and normal tissue. However, some limitations are associated with the analysis of multi-dimensional tumor gene expression profiles through the Wilcoxon rank-sum test. These findings indicate that these TME-related prognostic immunity-based models have not been adequately evaluated. These issues can be resolved primarily by improving the algorithm of IRGs in the TME and to identify more specific TME-related IRGs for lung adenocarcinoma. Therefore, it is essential to develop a new TME-related immune prognostic model for lung adenocarcinoma.
To address these aforementioned limitations, in the present study, a new method was developed to identify differentially expressed IRGs. First, TME-related differentially expressed IRGs were identified exclusively from tumor samples by evaluating tumor-infiltrating immune cells and stromal cells via the ESTIMATE algorithm; this probably effectively reflected the TME-related IRGs in tumor tissue. Second, differentially expressed IRGs were analyzed on the basis of significant differences in OS between the high- and low-immune-score groups in terms of lung adenocarcinoma, rather than differences between lung adenocarcinoma and normal tissue using the Wilcoxon rank-sum test. The prognostic model, based on prognosis-associated differentially expressed genes, might more accurately predict the prognosis of lung adenocarcinoma. Finally, intersecting differentially expressed IRGs with significant prognostic characteristics in both immune and stromal scores were used for subsequent analysis. Both immune cells and stromal cells in each tumor sample were assessed, thus better reflecting the characteristics of the TME. Therefore, the TME-related immune prognostic model developed herein was different from those developed previously. In this study, we developed a more robust prognostic model of TME-related IRGs in lung adenocarcinoma.
Furthermore, this study shows that patients with lung adenocarcinoma and high immune scores had a better prognosis than those with low immune scores, which might be due to the involvement of upregulated IRGs in immune cell infiltration factors, such as cytokines and B cell immune pathways. Clinical studies have also shown that lung cancer patients with high immune infiltration of helper T cells have a better prognosis than those with low infiltration (20, 21). These findings were consistent with our results. Previous studies have suggested that IL-2 is involved in antitumor T cell infiltration, increasing the efficacy of immunotherapy (22). IL-33 also promotes myeloid-derived suppressor cells (MDSCs) and interferes with CD8+ T and natural killer (NK) cell infiltration (23). These studies have suggested that certain cytokines are involved in antitumor immune pathways, potentially elucidating the mechanisms associated with prognosis.
Moreover, GSEA was performed to further investigate the potential mechanism underlying the differences in prognosis between the two groups. The present results indicate that the immunoregulation and immune cell activation pathways are potentially associated with a better prognosis. The underlying putative mechanism potentially involves the enrichment of B and T cell immune pathways. Furthermore, the infiltration of these immune cells is associated with an enhanced prognosis among patients with lung adenocarcinoma. Our results are concurrent with those of the aforementioned studies.
Furthermore, this study described the functional prediction of potential hub IRGs. An enhanced understanding of these potential hub genes is essential to elucidate their mechanisms of action in the TME in lung adenocarcinoma. The present results suggest that although these genes were prognosis-related IRGs, they harbored different mutations involved in different pathways in lung adenocarcinoma, indicating their potential involvement in different immunoregulatory pathways in lung adenocarcinoma. Further analysis of the function of these genes revealed that these hub genes and their CNVs were different. Moreover, the association between these hub genes and the infiltration of B cells, CD4+ T cells, CD8+ T cells, neutrophils, and other immune cells was also different. These results indicate that different CNVs of these hub genes warrant further differentiation to better understand the association between hub genes and immune cell infiltration. Based on the aforementioned results, our results indicate that CLEC17A, INHA, and XIRP1 are potential novel biomarkers for the prognosis of lung adenocarcinoma.
