- Department of Medical Imaging, Sun Yat-sen University Cancer Center, State Key Laboratory of Oncology in South China, Collaborative Innovation Center for Cancer Medicine, Guangzhou, China
Objectives: The diagnostic performance of intravoxel incoherent motion diffusion–weighted imaging (IVIM-DWI) in the differential diagnosis of breast tumors remains debatable among published studies. Therefore, this meta-analysis aimed to pool relevant evidence regarding the diagnostic performance of IVIM-DWI in the differential diagnosis of breast tumors.
Methods: Studies on the differential diagnosis of breast lesions using IVIM-DWI were systemically searched in the PubMed, Embase and Web of Science databases in recent 10 years. The standardized mean difference (SMD) and 95% confidence intervals of the apparent diffusion coefficient (ADC), tissue diffusivity (D), pseudodiffusivity (D*), and perfusion fraction (f) were calculated using Review Manager 5.3, and Stata 12.0 was used to pool the sensitivity, specificity, and area under the curve (AUC), as well as assess publication bias and heterogeneity. Fagan's nomogram was used to predict the posttest probabilities.
Results: Sixteen studies comprising 1,355 malignant and 362 benign breast lesions were included. Most of these studies showed a low to unclear risk of bias and low concerns regarding applicability. Breast cancer had significant lower ADC (SMD = −1.38, P < 0.001) and D values (SMD = −1.50, P < 0.001), and higher f value (SMD = 0.89, P = 0.001) than benign lesions, except D* value (SMD = −0.30, P = 0.20). Invasive ductal carcinoma showed lower ADC (SMD = 1.34, P = 0.01) and D values (SMD = 1.04, P = 0.001) than ductal carcinoma in situ. D value demonstrated the best diagnostic performance (sensitivity = 86%, specificity = 86%, AUC = 0.91) and highest post-test probability (61, 48, 46, and 34% for D, ADC, f, and D* values) in the differential diagnosis of breast tumors, followed by ADC (sensitivity = 76%, specificity = 79%, AUC = 0.85), f (sensitivity = 80%, specificity = 76%, AUC = 0.85) and D* values (sensitivity = 84%, specificity = 59%, AUC = 0.71).
Conclusion: IVIM-DWI parameters are adequate and superior to the ADC in the differentiation of breast tumors. ADC and D values can further differentiate invasive ductal carcinoma from ductal carcinoma in situ. IVIM-DWI is also superior in identifying lymph node metastasis, histologic grade, and hormone receptors, and HER2 and Ki-67 status.
Introduction
Breast cancer is one of the most common malignant tumors and the second leading cause of cancer death in females (1). Early detection and accurate diagnosis of breast cancer with various histological/molecular subtypes, such as estrogen receptor (ER), progesterone receptor (PR), human epidermal growth factor receptor 2 (HER2), and Ki-67 proliferation indexes, are helpful for developing individualized therapies and achieving a better prognosis. Screening the breast lesions with conventional mammography is challenging for clinician due to the low sensitivity in dense breast parenchyma (2). Dynamic contrast-enhanced magnetic resonance imaging (DCE-MRI) is a common MRI sequence in clinical practice, which can reflect the morphological and haemodynamic features of breast lesions. A previous meta-analysis which included studies using DCE-MRI as an adjunct to conventional mammography or ultrasound to clarify uncertain finding without microcalcification, demonstrated that breast MRI had an excellent diagnostic performance with a pooled sensitivity of 99% and specificity of 89% (3). However, the specificity is still variable due to background parenchymal enhancement and overlapped kinetic enhancement patterns between breast cancer and benign lesion. The false-positive findings may cause additional examination or unnecessary surgery (4).
Diffusion-weighted imaging (DWI) has become a promising technique in the differential diagnosis of breast lesions, which allows measurement of water molecular movement using apparent diffusion coefficient (ADC) values. The international European Society of Breast Imaging (EUSOBI) working group has confirmed the importance of breast DWI in the multiparametric breast MRI protocol to differentiate between breast cancer and benign lesions, distinguish in situ from invasive lesions, and predict the responses to neoadjuvant therapy over time (5). Breast cancer usually has high cellularity (low diffusivity) and high vascularity (high perfusion), which may impact ADC values in a diametrically opposite direction.
Intravoxel incoherent motion (IVIM) is an advanced imaging technique that was first proposed by Le Bihan et al. (6). This procedure can distinguish the incoherent motion of water molecules within the capillaries from molecular diffusion in the extravascular space (7). The true diffusion coefficient (D value), pseudodiffusion coefficient (D* value) and perfusion fraction (f value) were generated using a biexponential model with multiple b-values (8). Several studies have applied IVIM-DWI to discriminate breast cancer from benign breast lesions and characterize the histological/molecular subtypes of breast cancer better diagnostic performance than traditional ADC values (7, 9, 10). However, the diagnostic performance of IVIM-DWI-derived parameters in the differentiation of breast tumors is not consistent, and the application of this sequence remains debatable. For example, several studies (7, 11, 12) indicated that breast cancer had a higher D* value than did benign lesions, while other studies reported adverse (10, 13–15) or non-significant results (9, 16, 17). The studies of Cho et al. (9) and Lin et al. (13) suggested that the IVIM model can further distinguish invasive ductal carcinoma (IDC) from ductal carcinoma in situ (DCIS), while another study reported no significant difference in the D, D*, and f values between them (7). Last but not least, the small sample sizes in most studies were still insufficient to draw a robust conclusion for the performance of IVIM-DWI; therefore, clinical guidelines for the application in the breast have not been established. To address this problem, we perform a meta-analysis of all the published results regarding the diagnostic performance of IVIM-DWI in differentiating malignant and benign breast lesions. The controversial issues among the different studies will be addressed with more reliable evidence.
Materials and Methods
Data Sources
Studies on the differential diagnosis of breast tumors using IVIM-DWI parameters published in the past 10 years were systemically retrieved from PubMed, Embase and Web of Science by two senior librarians. A search formula was created using different combinations of medical subject headings or key words related to the following terms: IVIM, intravoxel incoherent motion, multiple b-values DWI, biexponential, true diffusion coefficient, pseudodiffusion coefficient, perfusion fraction, and breast or breast lesion/cancer/carcinoma. We also performed manual retrieval of the reference lists from the included studies.
