- 1Computational Genomics Division, National Institute of Genomic Medicine, Mexico City, Mexico
- 2Centro de Ciencias de la Complejidad, Universidad Nacional Autónoma de México, Mexico City, Mexico
Breast cancer is a major public health problem which treatment needs new pharmacological options. In the last decades, during the postgenomic era new theoretical and technological tools that give us novel and promising ways to address these problems have emerged. In this work, we integrate several tools that exploit disease-specific experimental transcriptomic results in addition to information from biological and pharmacological data bases obtaining a contextual prioritization of pathways and drugs in breast cancer subtypes. The usefulness of these results should be evaluated in terms of drug repurposing in each breast cancer molecular subtype therapy. In favor of breast cancer patients, this methodology could be further developed to provide personalized treatment schemes. The latter are particularly needed in those breast cancer subtypes with limited therapeutic options or those who have developed resistance to the current pharmacological schemes.
1. Introduction
Breast cancer is a major public health concern and a main cause of death in young women worldwide (Ferlay et al., 2015). Breast cancer is characterized by an heterogeneous nature at the histological, molecular, and systemic levels. To cope with this heterogeneity, multiple prognostic and therapeutic approaches have been developed to handle this disease. From classifications based on clinical parameters or histopathologic markers (for example estrogen receptor[ER], progesterone receptor [PR], and epidermic human growth factor receptor [HER2]) (Prat et al., 2015) to molecular classifications based on gene expression values of the samples, such is the case of PAM50 classification (Parker et al., 2009).
The four breast cancer intrinsic subtypes (Luminal A, Luminal B, Basal, and Her2-enriched) defined by PAM50 offer more accurate clinical information than the classifications based on histopathologic parameters (Prat et al., 2015). The predictive power of PAM50 regarding patient prognosis is an example of this increase in accuracy (Prat et al., 2014, 2015). Furthermore, the new breast cancer international guidelines (Coates et al., 2015; Senkus et al., 2015) not only mention the utility of PAM50 intrinsic subtypes, but the latter states that they are indeed a central piece of the therapeutic and classification algorithms.
It is worth mentioning that the scarcity of effective therapeutic options, in particular for certain subtypes—such as basal, or triple-negative tumors—still present a challenge for clinicians who often have to resort to highly unspecific cytotoxic therapies.
In order to help solve this problem, theoretical and methodological advances are aimed at the development of improved therapeutic options firmly based on a deeper biological understanding of the disease. Three of these advances are particularly important in our view.
First, high throughput technologies capable of retrieve a huge amount of biological data; for example, expression microarrays or RNAseq which allow us to approach the study of the transcriptome, i.e., the set of RNA molecules present in biological entities. Second, The conception that generally speaking, a biological function of the living cell is a result of many interacting molecules; It can not be attributed to just a single gene or a single molecule. The above can be represented as a set of genes in the genome linked through a network of interacting molecules in the cell, such as a “Pathway,” representing a higher order biological function (Kanehisa and Goto, 2000). Third, the development of areas such as pharmacogenomics, which try to analyze by means of a global approach all the genes involved in the response to a certain drug in a variety of conditions (Pirmohamed, 2001). Such pharmacogenomics approach has evolved from a view centered on isolated genes to one based on pathways (García-Campos et al., 2015). Pharmacogenomics has progressively adopt a variety of high throughput technologies including genomics, transcriptomics, proteomics, and others, to enhance its capacity for generating and testing hypotheses and transfer these hypotheses to the clinical practice (Wang, 2010).
Despite these advances, for many breast cancer patients prognosis remains poor for both survival and quality of life (which is further affected by side effects of the pharmacological treatment itself). New, more effective and less harmful therapeutic options are needed, especially for certain subgroups of breast cancer patients, for example, those who suffer from basal subtype or from a tumor which develop resistance to usual therapeutic schemes (Prat et al., 2014; Yu and Jones, 2016; Friese et al., 2017). Some causes of this deficit of therapeutic options are the high economic and logistic costs involved, not only in traditional drug development (i.e., the path from concept to drug's approval), but also in the clinical evaluation of its usefulness in diseases for which the drug was not initially conceived, or drug repurposing assessment. In both cases, a more accurate selection of pharmacological targets is crucial to achieve viability and high rate of success. For all of the aforementioned reasons, a main goal of the present study is to answer the question: is it possible to identify new associations between pharmacological targets and their respective pathways to each breast cancer molecular subtype? In order to answer this, we propose a computational analysis based on the systematic inquiry of all relevant deregulated pathways specific for each breast cancer subtype, and the assessment of target genes (belonging to the aforementioned pathways), which are susceptible to pharmacological modulation. This approach relies on large, well-curated gene expression datasets from high throughput technologies coming from the two flagship projects (TCGA and METABRIC) of transcriptomic characterization of mammary tumors. Such projects are hence the gold standard reference in terms of quality and quantity of samples. Adding to this, the use of state of the art computational methods will allow us to develop a reliable, trustworthy study that supports the results that will be presented.
2. Materials and Methods
2.1. Outline
The analysis pipeline followed in the present work is depicted in Figure 1. Briefly, we obtained two data sets of gene expression experiments, one data set came from METABRIC (Curtis et al., 2012) repository and the other one from TCGA (Ciriello et al., 2015). Each data set was build up trough different technologies, namely Microarrays (METABRIC) and RNAseq (TCGA). Then, the samples were classified in its respective molecular breast cancer subtype according the PAM50 algorithm (Parker et al., 2009). After that, we identified the most deregulated pathways in each breast cancer subtype through Pathifier algorithm (Drier et al., 2013). Each deregulated molecular pathway was associated to its known pharmacological targets according to information from pharmacological databases. Finally, these drug-subtype relations were classified according to information available in both the pharmacological databases as well as information from the gene expression data of the samples themselves. Supported by these analyses we develop a Database Based Drug Repurposing (DBDR) method that allows for the implementation of precision medicine approaches, that may be applied even at the individual (personalized medicine) level.