CLEC17A is a human lectin found in lymph node B cells and is involved in a variety of biological processes, including cell adhesion, intercellular interactions, and pathogen recognition (24). Previous studies have shown that CLEC17A is related to the B cell receptor signaling pathway and plays an important role in the pathogenesis of chronic lymphocytic leukemia (25). The present results further indicate that CLEC17A is associated with immune cell infiltration in lung adenocarcinoma, concurrent with previous reports. XIRP1 is a striated muscle protein and belongs to the Xin actin-binding repeat-containing protein (XIRP) family. Previous studies have shown that the XIRP1 gene is related to hypertension and nervous system development (26, 27). The function of the gene has not been reported in tumors. However, our study showed that it was not only related to the TME in lung adenocarcinoma but is also related to the prognosis of lung adenocarcinoma. INHA encodes a member of the transforming growth factor-beta (TGF-beta) superfamily of proteins. The function of the gene has not been reported in lung cancer. Our results showed that INHA was a marker of poor prognosis in lung adenocarcinoma. The possible mechanism was that INHA was involved in tumor angiogenesis, leading to tumor metastasis and poor prognosis (28). Further studies are required to elucidate the roles of these hub genes in the TME in the pathogenesis of lung adenocarcinoma.
This study also had some limitations. First, this study only mined data in the TCGA database and did not combine GEO database analysis. However, in our study, patient data were segregated into training and testing cohorts. In addition, the results were verified and analyzed in comprehensive TCGA and GSCALite datasets. Second, the function of the hub gene in our study was analyzed based on the TCGA database, and the validation function of the hub gene needs to be further confirmed by basic experiments. Constructing an immune-related prognosis model was the focus of our research; hence, there was no basic experiment on hub prognostic genes. Third, our study only analyzed the correlation of differentially expressed IRGs with immune cell infiltration, thus lacking the correlation analysis of the expression of PDL1 and tumor mutational burden.
Conclusions
The robust TME-related immune prognostic model developed herein effectively predicted the prognosis of patients with lung adenocarcinoma, thus potentially guiding personalized treatment of lung adenocarcinoma in accordance with prognostic stratification. Further studies are required to elucidate the regulatory mechanisms of these IRGs in the TME and develop new treatment strategies.
Data Availability Statement
Publicly available datasets were analyzed in this study. This data can be found here: https://portal.gdc.cancer.gov/.
Author Contributions
XQ designed the study, analyzed the data, and drafted the paper. CQ critically revised it for important intellectual content. BQ and XK assisted in data acquisition and analysis. YH and WH revised the manuscript. All authors read and approved the final version of the manuscript.
Funding
The authors received no funding for this work.
Conflict of Interest
The authors declare that the research was conducted in the absence of any commercial or financial relationships that could be construed as a potential conflict of interest.
Acknowledgments
The authors would like to thank TCGA databases for the availability of the data.
Supplementary Material
The Supplementary Material for this article can be found online at: https://www.frontiersin.org/articles/10.3389/fonc.2020.541330/full#supplementary-material
Supplementary Table 1. Immune-Stromal score of lung adenocarcinoma.
Supplementary Table 2. Differential IRGs estimated by immune score.
Supplementary Table 3. Differential IRGs estimated by stromal score.
Supplementary Table 4. Intersection of differential IRGs.
Abbreviations
IRGs, immune-related genes; TME, tumor microenvironment; TCGA, The Cancer Genome Atlas data; DAVID, Database for Annotation, Visualization and Integrated Discovery.