Study Selection
Studies that met the following criteria were included: (a) the research purpose was to differentiate malignant and benign breast lesions using IVIM-DWI parameters; (b) the mean and standard deviation (SD) of each parameter were provided; (c) the diagnostic performance regarding sensitivity and specificity, or true-positive (TP), false-negative (FN), false-positive (FP), and true-negative (TN) counts were reported; and (d) breast cancer was confirmed by pathology after initial MRI examination. Exclusion criteria mainly included (a) duplication from the same authors or institutions; (b) meta-analysis, conference abstract, review or any unpublished results; (c) animal experiments or studies not on breasts; (d) non-English studies; and (e) studies with b-values >2,500 s/mm2, to maximally avoid non-Gaussian diffusion.
Data Extraction
A spreadsheet was used to extract the mean values and SDs as well as the diagnostic performance of the ADC, D, D*, and f values with threshold value, area under the curve (AUC), sensitivity, specificity or the TP, FN, FP, and TN in the respective study by one author and reviewed by another author. Other information included first author, publication year, country, field strength and vendors, b-values, patient ages, tumor size, and published journals.
Quality Assessment
The quality of the studies and likelihood of bias were evaluated using Review Manager 5.3 software (Cochrane Collaboration), referring to the Quality Assessment of Diagnostic Accuracy Studies-2 (18). We assessed the risk of bias and applicability in four domains: patient selection, index tests, reference standards, and flow and timing (19).
Publication Bias and Heterogeneity Evaluation
Because two parts of the data were pooled in our study—quantitative values and the diagnostic performance of each parameter, funnel plots and Begg's test were used to visually and quantitatively assess the publication bias for the continuous variables, whereas Deek's plot was used to assess the publication bias of the sensitivity and specificity with Stata version 12.0 (StataCorp). For an asymmetric or skewed funnel plot, P < 0.05 in Begg's test or Deeks' test, indicated the potential of publication bias (20). The inconsistency index (I2) and Cochran's Q-tests were used to explore the heterogeneity of the included studies, with I2 >50% or P < 0.05 for the Cochran Q-test suggesting statistically significant heterogeneity; in these instances, a random-effect model was applied for subsequent pooling, or a fixed-effect model when I2 < 50% (21).
Data Synthesis
We constructed forest plots for continuous variables and calculated the standardized mean difference (SMD) between malignant and benign breast lesions using Review Manager 5.3 software. We used the bivariate mixed-effects binary regression model in Stata version 12.0 to pool the diagnostic performance with sensitivity, specificity, positive likelihood ratio (PLR), negative likelihood ratio (NLR), diagnostic odds ratio (DOR), and AUC. The summary receiver operating characteristic (SROC) curves and Fagan's nomograms were also plotted to determine the diagnostic values and predict the post-test probabilities of the ADC, D, D* and f values in obtaining a differential diagnosis of breast tumors. Meta-disc 1.4 was used to evaluate the threshold effects by calculating the Spearman correlation coefficient (r) between the logit (TP rate) and logit (FP rate).
Results
Literature Search and Selection
A flowchart detailing the study selection process is provided in Figure 1. Although the study by Iima et al. (22) included b-values of 2,000 and 2,500 s/mm2 which may induce non-Gaussian diffusion, they used a hybrid model to sufficiently separate the non-Gaussian diffusion from IVIM effects. We also performed a sensitivity analysis and compared the pooled results between before and after excluding the study, the results were not significantly changed. Therefore, we considered the study by Iima et al. is suitable to be included. Sixteen eligible studies with 1,355 malignant and 362 benign breast lesions were included for analysis. Basic information and diagnostic performance for each included study are detailed in Tables 1, 2. The breast cancer subtypes mainly included DCIS, IDC, lobular carcinoma in situ, invasive lobular carcinoma, intraductal papillary carcinoma, squamous cell carcinoma, mucinous carcinoma and malignant phyllodes tumors. Benign lesions consisted of fibroadenoma, intraductal papilloma, granulomatous mastitis, epithelial proliferative lesion, fibrocystic change, and phyllodes tumors.
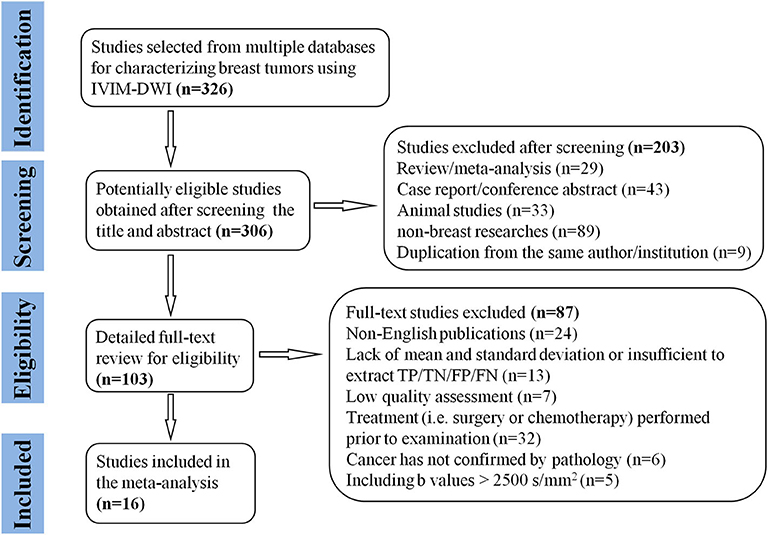
Figure 1. Flowchart detailing the study selection process. Sixteen studies met the inclusion criteria. FN, false negative; FP, false positive; TN, true negative; TP, true positive.
Quality Assessment
The distribution of the Quality Assessment of Diagnostic Accuracy Studies−2 scores for risk of bias and applicability concerns are shown in Figure 2. The overall quality of the included studies was acceptable. In the patient selection domain, six studies showed unclear risk of bias due to ambiguity regarding patient enrolment and study design. The applicability concerns remained unclear or high in five studies, because the malignant and benign tumor types were inconsistent between studies. Four studies were marked unclear risk of bias with high concerns of applicability for the index test domain, because the threshold values for ADC, D, D* or f were not provided. Six studies showed unclear or high risks of bias in reference standards domain because some of the benign lesions were diagnosed via long-term follow-up. Most studies had a low risk of bias regarding patient flow and timing domains because of the short time interval between MR examination and pathological confirmation (within 1 week).