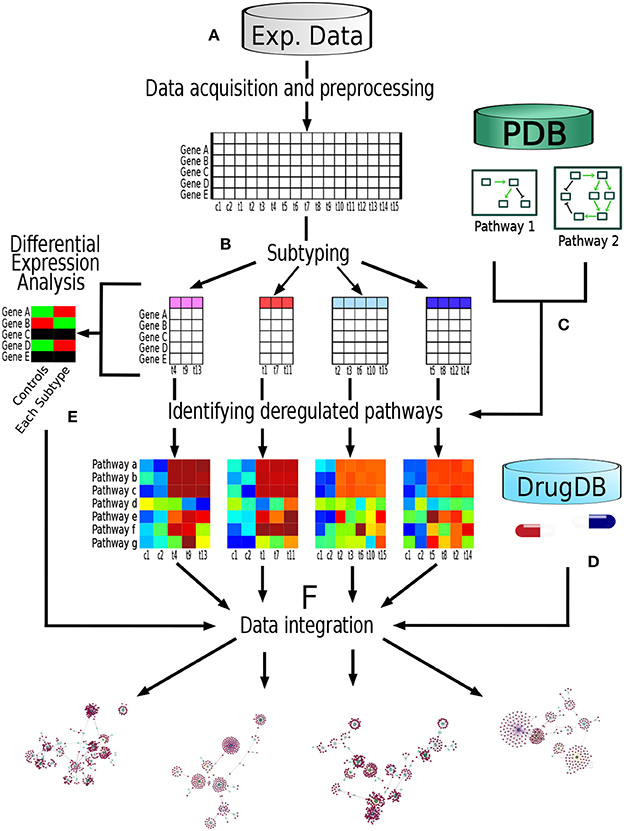
Figure 1. Pipeline performed in this study: (A) We obtained two data sets of gene expression experiments, one data set from METABRIC (Microarrays) and the other one from TCGA (RNAseq). Each dataset was analyzed independently using this pipeline. (B) Samples were classified in their respective molecular breast cancer subtype according the PAM50 algorithm. (C) From Pathway databases (PDB) we identified the most deregulated pathways in each breast cancer subtype through Pathifier algorithm. (D) For each pathway, genes known as pharmacological targets were identified, as well as the nature of said drug-target interaction according to pharmacological databases (DrugDB). (E) Differential expression analysis was performed comparing each molecular subtype against control samples. (F) Finally, information regarding the aforementioned steps was integrated.
2.2. Data Sources
As already discussed, our analysis is supported with information coming from the largest, more reliable whole-genome breast cancer gene expression datasets available, the ones provided by the METABRIC and TCGA consortia, respectively. We will briefly describe those datasets—at the level used here—. For more detailed information, please consult the original sources as referenced.
METABRIC (Molecular Taxonomy of Breast International Consortium) is a collection of over 2,000 clinically annotated primary fresh-frozen breast cancer specimens from tumor banks in the UK and Canada, more details about those data can be found in Curtis et al. (2012). To minimize batch effects, we only use the “discovery set” consisting in expression data from 997 samples of women with breast cancer and the “control set” a set of expression data from 144 samples from adjacent normal breast tissue.
Data were provided under request through the following download platform:
https://www.ebi.ac.uk/ega/about/your_EGA_account/download_streaming_client.
TCGA (The Cancer Genome Atlas) is a specialized database that stores high throughput technology data of at least 33 types of breast cancer obtained from more than eleven thousand patients. This big database is a reference point in terms of quality and sample size. We used the data set generated and published by Ciriello et al. (2015) which come from 808 biopsies of women with breast cancer and 112 from adjacent normal breast tissue.
Data were downloaded with their respective clinical information from the following internet address:
http://www.cbioportal.org/study?id=brca_tcga_pub2015#summary.
2.3. Preprocessing
In METABRIC Each RNA transcriptional profiling was extracted through the Illumina HT-12 v3 microarray platform. The consortium provides the data in the form of preprocessed expression matrices. The detailed description of the preprocessing performed in the data can be found in the original publication (Curtis et al., 2012).
Similarly to the previous case, the data used for TCGA are provided as preprocessed expression matrices. Details about data preprocessing could be found in the original publication (Wilkerson et al., 2014). Briefly (As reported by the author), RNA sequencing was performed on Illumina HiSeq. Resulting sequencing reads were aligned to the human hg19 genome assembly using MapSlice (Wang et al., 2010). Gene expression was quantified for the transcript models corresponding to the TCGA GAF 2.13 using RSEM4 and normalized within samples to a fixed upper quartile. Upper quartile normalized RSEM data were log2 transformed.
2.4. Subtyping
Samples were classified through PAM50 (Parker et al., 2009) algorithm by METABRIC and TCGA consortiums themselves. The proportions of samples per subtype inside each database are shown in Table 1.
2.5. Functional Enrichment Analysis
To identify the most deregulated molecular pathways we use Pathifier (Drier et al., 2013). Pathifier is an algorithm that integrates experimental data from high throughput technologies and pathway information from biological databases (Supplementary Material).
Pathifier provides as a result a deregulation value for each molecular pathway in each sample. This deregulation value is called “Pathway Deregulation Score” or PDS. This methodology has been used previously to observe deregulated pathways in breast cancer samples from TCGA (Drago-García et al., 2017). Importantly, Pathifier assigns a PDS for each individual sample, which in our case is a fundamental feature for choosing this method to assess the deregulation level for pathways of each breast cancer subtype.
In our case we use the molecular pathways defined by KEGG (Kanehisa and Goto, 2000) and the analysis was performed independently in each database (METABRIC and TCGA) for each tumor subgroup of breast cancer comparing it against its corresponding control group. Figure 2 shows the result of the calculation of Pathifier for the basal subtype of METABRIC against its respective controls.