References
1. Romaszko AM, Doboszyńska A. Multiple primary lung cancer: a literature review. Adv Clin Exp Med. (2018) 27:725–30. doi: 10.17219/acem/68631
2. Schneider BJ, Ismaila N, Altorki N. Lung cancer surveillance after definitive Curative-Intent therapy: ASCO guideline summary. JCO Oncol Pract. (2020) 16:83–6. doi: 10.1200/JOP.19.00722
3. Singhi EK, Horn L, Sequist LV, Heymach J, Langer CJ. Advanced non-small cell lung cancer: sequencing agents in the EGFR-mutated/ALK-rearranged populations. Am Soc Clin Oncol Educ Book. (2019) 39:e187–e97. doi: 10.1200/EDBK_237821
4. Possidente L, Landriscina M, Patitucci G, Borgia L, Lalinga V, Vita G. ALK rearrangement in specific subtypes of lung adenocarcinoma: immunophenotypic and morphological features. Med Oncol. (2017) 34:76. doi: 10.1007/s12032-017-0936-z
5. Sgambato A, Casaluce F, Maione P, Gridelli C. Targeted therapies in non-small cell lung cancer: a focus on ALK/ROS1 tyrosine kinase inhibitors. Expert Rev Anticancer Ther. (2018) 18:71–80. doi: 10.1080/14737140.2018.1412260
6. Nasim F, Sabath BF, Eapen GA. Lung cancer. Med Clin North Am. (2019) 103:463–73. doi: 10.1016/j.mcna.2018.12.006
7. Woodard GA, Jones KD, Jablons DM. Lung cancer staging and prognosis. Cancer Treat Res. (2016) 170:47–75. doi: 10.1007/978-3-319-40389-2_3
8. Dafni U, Tsourti Z, Vervita K, Peters S. Immune checkpoint inhibitors, alone or in combination with chemotherapy, as first-line treatment for advanced non-small cell lung cancer. A systematic review and network meta-analysis. Lung Cancer. (2019) 134:127–40. doi: 10.1016/j.lungcan.2019.05.029
9. Varn FS, Tafe LJ, Amos CI, Cheng C. Computational immune profiling in lung adenocarcinoma reveals reproducible prognostic associations with implications for immunotherapy. Oncoimmunology. (2018) 7:e1431084. doi: 10.1080/2162402X.2018.1431084
10. Guo D, Wang M, Shen Z, Zhu J. A new immune signature for survival prediction and immune checkpoint molecules in lung adenocarcinoma. J Transl Med. (2020) 18:123. doi: 10.1186/s12967-020-02286-z
11. Song Q, Shang J, Yang Z, Zhang L, Zhang C, Chen J, et al. Identification of an immune signature predicting prognosis risk of patients in lung adenocarcinoma. J Transl Med. (2019) 17:70. doi: 10.1186/s12967-019-1824-4
12. Wood SL, Pernemalm M, Crosbie PA, Whetton AD. The role of the tumor-microenvironment in lung cancer-metastasis and its relationship to potential therapeutic targets. Cancer Treat Rev. (2014) 40:558–66. doi: 10.1016/j.ctrv.2013.10.001
13. Altorki NK, Markowitz GJ, Gao DC, Port JL, Saxena A, Stiles B, et al. The lung microenvironment: an important regulator of tumour growth and metastasis. Nat Rev Cancer. (2019) 19:9–31. doi: 10.1038/s41568-018-0081-9
14. Bonanno L, Zulato E, Pavan A, Attili I, Pasello G, Conte P, et al. LKB1 and tumor metabolism: the interplay of immune and angiogenic microenvironment in lung cancer. Int J Mol Sci. (2019) 20:1874. doi: 10.3390/ijms20081874
15. Guo XY, Zhang YY, Liangtao Zheng LT, Zheng CH, Song JT, Zhang QM, et al. Global characterization of T cells in non-small-cell lung cancer by single-cell sequencing. Nat Med. (2018) 24:978–85. doi: 10.1038/s41591-018-0045-3
16. Stankovic B, Bjørhovde HA, Skarshaug R, Aamodt H, Frafjord A, Müller E, et al. Immune cell composition in human non-small cell lung cancer. Front Immunol. (2018) 9:3101. doi: 10.3389/fimmu.2018.03101
17. Jia D, Li SL, Li D, Xue HP, Yang D, Liu Y. Mining TCGA database for genes of prognostic value in glioblastoma microenvironment. Aging (Albany NY). (2018) 10:592–605. doi: 10.18632/aging.101415
18. Yang XD, Shi Y, Li M, Lu T, Xi JJ, Lin ZW, et al. Identification and validation of an immune cell infiltrating score predicting survival in patients with lung adenocarcinoma. J Transl Med. (2019) 17:217. doi: 10.1186/s12967-019-1964-6