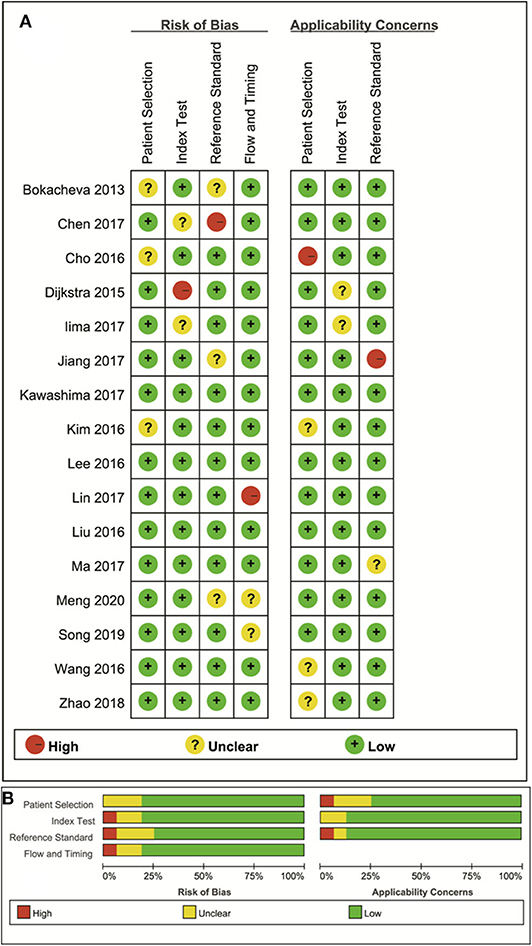
Figure 2. The distribution of risk of bias and applicability concerns for each included study using Quality Assessment of Diagnostic Accuracy Studies-2 (QUADAS-2) (A) and a summary of the methodological quality (B).
Quantitative Analysis
ADC Used for Diagnosis of Breast Tumor
Eight studies regarding ADC used in differentiating breast tumors were included for analysis. The χ2 = 31.73 and P < 0.001 of the heterogeneity test (I2 = 78%) suggested high heterogeneity among the included studies. The forest plot in Figure 3 shows the distribution of the ADC between malignant and benign breast lesions. A random-effects model generated an SMD of −1.38 (−1.76, −1.00) (P < 0.001) between malignant and benign breast lesions for ADC. The Begg's test suggested no publication bias relating to the ADC (P = 0.428).
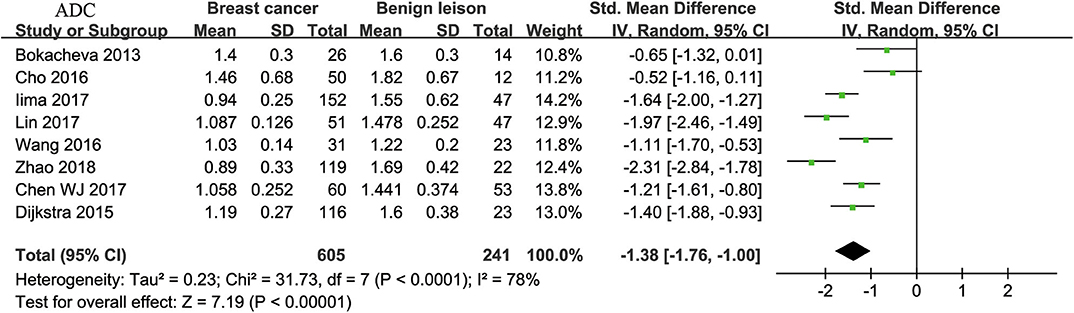
Figure 3. Forest plot of the mean value of the apparent diffusion coefficient (ADC) between malignant and benign breast lesions. The standardized mean differences (SMDs) indicated that breast cancers had a significantly lower ADC than benign lesions.
D Value Used for Diagnosis of Breast Tumor
Ten studies regarding D value used in differentiating breast tumors were included for analysis. The χ2 = 37.49 and P < 0.001 of the heterogeneity test (I2 = 76%) suggested high heterogeneity among the included studies. The forest plot in Figure 4 shows the distribution of D between malignant and benign breast lesions. A random-effects model generated an SMD of −1.50 (−1.85, −1.14) (P < 0.001) between malignant and benign breast lesions for D. The Begg's Test suggested no publication bias relating to D (P = 0.112).
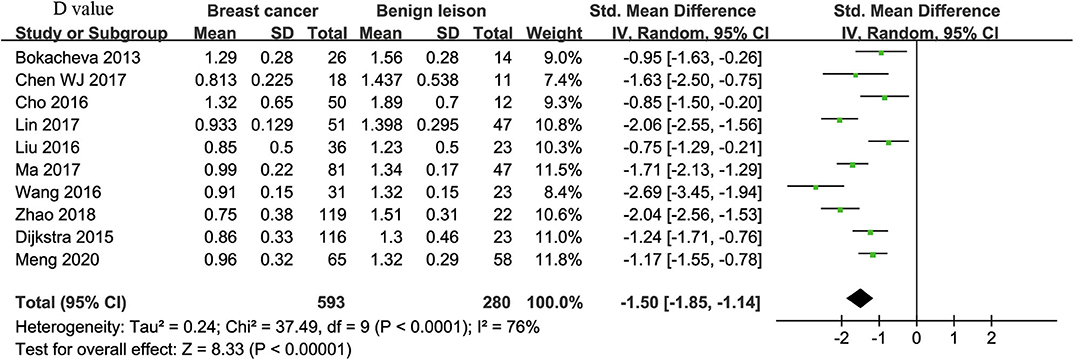
Figure 4. Forest plot of the mean value of tissue diffusivity (D) between malignant and benign breast lesions. The standardized mean differences (SMDs) indicated that breast cancers had a significantly lower D value than did benign lesions.
D* Value Used for Diagnosis of Breast Tumor
Twelve studies regarding D* value used in differentiating breast tumors were included for analysis. The χ2 = 123.02 and P < 0.001 of the heterogeneity test (I2 = 91%) suggested high heterogeneity among the included studies. The forest plot in Figure 5 shows the distribution of D* between malignant and benign breast lesions. A random-effects model generated an SMD of −0.30 (−0.76, 0.16) (P = 0.20) between malignant and benign breast lesions for D*. The Begg's test suggested no publication bias relating to D* (P = 0.208).