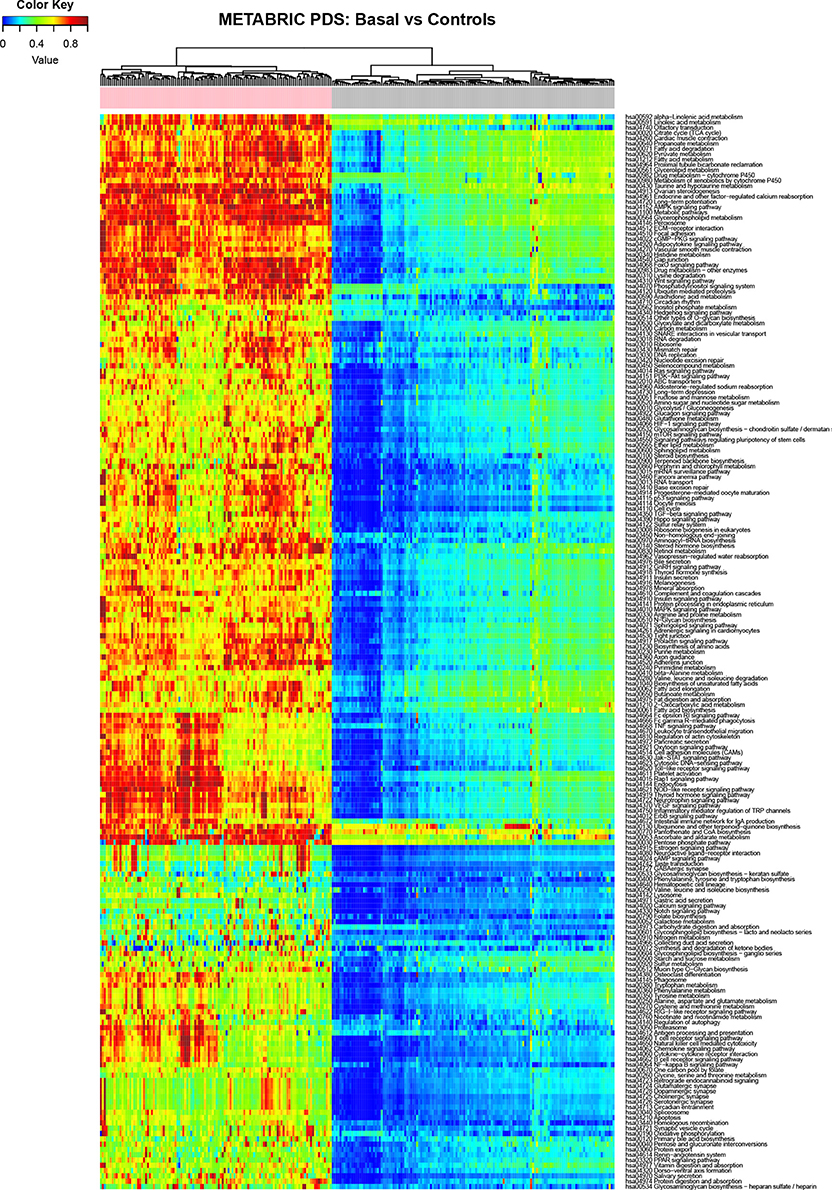
Figure 2. Heatmap depicting the PDS for the Basal subtype (basal samples are marked with a pink label) and normal breast tissue (gray labels) in the METABRIC study, rows correspond to molecular pathways and columns are individual samples.
In order to identify the most deregulated pathways by subtype we performed a z-score transformation over the PDS that belonged to each pathway(row). This transformation allow us to compare the deregulation scores (called “PDSz” here after) between different molecular pathways as described by the author of the method (Livshits et al., 2015). We looked for those pathways whose patients exhibited the highest PDSz consistently in both databases. This was done as follows: the algorithm returns “n” PDSz values (one PDSz per each patient) for a given pathway. We used the median thus obtaining only one deregulation value per molecular pathway in each database. then we sort the values and got the intersection in METABRIC and TCGA data of top 30 most deregulated pathways. Results are shown in Table 2.
2.6. Drug Target Associations
The aim of this procedure was to identify which of the studied genes (in the broad sense of the word) could be considered as pharmacological targets by virtue of a drug acting upon it, according to pharmacological databases. Drug-target associations were identified through a tool known as The Drug-Gene Interaction Database (www.dgidb.org). This platform integrates information of at least 15 pharmacological databases which include information about drugs, pharmacological targets, type of drug-target interaction, data sources, and other characteristics. That tool was used through its implementation as bioconductor package which name is RDGIdb (Wagner et al., 2015).
2.7. Prioritization of Pharmacological Targets
Drugs were classified according to the following criteria. First, if there was a molecular pathway with which the drug interacts—i.e., if the drug has any kind of physicochemical effect in at least one of the pathway's elements, having a noticeable effect in the pathway itself—. Second, if that molecular pathway resulted highly and consistently deregulated in a breast cancer molecular subtype (as it is found by applying pathway deregulation calculations in both gene expression datasets under study). Third, if the pharmacological target gene was differentially expressed in that breast cancer subtype in relation to healthy controls. Fourth, depending the type of interaction of the drug with the pharmacological target, in particular whether the effect of the drug (a) leads to a return of the target gene basal expression level (for presentation purposes,we will call homeostasis), (b) given an overexpressed/underexpressed target, leads to a higher overexpression/underexpression (anti-homeostasis), or (c) leads to an undetermined behavior of the target.
2.8. Differential Expression Analysis
Differential expression analysis is a procedure which allows to identify if the same gene holds different expression levels with respect to two conditions (in our case a breast cancer subtype compared to adjacent normal breast tissue). In this study, differential expression analysis was made through the limma (Ritchie et al., 2015) bioconductor (Gentleman et al., 2004) package for the case of METABRIC data and with DeSeq2 (Love et al., 2014) in the case of TCGA data. Threshold parameters used for those analysis were a absolute log fold chance (base 2) >1, a Benjamini-Hochberg adjusted p-value lesser than 0.001 and a FDR lesser than 0.1. All the code was developed using the Emacs speak statistics package (Rossini et al., 2004)
2.9. Individualized Proof-Of-Concept
Since Pathifier provides a PDS per sample, the deregulation of any given pathway is different for each patient. The final section is composed by two examples of deregulated pathways in two patients with basal subtype. There we show the PDS for their most deregulated pathways, the genes which are susceptible to pharmacological modulation, and also the drugs that modulate these targets and concomitantly, the associated pathways, paying special attention to those drugs that have a mechanism of action of direct and selective interaction with the identified target, thus reducing the undesired side effects.