19. Yue C, Ma H, Zhou Y. Identification of prognostic gene signature associated with microenvironment of lung adenocarcinoma. Peer J. (2019) 7:e8128. doi: 10.7717/peerj.8128
20. Liu X, Wu S, Yang Y, Zhao M, Zhu G, Hou Z. The prognostic landscape of tumor-infiltrating immune cell and immunomodulators in lung cancer. Biomed Pharmacother. (2017) 95:55–61. doi: 10.1016/j.biopha.2017.08.003
21. Sun J, Zhang Z, Bao S, Yan C, Hou P, Wu N, et al. Identification of tumor immune infiltration-associated lncRNAs for improving prognosis and immunotherapy response of patients with non-small cell lung cancer. J Immunother Cancer. (2020) 8:e000110. doi: 10.1136/jitc-2019-000110
22. Moynihan KD, Opel CF, Szeto GL, Tzeng A, Zhu EF, Engreitz JM, et al. Eradication of large established tumors in mice by combination immunotherapy that engages innate and adaptive immune responses. Nat Med. (2016) 22:1402–10. doi: 10.1038/nm.4200
23. Shen JX, Liu J, Zhang GJ. Interleukin-33 in malignancies: friends or foes? Front Immunol. (2018) 9:3051. doi: 10.3389/fimmu.2018.03051
24. Larsen FT, Bed'Hom B, Guldbrandtsen B, Dalgaard TS. Identification and tissue-expression profiling of novel chicken c-type lectin-like domain containing proteins as potential targets for carbohydrate-based vaccine strategies. Mol Immunol. (2019) 114:216–25. doi: 10.1016/j.molimm.2019.07.022
25. Johnston HE, Carter MJ, Larrayoz M, Clarke J, Garbis SD, Oscier D, et al. Proteomics profiling of CLL versus healthy B-cells identifies putative therapeutic targets and a subtype-independent signature of spliceosome dysregulation. Mol Cell Proteomics. (2018) 17:776–91. doi: 10.1074/mcp.RA117.000539
26. Ikawa T, Watanabe Y, Okuzaki D, Goto N, Okamura N, Yamanishi K, et al. A new approach to identifying hypertension-associated genes in the mesenteric artery of spontaneously hypertensive rats and stroke-prone spontaneously hypertensive rats. J Hypertens. (2019) 37:1644–56. doi: 10.1097/HJH.0000000000002083
27. Alazami AM, Patel N, Shamseldin HE, Anazi S, Al-Dosari MS, Alzahrani F, et al. Accelerating novel candidate gene discovery in neurogenetic disorders via whole-exome sequencing of prescreened multiplex consanguineous families. Cell Rep. (2015) 10:148–61. doi: 10.1016/j.celrep.2014.12.015
Keywords: tumor microenvironment, lung adenocarcinoma, prognostic model, TCGA database, ESTIMATE algorithm
Citation: Qi X, Qi C, Qin B, Kang X, Hu Y and Han W (2020) Immune-Stromal Score Signature: Novel Prognostic Tool of the Tumor Microenvironment in Lung Adenocarcinoma. Front. Oncol. 10:541330. doi: 10.3389/fonc.2020.541330
Received: 08 March 2020; Accepted: 14 August 2020;
Published: 23 September 2020.
Edited by:
Qing Zhou, Guangdong Provincial People's Hospital Lung Cancer Institute, ChinaReviewed by:
Elena Levantini, Beth Israel Deaconess Medical Center and Harvard Medical School, United StatesCheng Zhang, Fourth Affiliated Hospital of China Medical University, China
Copyright © 2020 Qi, Qi, Qin, Kang, Hu and Han. This is an open-access article distributed under the terms of the Creative Commons Attribution License (CC BY). The use, distribution or reproduction in other forums is permitted, provided the original author(s) and the copyright owner(s) are credited and that the original publication in this journal is cited, in accordance with accepted academic practice. No use, distribution or reproduction is permitted which does not comply with these terms.
*Correspondence: Yi Hu, huyi0401@aliyun.com; Weidong Han, hanwdrsw69@yahoo.com
†These authors have contributed equally to this work