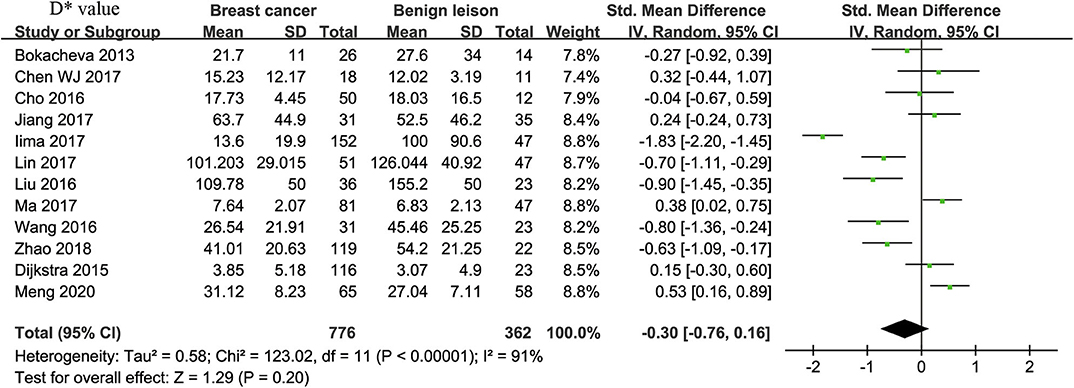
Figure 5. Forest plot of the mean value of pseudodiffusivity (D*) between malignant and benign breast lesions. The standardized mean differences (SMDs) indicated that there is no statistical difference between breast cancers and benign lesions in D* value.
f-Value Used for Diagnosis of Breast Tumor
Twelve studies regarding f value used in differentiating breast tumors were included for analysis. The χ2 = 20.07 and P = 0.04 of the heterogeneity test (I2 = 45%) suggested mild heterogeneity among the included studies. The forest plot in Figure 6 shows the distribution of f between malignant and benign breast lesions. A fixed-effects model generated an SMD of 0.89 (0.75, 1.02) (P < 0.001) between malignant and benign breast lesions for f value. The Begg's test suggested no publication bias in f (P = 0.880).
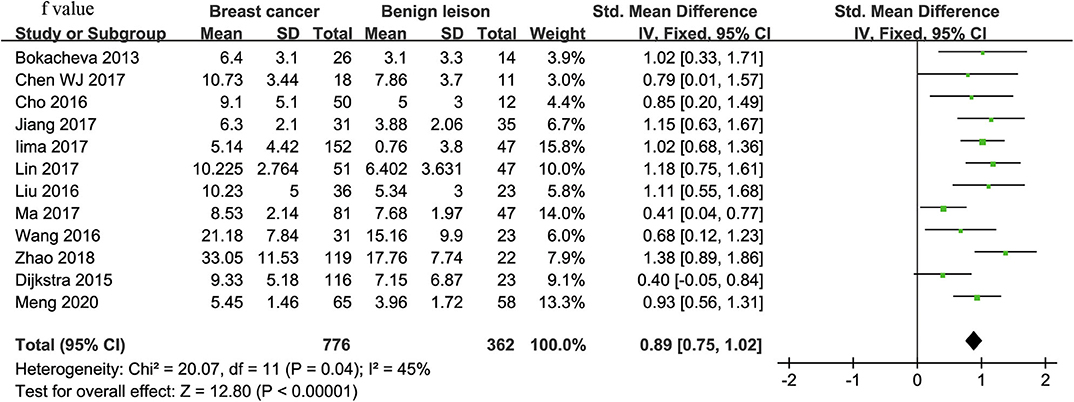
Figure 6. Forest plot of the mean value of perfusion fraction (f) between malignant and benign breast lesions. The standardized mean differences (SMDs) indicated that breast cancers had a significantly higher f value than did benign lesions.
Subgroup Analysis for Histological/Molecular Subtypes
Because the treatment strategy and prognosis were different between DCIS and IDC, and because several studies have provided differential information between DCIS and IDC as well as other pathologic prognostic factors such as tumor size, lymph node metastasis, histologic grade, and the molecular expression of ER, PR, HER2, and Ki-67 in breast cancer, we further pooled these results. The pooled results were listed in Table 3. The results suggested that IDC had lower ADC (SMD = 1.34, P = 0.01) and D values (SMD = 1.04, P = 0.001) than did DCIS. No significant difference was observed in the ADC, D, D*, and f values between tumors ≥2 cm and those <2 cm (all P ≥ 0.05). Higher D* (SMD = −0.23, P = 0.009) and f values (SMD = −0.28, P = 0.001) were observed in lesions with metastatic lymph nodes comparing to lesions with negative lymph nodes metastasis. HER2-positive cancer showed higher D* (SMD = −0.28, P = 0.003) and f values (SMD = −0.24, P = 0.009) than did HER2-negative cancer. Tumors with high Ki-67 expression had a lower D value than those with low Ki-67 expression (SMD = 0.26, P = 0.002). There was also a significant difference in the D* value between ER-positive and ER-negative tumors, PR-positive and PR-negative tumors, and low-grade and high-grade tumors (all P < 0.05).
Diagnostic Performance
The diagnostic performance as assessed by pooling sensitivity, specificity, PLR, NLR, DOR and the AUCs of the ADC, D, D*, and f values are listed in Table 4. Deek's funnel plots and asymmetry tests indicated no obvious publication bias for the ADC, D, and f values (P = 0.34, 0.28, and 0.21) but potential publication bias for the D* value (P = 0.03). Figure 7 plots the SROC curves of the ADC, D, D* and f values. Because not all the studies reported the diagnostic performance of IVIM-DWI in the detection of breast tumors, there were a small number of studies included for analysis in Table 2 and Figure 7. The D value demonstrated good diagnostic performance (sensitivity = 86%, specificity = 86%, AUC = 0.91) in the differential diagnosis of breast tumors, which was comparable to that of the ADC (sensitivity = 76%, specificity = 79%, AUC = 0.85), followed by the f (sensitivity = 80%, specificity = 76%, AUC = 0.85) and D* values (sensitivity = 84%, specificity = 59%, AUC = 0.71).