3. Results
3.1. Deregulated Pathways by Breast Cancer Subtype
3.1.1. Deregulated Pathways Were Consistent Across Different Technologies
The deregulated pathways by subtype were consistent across different technologies. Unsupervised hierarchical clustering was performed to see if the set of pathway deregulation levels related to one particular breast cancer subtype was consistent with the subtype itself or instead it was consistent with the technology where the data come from. Before carrying out this procedure, were calculated the PDSz (the level of deregulation associated to each pathway in each tumoral subtype) as described in section Materials and Methods. Thus, there were eight sets of PDSz, considering four tumoral subtypes and two different data sources. After that, unsupervised hierarchical clustering was applied. The result of this analysis is shown in Figure 3, where it can be seen that data do not group according to the technology by which they were obtained, instead they group according to the biological condition from which they come.
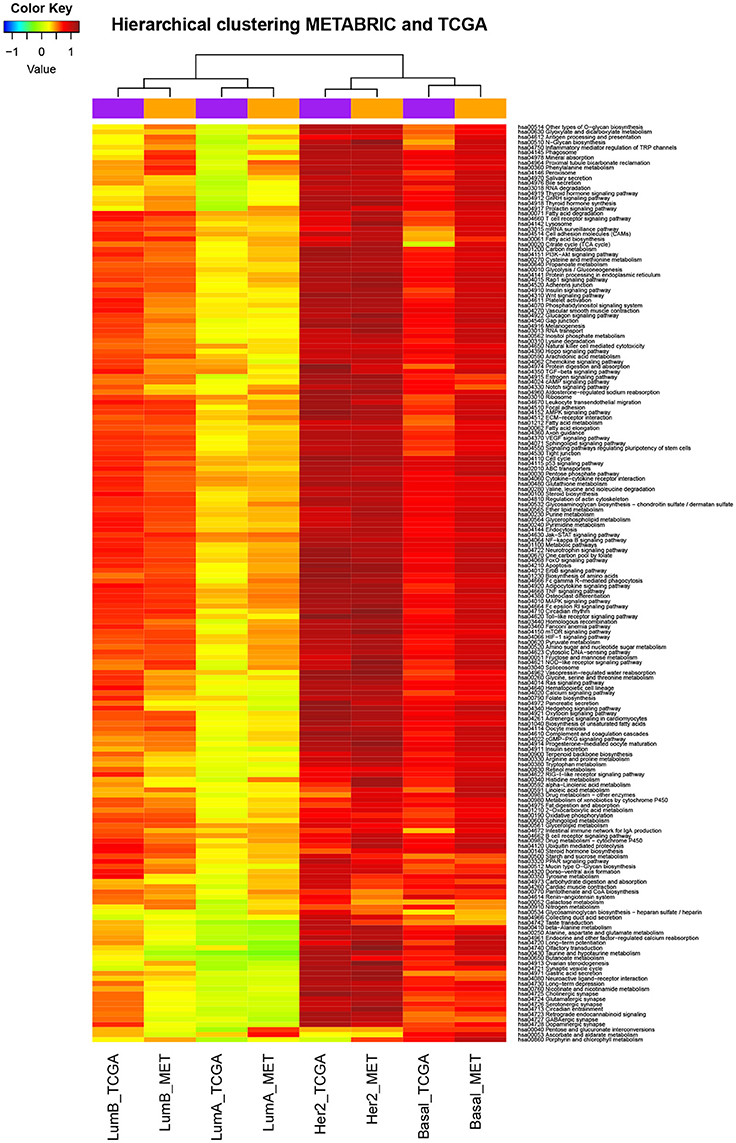
Figure 3. Deregulated pathways were consistent across different technologies. The data are the level of deregulation associated to each pathway in each breast cancer subtype (as is described more detailed in functional enrichment analysis subsection). Color scale corresponds to the level of deregulation in each pathway where red is very deregulated and blue is little deregulated. Rows correspond to KEGG molecular pathways, columns to subtypes of breast cancer according to the database of provenance (MET means METABRIC). We can observe that the breast cancer subtypes traditionally considered as more aggressive have higher levels of deregulation. Also we can seen that the data (sets of PDSz) do not group according to the technology by which they were obtained, instead they group according to the biological condition from which they come.
3.1.2. Agreement Between the Most Deregulated Pathways
For each tumoral subtype the 30 pathways with higher deregulation score were taken and then we selected the ones which coincided in both databases (from now on called the most deregulated pathways by breast cancer subtype). These are mentioned in Table 2.
Finally, information regarding the deregulation value of the pathway associated to the pharmacological target for the breast cancer subtype, the differential gene expression status of the pharmacological target, type of interaction between drug and pharmacological target, the relationship between the type of drug-target interaction and the differential expression status of the latter, is presented in Supplementary Material.
A brief example of the above mentioned database is provided in Table 3. Columns show several criteria by means of which the pharmacological targets were classified: Drug associated to the pharmacological target, Gene associated to the target, Pathway to the molecular pathway which pharmacological target belongs to. logFC refers to the logarithmic differential expression ratio of the pharmacological target gene in the breast cancer subtype relative to its controls. InteractionType to the type of interaction between the drug and the pharmacological target. Homeostasis is a term determined according to the differential expression status of the pharmacological target gene and the type of drug-target interaction. Finally, Source refers to the database from which the information was obtained.
3.2. Pharmacological Targets Are Associated to Specific Molecular Subtypes
Pharmacological target were selected from the pathways that are the most deregulated for a specific subtype of breast cancer and also coincide in both databases. Additionally, the target has to be differentially expressed.
The selected pathways and the number of associated pharmacological targets are shown in Table 4. There, rows are the most deregulated molecular pathways in both databases by breast cancer subtype as shown in Table 2. Columns are PAM50 breast cancer subtypes and number of pharmacological targets that are also differentially expressed in each subtype of breast cancer.
We can notice that there are many more pharmacological targets associated to each molecular subtype than those currently used for their clinical treatment. In Figure 4 we can see a multipartite network of pathways-targets-drugs for basal tumors. A number of potential therapies (not currently used) is evident, as is the redundancy of the network, which could be indicative for flexible therapeutic approaches.
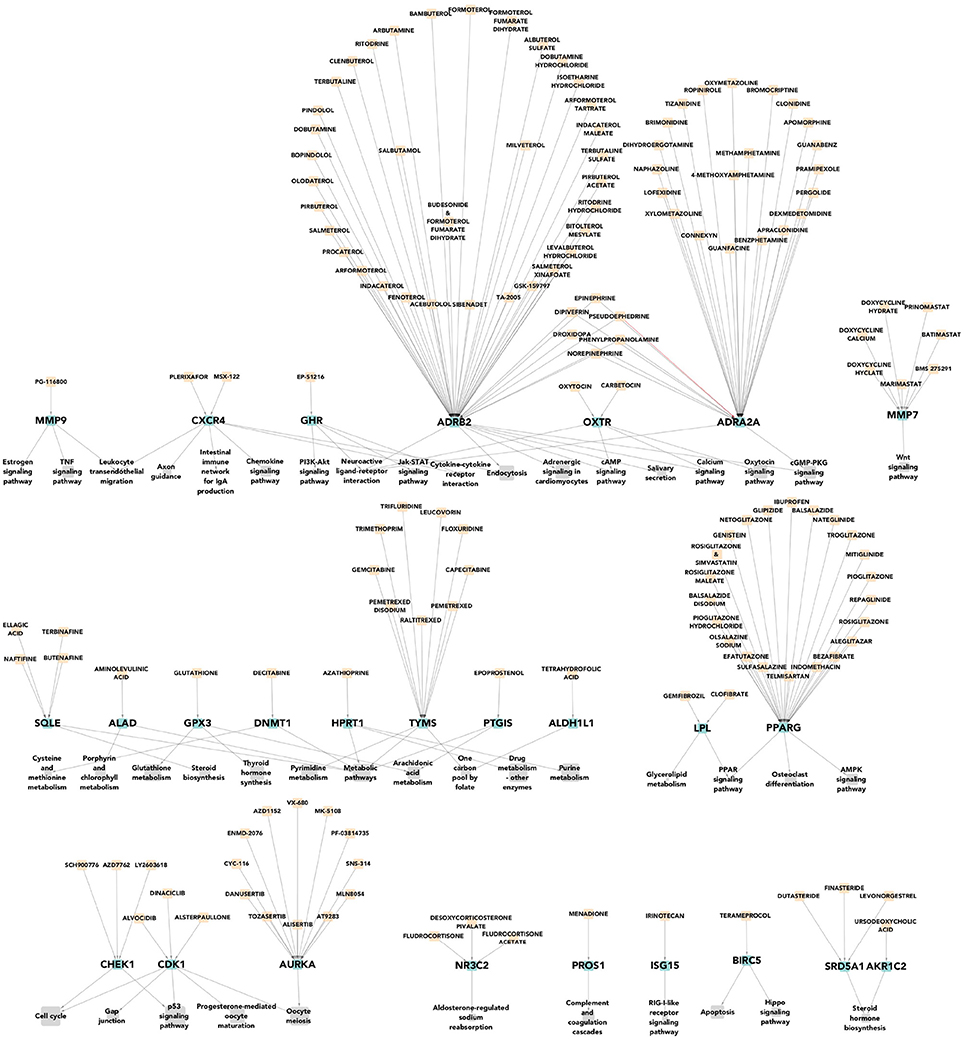
Figure 4. Pharmacological targets of the most deregulated pathways for basal breast cancer subtype. In gray the most deregulated molecular pathways by PDS are shown. In green color, we represent the differentially expressed pharmacological targets that had a drug which lead to homeostasis. Finally, in apricot color we show the drugs that affect expression of such pharmacological targets. We can observe that there are many more pharmacological targets associated to this specific tumoral subtype than those currently used for their clinical treatment.
Seventy-nine drugs leading to homeostasis over eight highly deregulated pathways have been found for the Basal breast cancer subtype. This acquires particular relevance since this subtype is closely associated to triple negative tumors, for which directed (i.e., non-purely cytotoxic) pharmacological therapies are scarce.
The fact that a high number of drugs already in the market (i.e., after passing phase I, II, and III clinical trials and receiving legal approval) are able to target directly some of the most deregulated pathways in this hard-to-treat tumors points out to the actual scope of pathway-based drug repurposing approaches. A further application of these findings on the road to personalized medicine is shown in the Discussion section.
4. Discussion
Breast cancer is a public health problem which treatment require new pharmacological options. In the present study, For each breast cancer molecular subtype we looked for deregulated pathways that are pharmacologically modulable and are not currently used in the corresponding breast cancer subtype therapy. We have identified new pharmacological targets (and their respective pathways) that are potentially useful in breast cancer subtypes treatment.
Significant amount of work has been dedicated to the discovery of novel drug-disease associations. Previous efforts have been made to associate drug response with gene signatures (Lamb et al., 2006; Lee, 2017). However, those tools were designed to be used in cell lines, their performance in real human cells has not been explored. Furthermore, those approaches were built up with a relatively small number of drugs, and they were not built specifically in the context of breast cancer.
With this in mind, we performed a data-driven approach to the actual phenomenology of the different breast cancer subtypes as represented by their distinctive gene expression patterns, to look up for sets of patient-specific deregulated pathways, then by resorting to molecular and drug databases. We designed a methodology to find out druggable targets whose associated drugs were already in the market, i.e., we built a drug repositioning strategy.
4.1. New Relations Between Pathways and Subtypes
Table 2 shows the set of the highly deregulated molecular pathways associated to each subtype of breast cancer. The description of how the associations were made is discussed in the section Materials and Methods, as well as in the Results section. Some of the associations found in the present study between breast cancer subtypes and molecular pathways are already known and intensively studied, in fact, there are pharmacological targets belonging to these molecular pathways which are currently employed for the treatment of the corresponding breast cancer subtype. Some of these relationships will be mentioned below.