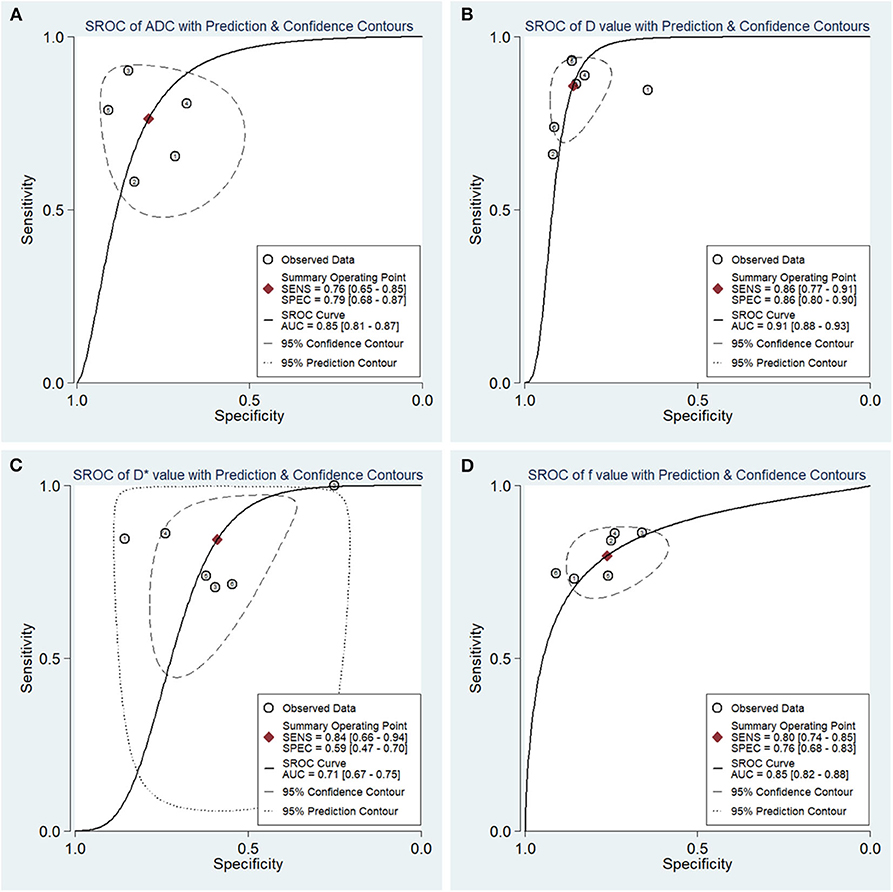
Figure 7. Summary receiver operating characteristic (SROC) curves of (A) the apparent diffusion coefficient (ADC), (B) tissue diffusivity (D), (C) pseudodiffusivity (D*), and (D) the perfusion fraction (f) in the diagnosis of breast lesions. D had the largest area under the curve (AUC) among the four parameters, followed by the ADC, f and D* values.
Posttest Probabilities
The likelihood ratio and post-test probability are also important for diagnosing a disease (31), which estimated whether a patient was diagnosed with a certain disease using the MRI parameters. Figure 8 plotted the Fagan's nomograms of the ADC, D, D*, and f values for predicting post-test probabilities. All the pre-test probabilities were set at 20% by default. We regarded the diagnosis of breast cancer as a positive event, corresponding to a higher f value and lower ADC and D values. Similarly, diagnosing benign lesions with a lower f value and higher ADC and D values represented a negative event. From a pre-test probability of 20%, the post-test probability increased to 48% with a PLR of 3.7 and decreased to 7% with an NLR of 0.30 based on the ADC. This indicated that the diagnostic probability for breast cancer will be obviously enhanced in cases with a lower ADC than in cases without an ADC measurement. By contrast, the probability of a breast cancer diagnosis will significantly drop from 20 to 7% when a negative event occurs (e.g., a higher ADC). Similarly, when using D for predicting a diagnosis, the post-test probability of diagnosing breast cancer will reach 61% with a PLR of 6.1 and drop to 4% with an NLR of 0.17. The inclusion of f increases the post-test probability of diagnosing breast cancer to 46% with a PLR of 3.4 and decreases it to 6% with an NLR of 0.27. These data indicated that IVIM parameters, especially the D value, increased the accuracy for diagnosing breast cancer.
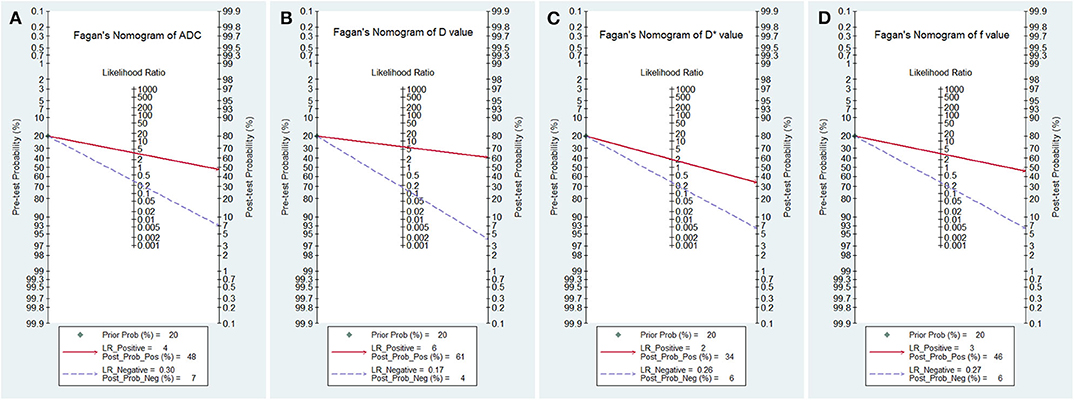
Figure 8. Fagan's nomogram of (A) the apparent diffusion coefficient (ADC), (B) tissue diffusivity (D), (C) pseudodiffusivity (D*), and (D) the perfusion fraction (f).
Discussion
IVIM-DWI is a non-invasive technique that shows superiority in reflecting tumor cellularity and perfusion without the need for contrast agent. It has already been applied in the differentiation of lung nodules (32), thyroid nodules (33), prostate (34) and brain tumors (35) with good diagnostic performance. Although IVIM has become a research hotspot in whole-body tumors, especially in breast tumors, to the best of our knowledge, there is still no study on breast tissues with a sufficient sample size to establish the value of IVIM for quantitatively distinguishing breast cancer from benign lesions and their molecular subtypes. Our study provides a timely summary of this issue by pooling all published evidence with strict inclusion criteria and quality assessments. The results showed a promising prospect for incorporating IVIM-DWI into MRI protocols for the breast.