With regard to Her2-enriched subtype, in our study, it is associated to the ErbB signaling pathway one of the most studied pathways for this subtype (the name of this subtype of breast cancer is due to the relationship with this pathway). The ErbB2 receptor is the mainstay of pharmacological treatment in patients with the Her2-enriched breast cancer subtype (Senkus et al., 2015). In the present study we also associate the Her2-enriched subtype with the molecular pathway of apoptosis and DNA replication, where it has already been described in the literature to detail how the overexpression of Her2 leads to uncontrolled cell proliferation and suppression of apoptosis (Carpenter and Lo, 2013).
Regarding the basal subtype, its association with the molecular pathways of apoptosis and cell cycle is not new; the increase in cell proliferation and apoptosis is a well-known characteristic of this subtype (Choo and Nielsen, 2010). Moreover, lapatinib (a Her2 inhibitor) has been shown to be useful in treatment when combined with other agents in breast cancer models of the basal phenotype (Liu et al., 2011), therefore the association of this molecular subtype with Her2 signaling pathway is not entirely new.
Although several associations found in the present study between molecular pathways and subtypes of breast cancer are under intense study, here we propose novel associations that do not have been previously reported, based on the present study. This strategy results promising to evaluate their usefulness in generating knowledge regarding breast cancer treatment.
4.2. New Relations Between Pharmacological Targets and Subtypes
We found different pharmacological targets associated to each breast cancer subtype. The number of pharmacological targets associated to each breast cancer subtype is shown in Table 4 and how they were associated is described in the Results section. In that table we can note that even in the cases where two breast cancer subtypes share the same deregulated molecular pathway, the number of differentially expressed pharmacological target genes is different. By observing Table 4 we see that in the molecular subtype Her2-enriched the molecular pathway “ErbB signaling” has five deregulated pharmacological targets. These are shown in detail in Figure 5. However only one of these five pharmacological targets is currently indicated in the clinical practice guidelines for breast cancer: ErbB2.
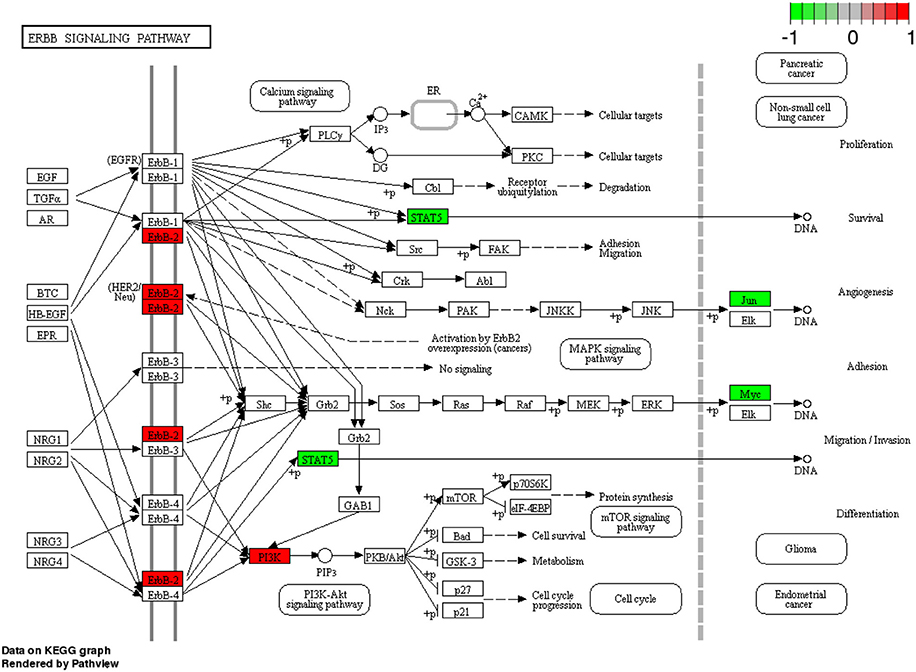
Figure 5. Pharmacological targets of ErbB2 signaling pathway in Her2-enriched subtype. The figure shows the five differentially expressed pharmacological targets (more than those currently exploited in the clinic) obtained with our approach.
Currently, 1–5 pharmacological targets are typically used in clinic, depending on the molecular subtype of the patient. In this work we show that more than a dozen pharmacological targets are associated to each breast cancer subtype. Therefore, a subsequent evaluation of these new pharmacological targets may lead to some new therapeutic options, needed in the clinic environment. Finally, it is worth to mention that each pharmacological target may have several (up to dozens) drugs or chemical compounds that exert actions over it. Such condition amplifies the ways to modulate the therapeutic effect.
4.3. DBDR in the Age of Personalized Medicine
In what follows we will present, as a proof-of-concept, an application of the DBDR approach to two individual breast cancer patients (within our datasets) with known gene expression profiles. Based on such expression profile, specific molecular subtype and main deregulated pathways can be known.
We decided to focus these examples in the basal subtype, since the scarcity of non-cytotoxic therapeutic options and the poor prognosis of patients having this phenotype. In the two following boxes we show the deregulated pathway with their respective PDS, their associated target genes, and the drugs that lead to homeostasis of said pathways. Each proposed drug set was checked so that all of the drug pairs do not have any known drug interaction. Finally we discussed a personalized set of drugs for each individual.
4.3.1. Individual Therapy Designed for Patient [MB.3058]
This is a patient classified as having a basal tumor with the following deregulated pathways:
In view of this particular set of deregulated pathways and gene expression patterns, individualized therapy for this patient may include the following drugs: TERBINAFINE, PEMETREXED, or CAPECITABINE, and SKI-1. Alvocidib, paclitaxel, alisertib y dinaciclib are not considered since the low PDS in their respective pathways.
4.3.2. Individual Therapy Designed for Patient [MB.5387]
This is a patient classified as having a basal tumor with the following deregulated pathways:
In view of this particular set of deregulated pathways and gene expression patterns, individualized therapy for this patient may include the following drugs: TERBINAFINE, PEMETREXED, or CAPECITABINE, SK1-I, ALVOCIDIB, or PACLITAXEL, ALISERTIB, and DINACICLIB.