In our study, the SMDs suggested that malignant breast tumors demonstrated lower ADC and D values and higher f values than did benign lesions. Breast cancer usually has dense cellularity with a high capacity for proliferation, which may reduce the extracellular space and limit the diffusion of water molecules thus causing a reduction in the diffusion coefficient. The pooled results also suggested that the D value improved the diagnostic performance with a slightly higher sensitivity, specificity, AUC, DOR and post-test probability than conventional ADC. Theoretically, the monoexponential model may miscalculate the water molecule movement in conjunction with microcirculation perfusion and therefore overestimate the ADC value (14). The D value can precisely calculate the true diffusion without the influence of perfusion-related diffusion (15), but a larger number and higher b-value applied in the IVIM model will significantly prolong the scanning times and introduce motion and susceptibility artifacts.
Interestingly, malignant breast tumors demonstrated a significantly higher f value but a non-significantly higher D* value than did the benign lesions. This mainly arose from increased angiogenesis in breast cancer (14). The f value also demonstrated a higher specificity of 0.76 and an AUC of 0.85 compared with the specificity of 0.59 and AUC of 0.71 for the D* value. In addition, the mean D* values of breast cancer ranged from 3.85 to 109.78 × 10−3 mm2/s with a huge SD among the included studies, which indicated that the D* value was not robust and could not further increase the diagnostic sensitivity and specificity; however, the f value was able to more accurately reflect tissue perfusion. Liu et al. (14) also stated that the D* value may be unreliable in the IVIM model due to the low signal-to-noise ratio and the poor measurement reproducibility.
The pooled results indicated lower ADC and D values in IDC than in DCIS, suggesting denser cellularity and a more limited extracellular volume fraction in IDC with more aggressive features (7). Therapeutic strategies and treatment efficacy are closely related to intrinsic biological subtypes of breast cancer (17). Our pooled results suggested that the lesions with metastatic lymph nodes had higher D* and f values than did lesions without lymph node metastasis. The IVIM model provides a surrogate marker for predicting lymph node status, and rich tumor perfusion owing to neovascularization may facilitate lymphatic metastasis (36). The results also suggested greater tumor perfusion (D* and f value) in HER2-positive cancer. HER2 is an important prognostic factor of breast cancer and is closely correlated with tumor proliferation, invasion and metastasis. It can promote tumor angiogenesis and lymphangiogenesis via regulation of vascular endothelial growth factor (VEGF) in breast cancer and therefore improve tumor perfusion (10). Our study also suggested that breast cancer with high Ki-67 expression has a significantly lower D value (P = 0.002) instead of ADC value (P = 0.27), which was mainly due to active proliferation and a higher cell density. The results suggested that the D value better reflected Ki-67 status than did ADC values when assessing the cell density and proliferation status. Our results also suggested a significant difference in the perfusion-related parameters (D*) between ER or PR statuses. The detection of ER and PR is of great significance for estimating the prognosis of breast cancer and guiding endocrine therapy, as patients with positive ER and PR expression showed high responsiveness to hormone therapies. Previous studies have reported that positive ER and PR expression inhibited tumor angiogenesis by decreasing the level of VEGF (7, 10, 37, 38), which leads to lower D* value in ER- and PR-positive tumors.
The correlation results suggested no significant threshold effects in the ADC (r = −0.100, P = 0.873), D (r = 0.342, P = 0.452), D* (r = −0.029, P = 0.957) and f values (r = 0.829, P = 0.524); thus, they are not the main contributors to the heterogeneity. The ADC, D, D*, and f values all demonstrated obvious heterogeneity, which should be further explored. First, most of the included studies did not control for age or menstrual cycle for analysis, which may have introduced heterogeneity. Second, 1.5T and 3.0T MR scanners with various combinations of b-values were used to perform IVIM-DWI in these studies, which may influence the accuracy of the calculations of diffusion and perfusion coefficients. Third, the post-processing methods were different, as some studies (9, 26) performed histogram analyses for the whole lesions, while the others assessed the lesions at the largest section as the region of interest. Last, the tumor subtypes were inconsistent in the malignant and benign groups; this may result in different biological characteristics and consequent variations in the IVIM values.
There were several limitations to this meta-analysis. First, the small number of studies regarding the histological/molecular subtypes of breast cancer was still insufficient to draw a robust conclusion. Second, we did not perform a horizontal comparison with other diffusion imaging techniques, such as diffusion tensor imaging and diffusion kurtosis imaging, both of which provide information that reflects directional characteristics and tissue complexity. A combination of these sequences may further improve the specificity in characterizing breast lesions.
Conclusions
IVIM-DWI parameters were adequate and superior to the ADC in differentiating breast tumors. They can further differentiate IDC from DCIS. Besides, IVIM-derived parameters also showed unique superiority in identifying lymph node metastasis, histologic grade, and hormone receptor, and HER2 and Ki-67 status. It is quite suitable when making treatment plans and prognosis assessments.
Data Availability Statement
All datasets generated for this study are included in the article/supplementary material.
Author Contributions
NH and YW conceived the study and revised the manuscript. JL and SZ drafted the manuscript. ZL and YK searched the databases and acquired the data. TM, JC, and CZ performed data analysis and interpretation. All authors contributed substantially to the preparation of the manuscript.
Funding
Research was supported by the National Key Research and Development Program of China (Grant No. 2017YFC0112605) the Medical Science Research Foundation of Guangdong Province of China (Grant No. 20180005) and the Opening Research Fund of Guangzhou Key Laboratory of Molecular and Functional Imaging for Clinical Translation (Grant No. 201905010003).
Conflict of Interest
The authors declare that the research was conducted in the absence of any commercial or financial relationships that could be construed as a potential conflict of interest.
Abbreviations
AUC, area under the curve; ADC, apparent diffusion coefficient; D, tissue diffusivity, D*, pseudodiffusivity; ER, estrogen receptor; f, perfusion fraction; HER2, human epidermal growth factor receptor 2; IVIM-DWI, intravoxel incoherent motion–diffusion–weighted imaging; SMD, standardized mean difference; I2, inconsistency index; PR, progesterone receptor.
References
1. Bray F, Ferlay J, Soerjomataram I, Siegel RL, Torre LA, Jemal A. Global cancer statistics 2018: GLOBOCAN estimates of incidence and mortality worldwide for 36 cancers in 185 countries. CA Cancer J Clin. (2018) 68:394–424. doi: 10.3322/caac.21492
2. Aberle DR, Chiles C, Gatsonis C, Hillman BJ, Johnson CD, McClennan BL, et al. Imaging and cancer: research strategy of the American college of radiology imaging network. Radiology. (2005) 235:741–51. doi: 10.1148/radiol.2353041760
3. Bennani-Baiti B, Bennani-Baiti N, Baltzer PA. Diagnostic performance of breast magnetic resonance imaging in non-calcified equivocal breast findings: results from a systematic review and meta-analysis. PLoS ONE. (2016) 11:e0160346. doi: 10.1371/journal.pone.0160346
4. Plana MN, Carreira C, Muriel A, Chiva M, Abraira V, Emparanza JI, et al. Magnetic resonance imaging in the preoperative assessment of patients with primary breast cancer: systematic review of diagnostic accuracy and meta-analysis. Eur Radiol. (2012) 22:26–38. doi: 10.1007/s00330-011-2238-8
5. Baltzer P, Mann RM, Iima M, Sigmund EE, Clauser P, Gilbert FJ, et al. Diffusion-weighted imaging of the breast-a consensus and mission statement from the EUSOBI international breast diffusion-weighted imaging working group. Eur Radiol. (2020) 30:1436–50. doi: 10.1007/s00330-019-06510-3
6. Le Bihan D, Breton E, Lallemand D, Grenier P, Cabanis E, Laval-Jeantet M. MR imaging of intravoxel incoherent motions: application to diffusion and perfusion in neurologic disorders. Radiology. (1986) 161:401–7. doi: 10.1148/radiology.161.2.3763909
7. Suo S, Cheng F, Cao M, Kang J, Wang M, Hua J, et al. Multiparametric diffusion-weighted imaging in breast lesions: association with pathologic diagnosis and prognostic factors. J Magn Reson Imaging. (2017) 46:740–50. doi: 10.1002/jmri.25612
8. Liang J, Li J, Li Z, Meng T, Chen J, Ma W, et al. Differentiating the lung lesions using intravoxel incoherent motion diffusion-weighted imaging: a meta-analysis. BMC Cancer. (2020) 20:799. doi: 10.1186/s12885-020-07308-z
9. Cho GY, Moy L, Kim SG, Baete SH, Moccaldi M, Babb JS, et al. Evaluation of breast cancer using intravoxel incoherent motion (IVIM) histogram analysis: comparison with malignant status, histological subtype, and molecular prognostic factors. Eur Radiol. (2016) 26:2547–58. doi: 10.1007/s00330-015-4087-3
10. Zhao M, Fu K, Zhang L, Guo W, Wu Q, Bai X, et al. Intravoxel incoherent motion magnetic resonance imaging for breast cancer: a comparison with benign lesions and evaluation of heterogeneity in different tumor regions with prognostic factors and molecular classification. Oncol Lett. (2018) 16:5100–12. doi: 10.3892/ol.2018.9312
11. Ma D, Lu F, Zou X, Zhang H, Li Y, Zhang L, et al. Intravoxel incoherent motion diffusion-weighted imaging as an adjunct to dynamic contrast-enhanced MRI to improve accuracy of the differential diagnosis of benign and malignant breast lesions. Magn Reson Imaging. (2017) 36:175–9. doi: 10.1016/j.mri.2016.10.005
12. Mao X, Zou X, Yu N, Jiang X, Du J. Quantitative evaluation of intravoxel incoherent motion diffusion-weighted imaging (IVIM) for differential diagnosis and grading prediction of benign and malignant breast lesions. Medicine. (2018) 97:e11109. doi: 10.1097/MD.0000000000011109
13. Lin N, Chen J, Hua J, Zhao J, Zhao J, Lu J. Intravoxel incoherent motion MR imaging in breast cancer: quantitative analysis for characterizing lesions. Int J Clin Exp Med. (2017) 10:1705–14.
14. Liu C, Wang K, Chan Q, Liu Z, Zhang J, He H, et al. Intravoxel incoherent motion MR imaging for breast lesions: comparison and correlation with pharmacokinetic evaluation from dynamic contrast-enhanced MR imaging. Eur Radiol. (2016) 26:3888–98. doi: 10.1007/s00330-016-4241-6
15. Wang Q, Guo Y, Zhang J, Wang Z, Huang M, Zhang Y. Contribution of IVIM to conventional dynamic contrast-enhanced and diffusion-weighted MRI in differentiating benign from malignant breast masses. Breast Care. (2016) 11:254–8. doi: 10.1159/000447765
16. Jin YN, Zhang Y, Cheng JL, Zheng DD, Hu Y. Monoexponential, biexponential, and stretched-exponential models using diffusion-weighted imaging: a quantitative differentiation of breast lesions at 3.0T. J Magn Reson Imaging. (2019) 50:1461–7. doi: 10.1002/jmri.26729
17. Kim Y, Ko K, Kim D, Min C, Kim SG, Joo J, et al. Intravoxel incoherent motion diffusion-weighted MR imaging of breast cancer: association with histopathological features and subtypes. Br J Radiol. (2016) 89:20160140. doi: 10.1259/bjr.20160140
18. Whiting PF, Rutjes AW, Westwood ME, Mallett S, Deeks JJ, Reitsma JB, et al. QUADAS-2: a revised tool for the quality assessment of diagnostic accuracy studies. Ann Intern Med. (2011) 155:529–36. doi: 10.7326/0003-4819-155-8-201110180-00009
19. Cheng Q, Huang J, Liang J, Ma M, Ye K, Shi C, et al. The diagnostic performance of DCE-MRI in evaluating the pathological response to neoadjuvant chemotherapy in breast cancer: a meta-analysis. Front Oncol. (2020) 10:93. doi: 10.3389/fonc.2020.00093
20. Huang C, Liang J, Lei X, Xu X, Xiao Z, Luo L. Diagnostic performance of perfusion computed tomography for differentiating lung cancer from benign lesions: a meta-analysis. Med Sci Monit. (2019) 25:3485–94. doi: 10.12659/MSM.914206
21. Liang J, Liu D, Gao P, Zhang D, Chen H, Shi C, et al. Diagnostic values of DCE-MRI and DSC-MRI for differentiation between high-grade and low-grade gliomas: a comprehensive meta-analysis. Acad Radiol. (2018) 25:338–48. doi: 10.1016/j.acra.2017.10.001