4.4. Scope and Limitations
A systems biology approach such as the one presented here is aimed at primary discovery and hypotheses generation. As such, there is a need to test the findings obtained here—in the context of the pharmacogenomics and lifestyles of actual patients—before they can be fully beneficial in a general clinical practice.
Considering the previous comments, we cannot stress enough that this theoretical approach is still far from constituting an actual aid to the clinician. For this reason, no further considerations on dose, drug combinations and patient features (other than tumor subtype, gene expression profile and deregulated pathways) are being considered.
5. Conclusions
New molecular pathways and their respective pharmacological targets of potential therapeutic utility were associated to each of the different breast cancer molecular subtypes. This allows subsequent focused assessment of the pharmacological targets associated to each subtype in order to find new therapeutic options for the treatment of patients suffering from this disease. The knowledge derived from this approach will result particularly useful for those patients that have developed pharmacological resistance to canonical treatment and/or suffer from tumors with reduced therapeutical options, such as basal or triple-negative breast cancers.
Here we present an application of pathway-based analyses based on the detailed study of two of the largest and more trustworthy international cohorts, including whole genome high quality transcriptomic data, coming from either RNASeq or microarray technologies: The METABRIC and TCGA collaborations. By analyzing high throughput gene expression data for thousands of patients, using a robust probabilistic approach to find the most deregulated pathways for each subtype (even at the per-patient level) and assessing the findings by crossing-out the information with highly curated knowledge-based drug target databases, we have been able to develop a methodology that allows for a detailed drug-repurposing scheme to treat the different breast cancer tumor types and that, in principle (as an introductory proof-of concept) may even be extended up to the single patient design, thus paving the way to personalized medicine to treat such complex pathologies as breast cancer using a pragmatic drug re-use approach.
Hopefully, the work presented here will contribute to save considerable amounts of financial resources—by resorting to drug repurposing strategies—, but above all, may soon allow us to save more and more lives of people suffering from this excruciating disease.
Author Contributions
RM-P carried out analysis and calculations, devised code, and database management duties and contributed to the writing of the manuscript. JE-E contributed to the discussion, reviewed analysis and contributed to the writing of the manuscript. EH-L designed and coordinated the project, contributed to the discussion, supervised, and reviewed the writing of the manuscript. All authors read and approved the final manuscript.
Funding
RM-P is a doctoral student from Programa de Doctorado en Ciencias Biomédicas, Universidad Nacional Autónoma de México (UNAM) and received fellowship 419866 from CONACYT. This work was supported by CONACYT (grant no. 285544/2016), as well as by federal funding from the National Institute of Genomic Medicine (Mexico). Additional support has been granted by the Laboratorio Nacional de Ciencias de la Complejidad (grant no. 232647/2014 CONACYT). EH-L is recipient of the 2016 Marcos Moshinsky Fellowship in the Physical Sciences.
Conflict of Interest Statement
The authors declare that the research was conducted in the absence of any commercial or financial relationships that could be construed as a potential conflict of interest.
Acknowledgments
Authors also thank to Miguel Ángel García-Campos from Weizman Institute of Sciences and Guillermo de Anda-Jáuregui from University of North Dakota for their valuable support and comments.
Supplementary Material
The Supplementary Material for this article can be found online at: https://www.frontiersin.org/articles/10.3389/fphar.2018.00905/full#supplementary-material
References
Carpenter, R. L., and Lo, H.-W. (2013). Regulation of apoptosis by her2 in breast cancer. J. Carcinog. Mutagen. 2013(Suppl. 7):003. doi: 10.4172/2157-2518.S7-003
Choo, J. R., and Nielsen, T. O. (2010). Biomarkers for basal-like breast cancer. Cancers 2, 1040–1065. doi: 10.3390/cancers2021040
Ciriello, G., Gatza, M. L., Beck, A. H., Wilkerson, M. D., Rhie, S. K., Pastore, A., et al. (2015). Comprehensive molecular portraits of invasive lobular breast cancer. Cell 163, 506–519. doi: 10.1016/j.cell.2015.09.033
Coates, A. S., Winer, E. P., Goldhirsch, A., Gelber, R. D., Gnant, M., Piccart-Gebhart, M., et al. (2015). Tailoring therapies–improving the management of early breast cancer: St gallen international expert consensus on the primary therapy of early breast cancer 2015. Ann. Oncol. 26, 1533–1546. doi: 10.1093/annonc/mdv221
Curtis, C., Shah, S. P., Chin, S.-F., Turashvili, G., Rueda, O. M., Dunning, M. J., et al. (2012). The genomic and transcriptomic architecture of 2,000 breast tumours reveals novel subgroups. Nature 486, 346–352. doi: 10.1038/nature10983
Drago-García, D., Espinal-Enríquez, J., and Hernández-Lemus, E. (2017). Network analysis of emt and met micro-rna regulation in breast cancer. Sci. Rep. 7:13534. doi: 10.1038/s41598-017-13903-1
Drier, Y., Sheffer, M., and Domany, E. (2013). Pathway-based personalized analysis of cancer. Proc. Natl. Acad. Sci. U.S.A. 110, 6388–6393. doi: 10.1073/pnas.1219651110
Ferlay, J., Soerjomataram, I., Dikshit, R., Eser, S., Mathers, C., Rebelo, M., et al. (2015). Cancer incidence and mortality worldwide: sources, methods and major patterns in globocan 2012. Int. J. Cancer 136, E359–E386. doi: 10.1002/ijc.29210
Friese, C. R., Harrison, J. M., Janz, N. K., Jagsi, R., Morrow, M., Li, Y., et al. (2017). Treatment-associated toxicities reported by patients with early-stage invasive breast cancer. Cancer 123, 1925–1934. doi: 10.1002/cncr.30547
García-Campos, M. A., Espinal-Enríquez, J., and Hernández-Lemus, E. (2015). Pathway analysis: state of the art. Front. Physiol. 6:383. doi: 10.3389/fphys.2015.00383
Gentleman, R. C., Carey, V. J., Bates, D. M., Bolstad, B., Dettling, M., Dudoit, S., et al. (2004). Bioconductor: open software development for computational biology and bioinformatics. Genome Biol. 5:R80. doi: 10.1186/gb-2004-5-10-r80
Kanehisa, M., and Goto, S. (2000). Kegg: Kyoto encyclopedia of genes and genomes. Nucleic Acids Res. 28, 27–30. doi: 10.1093/nar/28.1.27
Lamb, J., Crawford, E. D., Peck, D., Modell, J. W., Blat, I. C., Wrobel, M. J., et al. (2006). The connectivity map: using gene-expression signatures to connect small molecules, genes, and disease. Science 313, 1929–1935. doi: 10.1126/science.1132939
Lee, G. (2017). Design and Fabrication of Multilayer Structures in Dynamic Sensing and Transparent Nanocomposite Scintillators for High Energy Detection. Ph.D. thesis, Georgia Institute of Technology.