22. Iima M, Kataoka M, Kanao S, Onishi N, Kawai M, Ohashi A, et al. Intravoxel incoherent motion and quantitative non-gaussian diffusion MR Imaging: evaluation of the diagnostic and prognostic value of several markers of malignant and benign breast lesions. Radiology. (2018) 287:432–41. doi: 10.1148/radiol.2017162853
23. Bokacheva L, Kaplan JB, Giri DD, Patil S, Gnanasigamani M, Nyman CG, et al. Intravoxel incoherent motion diffusion-weighted MRI at 3.0 T differentiates malignant breast lesions from benign lesions and breast parenchyma. J Magn Reson Imaging. (2014) 40:813–23. doi: 10.1002/jmri.24462
24. Chen W, Zhang J, Long D, Wang Z, Zhu JM. Optimization of intra-voxel incoherent motion measurement in diffusion-weighted imaging of breast cancer. J Appl Clin Med Phys. (2017) 18:191–9. doi: 10.1002/acm2.12065
25. Jiang L, Lu X, Hua B, Gao J, Zheng D, Zhou Y. Intravoxel incoherent motion diffusion-weighted imaging versus dynamic contrast-enhanced magnetic resonance imaging: comparison of the diagnostic performance of perfusion-related parameters in breast. J Comput Assist Tomogr. (2018) 42:6–11. doi: 10.1097/RCT.0000000000000661
26. Lee YJ, Kim SH, Kang BJ, Kang YJ, Yoo H, Yoo J, et al. Intravoxel incoherent motion (IVIM)-derived parameters in diffusion-weighted MRI: associations with prognostic factors in invasive ductal carcinoma. J Magn Reson Imaging. (2017) 45:1394–406. doi: 10.1002/jmri.25514
27. Dijkstra H, Dorrius MD, Wielema M, Jaspers K, Pijnappel RM, Oudkerk M, et al. Semi-automated quantitative intravoxel incoherent motion analysis and its implementation in breast diffusion-weighted imaging. J Magn Reson Imaging. (2016) 43:1122–31. doi: 10.1002/jmri.25086
28. Kawashima H, Miyati T, Ohno N, Ohno M, Inokuchi M, Ikeda H, et al. Differentiation between luminal-a and luminal-b breast cancer using intravoxel incoherent motion and dynamic contrast-enhanced magnetic resonance imaging. Acad Radiol. (2017) 24:1575–81. doi: 10.1016/j.acra.2017.06.016
29. Meng N, Wang XJ, Sun J, Huang L, Wang Z, Wang KY, et al. Comparative study of amide proton transfer-weighted imaging and intravoxel incoherent motion imaging in breast cancer diagnosis and evaluation. J Magn Reson Imaging. (2020) 52:1175–86. doi: 10.1002/jmri.27190
30. Song SE, Cho KR, Seo BK, Woo OH, Park KH, Son YH, et al. Intravoxel incoherent motion diffusion-weighted MRI of invasive breast cancer: correlation with prognostic factors and kinetic features acquired with computer-aided diagnosis. J Magn Reson Imaging. (2019) 49:118–30. doi: 10.1002/jmri.26221
31. Wang K, Li Z, Wu Z, Zheng Y, Zeng S, Linning E, et al. Diagnostic performance of diffusion tensor imaging for characterizing breast tumors: a comprehensive meta-analysis. Front Oncol. (2019) 9:1229. doi: 10.3389/fonc.2019.01229
32. Jiang J, Fu Y, Hu X, Cui L, Hong Q, Gu X, et al. The value of diffusion-weighted imaging based on monoexponential and biexponential models for the diagnosis of benign and malignant lung nodules and masses. Br J Radiol. (2020) 93:20190400. doi: 10.1259/bjr.20190400
33. Song M, Yue Y, Jin Y, Guo J, Zuo L, Peng H, et al. Intravoxel incoherent motion and ADC measurements for differentiating benign from malignant thyroid nodules: utilizing the most repeatable region of interest delineation at 3.0 T. Cancer Imaging. (2020) 20:9. doi: 10.1186/s40644-020-0289-2
34. Cui Y, Li C, Liu Y, Jiang Y, Yu L, Liu M, et al. Differentiation of prostate cancer and benign prostatic hyperplasia: comparisons of the histogram analysis of intravoxel incoherent motion and monoexponential model with in-bore MR-guided biopsy as pathological reference. Abdom Radiol. (2019) 45:3265–77. doi: 10.1007/s00261-019-02227-5
35. Suh CH, Kim HS, Jung SC, Park JE, Choi CG, Kim SJ. MRI as a diagnostic biomarker for differentiating primary central nervous system lymphoma from glioblastoma: a systematic review and meta-analysis. J Magn Reson Imaging. (2019) 50:560–72. doi: 10.1002/jmri.26602
36. Mussurakis S, Buckley DL, Horsman A. Dynamic MR imaging of invasive breast cancer: correlation with tumour grade and other histological factors. Br J Radiol. (1997) 70:446–51. doi: 10.1259/bjr.70.833.9227224
37. Biesaga B, Niemiec J, Ziobro M. Microvessel density and status of p53 protein as potential prognostic factors for adjuvant anthracycline chemotherapy in retrospective analysis of early breast cancer patients group. Pathol Oncol Res. (2012) 18:949–60. doi: 10.1007/s12253-012-9525-9
Keywords: breast tumors, post-test probability, intravoxel incoherent motion diffusion-weighted imaging, differential diagnosis, meta-analysis
Citation: Liang J, Zeng S, Li Z, Kong Y, Meng T, Zhou C, Chen J, Wu Y and He N (2020) Intravoxel Incoherent Motion Diffusion-Weighted Imaging for Quantitative Differentiation of Breast Tumors: A Meta-Analysis. Front. Oncol. 10:585486. doi: 10.3389/fonc.2020.585486
Received: 20 July 2020; Accepted: 14 September 2020;
Published: 20 October 2020.
Edited by:
Sebastian Cerdan, Consejo Superior de Investigaciones Científicas (CSIC), SpainReviewed by:
Eric Edward Sigmund, New York University, United StatesValeria Righi, University of Bologna, Italy
Copyright © 2020 Liang, Zeng, Li, Kong, Meng, Zhou, Chen, Wu and He. This is an open-access article distributed under the terms of the Creative Commons Attribution License (CC BY). The use, distribution or reproduction in other forums is permitted, provided the original author(s) and the copyright owner(s) are credited and that the original publication in this journal is cited, in accordance with accepted academic practice. No use, distribution or reproduction is permitted which does not comply with these terms.
*Correspondence: Ni He, heni@sysucc.org.cn; YaoPan Wu, wuyp@sysucc.org.cn
†These authors have contributed equally to this work