Liu, T., Yacoub, R., Taliaferro-Smith, L. D., Sun, S.-Y., Graham, T. R., Dolan, R., et al. (2011). Combinatorial effects of lapatinib and rapamycin in triple-negative breast cancer cells. Mol. Cancer Ther. 10, 1460–1469. doi: 10.1158/1535-7163.MCT-10-0925
Livshits, A., Git, A., Fuks, G., Caldas, C., and Domany, E. (2015). Pathway-based personalized analysis of breast cancer expression data. Mol. Oncol. 9. 1471–1483. doi: 10.1016/j.molonc.2015.04.006
Love, M. I., Huber, W., and Anders, S. (2014). Moderated estimation of fold change and dispersion for RNA-seq data with DESeq2. Genome Biol. 15:550. doi: 10.1186/s13059-014-0550-8
Parker, J. S., Mullins, M., Cheang, M. C., Leung, S., Voduc, D., Vickery, T., et al. (2009). Supervised risk predictor of breast cancer based on intrinsic subtypes. J. Clin. Oncol. 27, 1160–1167. doi: 10.1200/JCO.2008.18.1370
Pirmohamed, M. (2001). Pharmacogenetics and pharmacogenomics. Br. J. Clin. Pharmacol. 52, 345–347. doi: 10.1046/j.0306-5251.2001.01498.x
Prat, A., Carey, L. A., Adamo, B., Vidal, M., Tabernero, J., Cortés, J., et al. (2014). Molecular features and survival outcomes of the intrinsic subtypes within her2-positive breast cancer. J. Natl. Cancer Inst. 106:dju152. doi: 10.1093/jnci/dju152
Prat, A., Pineda, E., Adamo, B., Galván, P., Fernández, A., Gaba, L., et al. (2015). Clinical implications of the intrinsic molecular subtypes of breast cancer. Breast 24, S26–S35. doi: 10.1016/j.breast.2015.07.008
Ritchie, M. E., Phipson, B., Wu, D., Hu, Y., Law, C. W., Shi, W., et al. (2015). limma powers differential expression analyses for rna-sequencing and microarray studies. Nucleic Acids Res. 43:e47. doi: 10.1093/nar/gkv007
Rossini, A. J., Heiberger, R. M., Sparapani, R. A., Maechler, M., and Hornik, K. (2004). Emacs speaks statistics: a multiplatform, multipackage development environment for statistical analysis. J. Comput. Graph. Stat. 13, 247–261. doi: 10.1198/1061860042985
Senkus, E., Kyriakides, S., Ohno, S., Penault-Llorca, F., Poortmans, P., Rutgers, E., et al. (2015). Primary breast cancer: Esmo clinical practice guidelines for diagnosis, treatment and follow-up. Ann. Oncol. 26(Suppl. 5), v8–v30. doi: 10.1093/annonc/mdv298
Wagner, A. H., Coffman, A. C., Ainscough, B. J., Spies, N. C., Skidmore, Z. L., Campbell, K. M., et al. (2015). DGIdb 2.0: mining clinically relevant drug–gene interactions. Nucleic Acids Res. 44, D1036–D1044. doi: 10.1093/nar/gkv1165
Wang, K., Singh, D., Zeng, Z., Coleman, S. J., Huang, Y., Savich, G. L., et al. (2010). Mapsplice: accurate mapping of rna-seq reads for splice junction discovery. Nucleic Acids Res. 38:e178. doi: 10.1093/nar/gkq622.
Wang, L. (2010). Pharmacogenomics: a systems approach. Wiley Interdiscp. Rev. Syst. Biol. Med. 2, 3–22. doi: 10.1002/wsbm.42
Wilkerson, M. D., Cabanski, C. R., Sun, W., Hoadley, K. A., Walter, V., Mose, L. E., et al. (2014). Integrated RNA and DNA sequencing improves mutation detection in low purity tumors. Nucleic Acids Res. 42:e107. doi: 10.1093/nar/gku489
Keywords: drug repositioning, breast neoplasms, systems biology, databases, genetic, pathway analysis, personalized medicine
Citation: Mejía-Pedroza RA, Espinal-Enríquez J and Hernández-Lemus E (2018) Pathway-Based Drug Repositioning for Breast Cancer Molecular Subtypes. Front. Pharmacol. 9:905. doi: 10.3389/fphar.2018.00905
Received: 27 April 2018; Accepted: 24 July 2018;
Published: 15 August 2018.
Edited by:
Zhi Sheng, Virginia Tech, United StatesReviewed by:
Vijay Pandey, Cancer Science Institute of Singapore, National University of Singapore, SingaporeRobin T. Varghese, Edward via College of Osteopathic Medicine, United States
Copyright © 2018 Mejía-Pedroza, Espinal-Enríquez and Hernández-Lemus. This is an open-access article distributed under the terms of the Creative Commons Attribution License (CC BY). The use, distribution or reproduction in other forums is permitted, provided the original author(s) and the copyright owner(s) are credited and that the original publication in this journal is cited, in accordance with accepted academic practice. No use, distribution or reproduction is permitted which does not comply with these terms.
*Correspondence: Enrique Hernández-Lemus, ehernandez@inmegen.gob.mx